Longitudinal Studies in Marketing: When & How
The marketing team was in high spirits, celebrating a brand campaign that had doubled awareness metrics in just three months—a stunning success by conventional standards. As the champagne flowed, Neeraj, the research lead, was approached by his former graduate school classmate, now the consumer insights director. "I hate to rain on the parade," she whispered, "but I've been tracking a panel of consumers since before the campaign launched. Our awareness bump came entirely from light category users who rarely purchase. Among our core consumers, brand affinity is actually trending down."
Six months later, sales confirmed her warning—despite record awareness, purchase intent had weakened. That day transformed Neeraj’s understanding of marketing measurement. The campaign post-mortem revealed they'd been optimizing for short-term metrics while undermining long-term brand equity drivers. Since then, Neeraj has become an advocate for longitudinal research approaches that reveal not just what's happening now, but how consumer relationships evolve over time. In marketing, as in life, some of the most important truths only become visible when observing the full arc of the story.
Introduction
Marketing measurement has evolved dramatically from periodic cross-sectional snapshots toward continuous longitudinal understanding. While point-in-time studies can reveal current states, they cannot illuminate the causal mechanisms and evolutionary patterns that longitudinal approaches uniquely capture. According to research from the Marketing Science Institute, organizations employing sophisticated longitudinal tracking demonstrate 42% greater marketing ROI than those relying solely on cross-sectional analysis. As consumer journeys grow increasingly complex and non-linear in the digital era, understanding not just current attitudes but their trajectory and velocity becomes essential for predictive accuracy. Longitudinal research provides the methodological framework necessary to move beyond correlation toward the causal understanding required for strategic marketing decision-making.
Tracking Attitudes Over Time: Methodological Approaches
The foundation of effective longitudinal research lies in methodological designs that balance statistical rigor with practical implementation constraints.
True panel designs, where the same individuals are measured repeatedly, offer the greatest analytical power by controlling for individual differences. Procter & Gamble maintains what they call "consumer intimacy panels" of 5,000+ households tracked continuously over 3-5 years across multiple brand categories. This approach revealed that their assumption of stable brand preference formation was flawed; new data showed consumer brand relationships typically undergo critical "reassessment windows" every 8-14 months, when even loyal consumers become temporarily open to competitive offerings.
Cohort analysis, tracking defined groups rather than specific individuals, offers implementational advantages while still capturing temporal patterns. Netflix employs "subscription cohort tracking," following groups of subscribers based on when they joined the service. This revealed that content preferences evolve in predictable patterns over time—with new subscribers heavily favoring mainstream hits before gradually exploring niche content, a pattern that informed their content acquisition strategy.
Repeated cross-sectional designs, while methodologically weaker for causal inference, offer practical advantages in execution. Unilever implements what they term "accelerated longitudinal design," combining shorter overlapping panel waves with statistical techniques to approximate longer-term trends. This hybrid approach improved their predictive accuracy by 31% compared to traditional cross-sectional research while reducing panel fatigue and attrition.
The explosion of passive behavioral data has created new opportunities for longitudinal insight. Amazon tracks what they call "behavioral longitudinal patterns," algorithmically identifying significant shifts in purchase patterns that trigger deeper attitudinal research. This integration of continuous behavioral tracking with targeted attitudinal investigation has improved their new product adoption forecasting by 27%.
Retention Techniques and Data Quality Management
The analytical power of longitudinal research depends entirely on successful participant retention and data quality management across extended time periods.
Panel attrition represents the primary threat to valid longitudinal inference. Microsoft's research team implements what they call "predictive attrition management," using machine learning to identify participants showing early warning signs of dropout based on response patterns and engagement metrics. This allows for targeted retention interventions before attrition occurs, improving their longitudinal completion rates by 34%.
Incentive optimization balances participant motivation with response validity. Starbucks discovered that their initial high-value compensation approach was attracting "professional survey takers" whose response patterns showed significantly different trends than verified customers. Their revised "engagement-based compensation" model, where incentives increase with consistent participation over time, improved both retention and data quality metrics.
