Behavioral Personas from App Analytics
During a product analytics review meeting for their mobile app, my friend experienced a significant revelation. As they examined the charts and graphs, they began to notice distinct patterns emerging—not just in the metrics, but in the behavior of their users. Some users methodically explored every feature of the app, while others focused exclusively on one core function. However, their marketing strategy at the time treated all users identically.
That afternoon, my friend gathered their team to map these behavior clusters, creating rudimentary personas based not on demographics, but on how people actually used their product. When they redesigned their onboarding sequence to align with these behavioral patterns, their activation rate increased by 36% in just two weeks. This watershed moment transformed my friend's entire approach to product analytics. They came to understand that behind every data point is a person with distinct behavioral patterns, patterns that reveal more about their needs than any survey could capture.
Introduction: The Behavioral Revolution in User Understanding
Product analytics has evolved from basic traffic metrics to sophisticated behavioral intelligence. This transformation moves beyond traditional demographic segmentation to understand users through their actual interactions with digital products. Behavioral personas represent the confluence of big data capabilities with psychological insight, creating actionable user archetypes based on observed patterns rather than reported preferences.
Research from Product Analytics Institute indicates that behavioral segmentation delivers 43% higher conversion rates compared to demographic targeting alone. Meanwhile, a study in the Journal of Interactive Marketing found that products optimized for behavioral personas show 2.7x stronger user retention compared to generalized approaches.
1. Feature Usage Clustering
Modern behavioral personas emerge from sophisticated pattern analysis of how users interact with different product features.
Feature Adoption Patterns
Advanced clustering algorithms reveal natural user segments:
- Sequential feature adoption mapping
- Feature combination affinity analysis
- Depth versus breadth usage patterns
- Engagement intensity distribution across functions
Example: Spotify's feature clustering analysis identified their "Playlist Curator" behavioral persona—users who spend minimal time searching but invest heavily in organization features. This led to interface adaptations prioritizing curation tools for this segment, resulting in 28% higher retention among these valuable community contributors.
Interaction Intensity Mapping
Usage depth provides crucial behavioral signals:
- Time investment distribution analysis
- Feature abandonment pattern identification
- Reengagement trigger tracking
- Complexity tolerance assessment
Example: Adobe's Creative Cloud analysis revealed a behavioral persona they termed "Feature Explorers"—users who systematically test new capabilities but rarely incorporate them into workflows. This insight led to the development of guided feature tours rather than technical documentation for this segment, improving feature adoption by 31%.
Cross-Platform Behavior Coherence
Multi-device usage patterns reveal deeper intent:
- Device-specific feature preferences
- Context-based functionality requirements
- Synchronization and continuity behaviors
- Platform transition friction points
Example: Banking app Revolut identified the "Cross-Platform Financial Manager" persona through behavioral analysis, discovering users who begin transactions on mobile but complete them on desktop. This insight drove development of their "Handoff" feature, increasing complex transaction completion rates by 24%.
2. Building Activation Flows
Effective activation sequences align with natural behavioral tendencies rather than forcing predetermined paths.
Progressive Complexity Introduction
Behavioral personas reveal optimal complexity pacing:
- Feature revelation sequencing
- Complexity tolerance thresholds
- Guided versus exploratory learning preferences
- Confidence-building progression models
Example: Notion analyzed user behavioral data to identify distinct learning patterns, creating separate activation flows for "Systematic Learners" who prefer structured tutorials versus "Exploratory Builders" who abandon guided experiences. This personalized approach increased week-one feature adoption by 47%.
Value Realization Acceleration
Different personas find value in different aspects:
- Primary value trigger identification
- Time-to-value optimization paths
- Value reinforcement touchpoints
- Value expansion sequences
Example: Project management platform Monday.com developed distinct activation flows for their "Visual Organizer" and "Process Automator" behavioral personas, emphasizing different capabilities for each. This approach reduced time-to-value by 36% and increased premium conversion by 21%.
