Predictive Lead Scoring: How AI Helps Sales and Marketing Teams
Introduction: The Evolution from Intuition to Intelligence
The perennial challenge of identifying which prospects merit sales attention has transformed from an art based on intuition to a science driven by artificial intelligence. Predictive lead scoring—the use of machine learning algorithms to evaluate and rank prospects based on their likelihood to convert—has emerged as a critical competitive advantage in an era of information abundance and attention scarcity. When Salesforce reported that high-performing sales teams are 2.8 times more likely to use AI in their sales processes, or when Adobe documented a 10% revenue increase after implementing predictive scoring, these weren't merely incremental improvements but paradigm shifts in go-to-market efficiency. According to research from Forrester, organizations implementing predictive lead scoring achieve a 30% reduction in cost per lead and a 25% increase in deal size. Harvard Business Review analysis indicates that companies using advanced AI for prospect qualification demonstrate 50% higher win rates than those relying on traditional methods. This evolution reflects broader transformations in B2B purchasing: increasingly complex buying committees, self-directed digital research journeys, and heightened expectations for personalized engagement. This article examines how predictive lead scoring is transforming sales and marketing alignment, the machine learning principles driving its effectiveness, and how technological advances are creating unprecedented opportunities for precision in prospect prioritization and personalization.
The Evolution of Lead Qualification: From Rules-Based to Algorithmic Intelligence
Lead scoring methodologies have undergone dramatic transformation:
Historical Trajectory and Methodological Shifts
- Traditional approaches (1990s-2000s) relied on simplistic point-based systems assigning fixed values to demographic and behavioral attributes.
- Professor V. Kumar's research identified the limitations of rules-based systems in capturing complex, non-linear relationships between variables.
- The inflection point came with the integration of advanced machine learning capabilities into mainstream CRM and marketing automation platforms.
The Taxonomy of Modern Predictive Scoring Approaches
- Behavioral analysis: Models evaluating engagement patterns and digital body language.
- Firmographic modeling: Algorithms identifying ideal customer profile fit beyond simple demographics.
- Technographic scoring: Systems evaluating technology stack compatibility and technological maturity.
- Intent analysis: AI systems detecting purchase intent signals from external data sources.
- Multi-touch attribution: Models connecting marketing touchpoints to conversion likelihood.
Technological Foundations: The Algorithms Behind Accurate Predictions
The effectiveness of predictive lead scoring is rooted in several machine learning approaches:
Supervised Learning and Conversion Pattern Recognition
- Algorithms trained on historical conversion data identify patterns invisible to human analysis.
- Research from MIT's Sloan School demonstrates how regression models outperform human intuition in identifying non-obvious conversion predictors.
- Example: Marketo's AI-driven scoring system identified that webinar attendance duration predicted conversion more accurately than download activity, contrary to previous assumptions.
Natural Language Processing and Intent Detection
- NLP algorithms analyze communication patterns and linguistic markers indicating purchase readiness.
- Stanford research shows that semantic analysis of prospect communications improves prediction accuracy by 37% compared to behavioral data alone.
- Example: HubSpot's integration of conversation intelligence analyzing email exchanges and meeting transcripts to identify buying signals within natural language.
Strategic Applications: Beyond Basic Lead Prioritization
Predictive scoring serves diverse strategic objectives beyond simple triage:
Ideal Customer Profile Refinement
- AI continuously refines target profiles based on conversion outcomes rather than assumptions.
- Example: Salesforce Einstein's discovery that company growth rate predicted conversion better than company size for SaaS products, fundamentally reshaping targeting strategies.
Dynamic Resource Allocation
- Predictive models optimizing sales coverage models and account-based marketing investments.
- Example: Microsoft's implementation of tiered engagement models automatically adjusting resource allocation based on algorithmic opportunity assessment.
Content and Channel Optimization
- Scoring systems identifying which content formats and channels influence high-value conversions.
- Example: Oracle's content scoring system revealing that technical white papers viewed by multiple stakeholders indicated 3.2x higher conversion probability than executive summaries.
Implementation Challenges: Navigating Complexity
Developing effective predictive scoring presents significant challenges:
Data Quality and Integration Requirements
- Predictive models require clean, integrated data from diverse systems.
- Professor Thomas Davenport's research emphasizes how fragmented data environments undermine predictive accuracy.
Explainability vs. Accuracy Tension
- Sophisticated "black box" models often outperform explainable ones, creating adoption challenges.
- Example: ZoomInfo's development of transparent scoring modifiers enabling sales teams to understand and trust algorithmic recommendations.
Algorithmic Bias Mitigation
- Predictive systems risk perpetuating historical biases in sales coverage.
- Industry research indicates systems trained on historical data may undervalue emerging market segments or non-traditional buyer profiles.
Future Trajectories: The Evolution of AI-Driven Prospect Intelligence
The predictive scoring landscape continues to evolve:
Multi-Model Ensemble Approaches
- Next-generation systems combining multiple specialized algorithms for higher accuracy.
- Example: 6sense's combination of intent, engagement, and firmographic models into unified scoring frameworks.
Prescriptive Guidance Beyond Scoring
- Evolution from score generation to specific action recommendations.
- Example: Drift's conversational intelligence not only scoring leads but recommending optimal engagement strategies based on detected buying patterns.
Third-Party Data Integration and Privacy Balancing
- Scoring systems incorporating external intent and company signals while navigating evolving privacy landscapes.
- Example: Bombora's integration of anonymous intent data from thousands of B2B websites to identify companies researching specific topics.
Conclusion: The Strategic Imperative of Algorithmic Intelligence
Predictive lead scoring represents more than an incremental improvement in sales efficiency—it constitutes a fundamental shift in how organizations identify, prioritize, and engage potential customers. By leveraging machine learning to uncover non-obvious patterns in prospect behavior and attributes, companies can achieve dramatic improvements in resource allocation, conversion rates, and revenue growth. The most successful implementations will be those that balance algorithmic sophistication with practical usability, maintain human judgment in the process, and continuously refine models based on outcome data. As B2B purchasing journeys become increasingly complex and digital-first, organizations that develop sophisticated predictive capabilities will establish significant competitive advantages in connecting with high-potential prospects at moments of maximum receptivity.
