Beyond the Buzz – AI and ML in Marketing
Artificial intelligence (AI) has become a ubiquitous term in today's marketing landscape, often used interchangeably with machine learning (ML). However, understanding the distinction between these two concepts is crucial for marketers aiming to harness their potential effectively. While AI encompasses the broader scope of machines exhibiting human-like intelligence, ML is a subset focused on systems learning from data to improve over time. This nuanced difference has significant implications for marketing strategies, tools, and outcomes.
Defining AI and ML in Marketing
AI refers to the capability of machines to perform tasks that typically require human intelligence, such as reasoning, problem-solving, and understanding language. In marketing, AI can automate decision-making processes, analyze large datasets, and even generate creative content. For example, AI-driven chatbots can engage with customers in real-time, providing personalized responses and solutions.
ML, on the other hand, is a branch of AI that enables systems to learn and improve from experience without explicit programming. In the marketing realm, ML algorithms can analyze consumer behavior patterns to predict future actions, allowing for more targeted campaigns. For instance, ML can help in segmenting audiences based on purchasing behavior, enabling marketers to tailor messages that resonate with specific groups.
Practical Applications in Marketing
Understanding the practical applications of AI and ML can help marketers leverage these technologies more effectively.
1. Data Analysis and Customer Insights
Both AI and ML excel at processing vast amounts of data to uncover insights that might be invisible to human analysts. By analyzing customer interactions, purchase histories, and online behaviors, these technologies can identify trends and preferences, enabling marketers to craft strategies that align with consumer desires.
2. Personalization
ML algorithms can analyze individual user behavior to deliver personalized content, product recommendations, and advertisements. This level of personalization enhances user experience and increases the likelihood of conversion. For example, streaming services use ML to suggest shows or movies based on viewing history, improving engagement and satisfaction.
3. Predictive Analytics
ML models can forecast future consumer behaviors by identifying patterns in historical data. This capability allows marketers to anticipate market trends, optimize inventory, and plan effective promotional campaigns. For instance, retailers can predict which products will be in demand during certain seasons and adjust their marketing efforts accordingly.
4. Content Creation
Generative AI, a subset of AI, can produce content such as articles, social media posts, and even video scripts. While human oversight ensures quality and relevance, AI can handle repetitive tasks, allowing creative teams to focus on strategy and innovation. For example, fashion brands have utilized AI to create unique promotional images, blending traditional photography with AI-generated scenes to produce cost-effective and innovative visuals.
5. Ad Targeting and Optimization
AI-powered platforms can automatically adjust ad placements and bidding strategies in real-time to maximize ROI. By learning from ongoing campaign performance, these systems ensure that ads reach the most receptive audiences at optimal times. For example, Google's Performance Max campaigns utilize AI to identify potential customers across various channels, optimizing ad delivery without manual intervention.
Navigating the Hype: Evaluating AI and ML Solutions
With the proliferation of AI and ML in marketing, it's essential to critically assess the capabilities of various tools and platforms.
1. Understand the Technology
Not all solutions labeled as "AI-powered" truly leverage advanced AI or ML. Some may rely on basic algorithms that don't learn or adapt over time. Marketers should seek transparency from vendors about the underlying technology to ensure it meets their needs. As highlighted by Emily Yale, Principal Data Scientist at F5 Shape Security, "If your AI system is AI only because it is using ML, stop diluting its description by calling it an AI system and call it an ML system instead."
2. Assess Data Requirements
ML models require substantial and relevant data to function effectively. Evaluate whether your organization can provide the necessary data and whether the ML system can integrate seamlessly with your existing data infrastructure.
3. Consider Customization and Control
AI systems that operate autonomously may offer less flexibility for customization. In contrast, ML systems often allow for more tailored approaches, enabling marketers to adjust parameters and strategies based on specific goals and insights.
4. Evaluate Vendor Expertise
The effectiveness of AI and ML tools depends significantly on the expertise behind them. Vendors should have a deep understanding of both the technology and the marketing landscape to provide solutions that deliver real value.
Challenges and Considerations
While AI and ML offer numerous benefits, marketers should be mindful of potential challenges.
1. Consumer Perception
Some consumers are skeptical about AI, fearing job displacement or loss of human touch. A study highlighted that consumers are less likely to buy products labeled as AI-powered due to such concerns. Marketers should focus on how AI enhances the customer experience rather than emphasizing the technology itself.