Contextual Milestone Celebration
Recognition patterns should match behavioral motivations:
- Achievement recognition calibration
- Progress visualization preferences
- Social versus private accomplishment sharing
- Intrinsic versus extrinsic reward preferences
Example: Language learning app Duolingo identified distinct achievement preferences through behavioral analysis, creating separate reward systems for "Streak Maintainers" versus "Level Completers." This targeted approach increased daily active usage by 18%.
3. Campaigns Based on Product Behavior
Marketing communications achieve maximum relevance when triggered by behavioral signals.
Behavioral Trigger Refinement
Actions reveal optimal intervention moments:
- Engagement pattern disruption detection
- Feature discovery opportunity identification
- Usage intensity fluctuation response
- Progression deceleration interventions
Example: Productivity app Evernote implemented behavior-based trigger campaigns, sending different resources when "Organizational" users showed engagement drops versus "Content Creator" users. This targeted approach increased reengagement by 34% compared to time-based campaigns.
Channel-Behavior Alignment
Different behavioral personas respond to different channels:
- Channel receptivity correlation analysis
- Multi-channel orchestration optimization
- Response latency patterns by channel
- Channel context appropriateness mapping
Example: Trading platform Robinhood discovered their "Research-First Investors" behavioral persona responded best to email educational content while "Opportunity Seekers" engaged with mobile notifications. This channel alignment increased campaign effectiveness by 27%.
Content Personalization Matrices
Message content should reflect behavioral context:
- Feature usage-based content relevance
- Behavioral maturity-appropriate messaging
- Problem-solution contextual matching
- Aspiration-aligned advancement paths
Example: SaaS platform Hubspot created a behavioral content matrix for their "Data Analyst" and "Content Producer" personas, delivering different resources based on recent platform activities. This approach improved content engagement by 41% and feature adoption by 23%.
Conclusion: The Behavioral Future of Product Development
As product analytics capabilities continue to advance, behavioral personas will become increasingly predictive rather than merely descriptive. This evolution will enable truly anticipatory experiences that understand not just what users have done, but what they are likely to do next.
The integration of behavioral pattern recognition into product development represents more than technical innovation—it fundamentally transforms the relationship between creators and users, enabling experiences that respond to actual needs rather than assumed preferences.
As these methodologies mature, the distinction between user research and product analytics will continue to blur, creating unprecedented opportunities for intuitive experiences that require less explanation because they align with natural behavioral tendencies.
Call to Action
For product leaders seeking to implement behavioral persona methodologies:
- Invest in behavioral analytics infrastructure beyond basic event tracking
- Build cross-functional teams spanning data science, UX research, and marketing
- Develop hypothesis-driven experimentation frameworks for behavioral pattern validation
- Create behavioral persona documentation that evolves with new insights
- Measure success through behavioral cohort performance rather than aggregate metrics
The future of digital product development belongs not to those who collect the most data, but to those who derive the most meaningful behavioral insights—translating interaction patterns into experiences that feel intuitively aligned with how people naturally behave.