Call to Action
For sales and marketing leaders seeking to develop effective predictive scoring capabilities:
- Conduct comprehensive data quality audits across marketing automation, CRM, and communication systems.
- Implement phased approaches prioritizing model transparency over maximum complexity in early stages.
- Develop cross-functional teams that combine data science expertise with frontline sales experience.
- Establish clear feedback loops capturing sales outcomes to continuously refine predictive models.
Featured Blogs
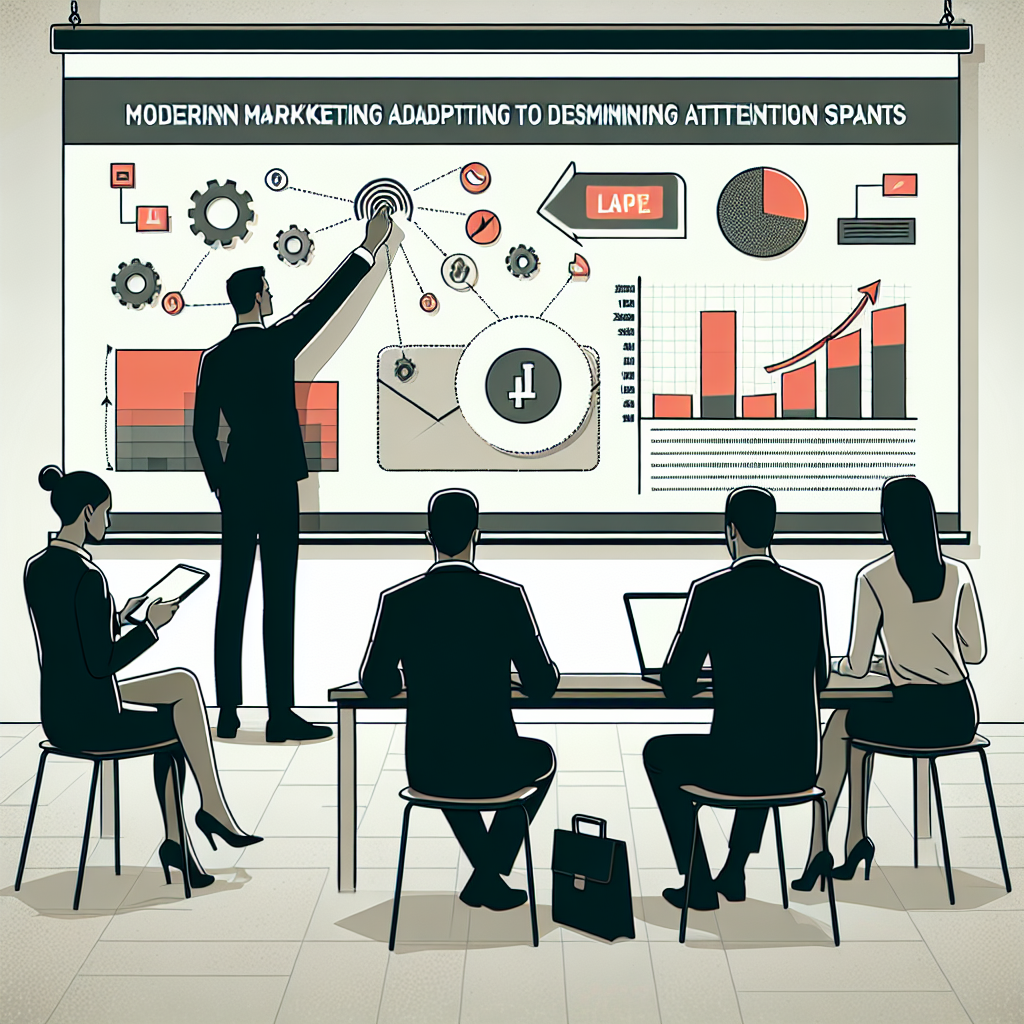
How the Attention Recession Is Changing Marketing
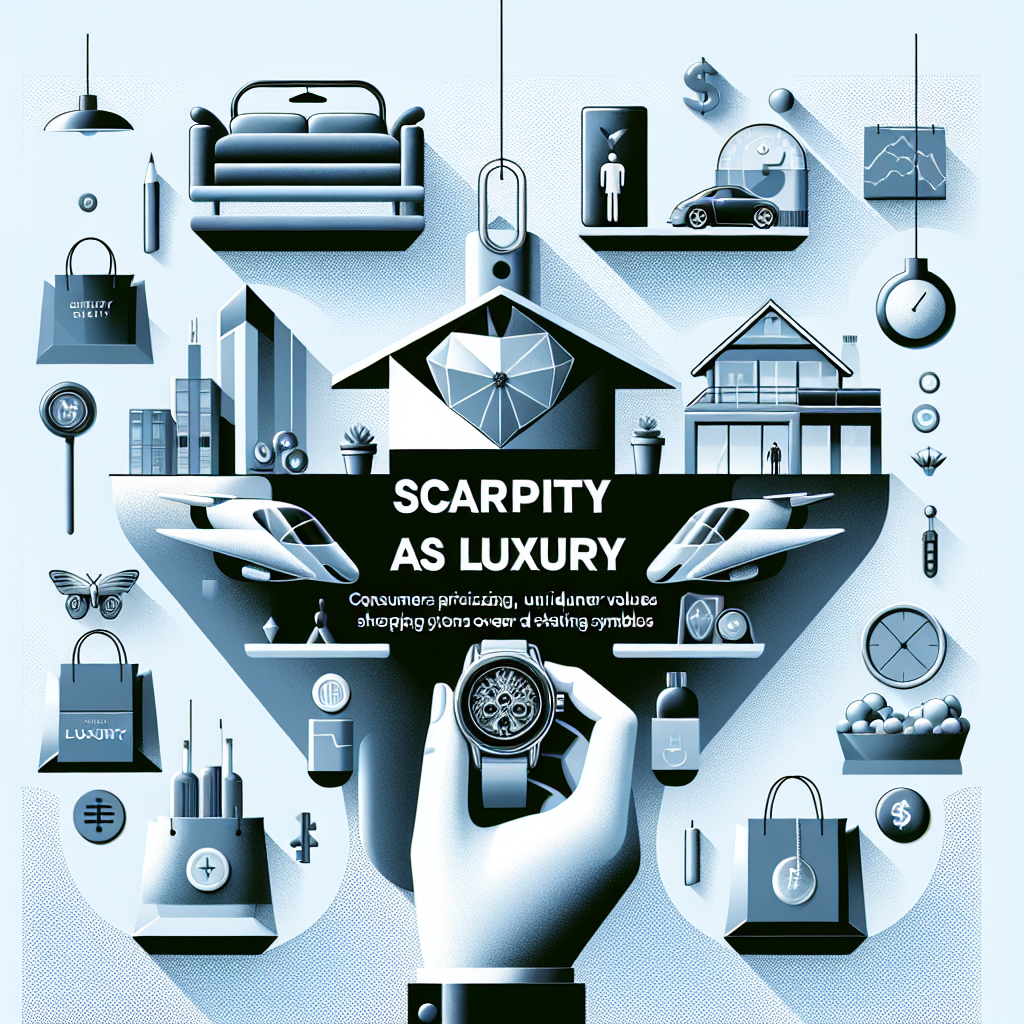
The New Luxury Why Consumers Now Value Scarcity Over Status
