The Role of AI in Product Recommendation Engines
Introduction: The Evolution of Personalization in Digital Commerce
In the modern digital marketplace, consumers face an unprecedented paradox of choice. E-commerce platforms offer millions of products, content streaming services provide vast libraries, and information sources produce unlimited content—creating both opportunity and overwhelming complexity for consumers. This challenge has catalyzed the development of increasingly sophisticated product recommendation engines, which have evolved from basic rule-based systems to complex artificial intelligence algorithms capable of understanding and predicting consumer preferences with remarkable accuracy. According to research from Boston Consulting Group, companies using advanced personalization strategies see revenue increases of 6-10%, rates two to three times faster than companies with less developed personalization capabilities. At the heart of this transformation are AI-powered recommendation systems that analyze vast datasets of consumer behavior, contextual signals, and product attributes to deliver hyper-personalized suggestions. As Wharton professor Peter Fader notes in his customer-centricity framework, "Not all customers are created equal"—a principle that recommendation engines operationalize at scale. This article examines how AI is revolutionizing product recommendations, the underlying algorithms and strategies, implementation challenges, and the future trajectory of personalized discovery in an increasingly AI-mediated commercial environment.
1. The Technical Evolution: From Rules to Intelligence
Recommendation systems have progressed through several generations of increasing sophistication:
a) Algorithmic Foundations and Approaches
Modern recommendation engines employ diverse methodologies:
- Collaborative filtering: Leveraging similarities between user preferences
- Content-based filtering: Analyzing item attributes and user preferences
- Knowledge-based recommendations: Utilizing explicit knowledge about users, items, and domain expertise
- Deep learning models: Neural networks that identify complex, non-linear patterns in user behavior
b) From Static to Dynamic Personalization
Recommendation systems have evolved from batch processing to real-time adaptation:
- Early systems relied on overnight recalculations of recommendations
- Contemporary platforms adjust in real-time based on browsing behavior
- Contextual awareness now incorporates time, location, device, and even weather
c) Multimodal Data Integration
Advanced systems leverage diverse data sources:
- Behavioral data: Clicks, views, purchase history, dwell time
- Explicit preferences: Ratings, reviews, saved items
- Visual and semantic analysis: Image recognition of product features and style
- Voice and natural language processing for preference extraction
2. Strategic Business Applications Across Industries
AI-powered recommendations drive value across diverse sectors:
a) Retail and E-commerce Transformation
The impact of recommendation engines on digital commerce is profound:
- Example: Amazon attributes up to 35% of its revenue to its recommendation engine
- Fashion retailer ASOS implemented visual search and style-matching AI, increasing average order value by 3% and conversion by 2%
b) Content and Media Personalization
Recommendation engines have reshaped media consumption patterns:
- Example: Netflix's recommendation system saves an estimated $1 billion annually by reducing churn
- Spotify's Discover Weekly uses collaborative filtering and natural language processing of playlist descriptions to create personalized music recommendations with 40% playlist add rates
c) Financial Services and Complex Products
Even highly regulated industries benefit from recommendation intelligence:
- Example: Bank of America's Life Plan platform provides personalized financial product recommendations based on life goals
- Insurance provider Progressive uses AI to recommend appropriate coverage levels based on customer profiles
3. The Psychology of Recommendations
Effective recommendation engines align with cognitive decision-making processes:
a) Balancing Familiarity and Discovery
Behavioral economist Dan Ariely's research on choice architecture applies directly to recommendation strategy:
- Serendipity engineering: Intentionally introducing novel but relevant recommendations
- Familiarity ratios: Balancing known preferences with discovery opportunities
- Confidence indicators that signal recommendation strength to users
b) Decision Fatigue and Cognitive Load
Recommendation engines address the paradox of choice identified by psychologist Barry Schwartz:
- Reduction of cognitive effort through curated alternatives
- Progressive disclosure of options rather than overwhelming arrays
- Contextual relevance that simplifies decision complexity
c) Trust Development Through Recommendation Quality
Stanford persuasion researcher BJ Fogg's credibility framework highlights factors affecting recommendation acceptance:
- Explanation interfaces that build algorithmic transparency
- Calibration of recommendation confidence to build trust
- Recovery mechanisms that learn from rejected recommendations
4. Case Studies: Recommendation Excellence in Action
Several organizations demonstrate the strategic impact of advanced recommendation systems:
a) Stitch Fix's Hybrid Intelligence Approach
Stitch Fix combines AI recommendations with human stylists, creating a "centaur model" that pairs algorithmic efficiency with human judgment. This approach has yielded 30% higher client retention than pure algorithm approaches.
b) The Home Depot's Project Contextual Recommendations
Home Depot implemented AI that understands project context, recommending related items to complete home improvement tasks. This contextualization increased project completion rates by 35% and average basket size by 12%.
c) Sephora's Beauty Recommendation Engine
Sephora's system integrates purchase history, skin type, tone, and preferences with visual recognition of user-uploaded photos, creating personalized beauty recommendations that drove a 20% increase in repeat purchases.
5. Implementation Challenges and Emerging Solutions
Several obstacles affect recommendation system implementation:
a) The Cold Start Problem
New users and products present data sparsity challenges:
- Transfer learning from similar users/items
- Multi-armed bandit approaches to efficiently explore preferences
- Zero-shot learning that extrapolates from features rather than history
b) Filter Bubbles and Recommendation Diversity
As Professor Eli Pariser warns in his filter bubble theory:
- Echo chamber risks of over-personalization
- Diversity metrics to ensure breadth of recommendations
- Ethical considerations in preference reinforcement
c) Privacy and Regulatory Considerations
Personalization exists in tension with privacy concerns:
- Federated learning approaches that personalize without centralizing data
- Privacy-preserving techniques like differential privacy
- Transparency requirements under regulations like GDPR and CCPA
Conclusion: The Future of AI-Driven Recommendations
AI-powered recommendation engines represent a fundamental shift in how consumers discover products and content—moving from active search to passive, personalized discovery. As marketing strategist Seth Godin observes, "Don't find customers for your products; find products for your customers." Advanced recommendation engines operationalize this philosophy at scale. The future points toward increasingly sophisticated systems that balance algorithmic precision with human-centered design, privacy consciousness with personalization depth, and commercial objectives with consumer well-being. Organizations that master this balance will create not just conversion opportunities but discovery experiences that build lasting relationships with consumers in an increasingly AI-mediated marketplace.
