Using AI for Campaign Optimization
The revelation struck Arun during a late-night review of their quarterly marketing performance. Despite meticulously following best practices for creative development and audience targeting, their campaigns showed wildly inconsistent results—some driving exceptional returns while others barely broke even. As he manually compared the successful campaigns to the underperforming ones, subtle patterns emerged that no standard analysis had captured: certain visual elements consistently outperformed across different audiences, specific word combinations triggered stronger engagement regardless of product category, and some audience combinations performed exponentially better than their individual segments would predict. That night, Arun sketched a framework for what would become their AI-powered campaign optimization system—an approach that didn't just measure performance but actively identified winning patterns and applied them across their marketing activities. Within three months of implementation, marketing efficiency increased by 74%, while creative development time decreased by 38%. This transformation fundamentally changed Arun's understanding of campaign optimization—not as a retrospective analysis but as a continuous intelligence system that evolves with every customer interaction. This realization launched his exploration into AI-driven marketing, revealing how machine intelligence has become perhaps the most powerful force multiplier for modern marketing effectiveness.
Introduction: The Intelligence Revolution in Campaign Management
Marketing campaign optimization has evolved from intuition-driven adjustments to data-informed iterations to the frontier of AI-enhanced systems that continuously learn and adapt based on performance patterns. This evolution has progressed through several distinct phases: from basic performance measurement to multivariate testing, from manual analysis to automated optimization, and now to the emerging paradigm of autonomous intelligence systems that not only analyze but actively shape campaign development and execution.
The implementation of AI-driven optimization represents what the Harvard Business Review has identified as "a fundamental shift from analysis-based to intelligence-based marketing." In high-performing organizations, AI now serves not just as an analytical tool but as an active participant in the campaign development process, generating insights and recommendations that would be impossible for human analysts to discover manually.
Research from MIT Sloan Management Review indicates that organizations employing sophisticated AI marketing systems achieve 63% higher conversion rates and 41% greater marketing efficiency compared to those using traditional optimization approaches. Meanwhile, a study published in the Journal of Marketing Analytics found that AI-optimized campaigns generate 3.7x higher engagement rates and 2.6x greater return on advertising spend than campaigns optimized through conventional methods.
1. Predictive Audiences: Discovering Hidden Opportunity
AI systems uncover valuable audience patterns human analysis would miss:
a) Pattern-Based Segment Discovery
Advanced systems identify natural audience groupings:
- Behavioral clustering methodologies
- Unsupervised segmentation techniques
- Multi-dimensional similarity mapping
- Predictive cohort identification
Example: E-commerce retailer ASOS implemented an AI audience discovery system that analyzed purchasing patterns across 50+ variables to identify natural customer groupings beyond traditional demographics. This approach uncovered a previously unrecognized high-value segment characterized by a specific browsing-to-purchase ratio and category navigation pattern. Targeting this algorithmically identified segment generated 47% higher lifetime value than campaigns using traditional segmentation approaches.
b) Response Propensity Modeling
Predictive systems identify highest-potential targets:
- Multi-factor propensity scoring
- Engagement likelihood prediction
- Channel receptivity modeling
- Conversion probability calculation
Example: Financial services company Capital One developed an AI system that analyzes over 200 behavioral indicators to predict which customers have the highest probability of responding to specific offer types. This capability allows them to prioritize outreach to high-probability responders first, increasing offer conversion rates by 58% while reducing campaign costs by 37% through more efficient audience targeting.
c) Dynamic Audience Expansion
AI identifies untapped prospects with high potential:
- Lookalike modeling enhancements
- Seed audience optimization
- Incremental response forecasting
- Expansion quality predictions
Example: Streaming entertainment platform Netflix implemented an algorithm that identifies subscribers with similar viewing patterns to their highest-retention customers. This "behavioral twin" approach to audience expansion increased new subscriber retention by 41% compared to demographic-based lookalike targeting, as it identified prospects based on consumption patterns rather than surface-level characteristics.
