Analyzing Open-Ended Survey Responses at Scale
The breaking point for Neeraj came during a crucial product feedback analysis project. Faced with nearly 10,000 open-ended responses in spreadsheets, Neeraj observed his team of analysts struggling through their third consecutive late night, manually coding responses into thematic categories. Despite their expertise, fatigue was leading to inconsistent categorization and critical insights being missed. When a senior analyst admitted to skimming responses after reaching hour fourteen, Neeraj realized that their traditional approach had reached its limits. This crisis prompted the team to explore more sophisticated methods for qualitative analysis at scale—a journey that transformed not only their methodology but also the strategic value of their insights program. What began as a methodological necessity has since become Neeraj's team's greatest competitive advantage, enabling them to deliver deeper consumer understanding than their competitors.
Introduction: The Qualitative Analysis Challenge
Open-ended survey responses represent one of marketing research's most valuable yet challenging data sources. Unlike structured quantitative data, these unfiltered consumer voices offer authentic perspectives, unexpected connections, and the contextual "why" behind the quantitative "what." According to the Marketing Research Association, studies incorporating properly analyzed open-ended responses demonstrate 47% higher executive confidence and 32% greater likelihood of influencing strategic decisions compared to purely quantitative approaches.
However, this value comes with significant methodological challenges. Traditional manual analysis becomes impractical beyond a certain scale, while oversimplified automated approaches risk missing nuance and context. Research from Northwestern University's Integrated Marketing Communications program indicates the average 1,000-response qualitative dataset contains approximately 80-120 distinct themes, many of which are missed by basic automated analysis.
As noted by Harvard Business School professor Gerald Zaltman, "The most valuable consumer insights often hide in the unstructured, messy data that's hardest to analyze systematically—that's precisely why they remain undiscovered by competitors."
1. Manual vs. AI Coding Approaches
The evolution from purely manual coding to AI-assisted approaches represents a fundamental shift in qualitative analysis capabilities and outcomes.
The Traditional Coding Paradigm
Manual coding by trained analysts offers substantial benefits:
- Contextual understanding of language nuances and cultural references
- Recognition of implicit meanings and emotional subtext
- Adaptation to unexpected or novel themes
- Integration of non-verbal cues in multimodal responses
However, this approach faces critical limitations at scale including coder fatigue, inconsistency across analysts, throughput constraints, and significant resource requirements. Research by Kantar found manual coding consistency drops by approximately 23% after four hours of continuous coding and 47% when conducted across multiple days.
Machine Learning Augmentation
Advanced organizations now implement hybrid approaches combining human expertise with AI capabilities:
- Initial machine clustering with human refinement
- Human-coded training data powering supervised learning models
- Confidence scoring identifying responses requiring human review
- Continuous learning systems improving through ongoing feedback
Consumer goods giant Unilever implemented this approach across their insights function, reducing analysis time by 73% while increasing theme identification by 31% compared to previous manual approaches.
Model Selection and Training Considerations
Effective implementation requires strategic model choices beyond generic solutions:
- Domain-specific training data reflecting industry vocabulary and consumer language
- Balanced training sets representing diverse response types
- Multi-language capabilities for global research applications
- Category-specific concept recognition for specialized topics
Financial services firm Capital One developed custom models for different product categories, improving insight extraction by 42% compared to general-purpose sentiment analysis tools.
2. NLP Applications in Marketing Research
Natural Language Processing technologies have transformed qualitative analysis capabilities, enabling previously impossible analytical approaches.
Beyond Sentiment Analysis
While sentiment analysis dominated early NLP applications, contemporary approaches offer much richer analytical capabilities:
- Topic modeling identifying thematic patterns without predefined categories
- Entity extraction recognizing products, features, and comparison points
- Intent identification revealing underlying motivations and goals
- Emotion detection capturing nuanced emotional states beyond positive/negative
Automotive manufacturer Toyota applies comprehensive NLP analysis to product reviews, identifying not just sentiment but specific feature associations, comparison patterns, and usage contexts that have informed product development priorities worth over $40 million in estimated value.