Call to Action
For business leaders seeking to enhance their recommendation capabilities:
- Audit your current recommendation approach against industry benchmarks
- Develop a comprehensive data strategy that balances personalization with privacy
- Implement A/B testing frameworks to continuously optimize recommendation algorithms
- Consider hybrid approaches combining AI with human expertise
- Establish clear metrics that measure both immediate conversion impact and long-term customer value
Featured Blogs
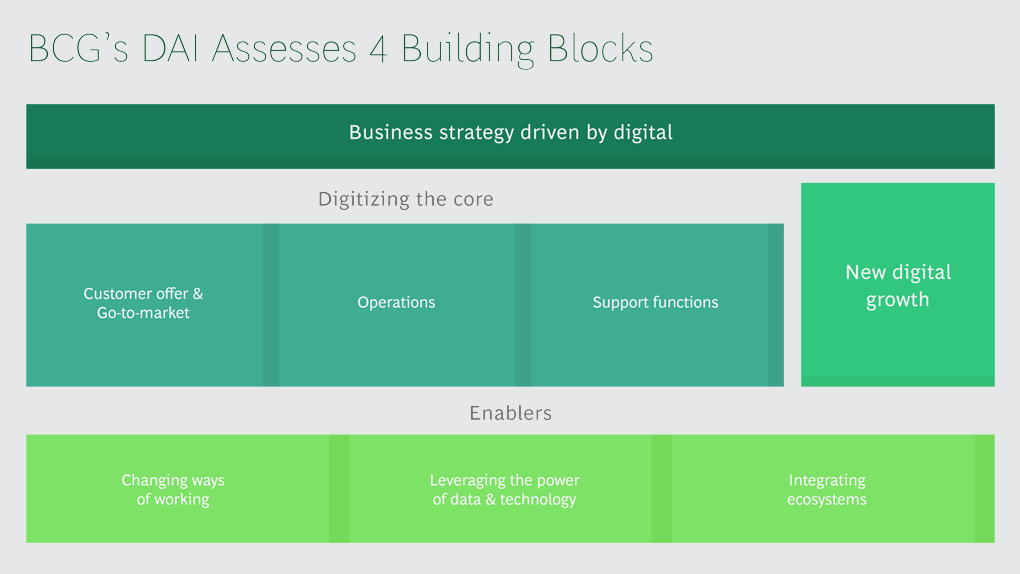
BCG Digital Acceleration Index