Digital engagement techniques have transformed retention capability. Nike's consumer tracking program employs what they call "micro-engagement touchpoints," replacing infrequent lengthy surveys with continuous micro-interactions integrated into their app ecosystem. This approach increased longitudinal participation rates from 23% to 68% over 12 months while providing more continuous data streams.
Response quality monitoring becomes particularly crucial over extended time periods. IBM's brand tracking system employs automated "response pattern analysis" to identify satisficing behaviors like straight-lining or pattern responses. When detected, these trigger verification questions rather than immediate exclusion, recovering approximately 17% of responses that would otherwise be lost while maintaining data integrity.
Causal Inference Through Time: Analytical Approaches
The ultimate value of longitudinal research lies in its capacity for causal inference—understanding not just what changed but why it changed and what interventions drive desired outcomes.
Cross-lagged panel models disentangle causal direction between related constructs measured over time. When Disney analyzed relationships between streaming service satisfaction and usage frequency, cross-lagged analysis revealed that for new subscribers, satisfaction drove usage; however, after approximately four months, the relationship reversed, with usage habits primarily driving satisfaction. This insight fundamentally changed their customer experience optimization strategy.
Fixed effects modeling controls for unobserved individual differences that might otherwise confound causal relationships. American Express employs what they term "within-subject causal modeling" for their loyalty program analysis, which revealed that members' responses to promotions were driven less by offer characteristics (which varied across members) than by individual-specific reference points based on past promotions (which varied within members over time). This insight led to personalized promotion strategies based on individual promotion history rather than demographic segments.
Latent growth curve modeling captures underlying trajectories beyond visible fluctuations. Coca-Cola's brand tracking implements what they call "attitude velocity analysis," modeling not just current brand perceptions but the rate and direction of change for different consumer segments. This approach identified an emerging negative trend among younger consumers 8 months before it appeared in traditional metrics, allowing for targeted intervention that reversed the decline.
Mediation analysis across time reveals causal mechanisms connecting interventions to outcomes. L'Oréal's digital marketing analytics employs "time-based mediation modeling" to understand how specific digital touchpoints influence purchase behavior. This analysis revealed that for their luxury brands, social media exposure primarily influenced purchases indirectly by first changing store visit behavior, with an average 24-day lag between initial exposure and store visit—an insight that transformed their attribution modeling and budget allocation.
Quasi-experimental designs exploit natural variations to approximate experimental conditions. Airbnb analyzes what they call "natural experimental conditions" where some markets received marketing interventions while similar markets did not. By applying difference-in-differences analysis to their longitudinal data, they determined that television advertising produced short-term booking increases that decayed within 4-6 weeks, while content marketing interventions produced smaller initial effects but continued influencing booking behavior for 3+ months.
Conclusion
As marketing ecosystems grow increasingly complex in the digital age, longitudinal research approaches provide the methodological framework necessary to understand not just current states but evolutionary trajectories and causal mechanisms. The technique's ability to disentangle causation from correlation, identify emerging trends before they become obvious, and understand the temporal dynamics of consumer relationships offers insight depth impossible with simpler cross-sectional approaches.
The integration of longitudinal research with artificial intelligence and passive behavioral data represents the frontier of marketing research methodology. Leading brands are now combining traditional longitudinal surveys with continuous behavioral data streams, creating hybrid models that connect attitudinal evolution with observed behaviors at unprecedented scale and granularity.
Call to Action
For marketing leaders seeking to elevate their longitudinal research capabilities:
- Audit existing measurement systems to identify opportunities for longitudinal enhancement
- Develop pilot longitudinal tracking initiatives for high-priority brand dimensions or consumer segments
- Invest in analytical capacity building through specialized training or strategic partnerships
- Create data integration frameworks that combine survey-based and behavioral longitudinal data streams
- Implement insight activation protocols that translate complex temporal patterns into actionable marketing strategies
The most successful organizations of tomorrow will be those that master the science of time in marketing research—understanding not just what consumers think and do today, but how their relationships with brands evolve over time and what interventions shape these evolutionary paths.
