The Intersection of AI and Social Listening: Identifying Trends in Real Time
Introduction: The Evolution of Market Intelligence in the Social Era
The digital landscape has fundamentally transformed how consumers express preferences, share experiences, and influence others. Each day, over 500 million tweets, 95 million Instagram posts, and billions of other social interactions create a vast, unstructured dataset of consumer sentiment and emerging trends. Traditional market research methodologies—focus groups, surveys, and periodic analyses—cannot capture this real-time stream of authentic consumer expression. Enter AI-powered social listening—the systematic use of artificial intelligence to monitor, analyze, and interpret social media data for actionable market intelligence. According to Gartner, organizations that effectively leverage social listening can identify emerging issues 72% faster than competitors relying on traditional feedback mechanisms. As marketing strategist David Meerman Scott notes, "The most successful companies today are the ones that have learned to operate in real time." This capability has become even more critical in an era of rapid preference shifts and viral trend propagation. This article examines how AI is transforming social listening from basic monitoring to predictive intelligence, its applications across industries, implementation challenges, and emerging trajectories in an increasingly dynamic marketplace where the ability to detect and respond to trends in real time represents a significant competitive advantage.
1. The Technological Evolution: From Monitoring to Predictive Intelligence
Social listening technologies have progressed through several generations of increasing sophistication:
a) From Keywords to Contextual Understanding
The journey from basic to nuanced social media analysis:
- Early systems relied on simple keyword counting and sentiment lexicons
- Modern approaches employ natural language processing for semantic understanding
- Advanced systems recognize sarcasm, metaphor, and cultural references
- Emerging capabilities include multimodal analysis of text, images, audio, and video
b) AI Algorithms and Analytical Approaches
Contemporary social listening leverages multiple specialized techniques:
- Machine learning models detecting pattern anomalies in conversation volume
- Neural networks identifying emerging language patterns and neologisms
- Transformer models understanding conversational context and thread relationships
- Reinforcement learning systems that continuously improve detection accuracy
c) From Retrospective Analysis to Predictive Modeling
The evolution from backward-looking to forward-looking insights:
- First-generation systems provided historical conversation summaries
- Current platforms offer real-time alerts and emerging topic identification
- Advanced solutions predict trend trajectory and potential impact
- Next-generation approaches simulate trend propagation across network types
2. Strategic Applications Across Marketing Functions
AI-powered social listening delivers value throughout the marketing ecosystem:
a) Product Development and Innovation
Professor Clayton Christensen's jobs-to-be-done framework finds new expression through social listening:
- Example: Unilever identifies unmet needs by analyzing complaint patterns across competitors
- Consumer electronics firms like Samsung detect feature requests in user communities
- Automotive companies monitor DIY modifications to identify aftermarket opportunities
b) Brand Health and Reputation Management
Real-time monitoring enables proactive brand protection:
- Example: Airbnb's crisis detection system identified emerging concerns about cleaning protocols during the pandemic
- Financial services firms monitor for early warning signs of trust issues
- Healthcare companies track adverse event mentions requiring regulatory reporting
c) Competitive Intelligence and Market Positioning
Social data provides unfiltered competitive insights:
- Example: Netflix analyzes viewer reactions to competitor content to inform acquisition decisions
- CPG companies track emerging flavor trends across food and beverage categories
- Retail brands monitor competitor promotions and their effectiveness
3. The Science of Trend Detection
Effective trend identification requires sophisticated analytical frameworks:
a) Diffusion Models and Network Effects
Marketing scientist Jonah Berger's contagion frameworks apply directly to trend analysis:
- Identification of high-influence nodes in conversation networks
- Detection of cross-platform migration patterns for emerging topics
- Velocity and acceleration metrics for trend trajectory prediction
- Critical mass thresholds indicating mainstream adoption potential
b) Semantic Drift and Linguistic Innovation
Language evolution provides early signals of cultural shifts:
- Tracking of semantic expansion as terms acquire new meanings
- Identification of linguistic recombination creating new concepts
- Detection of code-switching between consumer segments
- Measurement of sentiment evolution around established concepts
c) From Detection to Attribution and Valuation
Advanced systems connect trends to business outcomes:
- Correlation of social signals with sales and revenue impact
- Attribution modeling for organic versus promoted conversation
- Competitive share of voice analysis and its business implications
- Predictive modeling of trend lifespan and commercial potential
4. Case Studies: AI-Powered Social Listening in Action
Several organizations demonstrate strategic implementation:
a) PepsiCo's Trend Prediction Engine
PepsiCo developed an AI-powered system analyzing millions of social conversations to predict flavor trends 12-18 months before mainstream adoption, directly informing R&D priorities and reducing failed product launches by 25%.