Contextual Understanding Advances
Modern NLP systems increasingly recognize contextual factors critical to accurate interpretation:
- Sarcasm and irony detection preventing misinterpretation
- Cultural reference identification across different markets
- Ambiguity resolution through contextual analysis
- Demographic language pattern recognition
Hospitality leader Marriott implemented advanced contextual analysis for guest feedback, revealing substantial differences in how satisfaction is expressed across cultural backgrounds and leading to a 28% improvement in problem resolution protocols.
Longitudinal Analysis Capabilities
Sophisticated NLP applications track thematic evolution over time:
- Emerging theme identification through anomaly detection
- Trend analysis showing shifting consumer priorities
- Language evolution tracking as terminology changes
- Causal mapping between marketing activities and response themes
Consumer electronics company Samsung deployed longitudinal NLP analysis across five years of consumer feedback, identifying emerging feature priorities 8-14 months before they appeared in quantitative tracking studies.
3. Word Clouds vs. Thematic Grouping
Visualization and presentation approaches significantly impact how effectively qualitative insights drive decision-making.
The Limitations of Simplistic Visualization
Basic word clouds present significant analytical limitations:
- Overemphasis on frequency without context or meaning
- Loss of phrase integrity and multi-word concepts
- Absence of relationship mapping between concepts
- Inability to distinguish between similar words used differently
Research by the Marketing Science Institute found executives shown word cloud visualizations were 43% less likely to approve resources for initiatives compared to those shown more sophisticated thematic visualizations of the same data.
Advanced Visualization Frameworks
Leading organizations employ more sophisticated approaches to insight visualization:
- Concept mapping showing relationships between themes
- Hierarchical clustering displaying thematic organization
- Sentiment-colored thematic visualization
- Interactive filtering enabling dynamic exploration
Technology company Adobe restructured their customer feedback visualization around thematic hierarchy rather than word clouds, increasing executive engagement with findings by 67% and resource allocation to identified issues by 41%.
From Visualization to Actionability
The most effective qualitative analysis approaches directly connect insights to action frameworks:
- Decision matrices linking themes to specific organizational functions
- Priority mapping based on theme prevalence and business impact
- Competitive benchmarking within thematic categories
- Journey-stage integration positioning themes within customer experience
Telecommunications provider Verizon implemented "theme-to-action" protocols assigning specific organizational ownership to discovered themes, increasing insight implementation rates by 58% compared to previous approaches.
Conclusion: The Future of Qualitative Analysis
As artificial intelligence capabilities continue advancing, qualitative analysis approaches will undergo further transformation. Emerging technologies including large language models, multimodal analysis integrating text with visual and audio data, and advanced semantic networks promise new analytical dimensions beyond current capabilities.
The organizations gaining maximum value from qualitative data increasingly view analysis as an integrated insight ecosystem rather than a discrete methodological step. This approach incorporates qualitative analysis throughout the research lifecycle, creating continuous feedback loops between quantitative and qualitative understanding.
The future belongs to research operations that balance technological sophistication with fundamental human understanding. The most powerful insights emerge not from algorithms alone but from thoughtful integration of computational power with human interpretive expertise.
Call to Action
For research professionals seeking to elevate qualitative analysis capabilities:
- Audit current open-ended analysis approaches against emerging best practices
- Invest in hybrid human-AI workflows that leverage the strengths of both
- Develop visualization frameworks that communicate thematic understanding beyond word frequency
- Create cross-functional interpretation sessions that bring diverse perspectives to qualitative data
- Build direct connections between thematic insights and specific action owners
In today's insight-driven business environment, the competitive advantage lies not in collecting consumer opinions but in systematically transforming unstructured feedback into structured, actionable intelligence that drives better decisions than your competitors can make.