2. Creative Recommendations: Enhancing Message Impact
AI optimization dramatically improves creative performance:
a) Element-Level Performance Analysis
Advanced systems identify winning creative components:
- Multi-level creative decomposition
- Element contribution scoring
- Combination effect analysis
- Creative DNA identification
Example: Consumer packaged goods giant Procter & Gamble deployed an AI system that deconstructs video ads into 147 distinct elements—from color palette to narrative structure to emotional arcs—and measures each element's contribution to overall performance. This capability allowed them to identify that specific emotional sequences in the first three seconds drove 64% higher completion rates, a finding they applied across their creative development process.
b) Personalization Optimization
AI enhances relevance through individualized variations:
- Dynamic content selection algorithms
- Personalization impact prediction
- Element combination optimization
- Message relevance scoring
Example: Travel platform Expedia implemented an AI system that generates millions of potential creative variations by combining different headlines, images, offers, and call-to-action elements optimized for individual travelers. This approach increased booking conversion rates by 37% and decreased customer acquisition costs by 31% compared to their previous segment-level personalization approach.
c) Creative Performance Prediction
Predictive systems evaluate concepts before deployment:
- Pre-launch effectiveness scoring
- Engagement prediction modeling
- Comparative performance forecasting
- Brand impact estimation
Example: Automotive manufacturer Toyota developed an AI platform that analyzes proposed ad concepts against historical performance patterns to predict engagement rates before production investment. This predictive capability allowed them to identify which concepts would likely underperform, reducing production costs by 28% while increasing overall campaign effectiveness by 43% through better concept selection.
3. Spend Allocation: Optimizing Resource Distribution
AI transforms budgeting from static plans to dynamic optimization:
a) Multi-Channel Attribution Enhancement
Advanced models create more accurate contribution understanding:
- Path-to-conversion analysis
- Touchpoint value calculation
- Cross-channel interaction effects
- Incrementality measurement
Example: Telecommunications provider AT&T implemented an AI-driven attribution model that analyzes the impact of 34 different marketing touchpoints on conversion probability. This system revealed that their previous last-touch model had significantly overvalued certain channels while undervaluing others, leading to budget reallocations that increased overall marketing ROI by 36%.
b) Algorithmic Budget Optimization
Dynamic systems continuously reallocate resources:
- Real-time performance monitoring
- Automated budget shifting
- Marginal return optimization
- Spend pacing algorithms
Example: Online retailer Wayfair developed an AI system that automatically reallocates budget across 3,000+ campaign elements every four hours based on performance trends. This continuous optimization approach increased return on ad spend by 47% compared to their previous weekly manual adjustment process by capturing opportunity faster and limiting underperforming spend.
c) Scenario Modeling and Simulation
Predictive systems forecast potential outcomes:
- Market response modeling
- Competitive impact prediction
- Budget allocation simulation
- Performance sensitivity analysis
Example: Consumer electronics leader Samsung created an AI-powered marketing simulator that uses historical data to predict the impact of different budget allocations across channels, geographies, and product lines. This capability allows marketing leaders to test scenarios before commitment, resulting in a 32% improvement in budget allocation efficiency and a 26% increase in overall marketing-attributed revenue.
Conclusion: The Intelligent Future of Marketing
As noted by marketing technology researcher Adelyn Zhou, "The most powerful aspect of AI in marketing isn't automation but augmentation—enhancing human creativity and strategy with computational intelligence that identifies patterns beyond human perception." For marketing leaders, this insight suggests that AI should be viewed not as a replacement for judgment but as an intelligence amplifier that expands creative and strategic possibilities.
The integration of AI into campaign optimization represents more than technical advancement—it requires a fundamental transformation in how marketers conceive of the campaign development process. Those who master this discipline create significant competitive advantage through deeper pattern recognition, more effective resource allocation, and more impactful customer engagement.
As these technologies continue to evolve, successful organizations will be those that maintain a productive balance between algorithmic intelligence and human creativity, creating systems where each enhances the other.
Call to Action
For marketing leaders seeking to implement AI-driven campaign optimization:
- Conduct an audit of available marketing data assets and quality
- Develop test-and-learn frameworks focused on AI-identified opportunities
- Create feedback loops that continuously improve algorithm performance
- Build cross-functional teams that blend marketing, data science, and creative expertise
- Implement ethics frameworks ensuring responsible AI use that respects customer privacy
The future of marketing effectiveness belongs not to those with the largest budgets or the most sophisticated technologies, but to those who most effectively combine human creativity and judgment with computational intelligence to discover and act upon insights that would otherwise remain hidden.