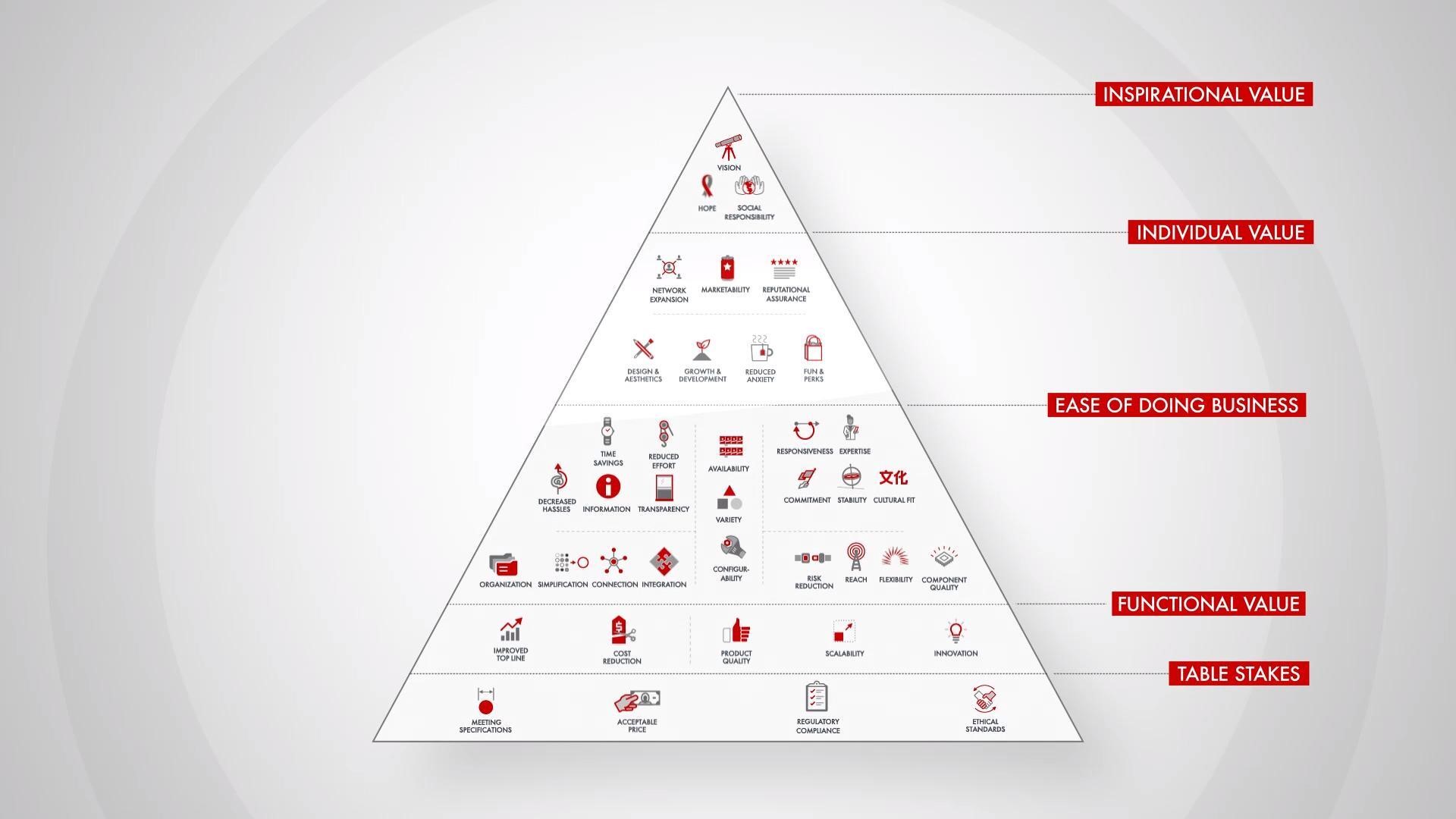
Bain’s Elements of Value Framework
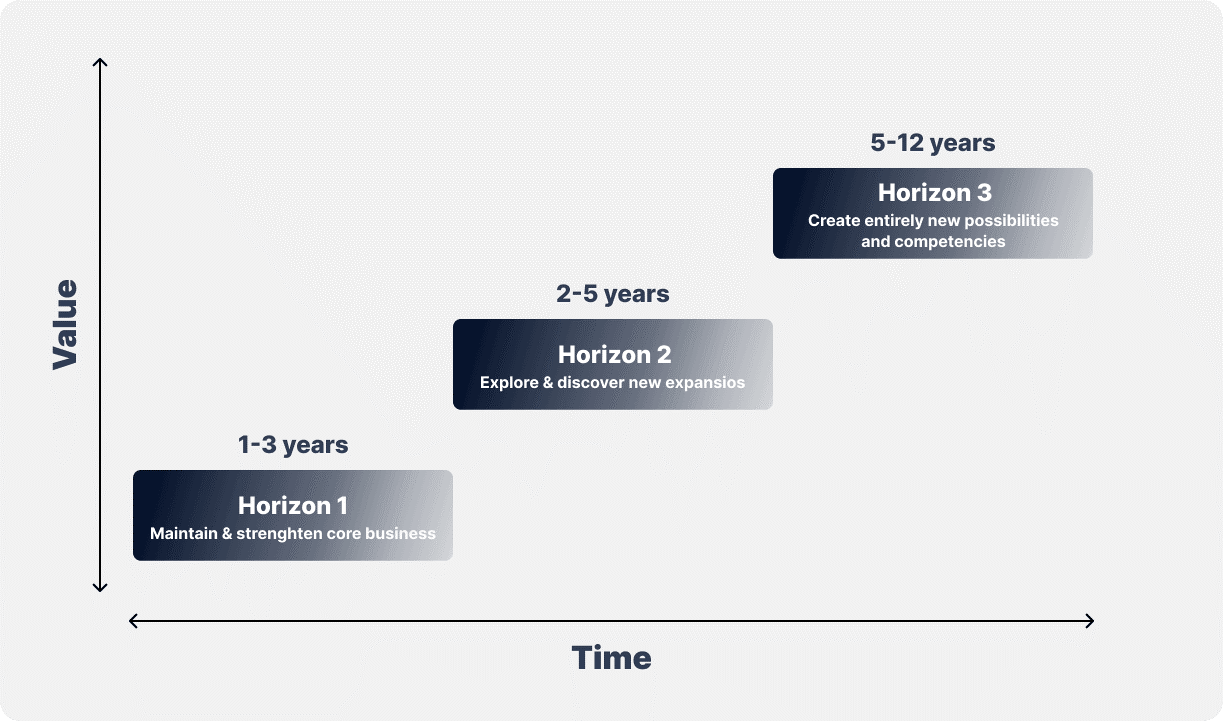
McKinsey Growth Pyramid
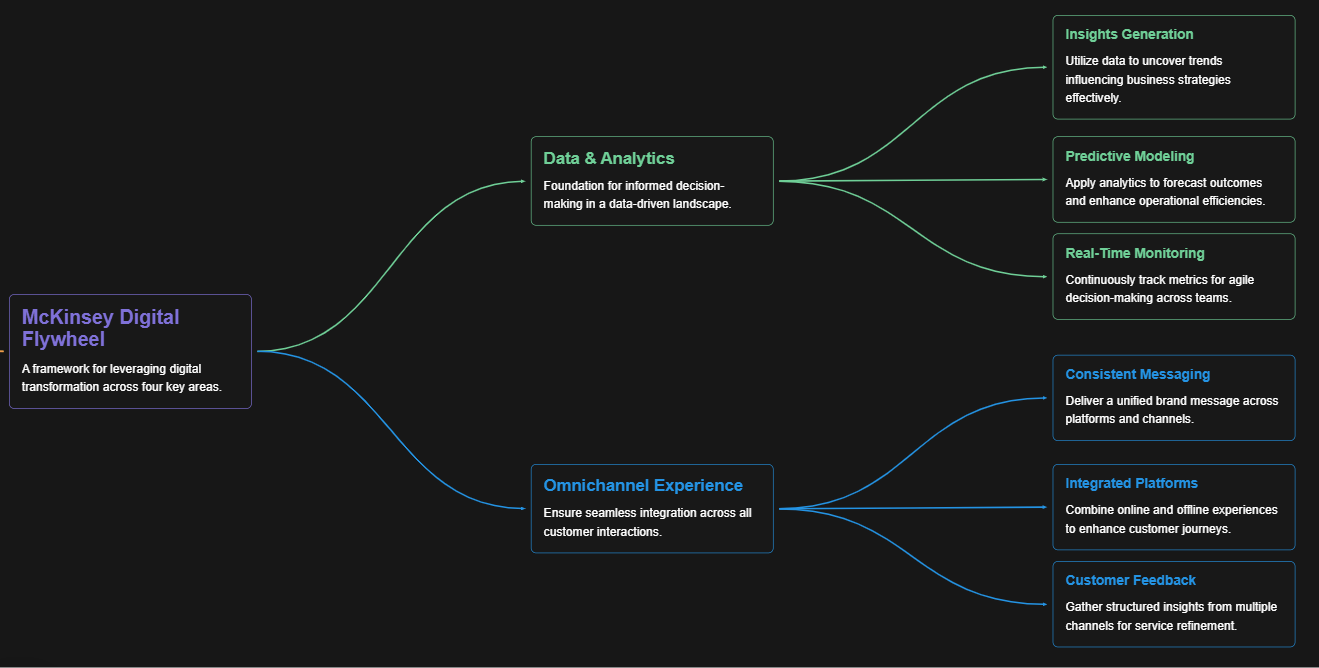
McKinsey Digital Flywheel
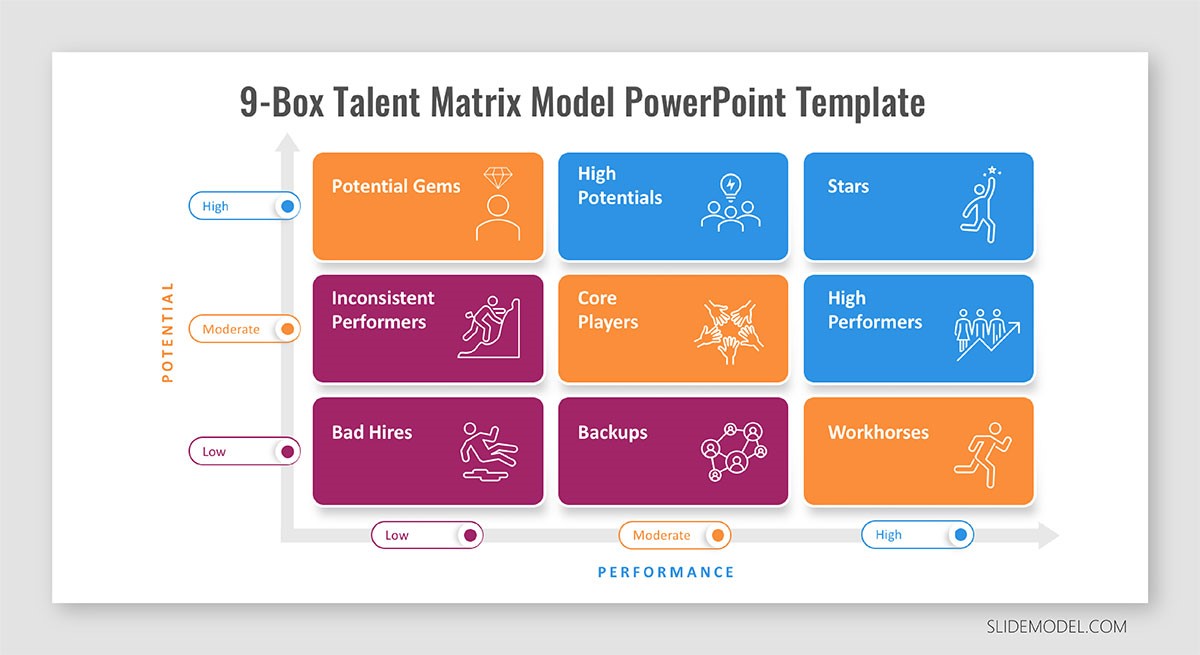
McKinsey 9-Box Talent Matrix