b) Adidas's Real-Time Marketing Adaptation
Adidas implemented social listening AI that detects emerging micro-trends in streetwear culture, enabling their marketing team to adjust campaign messaging and visual assets within hours rather than weeks, resulting in 40% higher engagement rates.
c) Delta Airlines' Service Recovery Protocol
Delta deployed sentiment analysis across social channels to identify emotionally charged customer interactions in real-time, enabling proactive service recovery that improved customer retention by 18% for affected travelers.
5. Implementation Challenges and Success Factors
Several obstacles affect social listening implementation:
a) Data Access and Representation Challenges
Comprehensive trend detection faces technical limitations:
- API restrictions limiting data accessibility from major platforms
- Demographic skew in social media usage requiring calibration
- Private messaging migration reducing visible conversation volume
- Geographic and linguistic variations in platform adoption
b) Signal-to-Noise Ratio Optimization
Extracting meaningful signals requires sophisticated filtering:
- Disambiguation techniques for polysemous terms and concepts
- Bot and inauthentic activity detection and filtering
- Proportional representation adjustment for platform demographics
- Controlled vocabulary mapping for consistent trend tracking
c) Organizational Integration and Action Protocols
Technical insights must translate to operational responsiveness:
- Cross-functional workflows for insight dissemination
- Decision frameworks for trend validation and response
- Speed-versus-accuracy balancing in opportunity assessment
- Cultural adaptation to real-time versus campaign-based thinking
Conclusion: The Future of AI-Enhanced Market Intelligence
The intersection of AI and social listening represents a fundamental shift in market intelligence—moving from periodic structured research to continuous unstructured insight generation. As renowned marketing professor Philip Kotler observes, "The art of marketing is the art of brand building. If you are not a brand, you are a commodity." AI-powered social listening enables this brand building through unprecedented market awareness, allowing organizations to detect emerging opportunities, threats, and cultural shifts before competitors. As these technologies continue to evolve, we can expect increasing integration with other data sources—from search behavior to purchase data to offline conversations—creating a comprehensive digital nervous system for the organization. Companies that master this capability gain significant advantages in consumer relevance, operational agility, and strategic foresight in markets increasingly defined by rapid change and fleeting competitive advantages.
