AI-Powered Copywriting and Creative Generation
The revelation came to Arun during a critical product launch when the copywriting team fell behind schedule. With hours remaining before the deadline, he reluctantly turned to an AI writing assistant to generate initial drafts for the campaign. As he browsed through the generated headlines and copy, Arun was struck by a peculiar mix of brilliance and blandness—some concepts were genuinely compelling, while others felt generic and lifeless. After carefully editing and testing the AI outputs against their typical human-written copy, they discovered something unexpected: the AI-assisted campaigns performed 43% better in A/B tests, but only after human refinement. The experience completely reshaped Arun's understanding of creative workflows. What began as a desperate deadline solution evolved into a fundamental rethinking of how humans and machines could collaborate in creative processes. The machines weren't replacing copywriters—they were transforming them into creative directors, focusing their talents on conceptual strategy and refinement rather than first-draft production. This experience launched Arun's exploration into the emerging field of AI-augmented creativity, revealing how this partnership between human and artificial intelligence is reshaping the creative landscape.
Introduction: The AI Revolution in Creative Production
Marketing content creation has evolved from manual production to increasingly augmented workflows. This evolution has progressed through distinct phases: from fully human creation to template-based production, from rules-based generation to early algorithmic approaches, and now to the frontier of AI-powered creative systems that can generate sophisticated marketing content with minimal human guidance.
The advancement of generative AI in creative contexts represents what the World Economic Forum has called "the most significant shift in creative production since desktop publishing"—transforming not just creation speed but the fundamental relationship between humans and creative processes.
Research from McKinsey indicates that marketers using AI-assisted creative workflows achieve 37% higher productivity and reduce content production costs by 42% compared to traditional approaches. Meanwhile, a study published in the Harvard Business Review found that AI-human creative collaborations scored 35% higher on originality and 28% higher on effectiveness compared to either human-only or AI-only outputs.
As creativity researcher Margaret Boden notes in her framework of creativity types, computational systems excel at "combinatorial creativity"—recombining existing elements in novel ways—while humans maintain advantages in "transformational creativity" that changes conceptual spaces themselves. The most effective approaches leverage the complementary strengths of both.
1. Prompt Engineering Basics
The foundation of effective AI-powered content creation lies in sophisticated prompt design.
Instruction Design Principles
Modern prompt engineering follows established frameworks:
- Context setting and background inclusion
- Constraint specification and boundary definition
- Stylistic guidance and voice calibration
- Example provision and few-shot learning
Example: Samsung's digital marketing team developed a "prompt architecture" with three distinct components: brand voice parameters, campaign objective framing, and specific structural requirements. This standardized approach increased usable AI output from 23% to 67% on first generation, significantly reducing production cycles.
Model Selection Strategies
Choosing appropriate AI models for specific creative tasks:
- Task-specific model evaluation frameworks
- Capability-requirement alignment methodologies
- Fine-tuning versus prompt engineering trade-offs
- Model temperature and parameter optimization
Example: Ogilvy's AI creative lab established a "model matchmaking" system that pairs specific creative needs with optimized language models. Their testing revealed that sentiment-focused content performs 31% better with specific emotion-trained models, while technical product descriptions require models optimized for accuracy over creativity.
2. Review Systems
Beyond generation, sophisticated quality control systems ensure AI outputs meet creative standards.
Quality Assurance Frameworks
Systematic evaluation methods for AI-generated content:
- Multi-dimensional quality scoring matrices
- Brand safety and compliance verification
- Originality and plagiarism detection
- Voice consistency measurement
Example: L'Oréal implemented a three-stage AI content review system that evaluates outputs across 17 dimensions including brand alignment, cultural sensitivity, and regulatory compliance. This approach reduced publication errors by 87% and decreased approval cycles by 64% compared to their previous workflow.