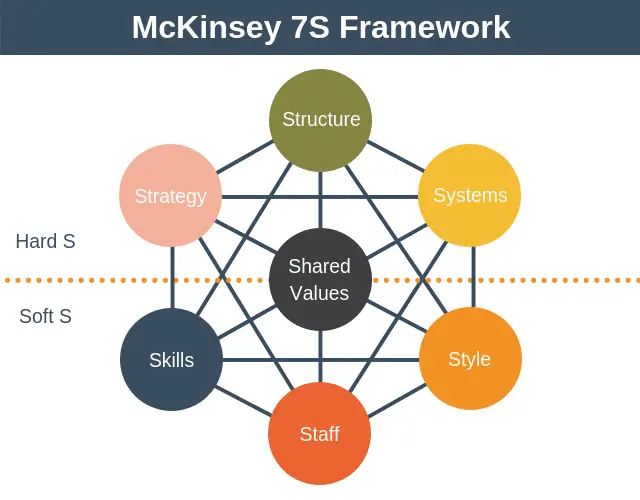
McKinsey 7S Framework
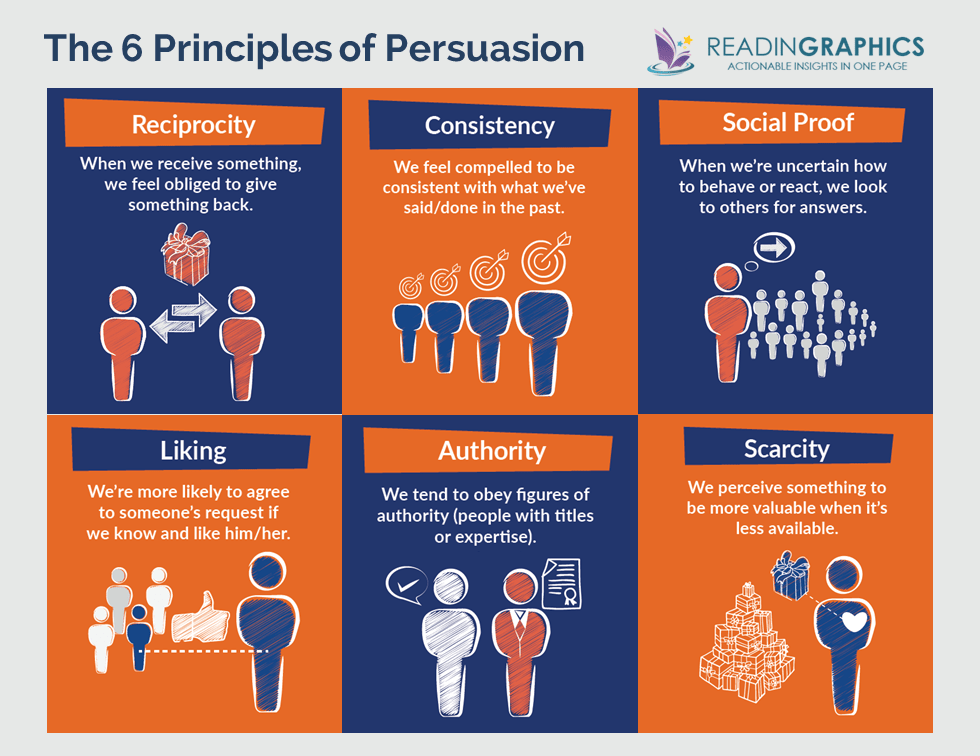
The Psychology of Persuasion in Marketing
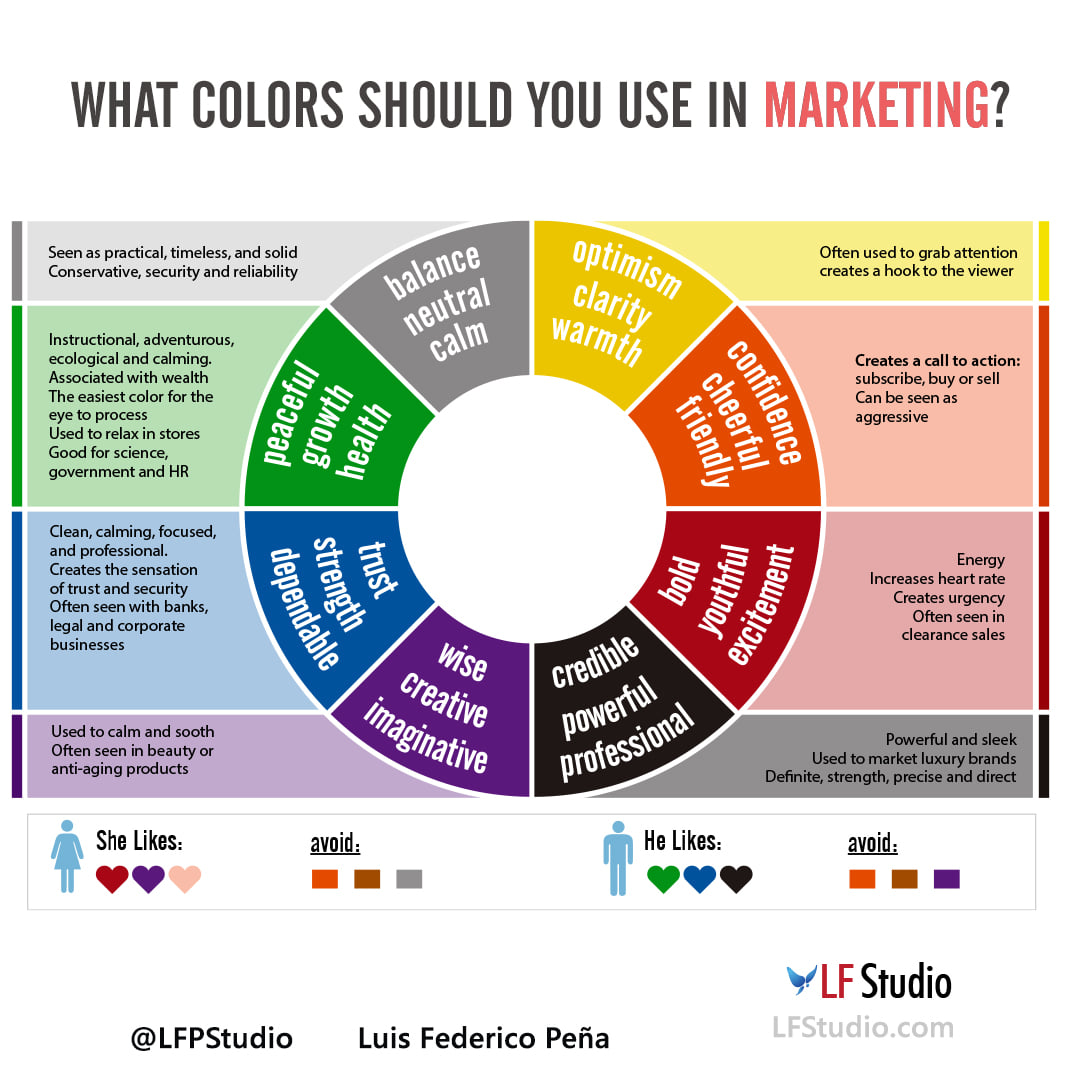
The Influence of Colors on Branding and Marketing Psychology
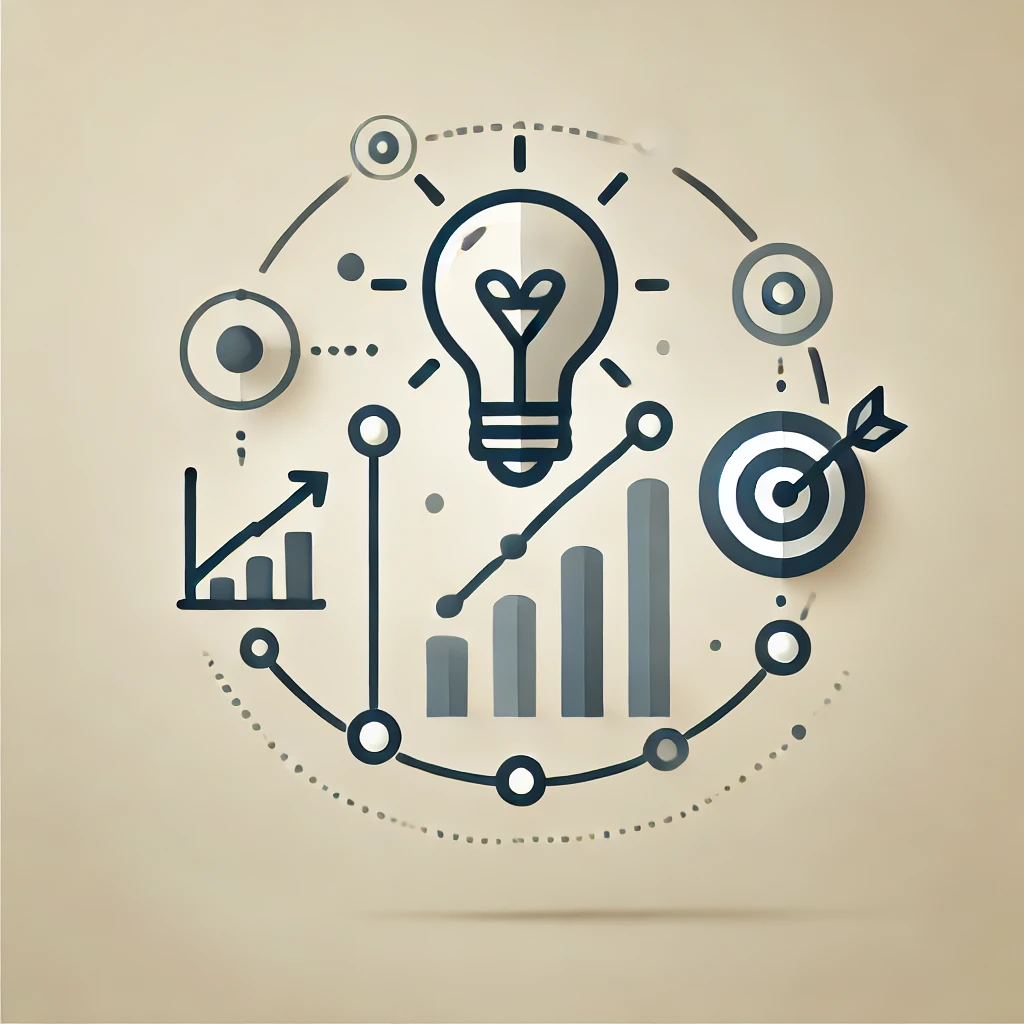
What is Marketing?