Call to Action
For marketing leaders seeking to enhance social listening capabilities:
- Audit existing social monitoring approaches to identify gaps in real-time insight generation
- Develop clear use cases and success metrics aligned with strategic business priorities
- Implement cross-functional response protocols before deploying advanced detection systems
- Build expertise in both AI technology and cultural trend interpretation
- Establish ethical guidelines addressing privacy and responsible use of consumer conversation data
Featured Blogs
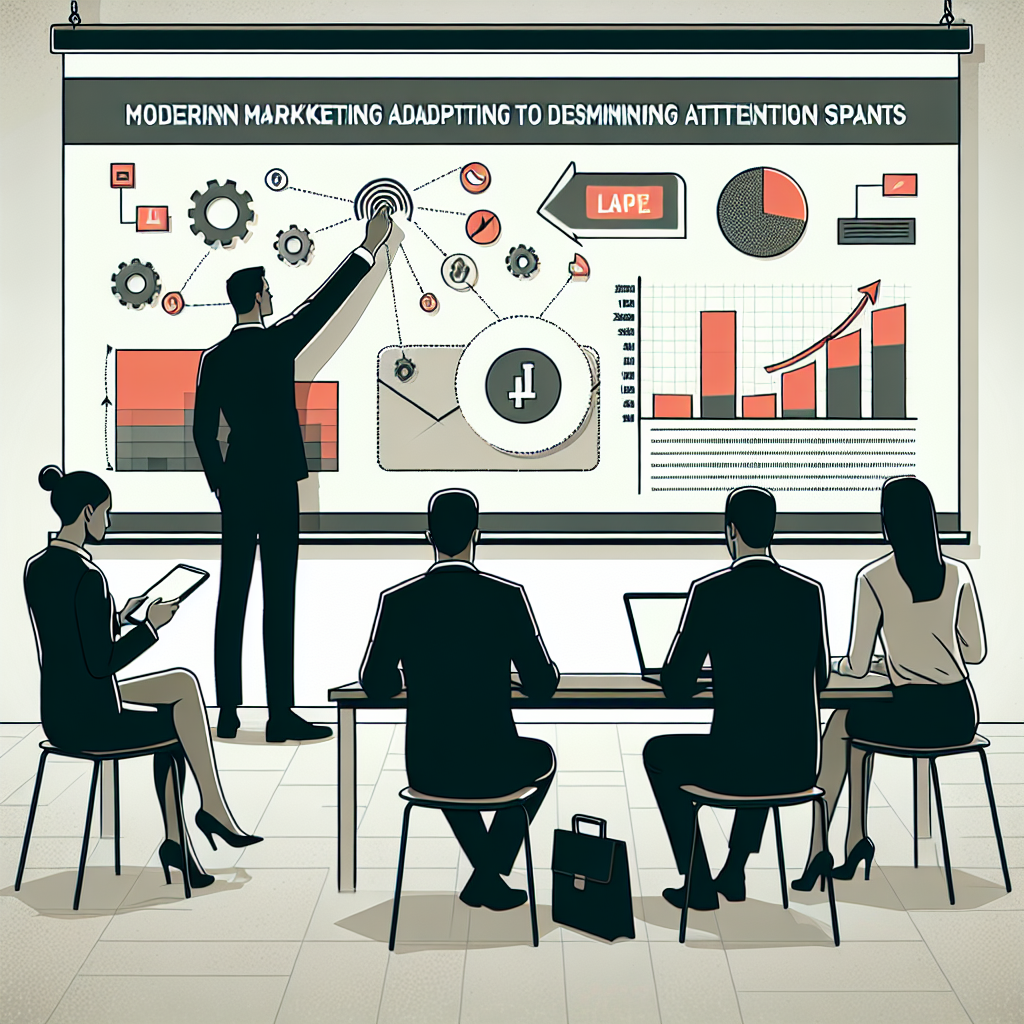
How the Attention Recession Is Changing Marketing