Featured Blogs
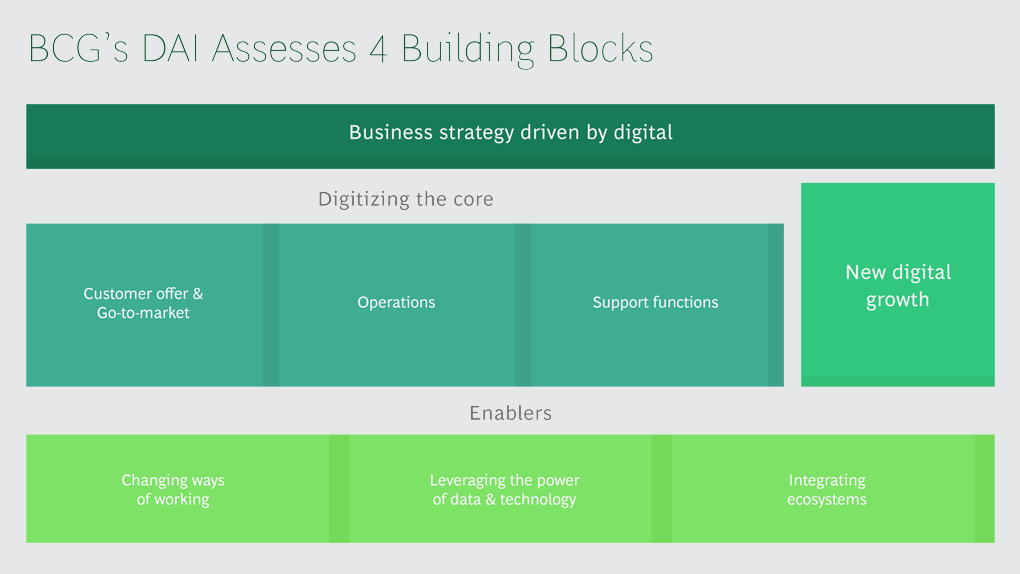
BCG Digital Acceleration Index
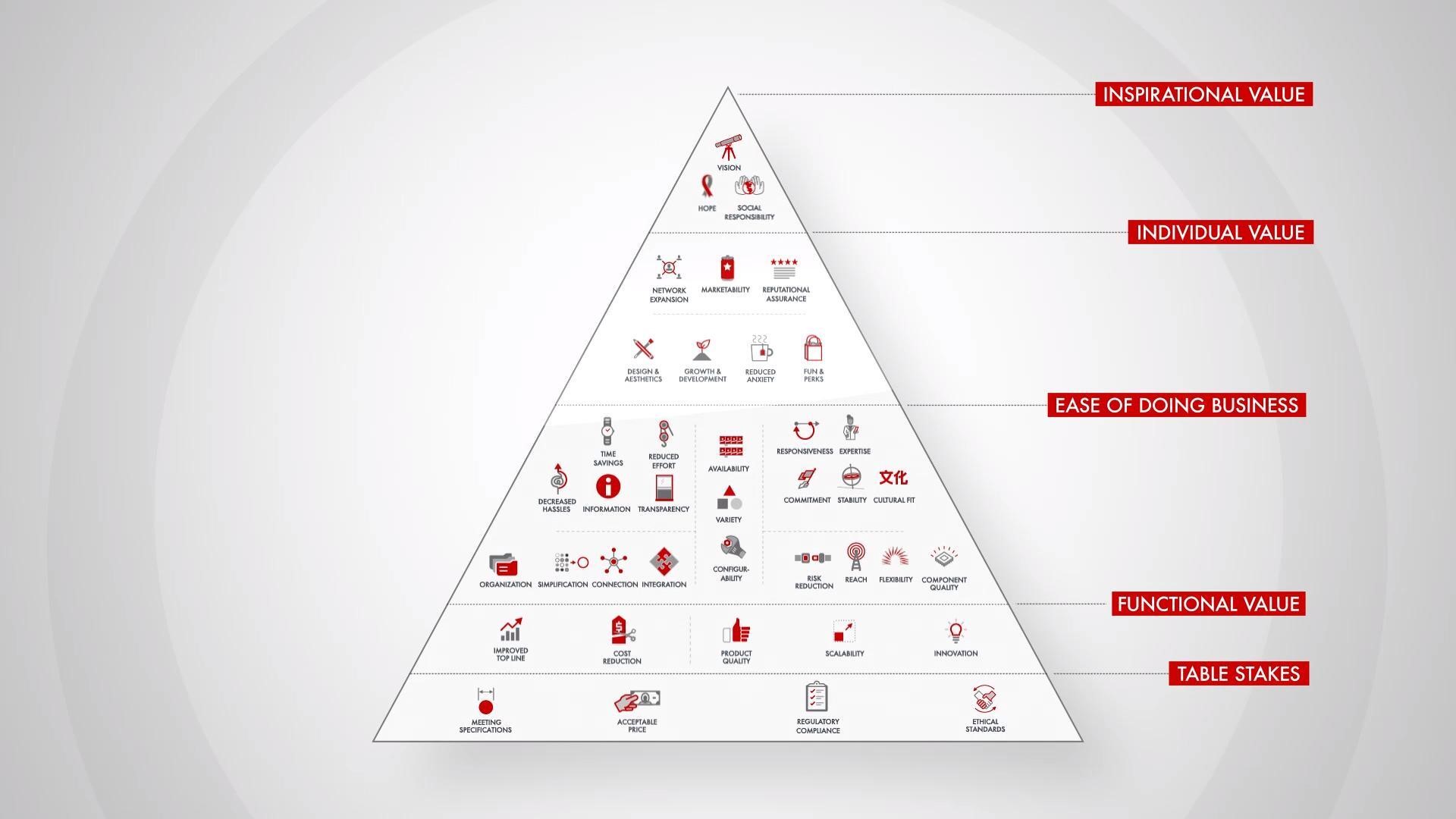