2. Data Privacy
AI and ML systems rely on vast amounts of data, raising concerns about privacy and security. Compliance with regulations like GDPR is essential to maintain consumer trust and avoid legal repercussions.
3. Integration with Existing Systems
Implementing AI and ML solutions requires integration with current marketing technologies. Compatibility issues can lead to inefficiencies or data silos, hindering the effectiveness of these advanced tools.
4. Resource Investment
Developing and maintaining AI and ML systems can be resource-intensive. Organizations must consider the costs of technology, talent, and training to ensure successful implementation and sustainability.
Featured Blogs
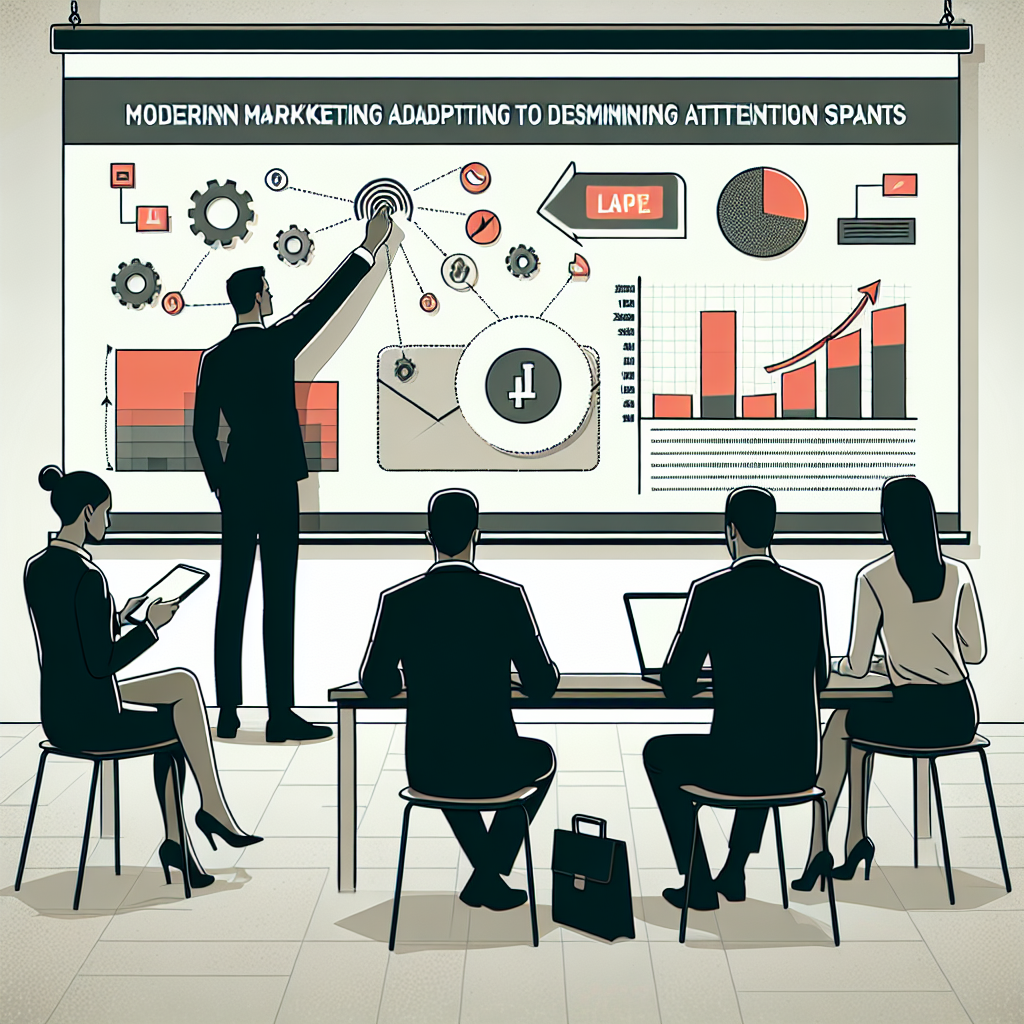