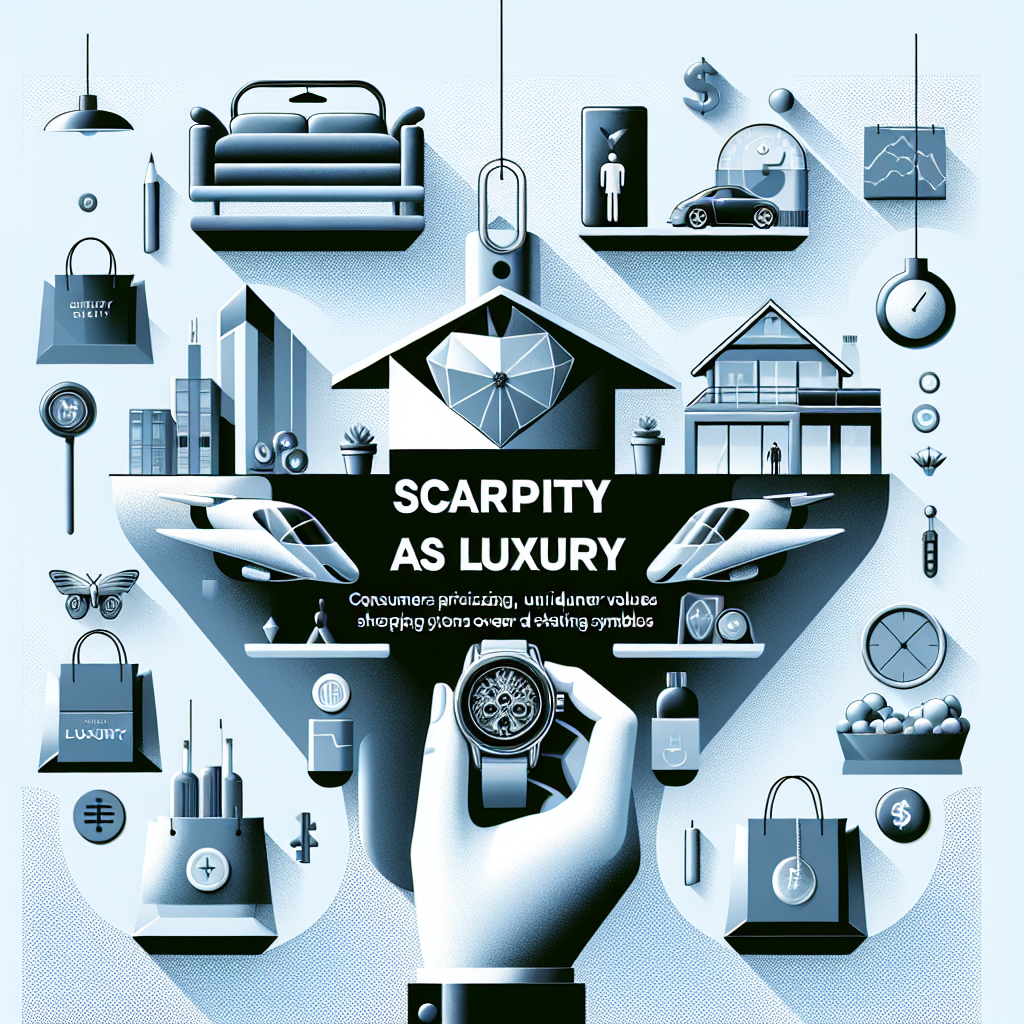
The New Luxury Why Consumers Now Value Scarcity Over Status
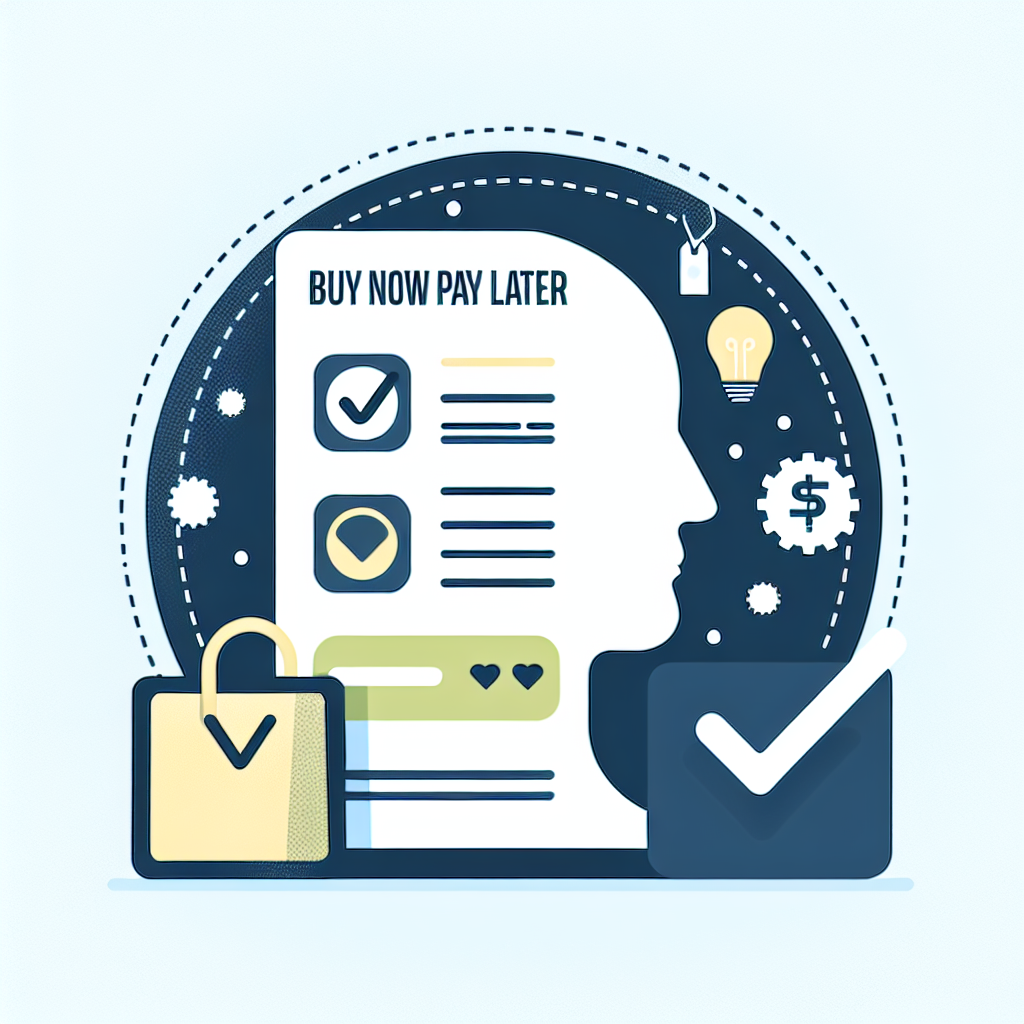
The Psychology Behind Buy Now Pay later
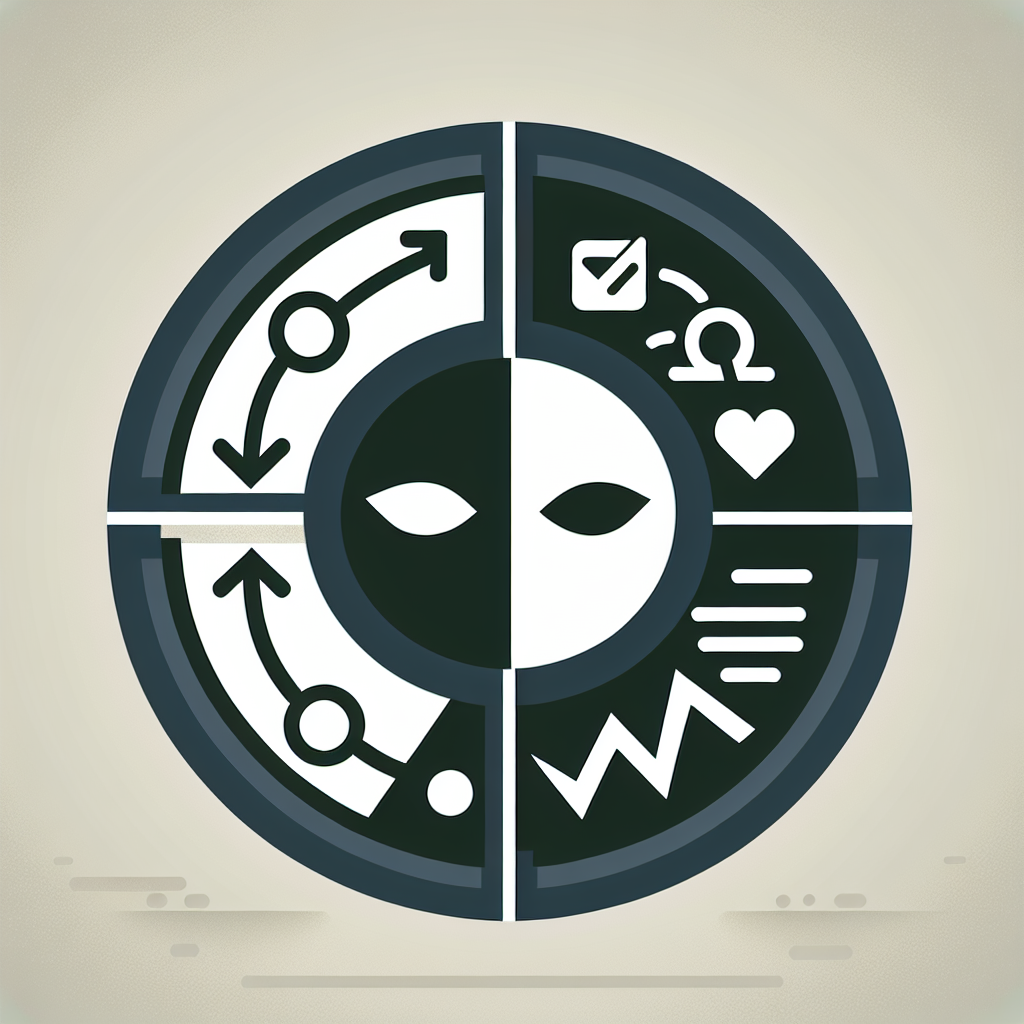