Featured Blogs
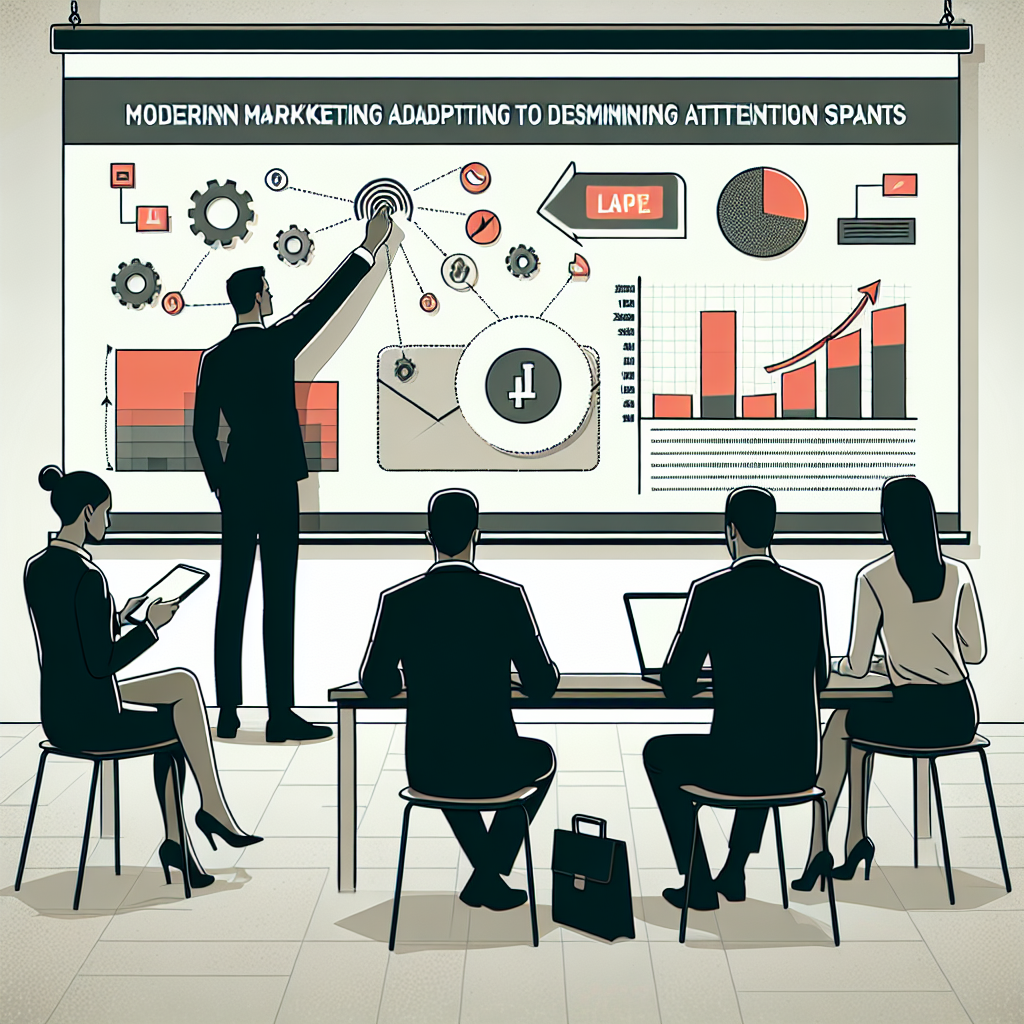
How the Attention Recession Is Changing Marketing