Recent Blogs
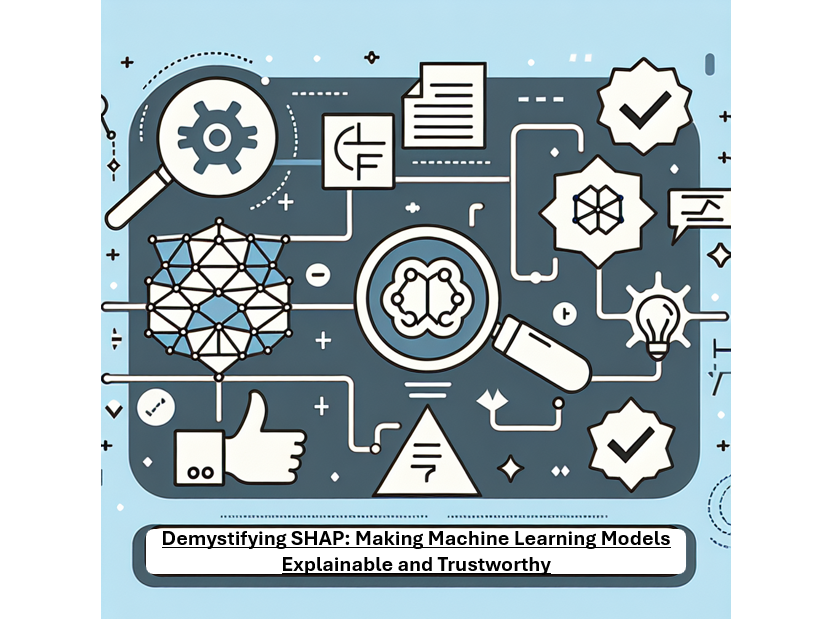
Demystifying SHAP: Making Machine Learning Models Explainable and Trustworthy
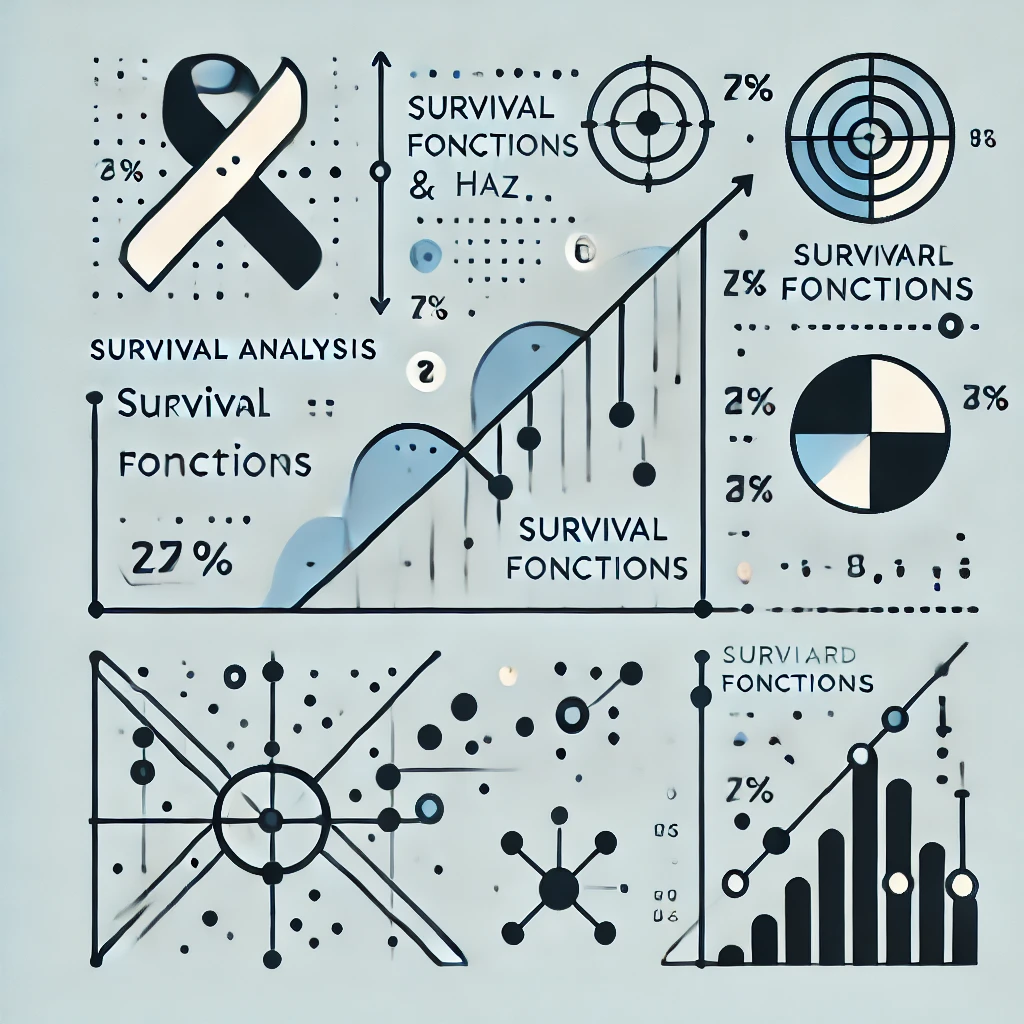