Featured Blogs
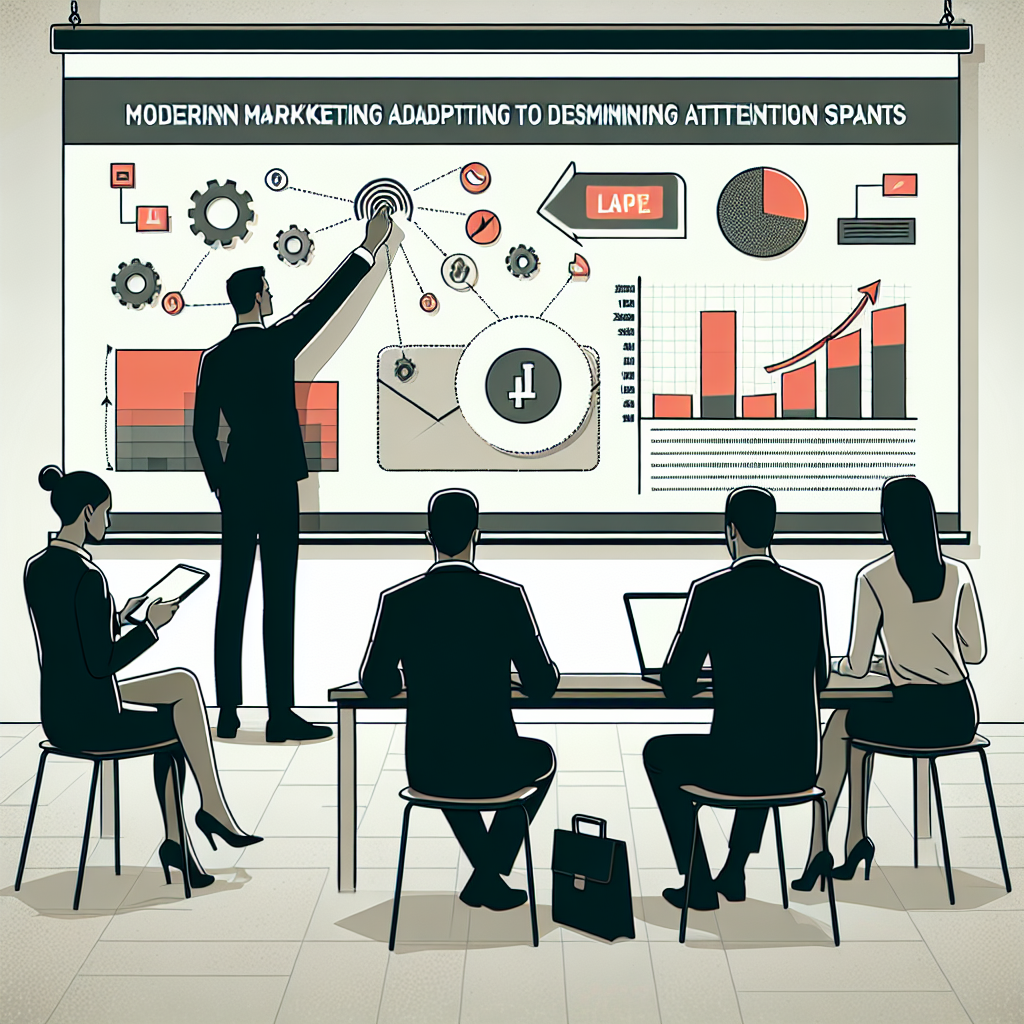
How the Attention Recession Is Changing Marketing