The Role of Dark Patterns in Digital Marketing and Ethical Concerns
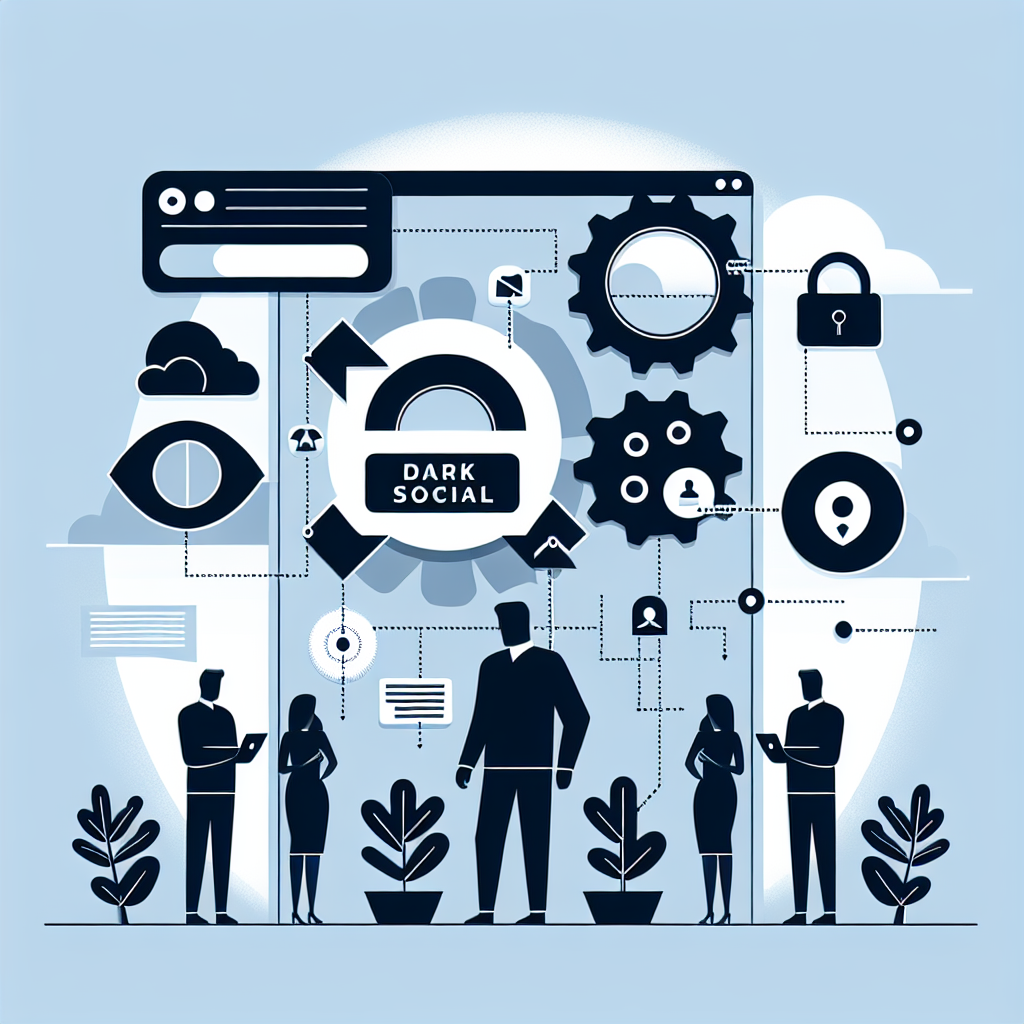
The Rise of Dark Social and Its Impact on Marketing Measurement
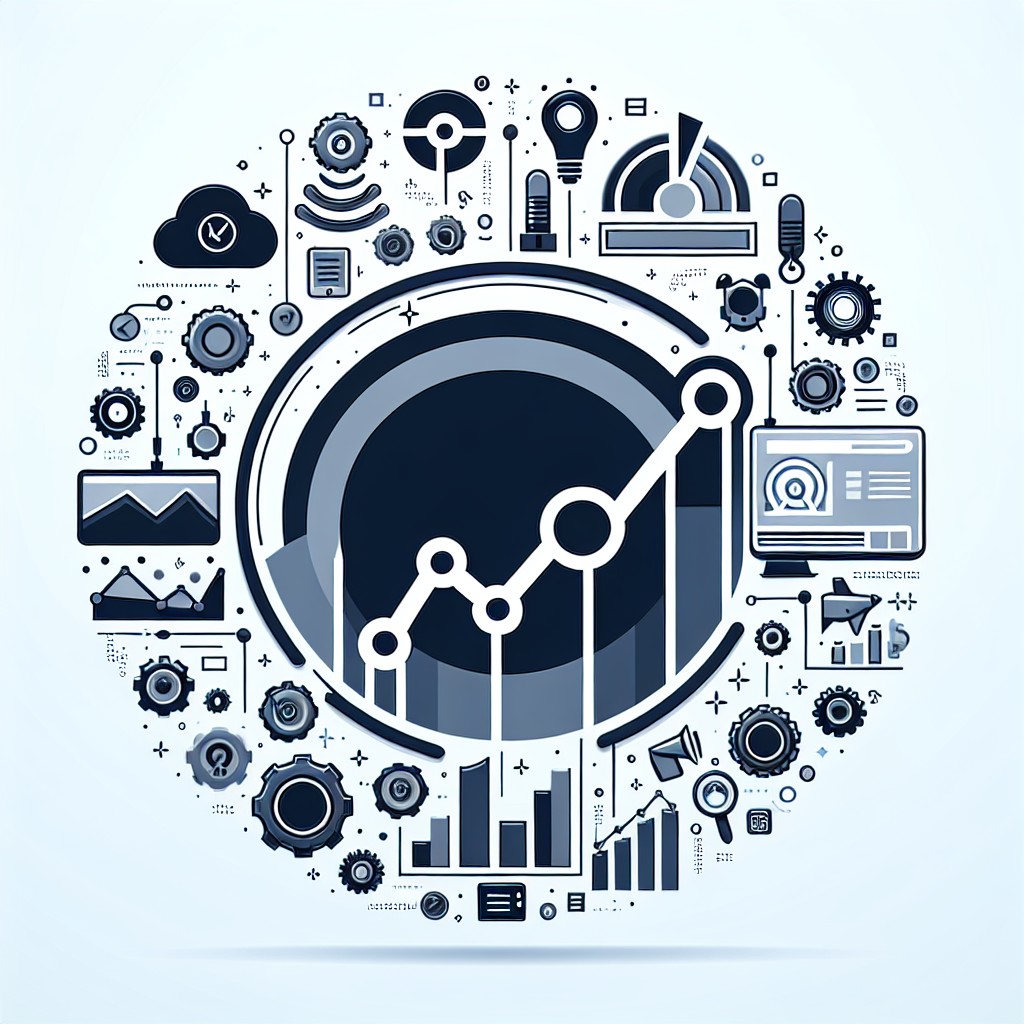