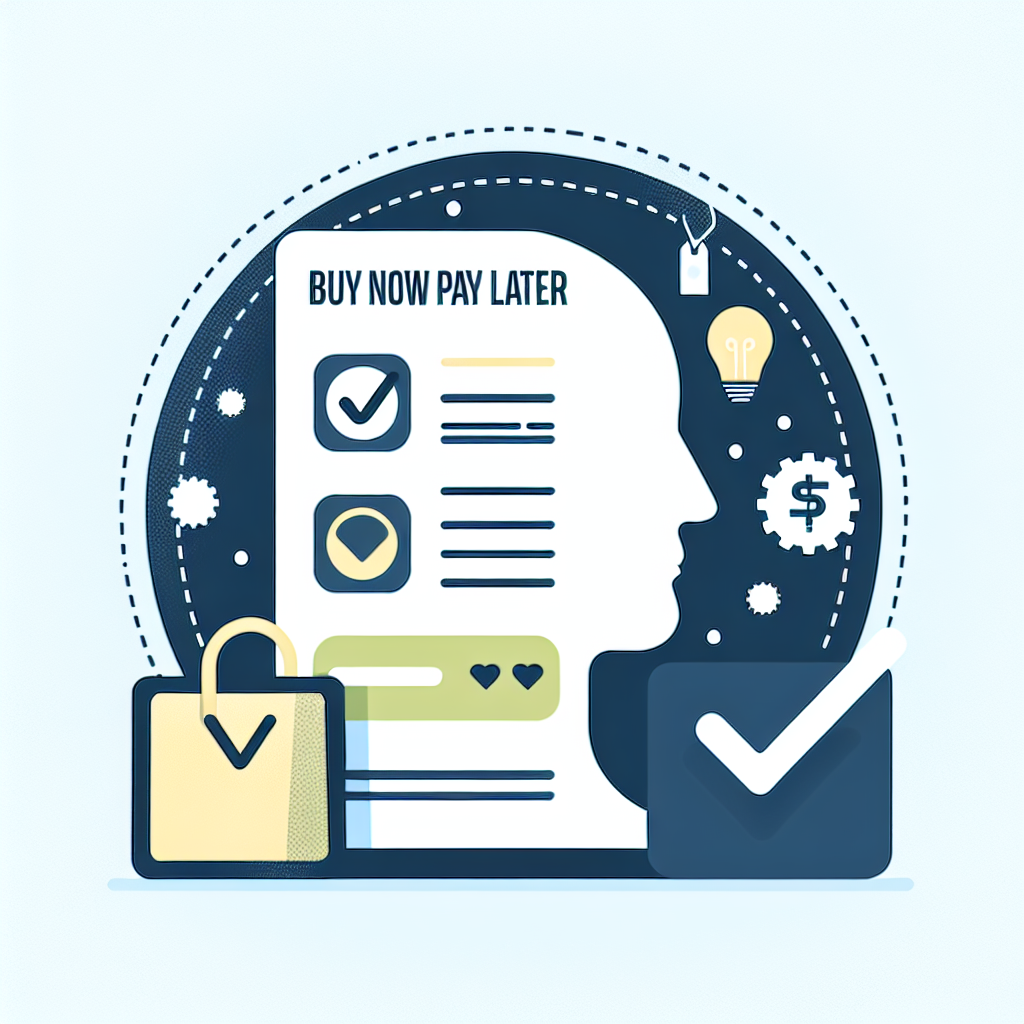
The Psychology Behind Buy Now Pay later
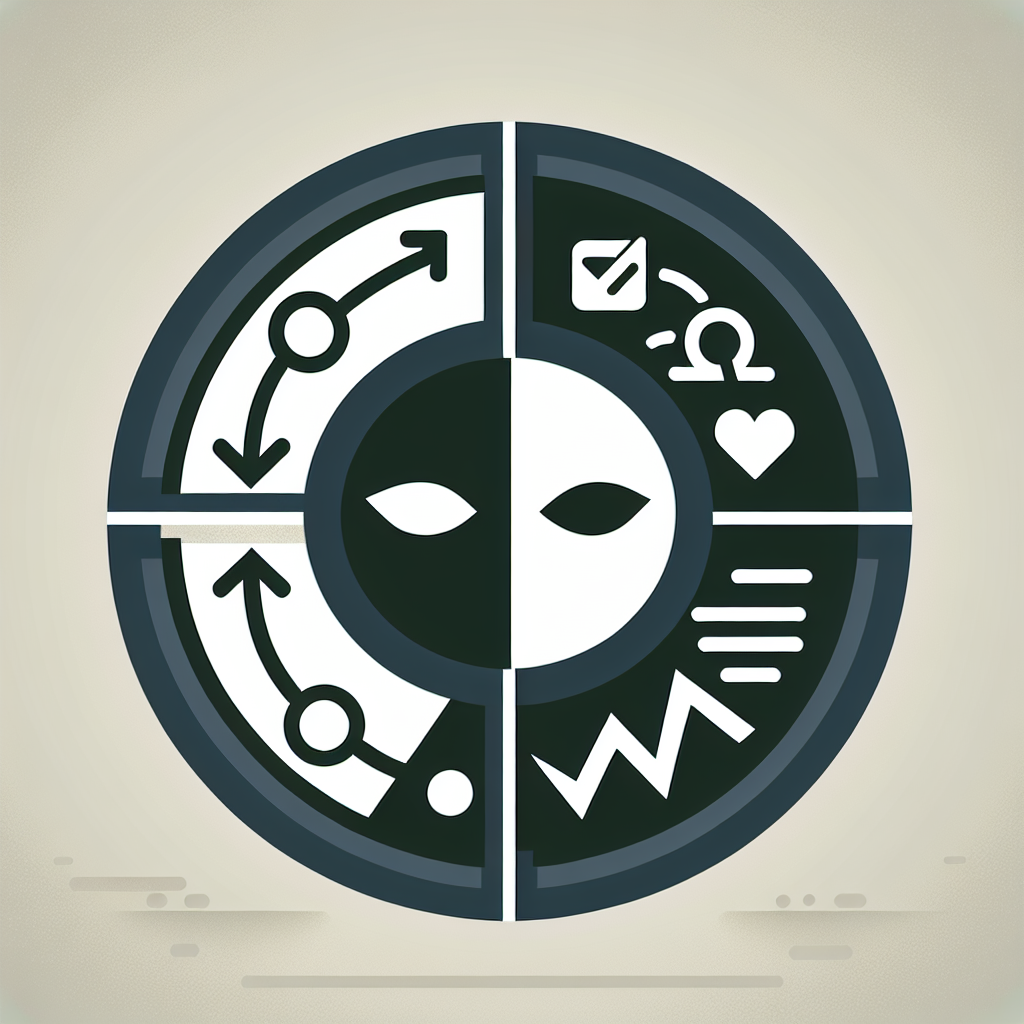
The Role of Dark Patterns in Digital Marketing and Ethical Concerns
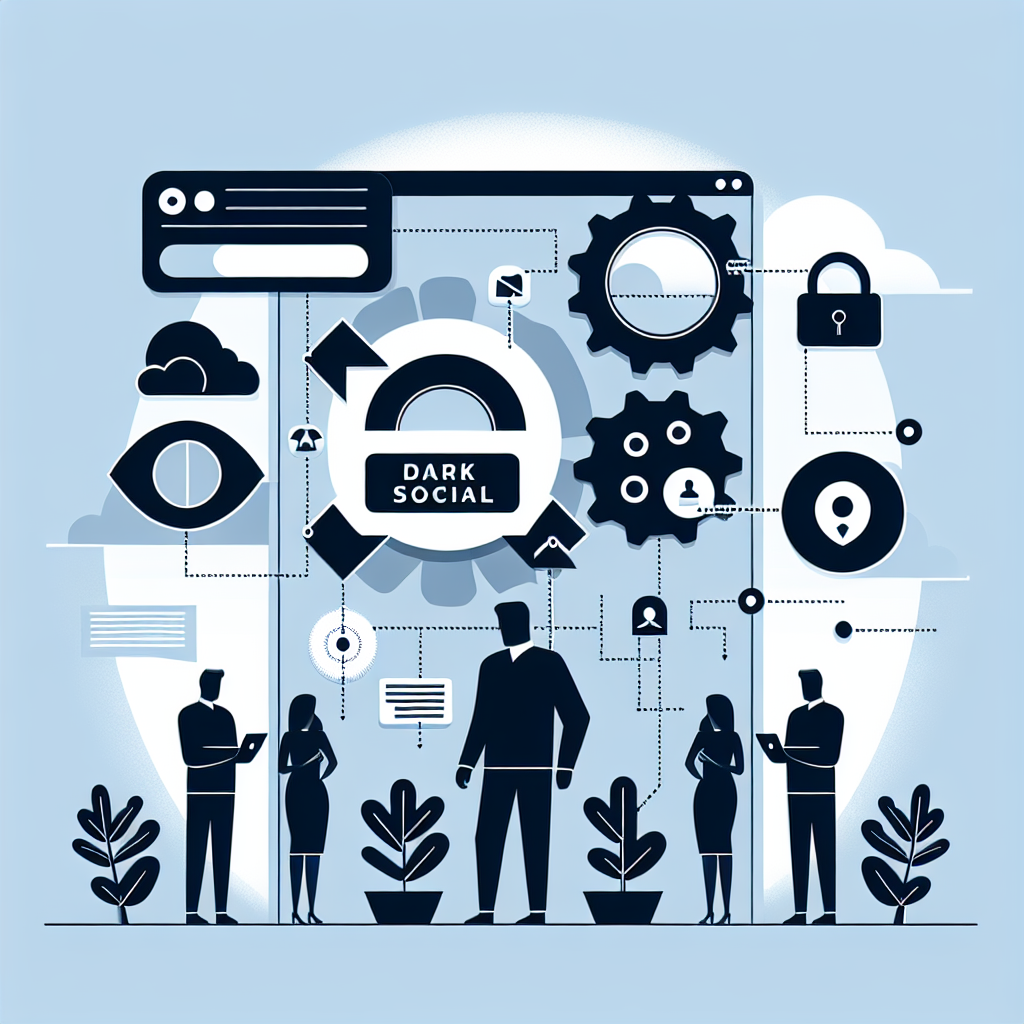