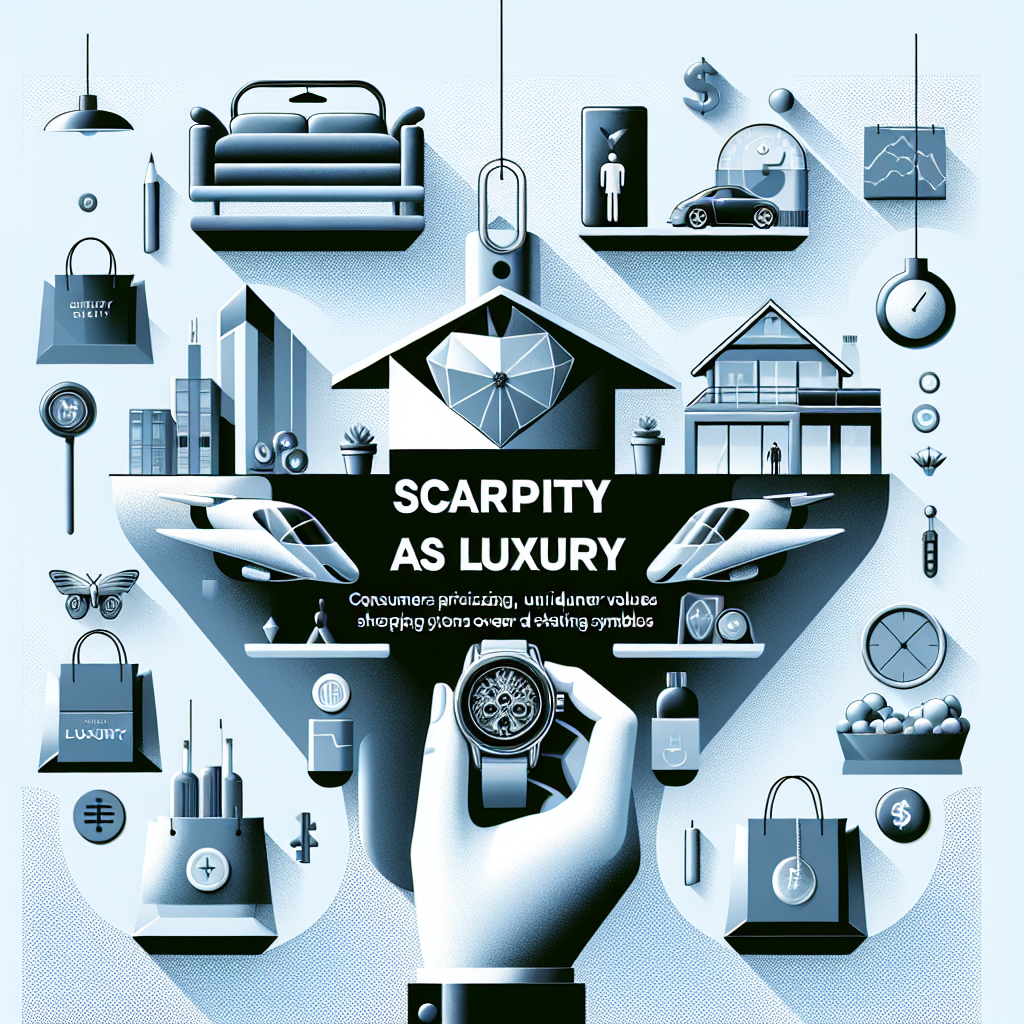
The New Luxury Why Consumers Now Value Scarcity Over Status