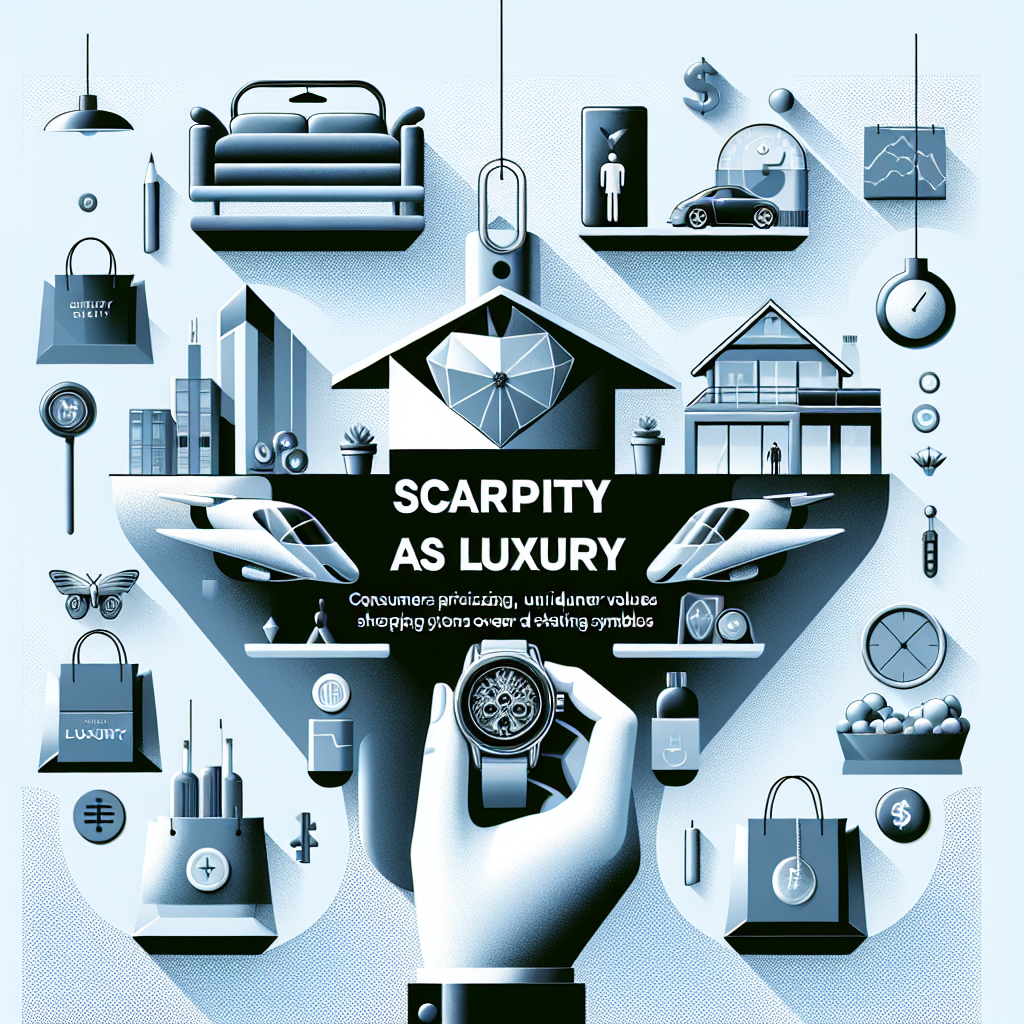
The New Luxury Why Consumers Now Value Scarcity Over Status