Bain’s Elements of Value Framework
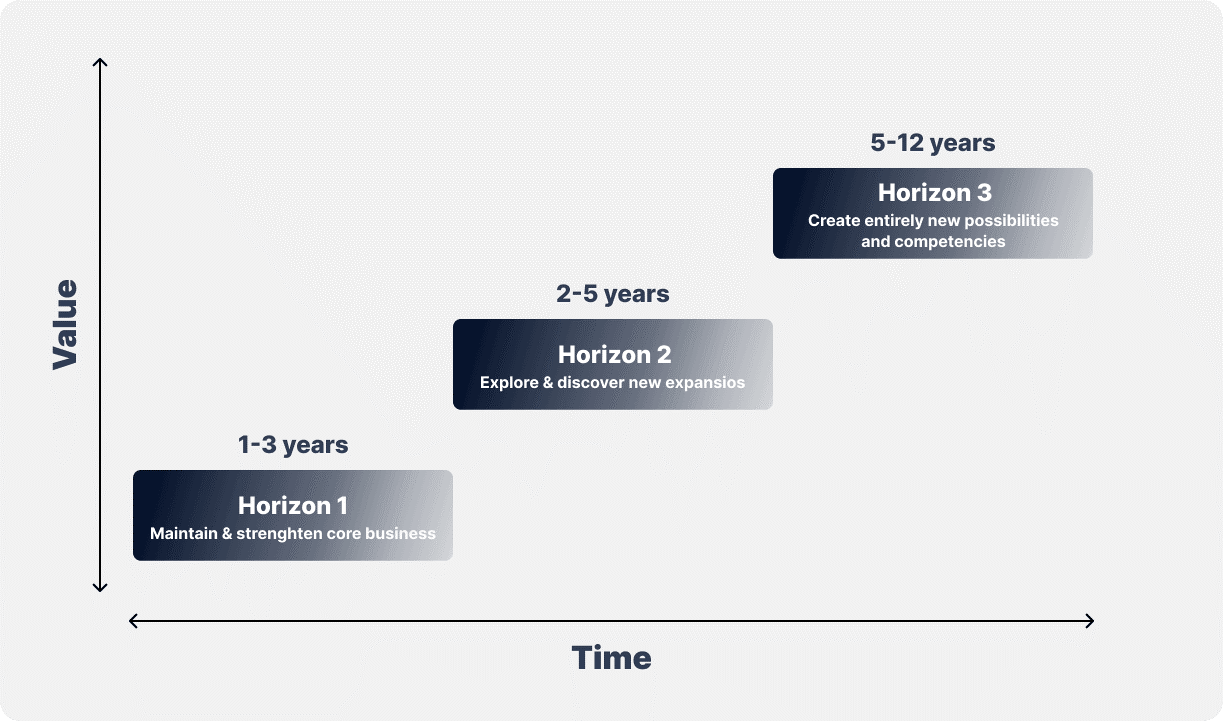
McKinsey Growth Pyramid