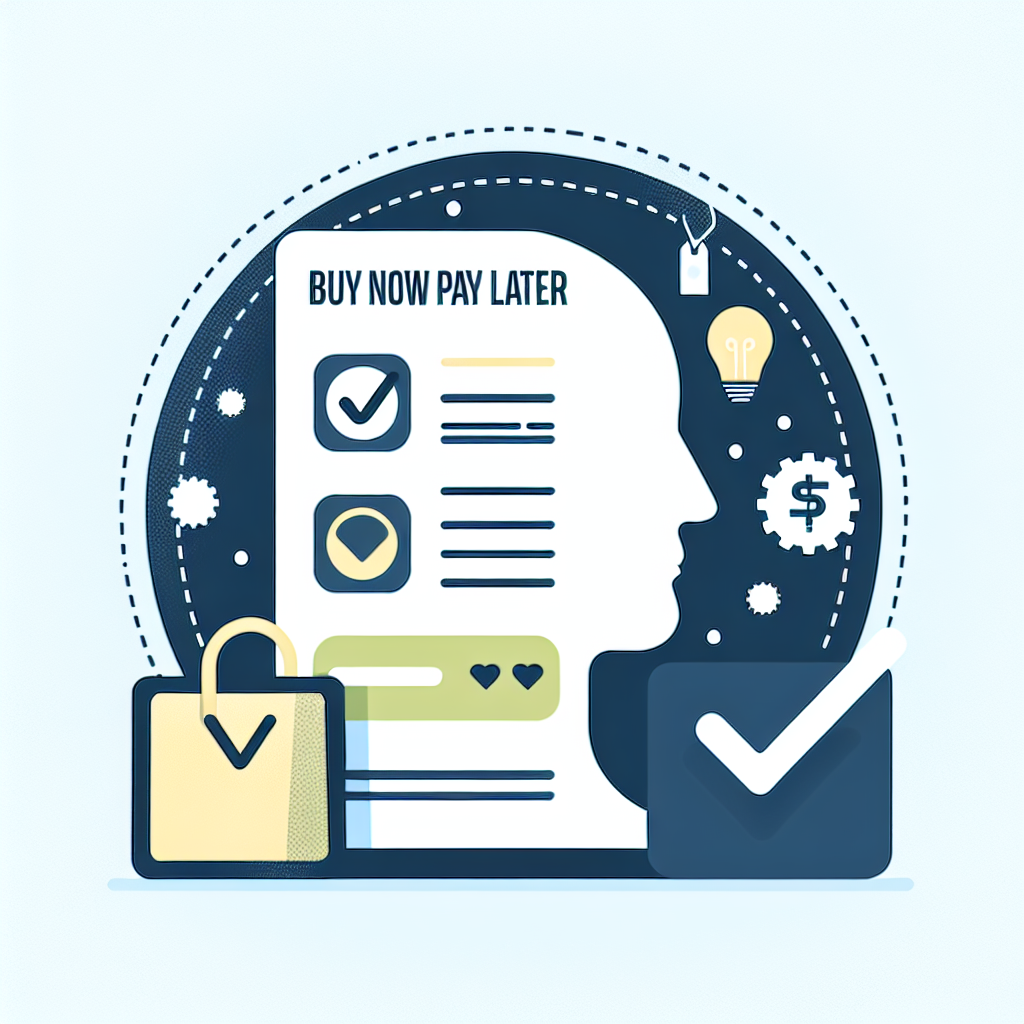
The Psychology Behind Buy Now Pay later
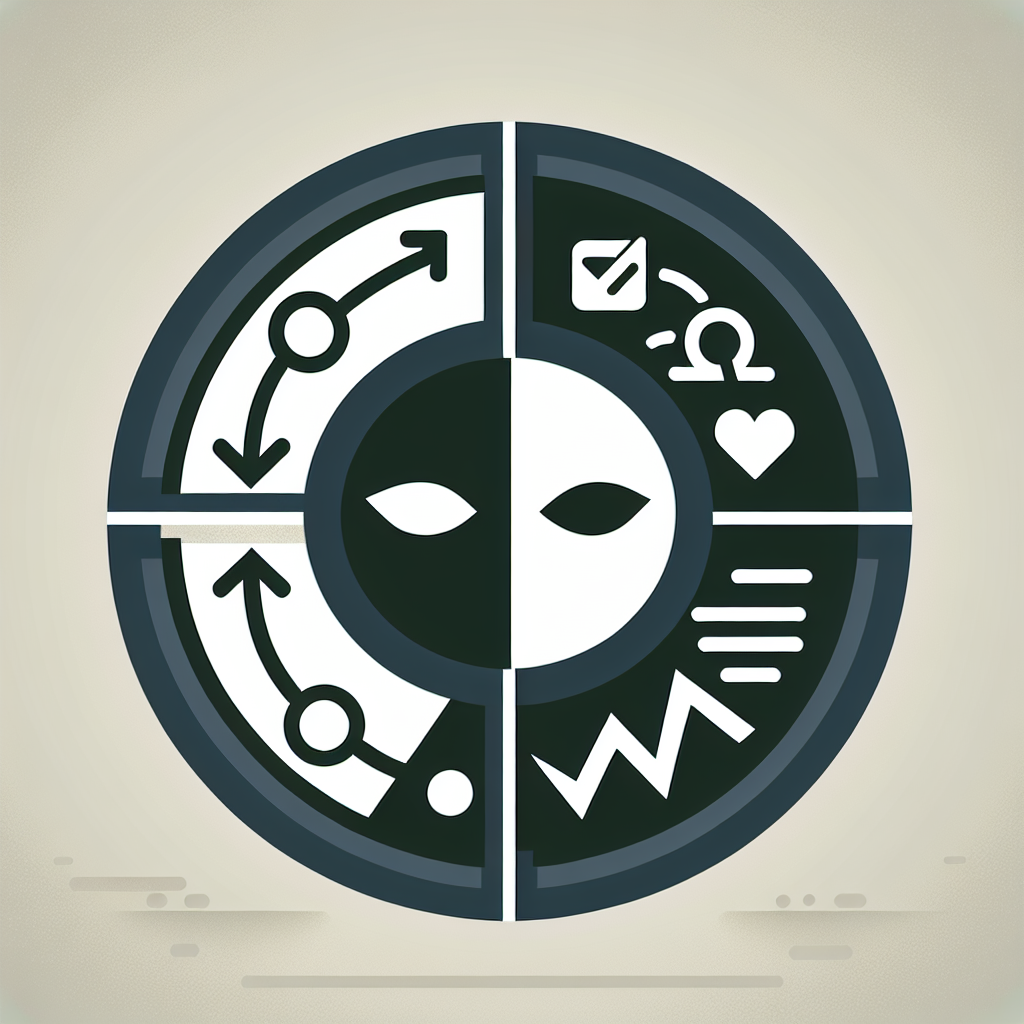