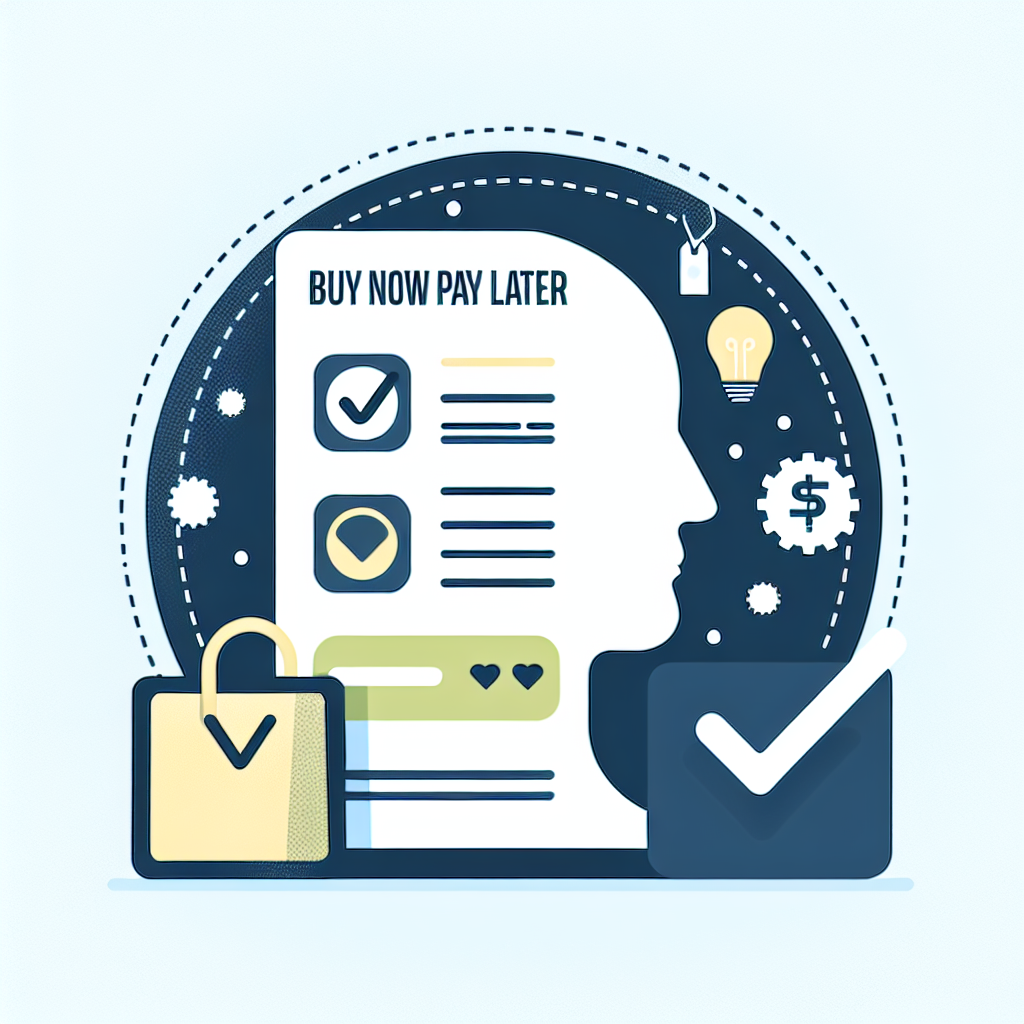
The Psychology Behind Buy Now Pay later
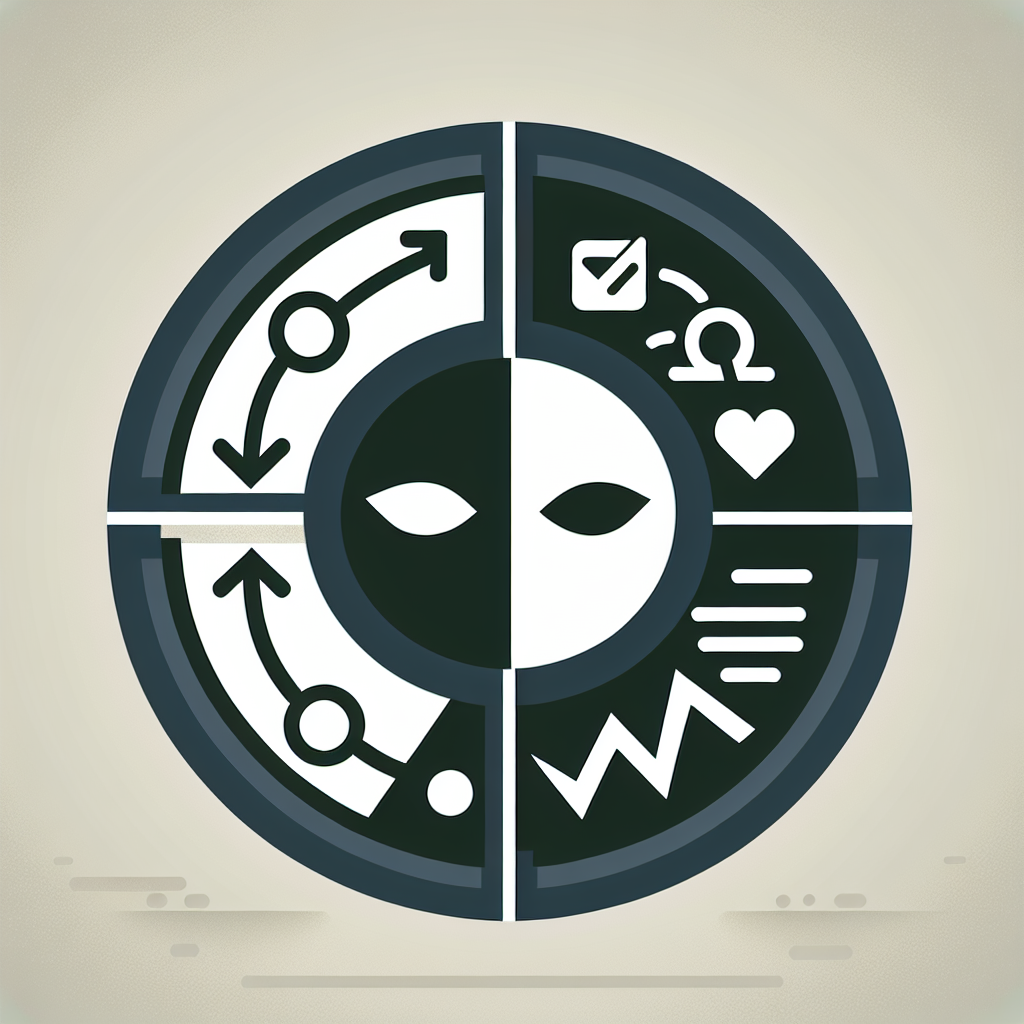