The Rise of Dark Social and Its Impact on Marketing Measurement
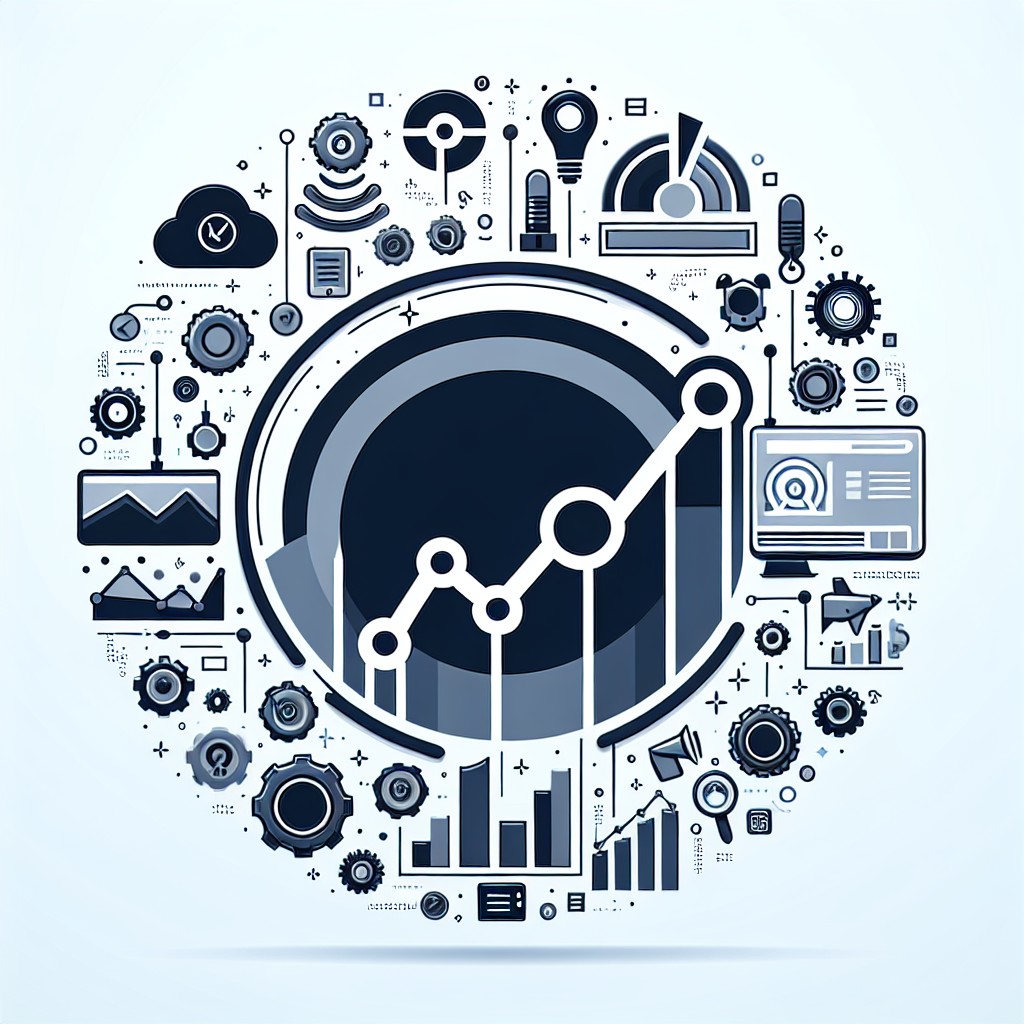
The Future of Retail Media Networks and What Marketers Should Know
Recent Blogs
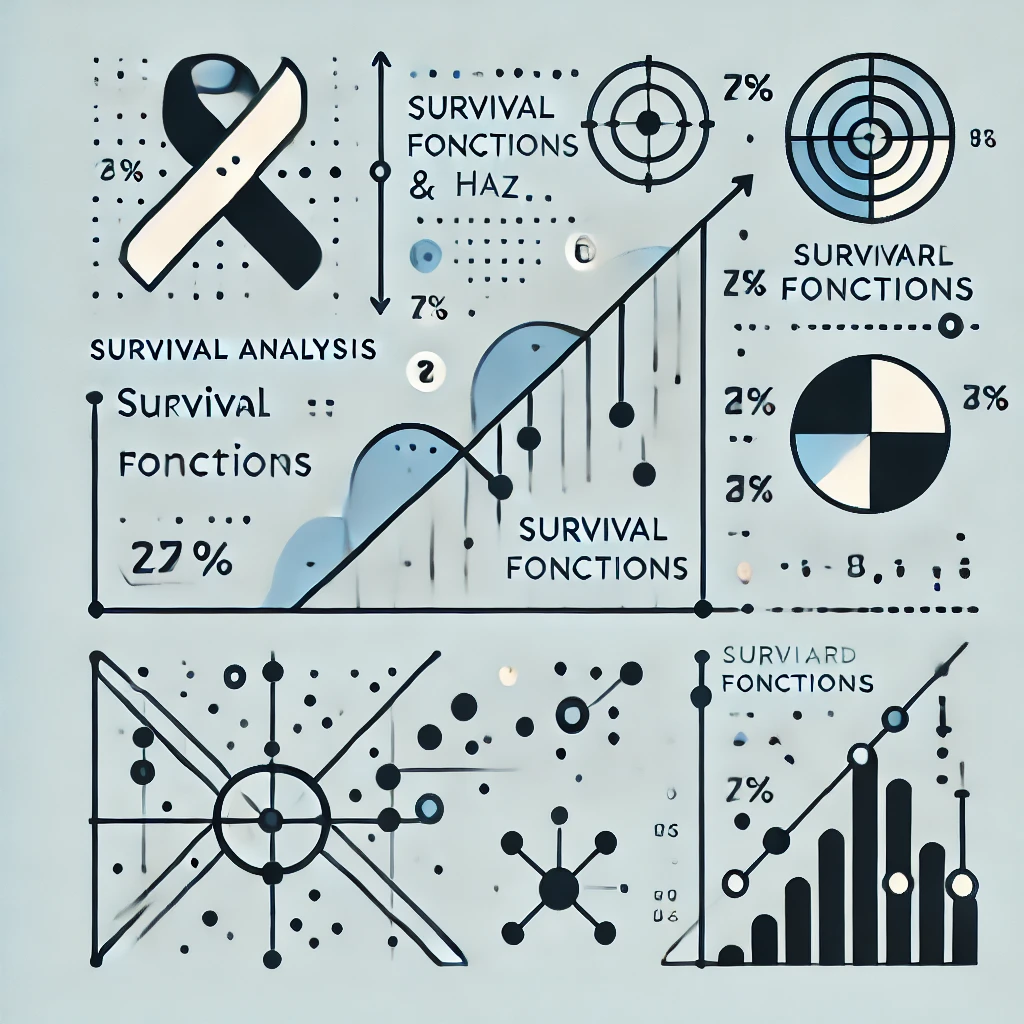