Featured Blogs
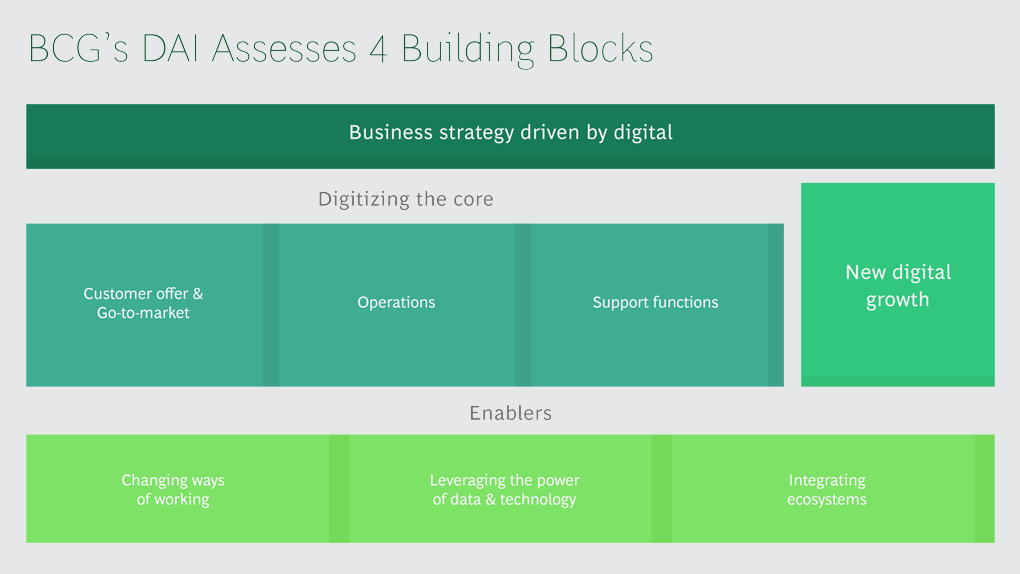
BCG Digital Acceleration Index
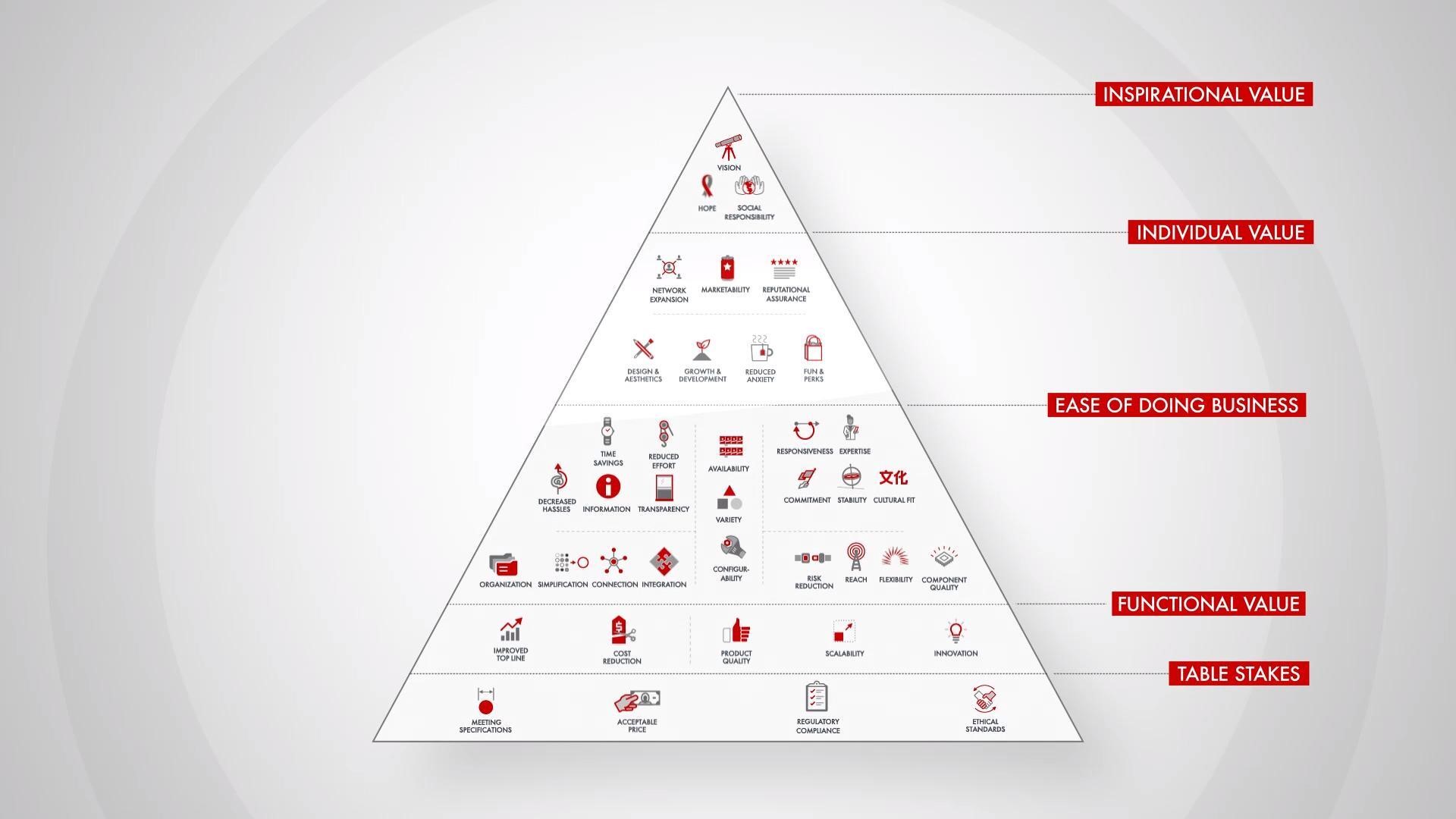