The Role of Dark Patterns in Digital Marketing and Ethical Concerns
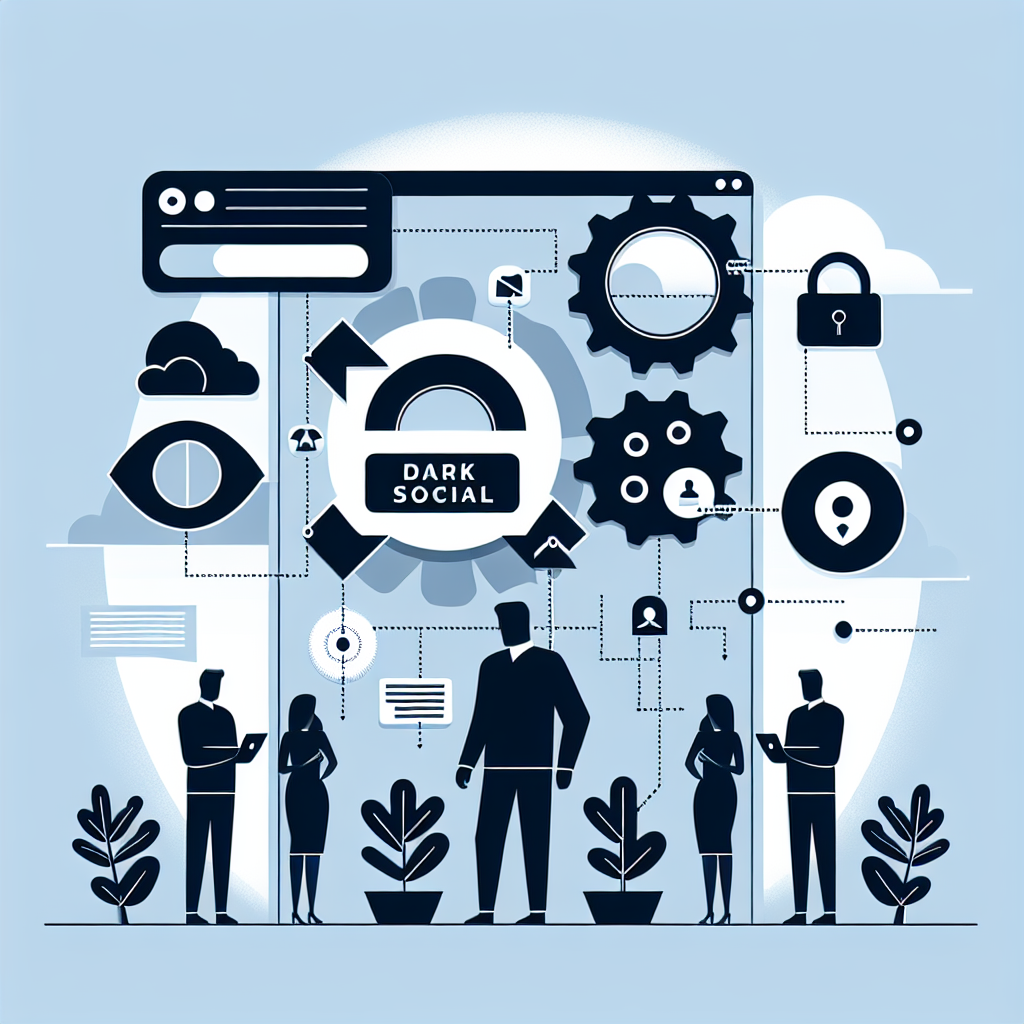