Survival Analysis & Hazard Functions: Concepts & Python Implementation
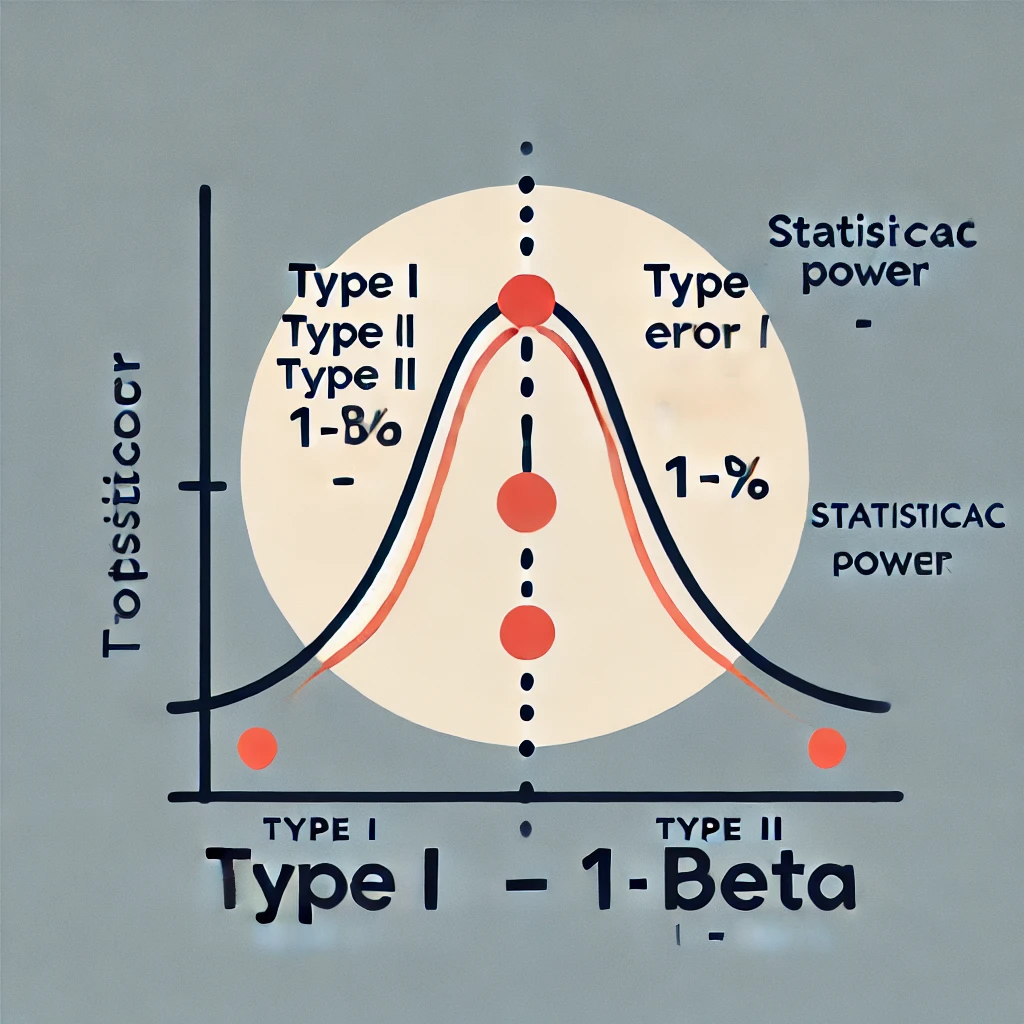
Power of a Statistical Test: Definition, Importance & Python Implementation
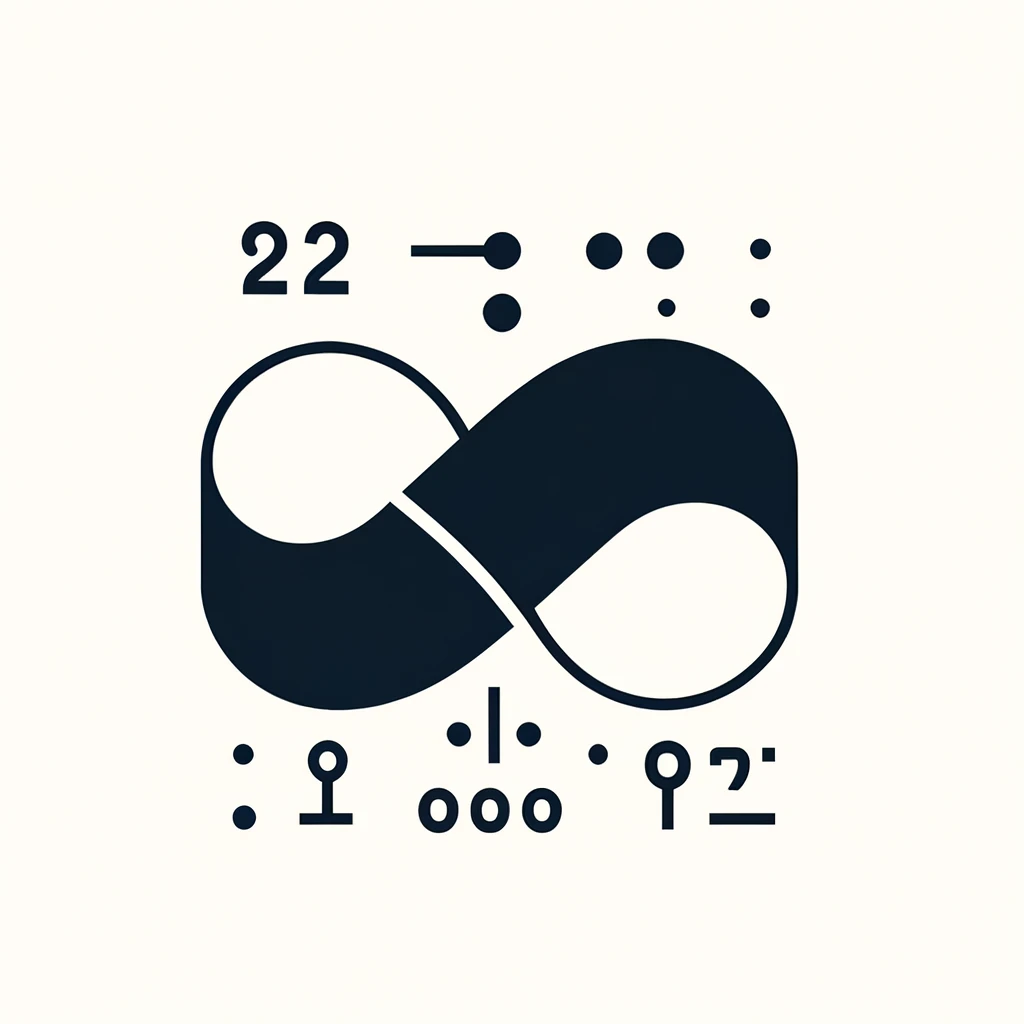