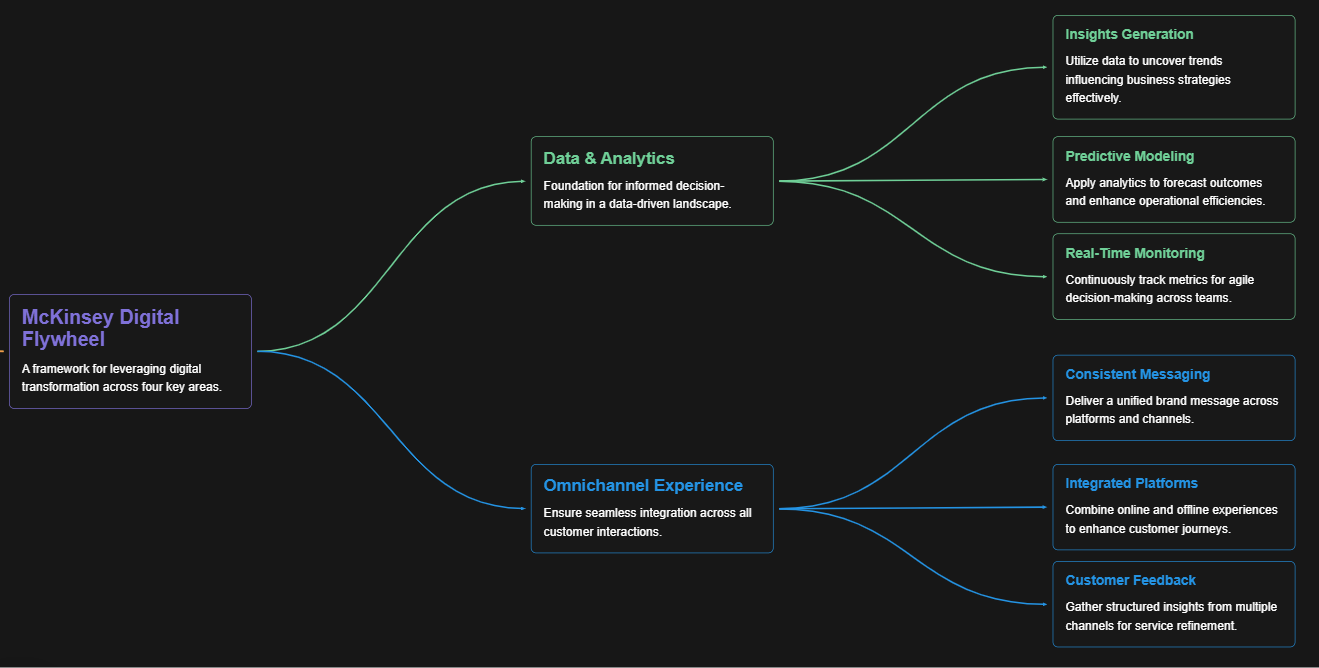
McKinsey Digital Flywheel
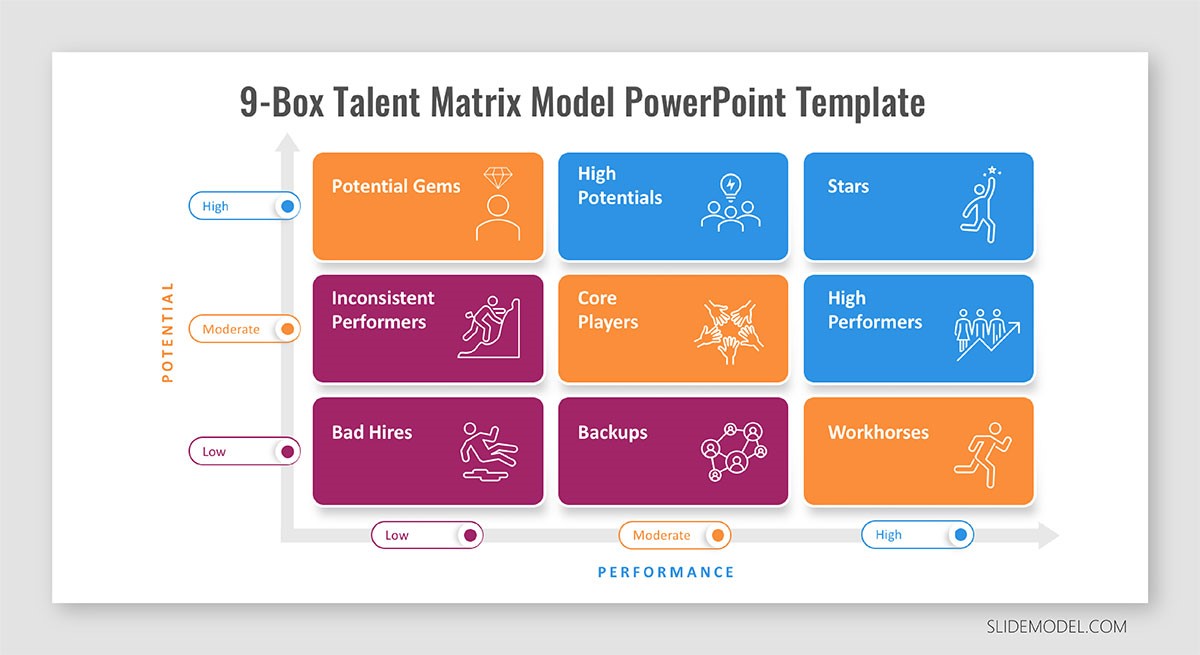