Survival Analysis & Hazard Functions: Concepts & Python Implementation
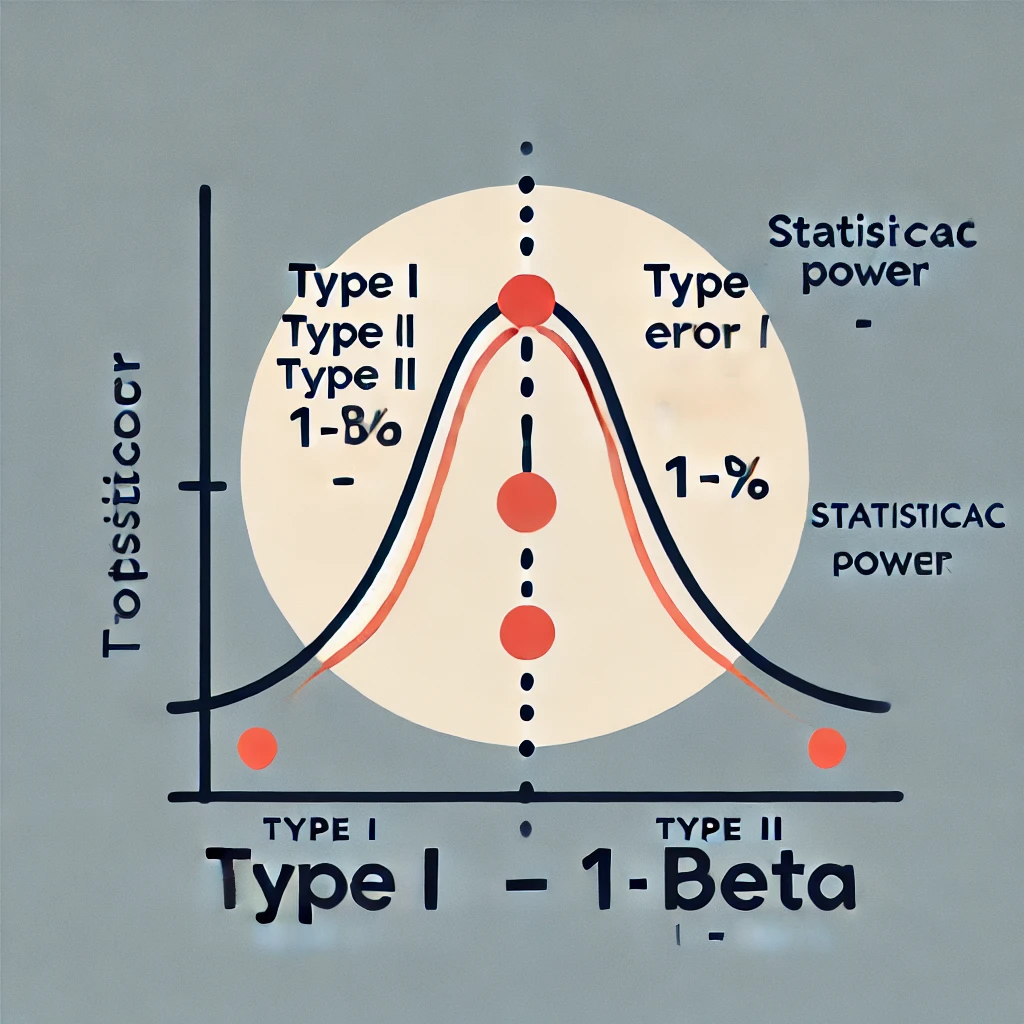
Power of a Statistical Test: Definition, Importance & Python Implementation
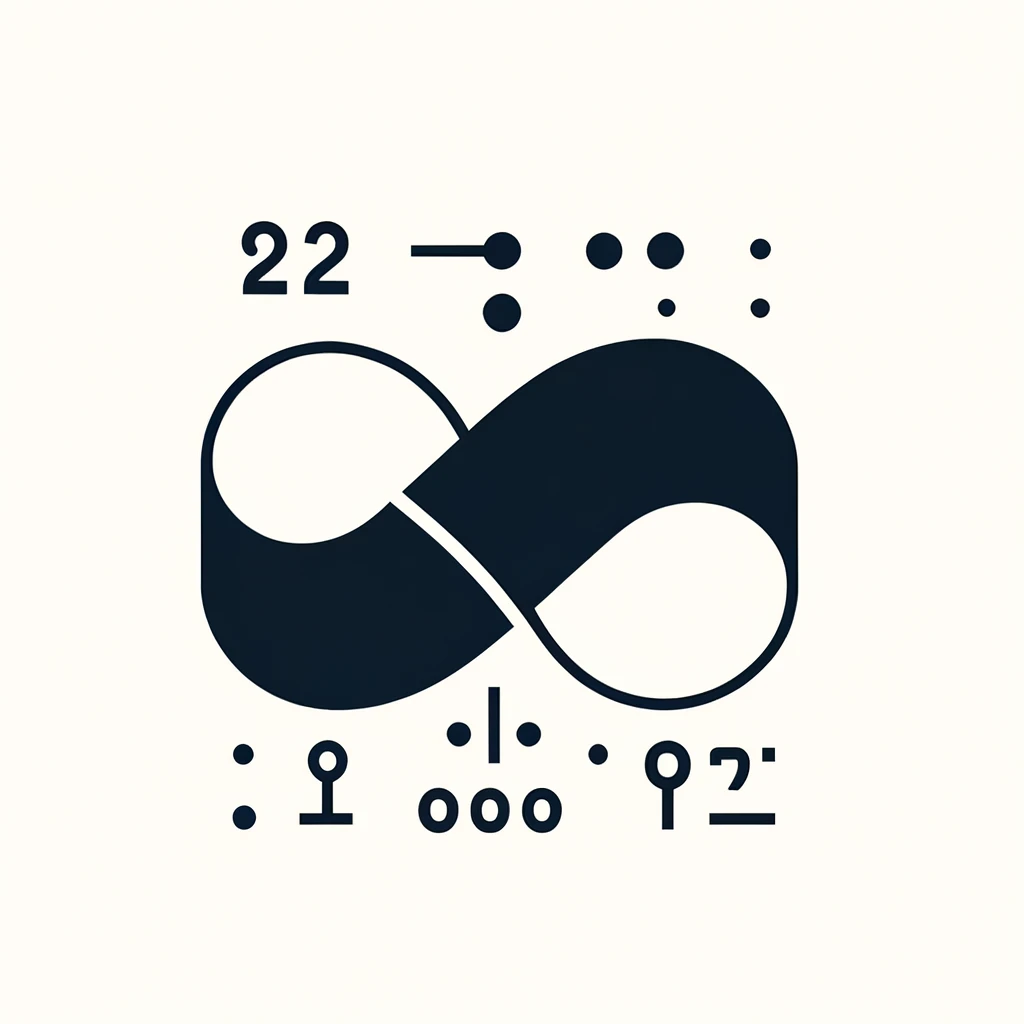