Logistic Regression & Odds Ratio: Concepts, Formula & Applications
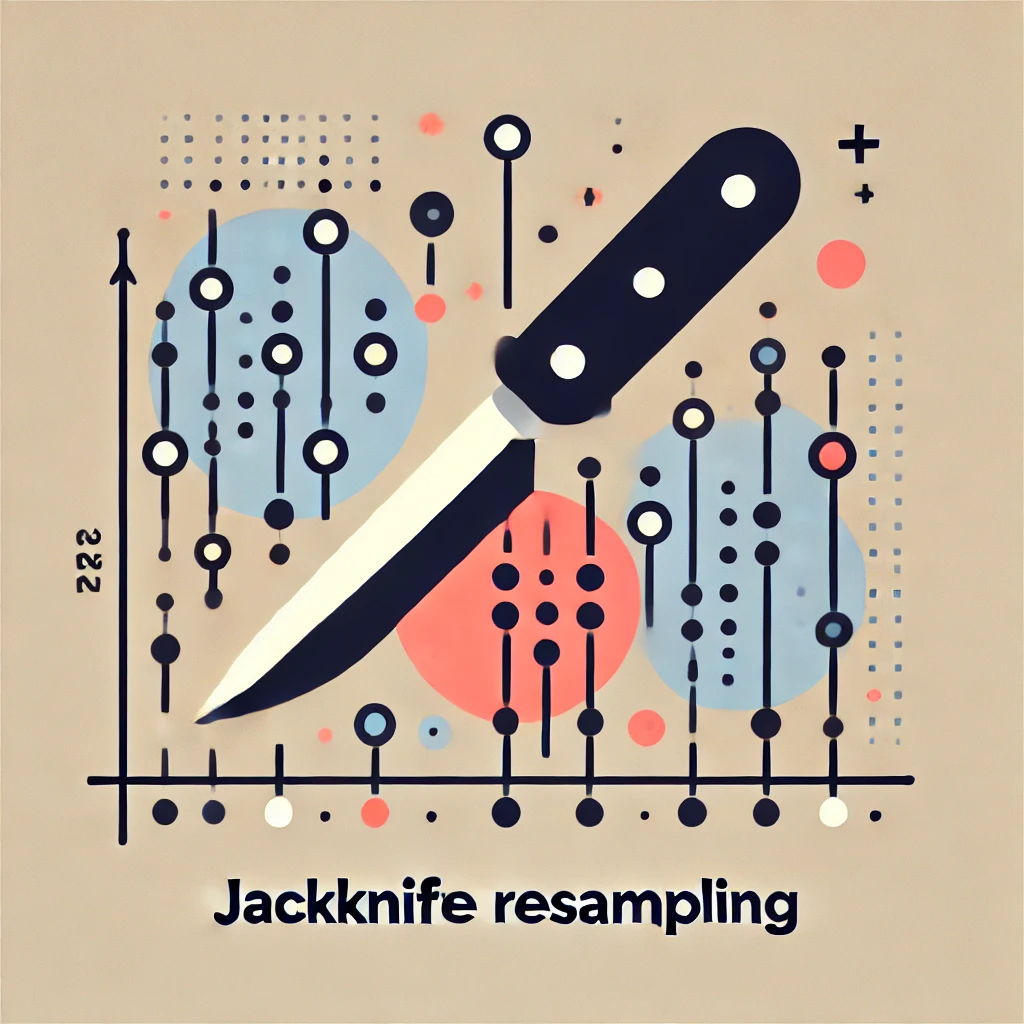
Jackknife Resampling: Concept, Steps & Applications
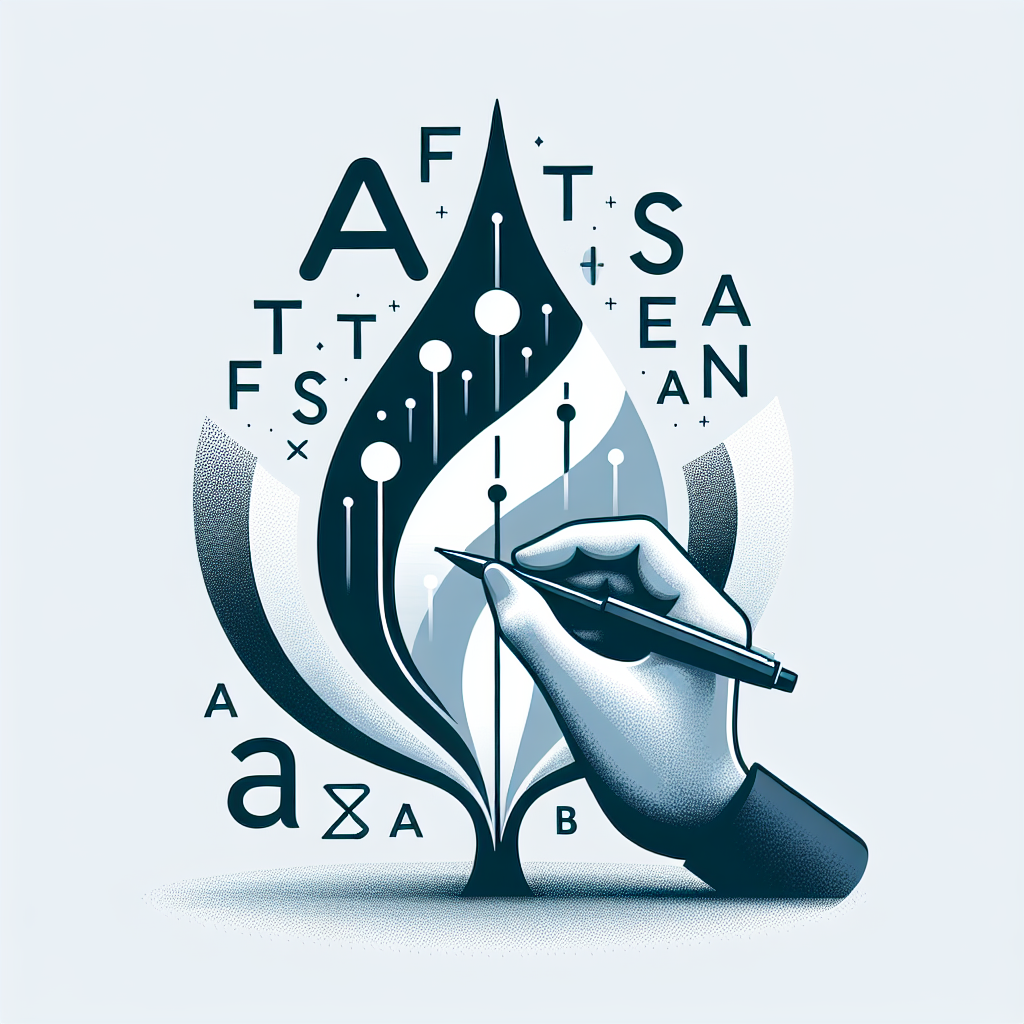
F test and Anova
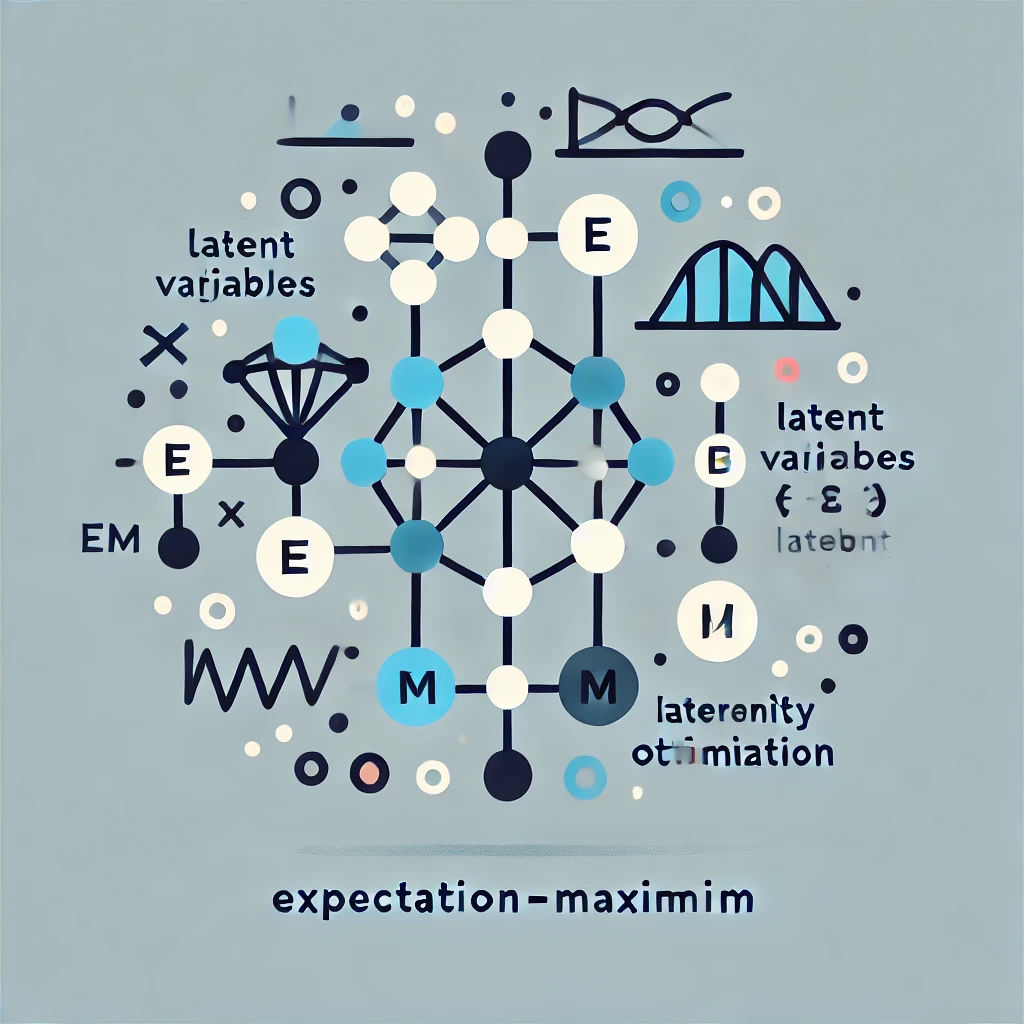