McKinsey 9-Box Talent Matrix
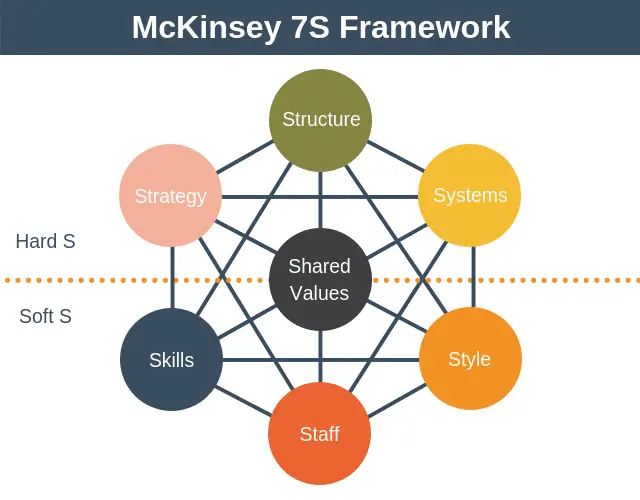
McKinsey 7S Framework
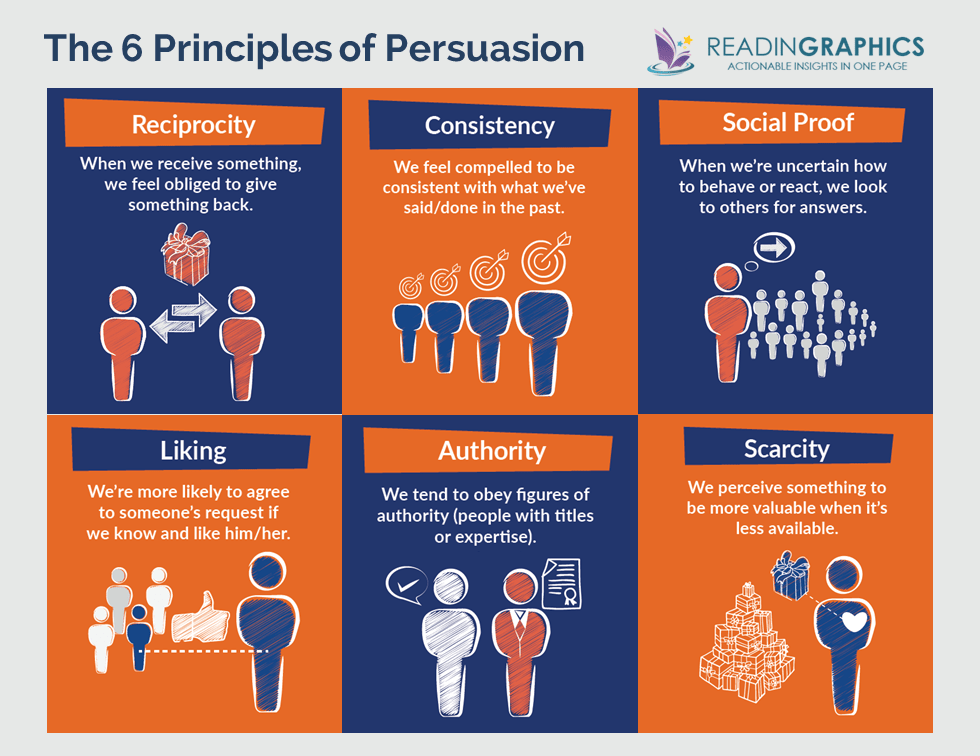
The Psychology of Persuasion in Marketing
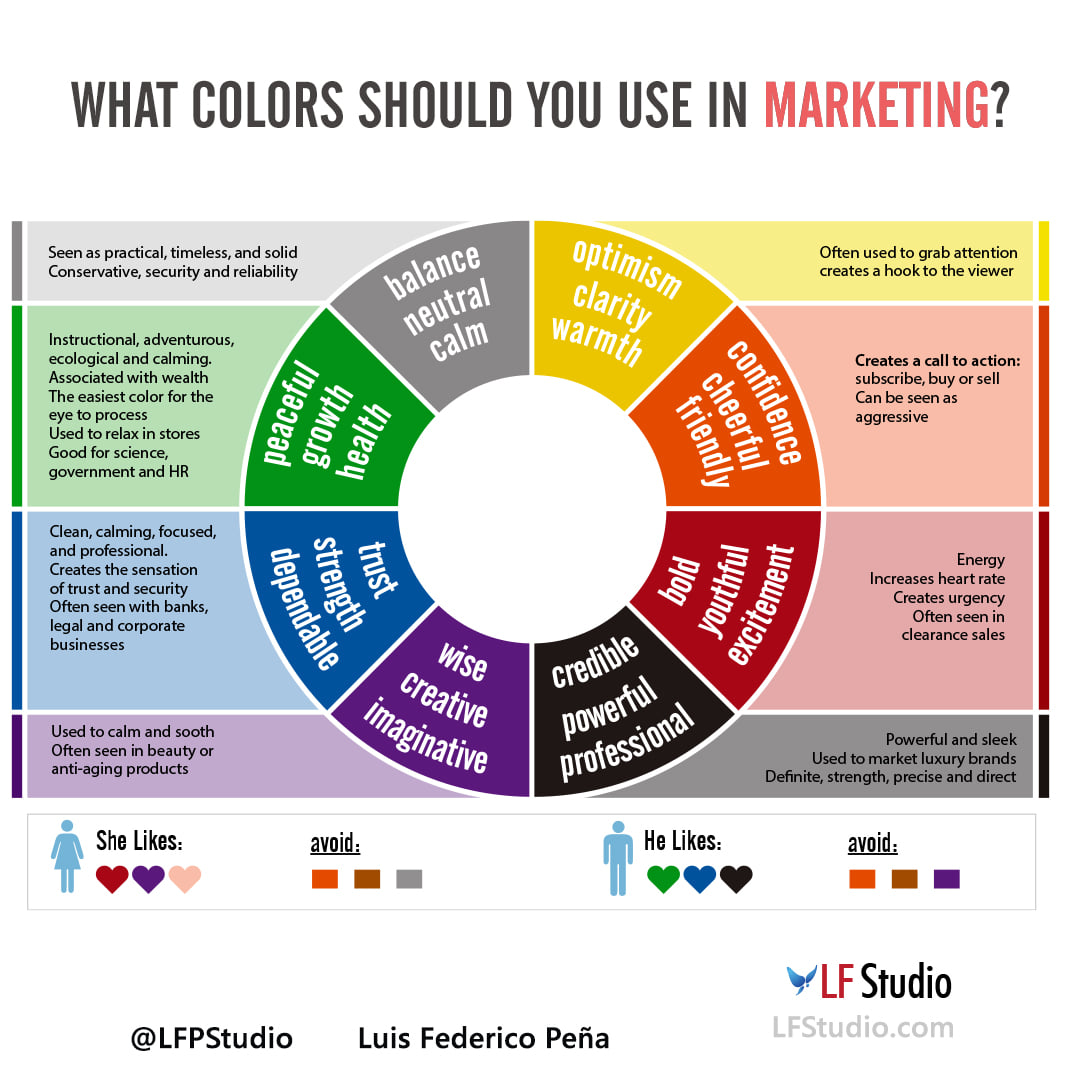