The Rise of Dark Social and Its Impact on Marketing Measurement
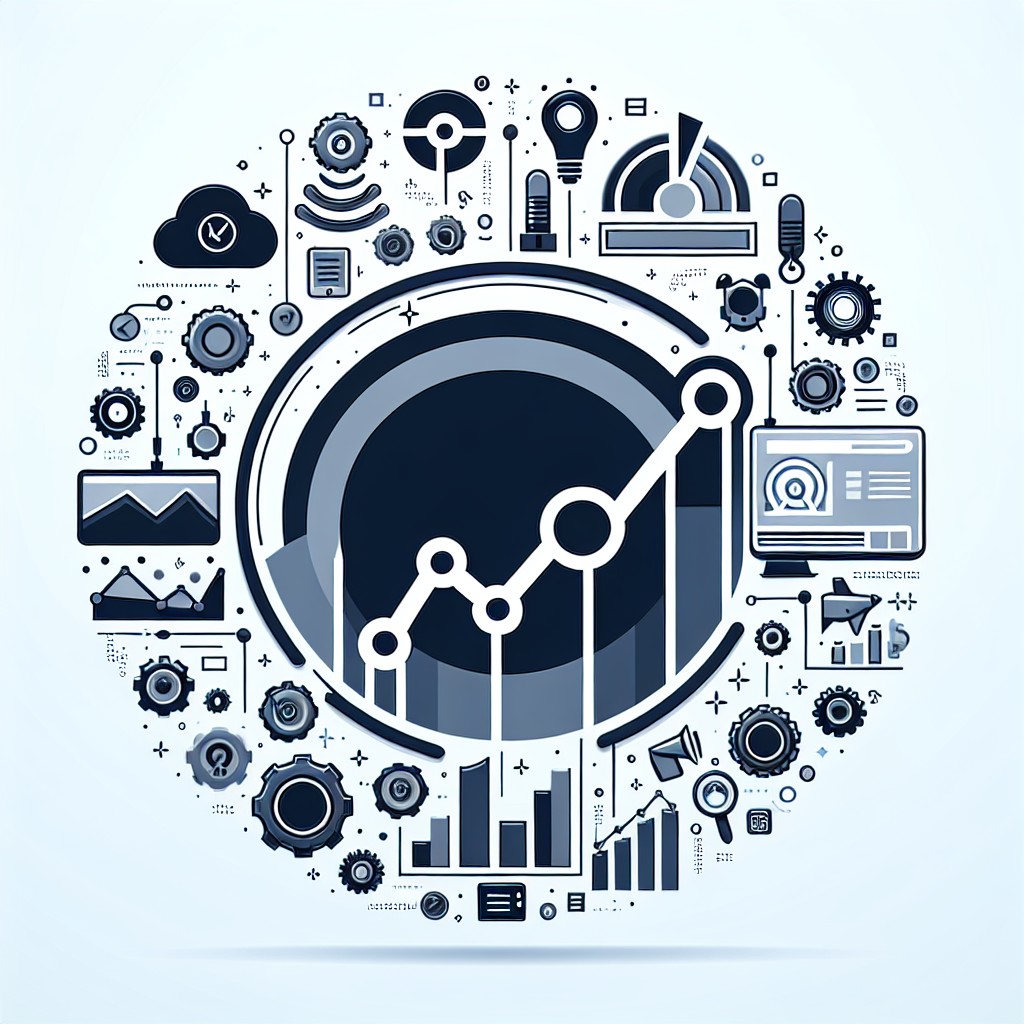