Bain’s Elements of Value Framework
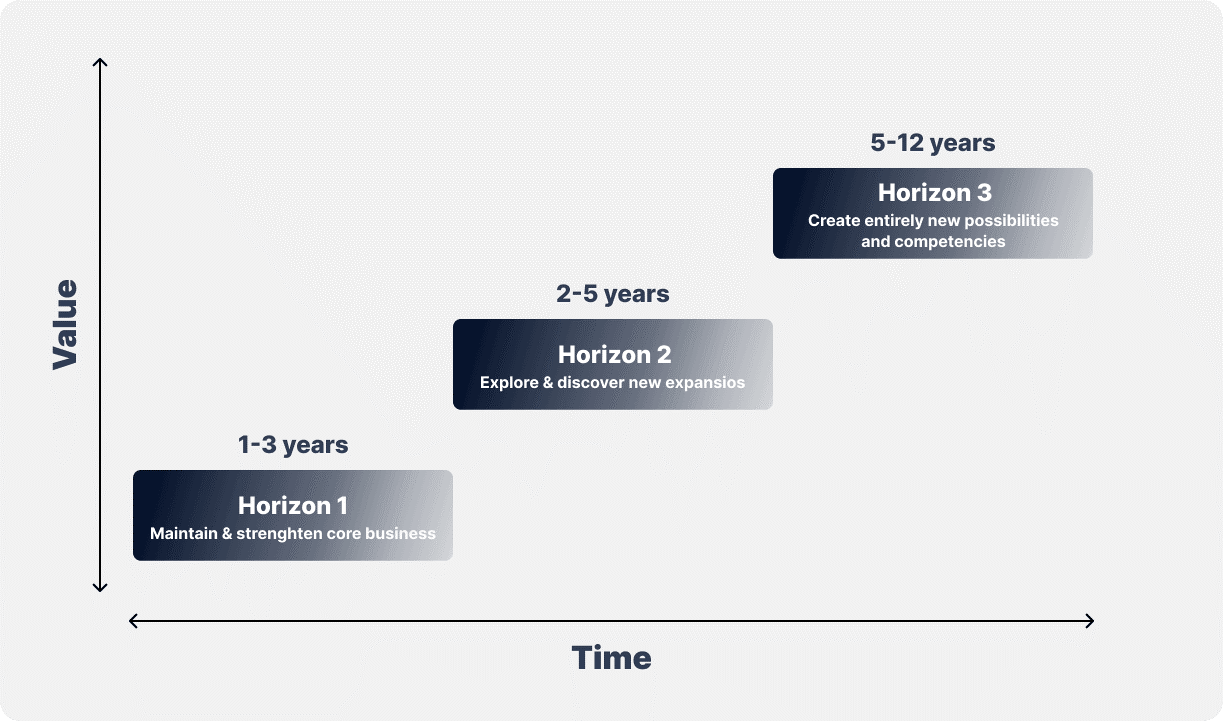
McKinsey Growth Pyramid