The Role of Dark Patterns in Digital Marketing and Ethical Concerns
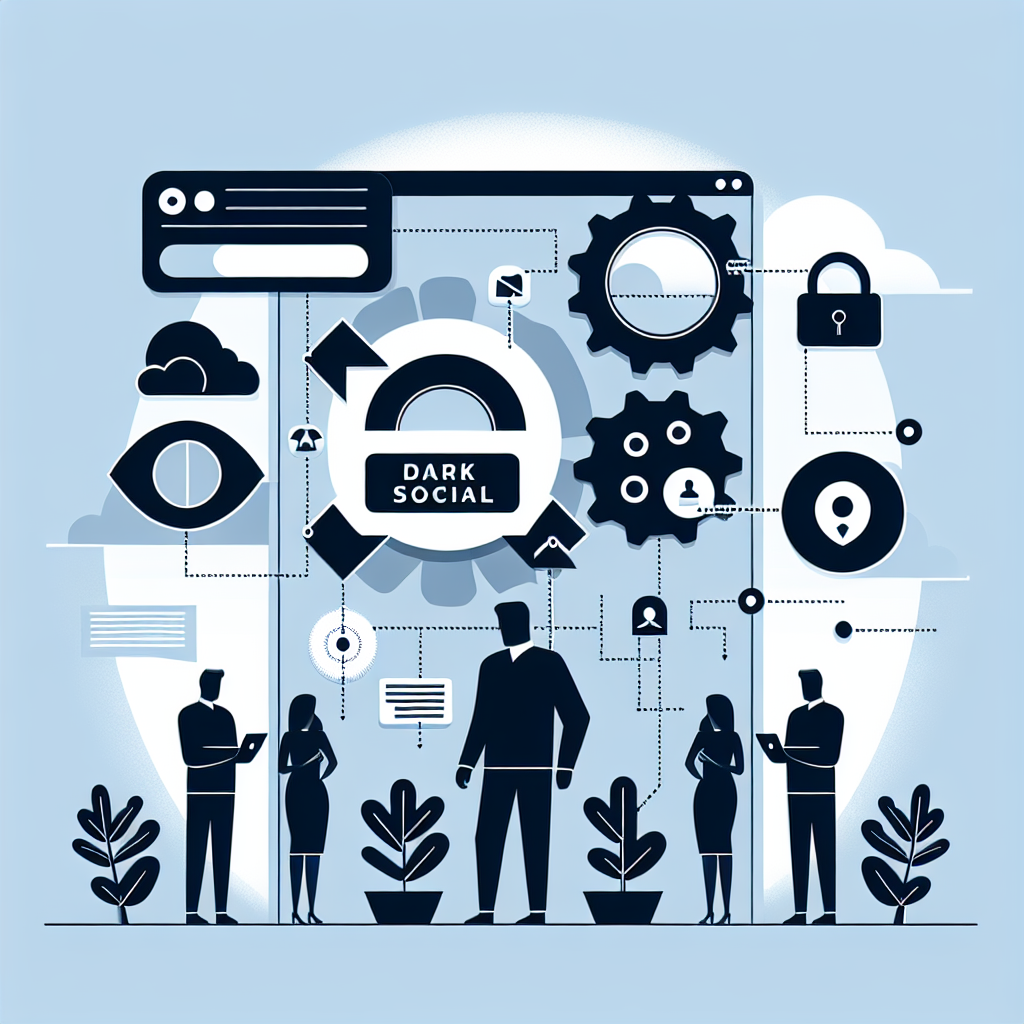