How the Attention Recession Is Changing Marketing
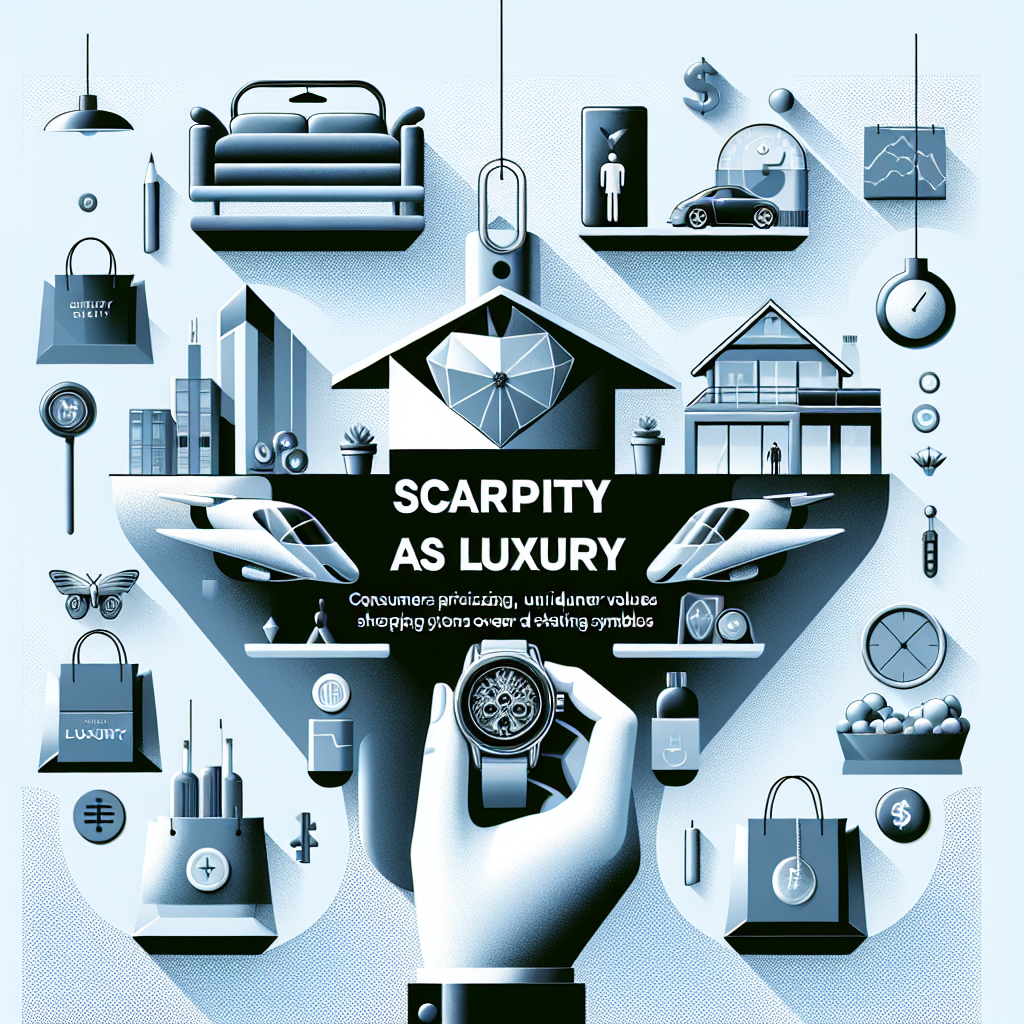
The New Luxury Why Consumers Now Value Scarcity Over Status
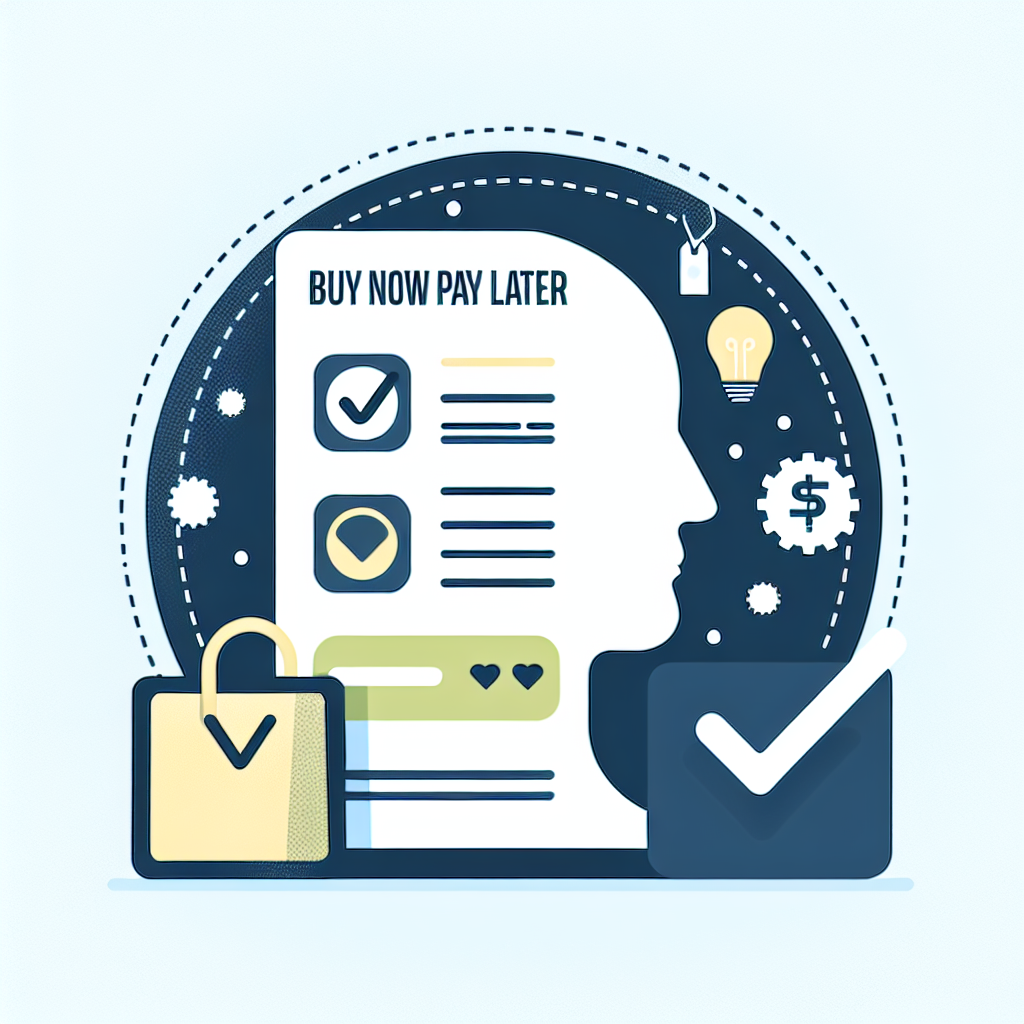
The Psychology Behind Buy Now Pay later