Human-in-the-Loop Collaboration Models
Structured approaches for human-AI creative partnerships:
- Iterative refinement workflows
- Expert guidance systems
- Feedback incorporation methods
- Learning loop implementation
Example: The New York Times experimented with an "AI augmentation" system for headline generation that presents writers with AI-generated alternatives alongside engagement predictions. Writers using the system produced headlines that performed 22% better in A/B tests while reporting higher job satisfaction through reduced creative pressure.
3. A/B Testing AI vs. Human
Empirical evaluation approaches determine optimal human-AI collaboration points.
Performance Comparison Frameworks
Systematic testing methodologies compare creative sources:
- Controlled creative experimentation design
- Multi-variant testing approaches
- Performance attribution models
- Statistical significance determination
Example: Spotify developed a "Creative Origin Testing" framework that compared fully human, AI-assisted, and AI-generated copy across 1,200 ad variations. The results revealed that AI-generated headlines outperformed human versions by 19% on click-through rates, while human-written body copy outperformed AI by 23% on conversion metrics—leading to a hybrid approach optimized for each component.
Audience Response Differentiation
Nuanced understanding of audience responses to AI-generated content:
- Demographic response pattern analysis
- Engagement depth comparison
- Emotional response measurement
- Trust and authenticity perception
Example: Unilever conducted extensive testing comparing audience responses to disclosed AI content versus undisclosed AI content versus human content. They discovered that Generation Z showed no statistical preference between sources, while older demographics demonstrated 17% higher trust in human-created content—informing segment-specific creative strategies.
Conclusion: The AI Future of Marketing Creativity
As creativity researcher Sir Ken Robinson observed: "Creativity is not a separate faculty but the result of interactions between different disciplinary