The Influence of Colors on Branding and Marketing Psychology
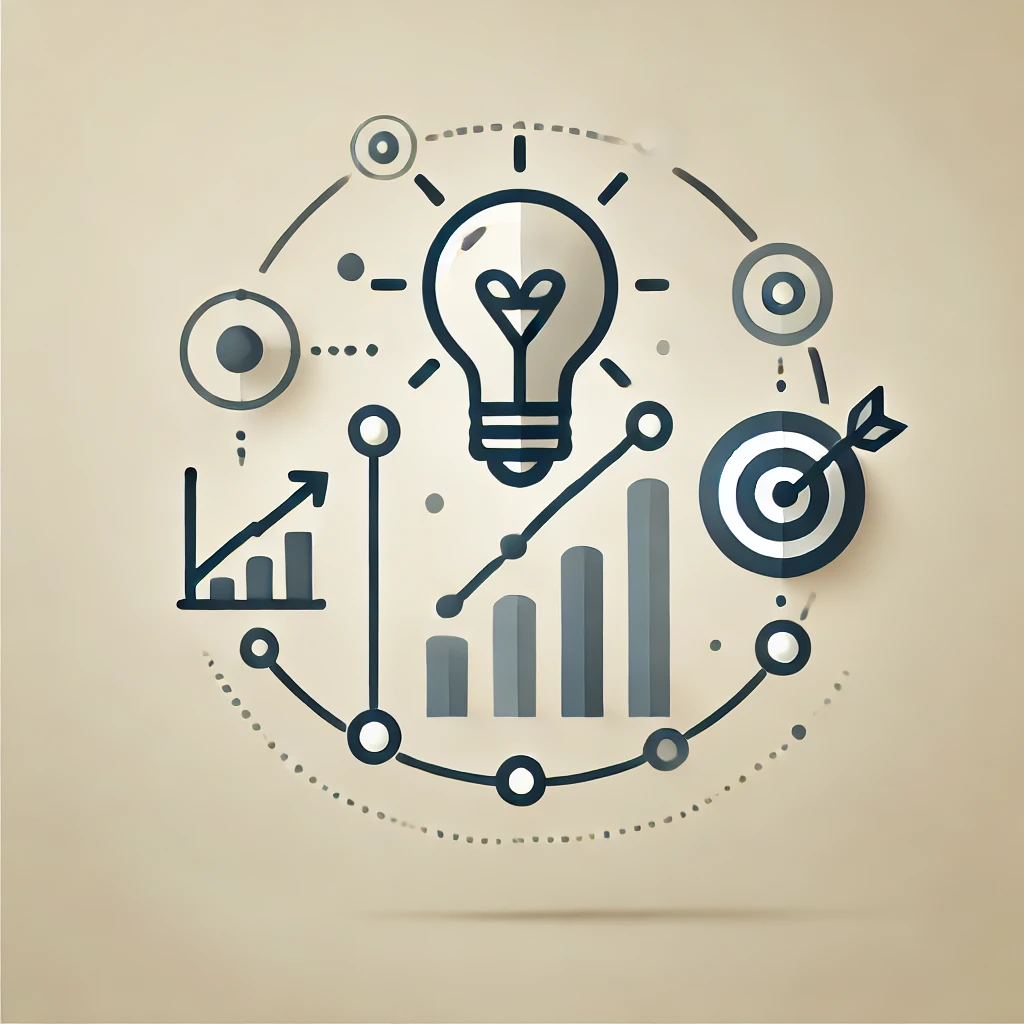