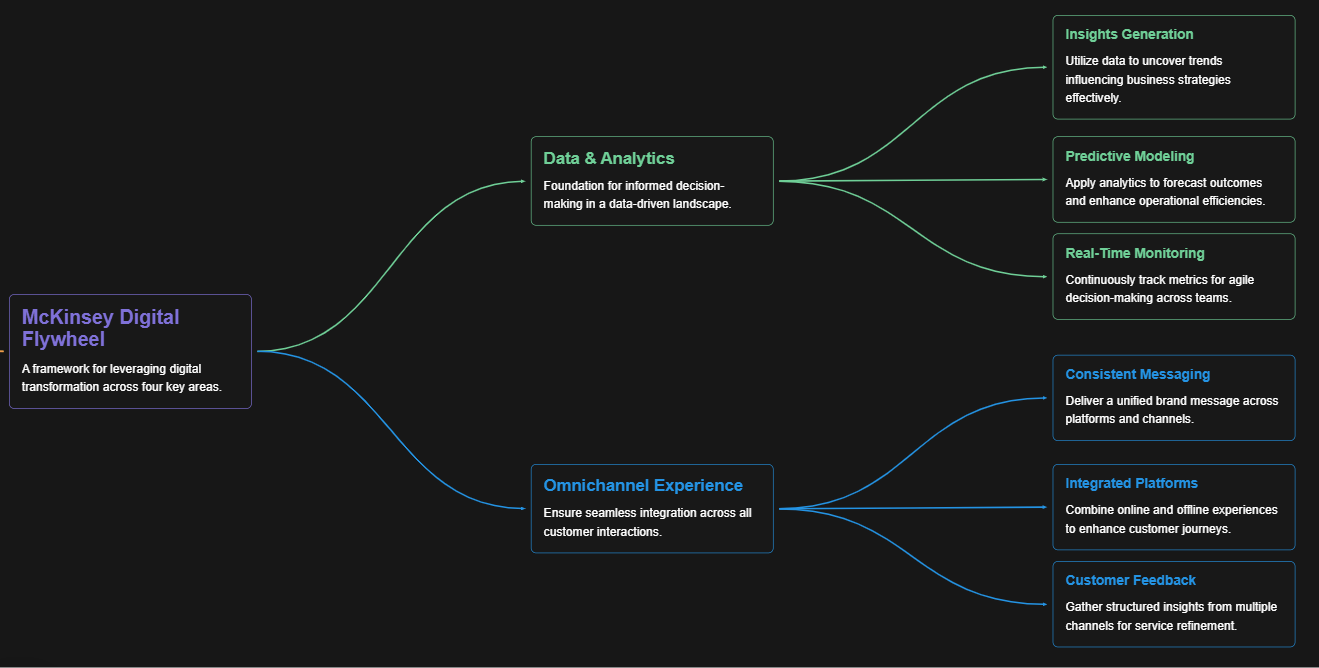
McKinsey Digital Flywheel
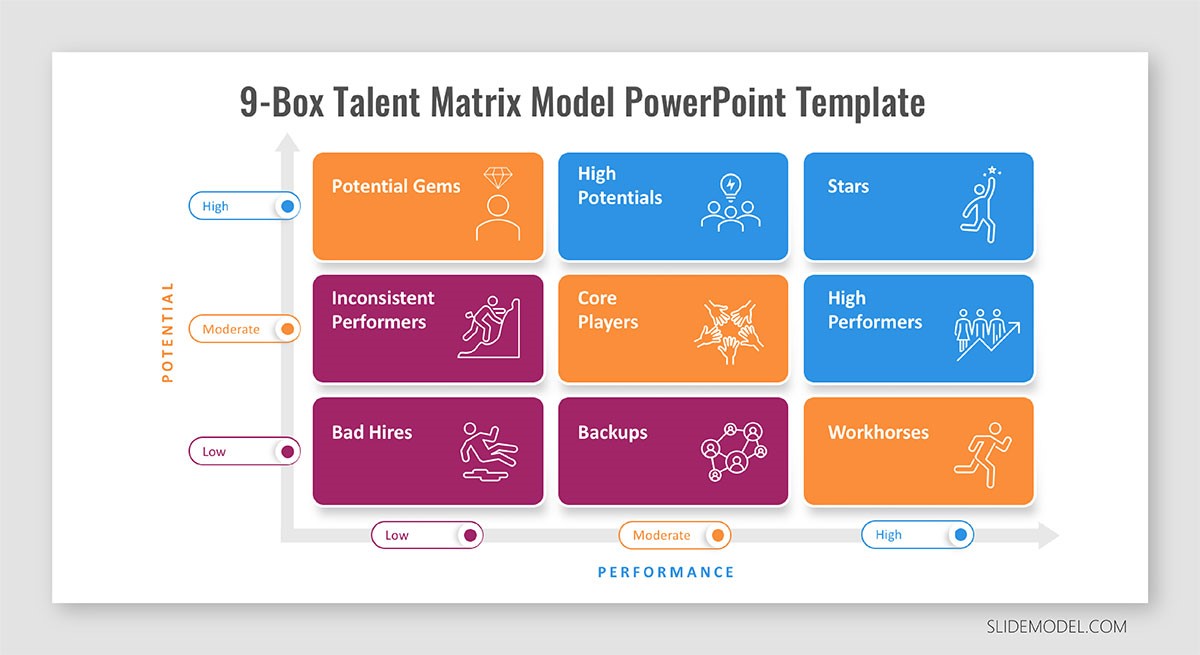