Logistic Regression & Odds Ratio: Concepts, Formula & Applications
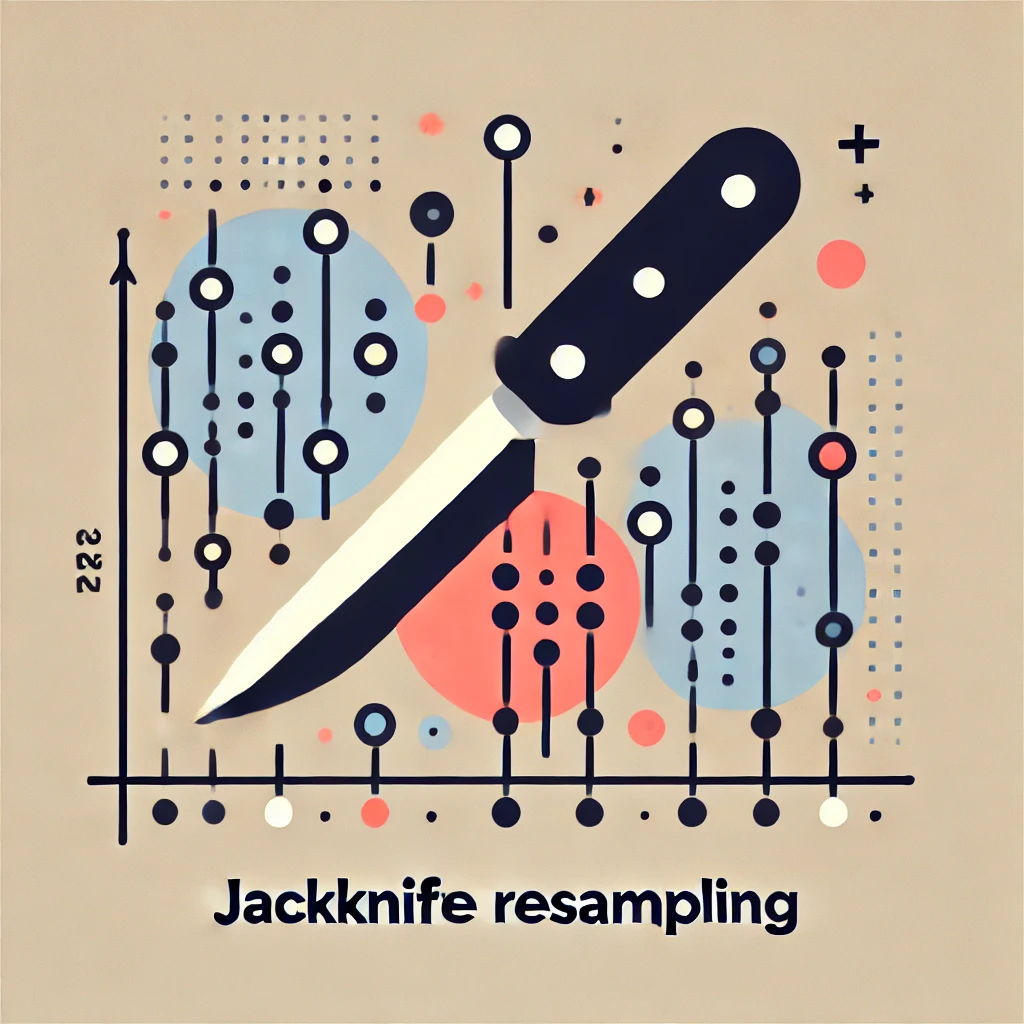
Jackknife Resampling: Concept, Steps & Applications
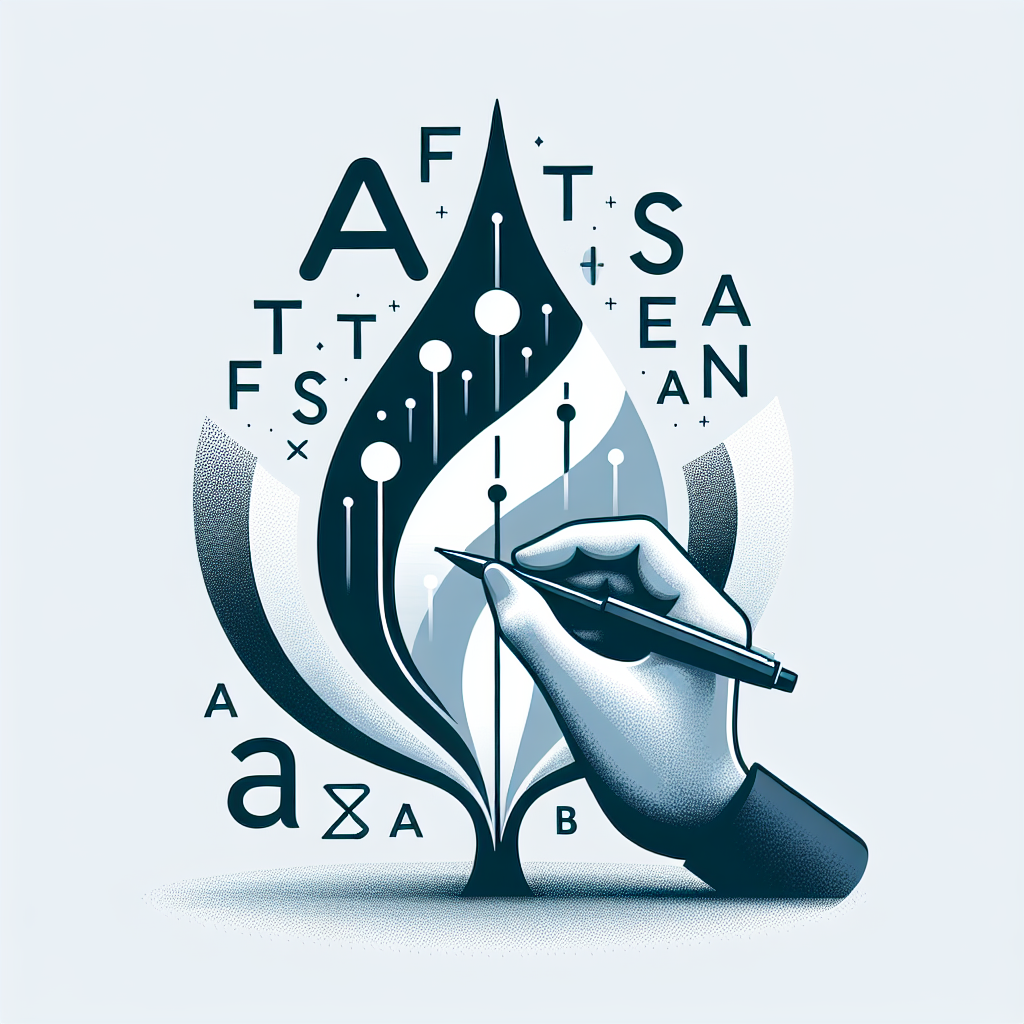