ways of seeing things." The emerging partnership between human creativity and artificial intelligence represents precisely this type of interaction—combining computational pattern recognition with human conceptual understanding to create approaches neither could achieve alone.
The integration of AI into creative workflows represents more than efficiency improvement—it fundamentally transforms the creative process itself, shifting human focus from production to direction and enabling exploration of creative possibilities at unprecedented scale and speed.
As these technologies mature, the traditional boundaries between creative ideation and production will continue to blur, creating unified creative workflows where AI handles routine production while human creativity focuses on strategic differentiation and emotional resonance.
Call to Action
For marketing leaders looking to pioneer AI-augmented creative approaches:
- Develop prompt engineering capabilities as a core creative competency
- Build structured testing programs comparing creative sources across channels
- Create feedback mechanisms that continuously improve AI creative quality
- Establish ethical guidelines addressing authenticity, disclosure, and creative attribution
- Implement training programs helping creative teams transition to AI-augmented workflows
The future of marketing creativity belongs not to those who reject AI nor to those who rely on it exclusively, but to those who develop sophisticated collaboration models leveraging the complementary strengths of human and artificial intelligence.
Featured Blogs
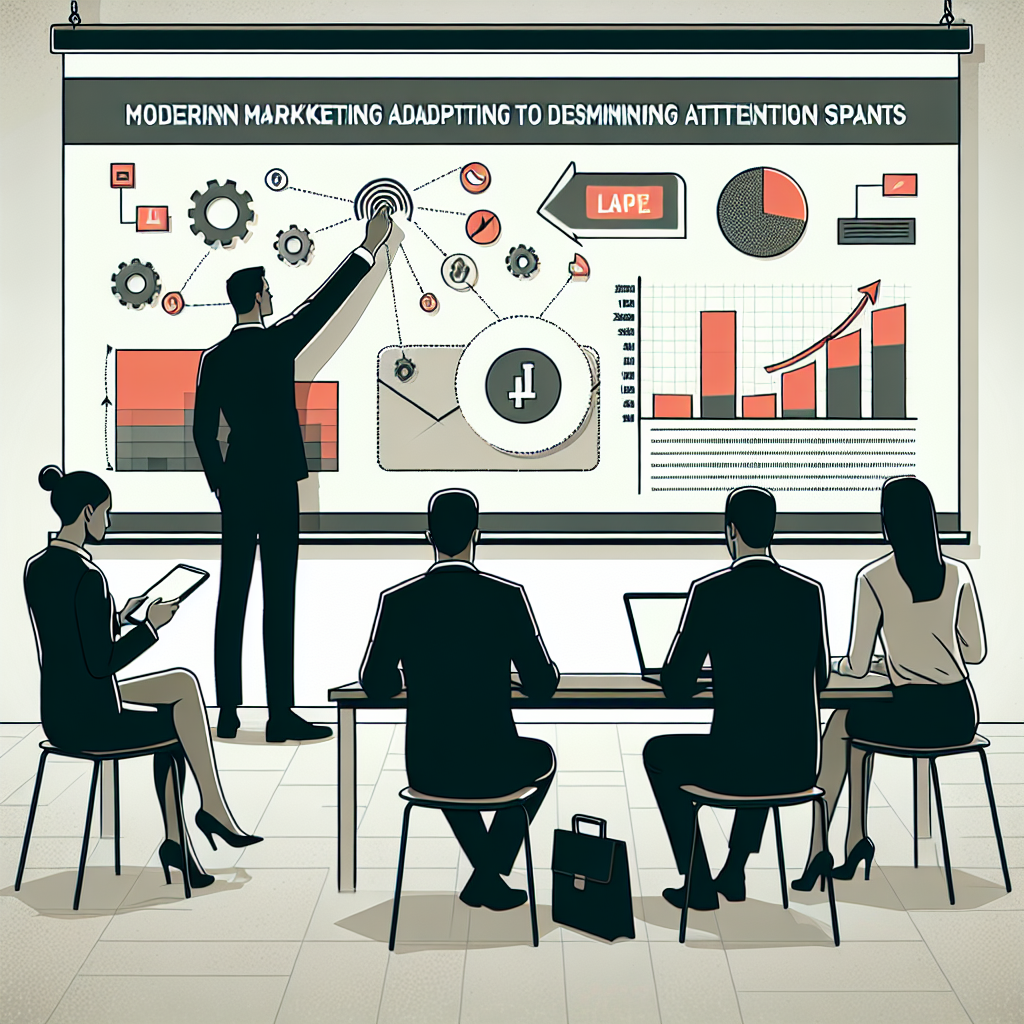
How the Attention Recession Is Changing Marketing
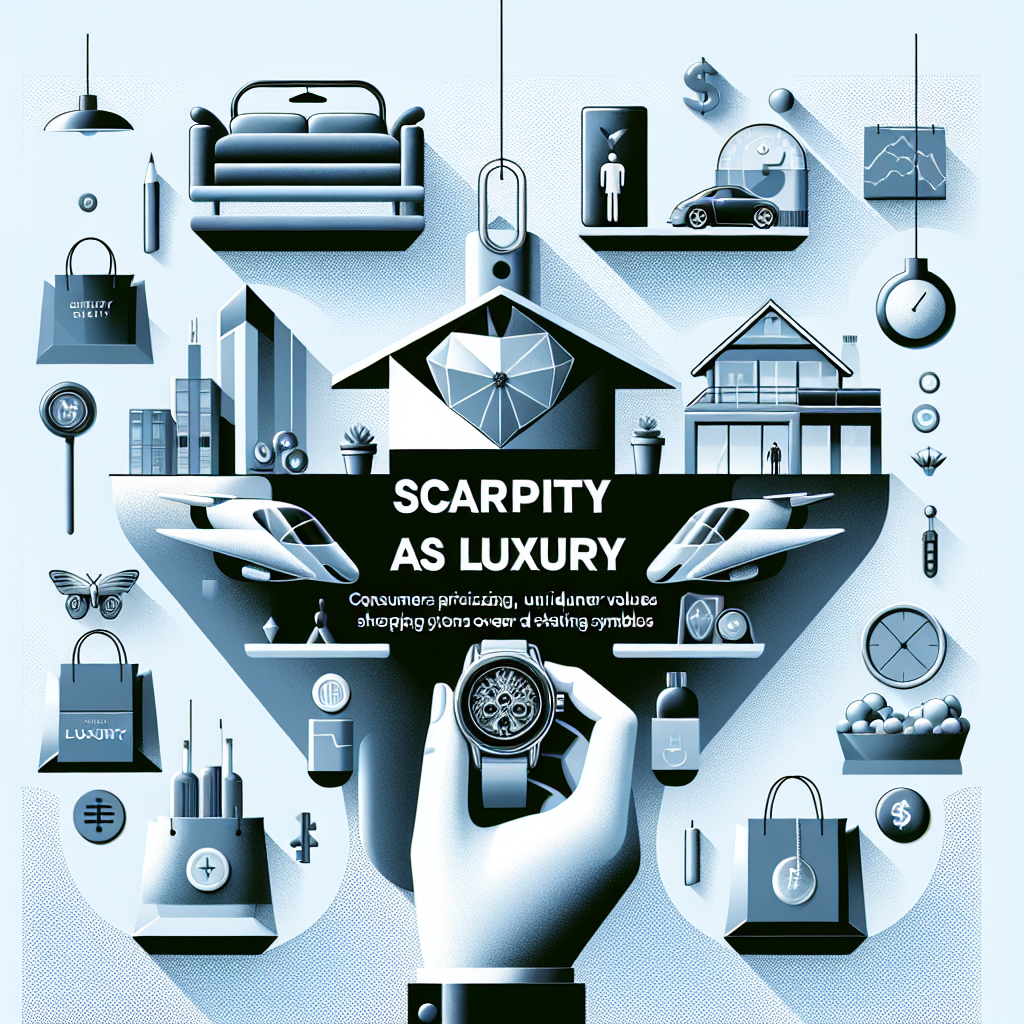
The New Luxury Why Consumers Now Value Scarcity Over Status
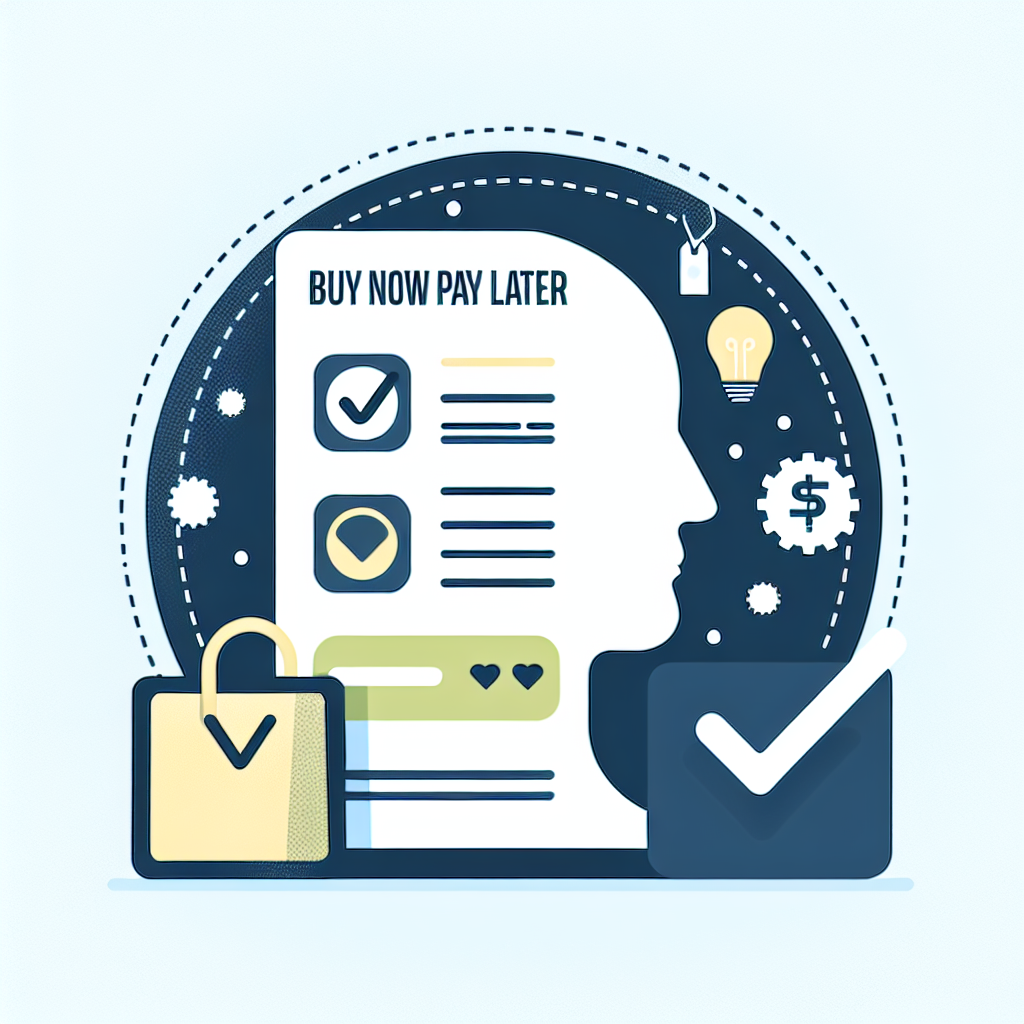
The Psychology Behind Buy Now Pay later
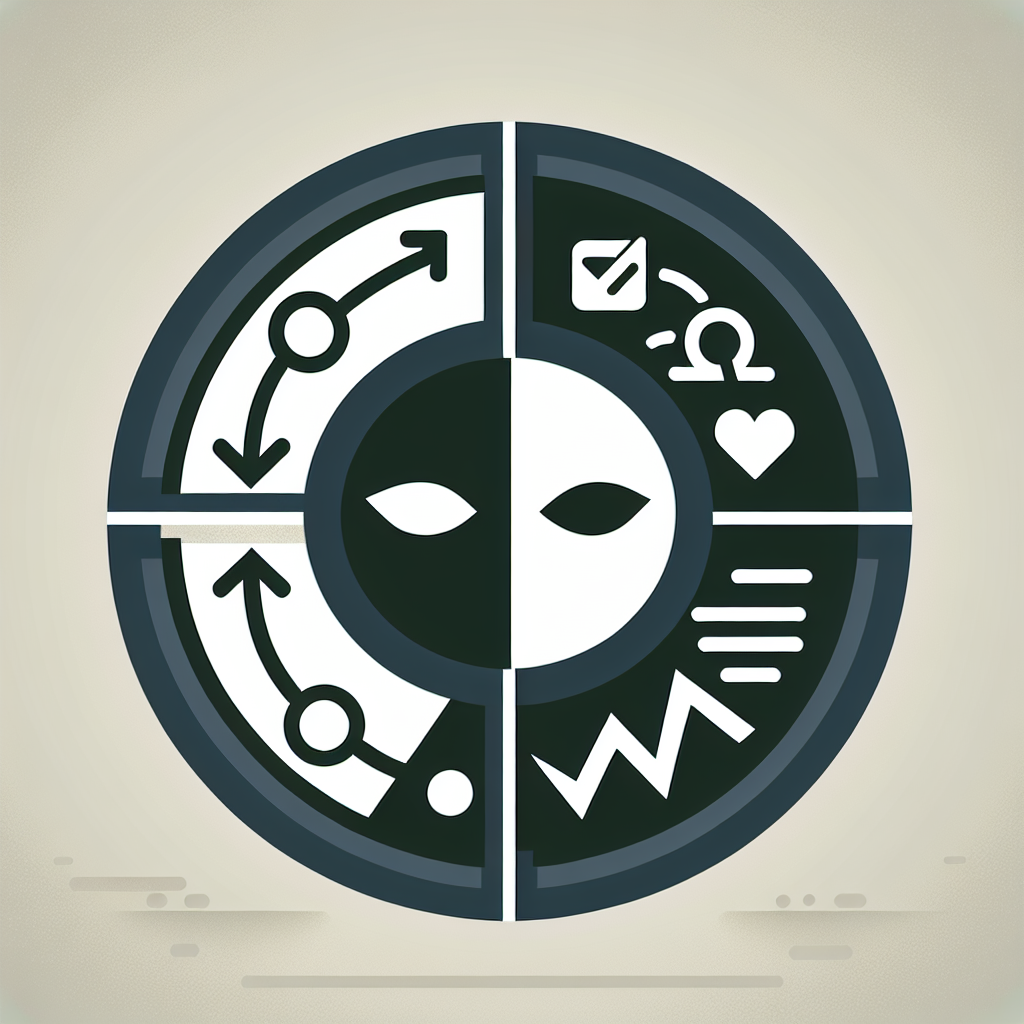
The Role of Dark Patterns in Digital Marketing and Ethical Concerns
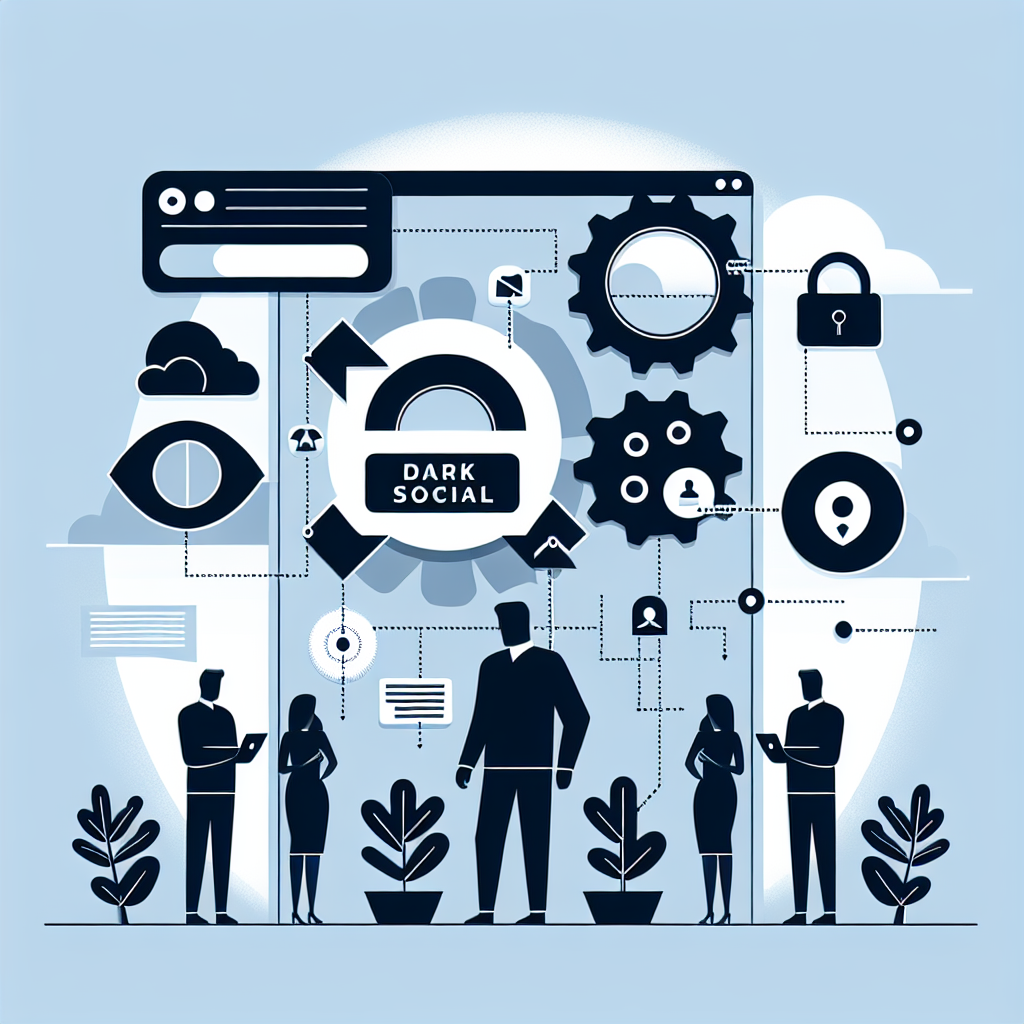
The Rise of Dark Social and Its Impact on Marketing Measurement
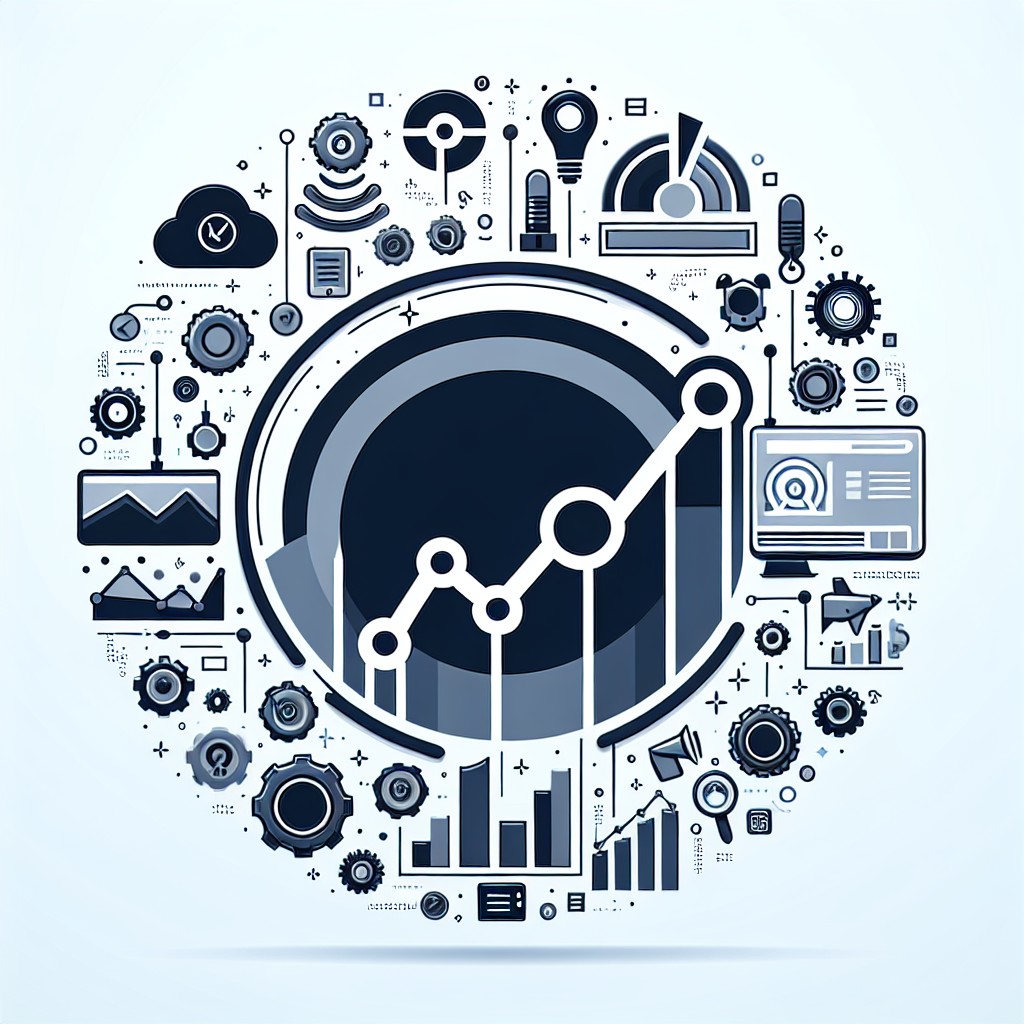