McKinsey 9-Box Talent Matrix
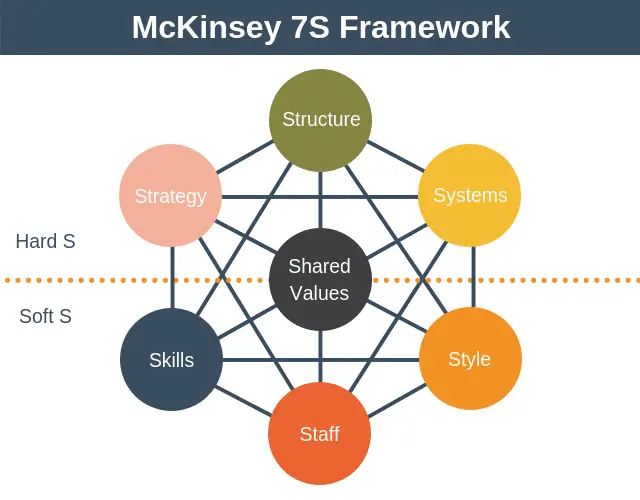
McKinsey 7S Framework
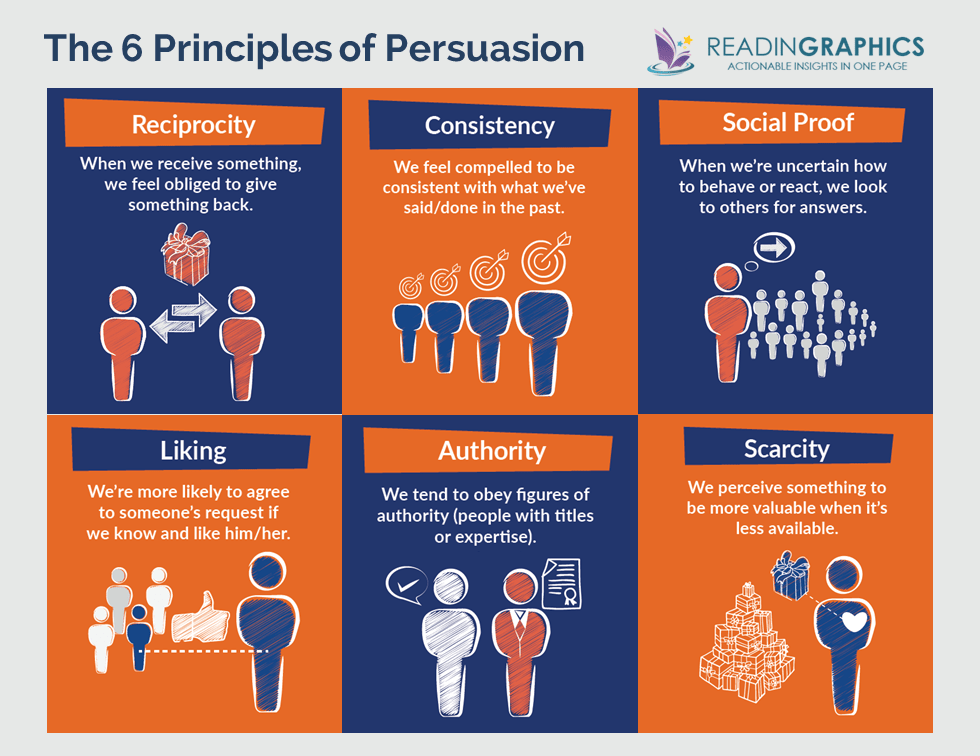
The Psychology of Persuasion in Marketing
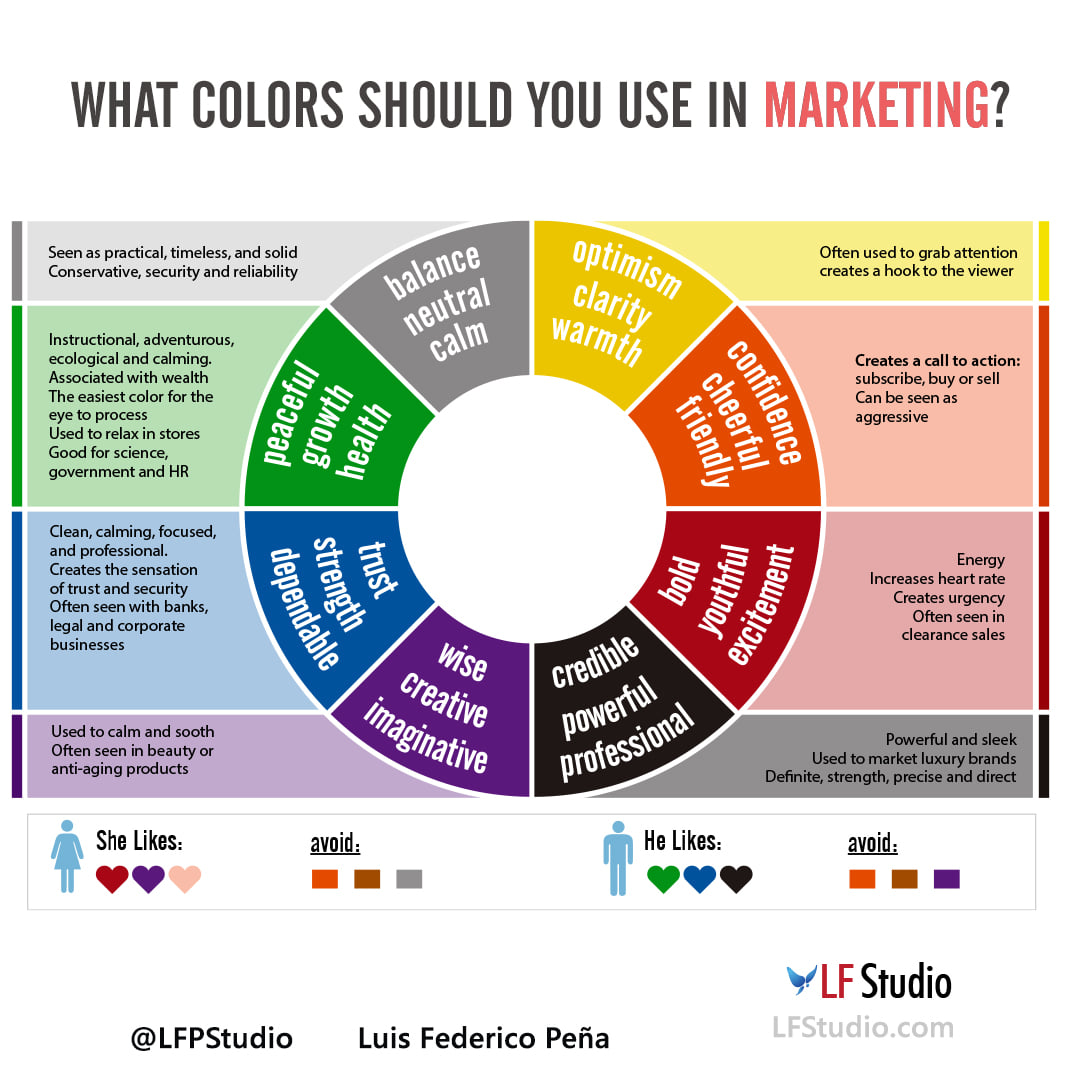