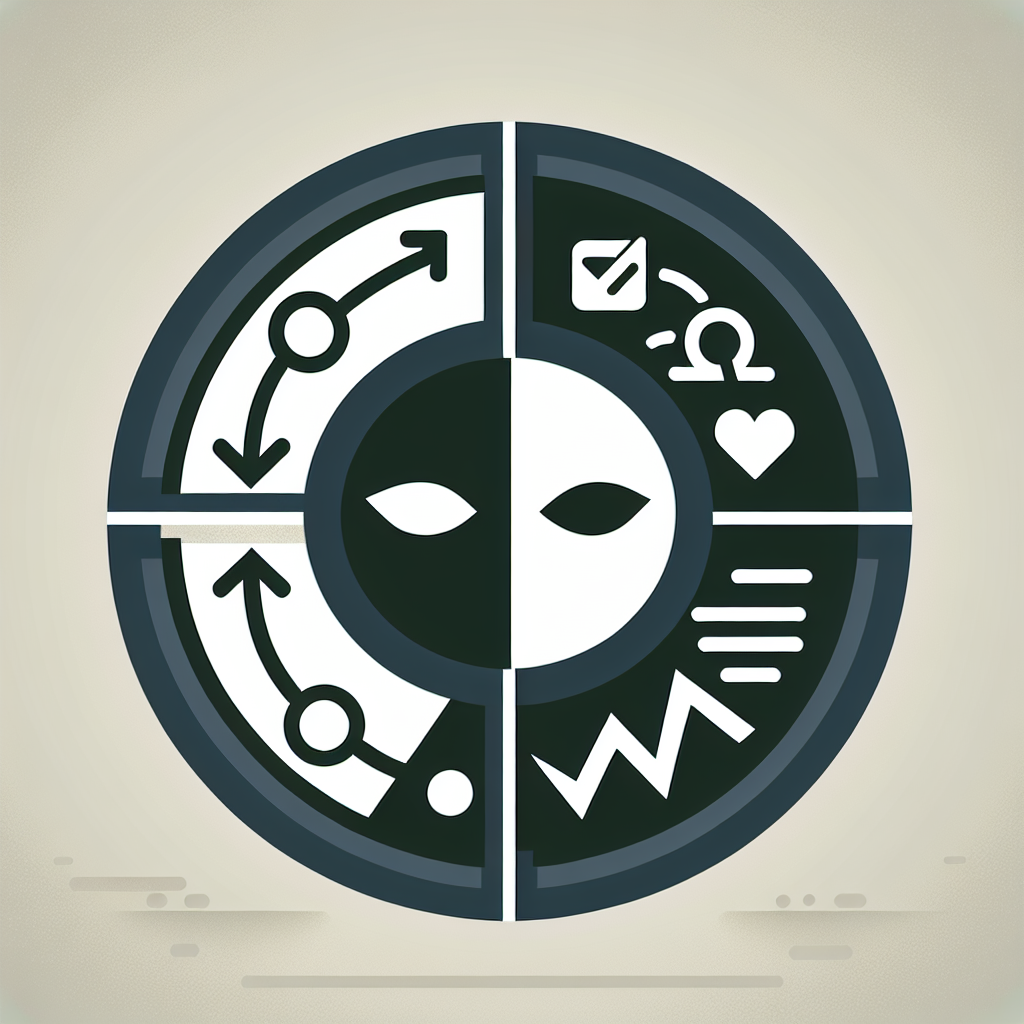
The Role of Dark Patterns in Digital Marketing and Ethical Concerns
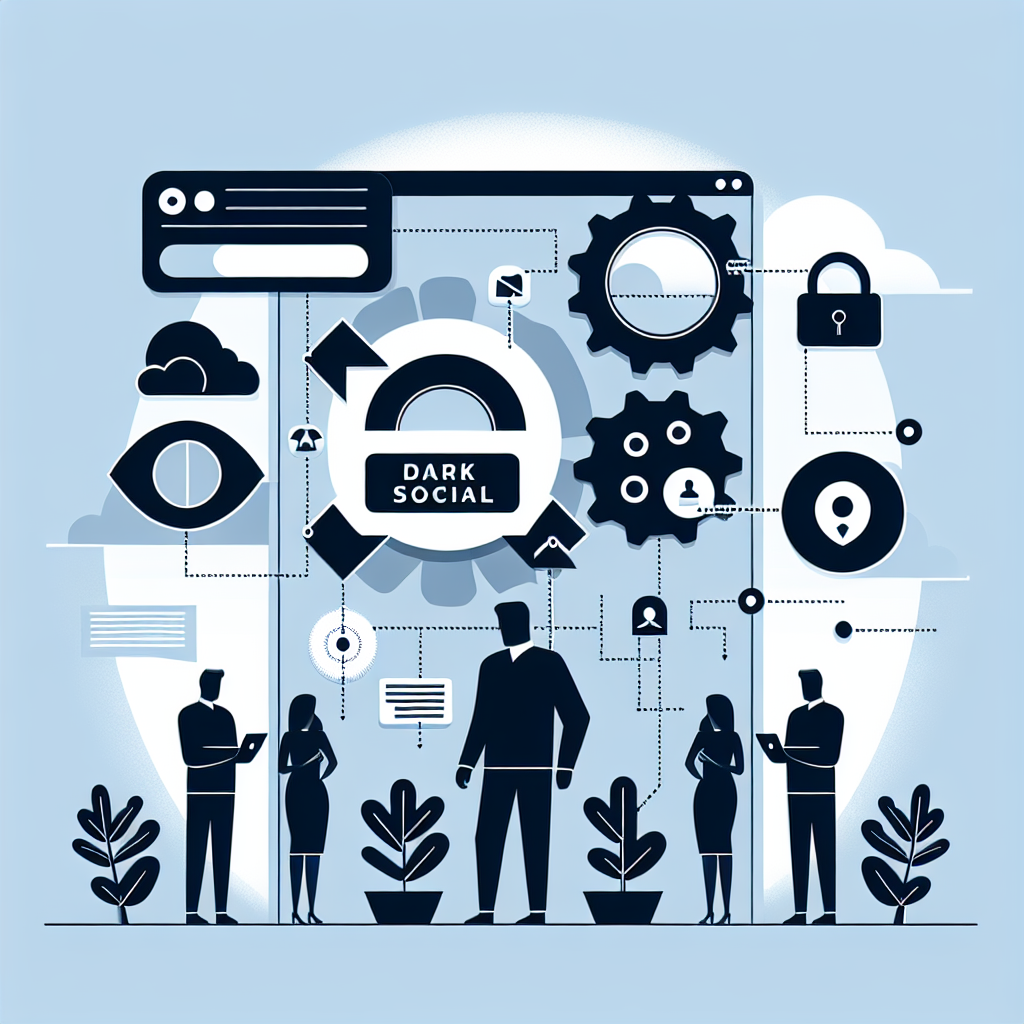
The Rise of Dark Social and Its Impact on Marketing Measurement
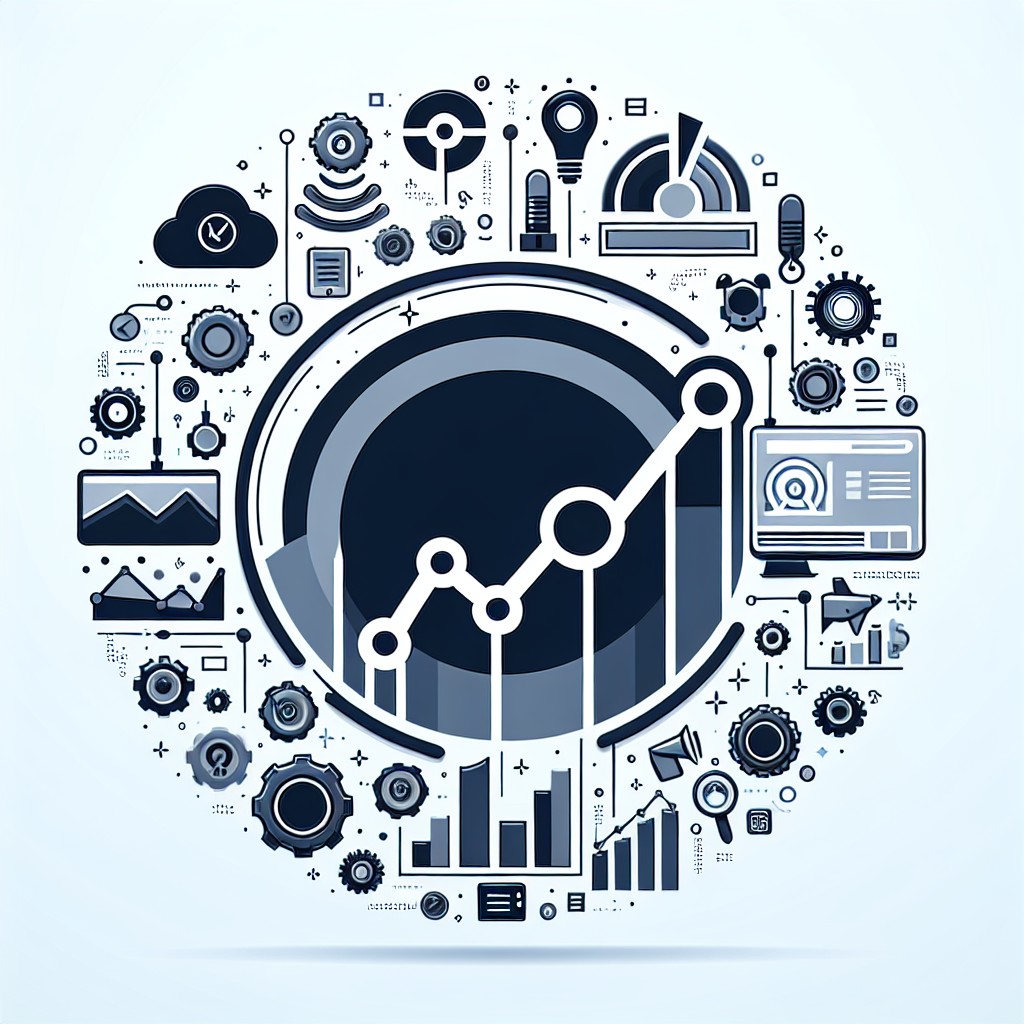