The Rise of Dark Social and Its Impact on Marketing Measurement
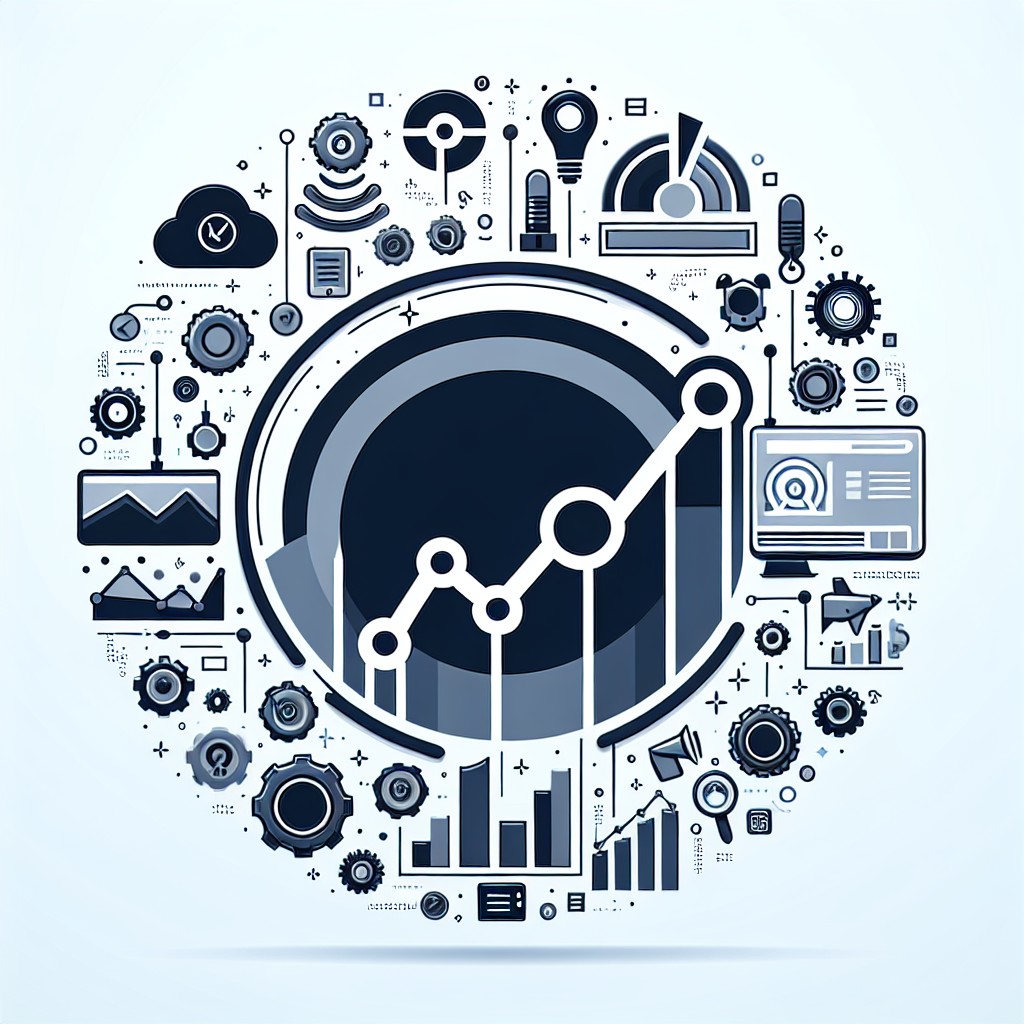