The Influence of Colors on Branding and Marketing Psychology
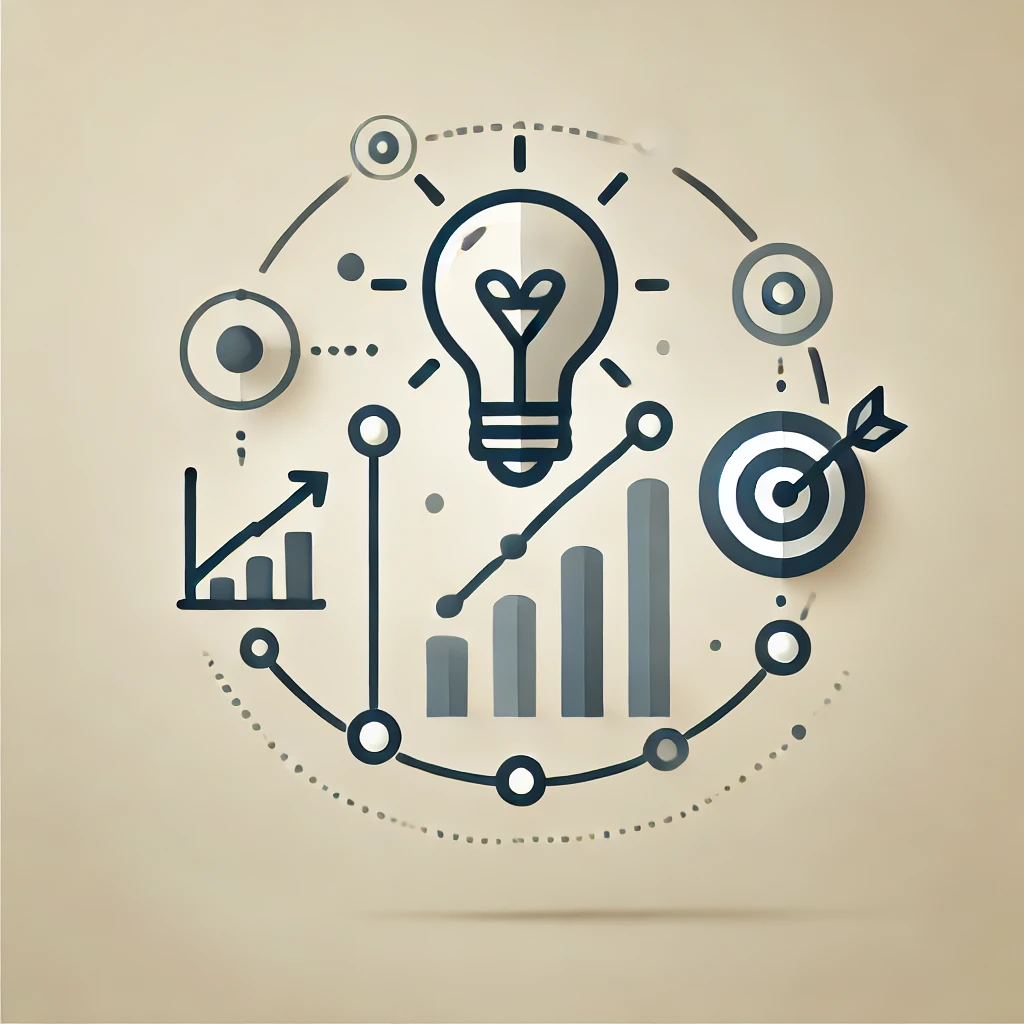