Multicollinearity in Regression
Multicollinearity is a statistical phenomenon wherein multiple predictor variables in a multiple regression model are correlated. Unlike simple correlation, which involves two variables, multicollinearity concerns multiple variables and their interrelationships. Its presence complicates the estimation of regression coefficients, as high correlations between predictors cloud the unique contribution of each variable, potentially skewing the model's interpretative precision.
Table of Contents
- Introduction to Multicollinearity
- Causes of Multicollinearity
- Identifying Multicollinearity
- Consequences of Multicollinearity
- Addressing Multicollinearity
- Significance of Multicollinearity in Regression Analysis
- Applications of Multicollinearity in Regression
- Implementation of Multicollinearity in Python
- Conclusion
Introduction to Multicollinearity
Multicollinearity is a statistical phenomenon wherein multiple predictor variables in a multiple regression model are correlated. Unlike simple correlation, which involves two variables, multicollinearity concerns multiple variables and their interrelationships. Its presence complicates the estimation of regression coefficients, as high correlations between predictors cloud the unique contribution of each variable, potentially skewing the model's interpretative precision.
Causes of Multicollinearity
Several factors can lead to Multicollinearity:
- Redundant Variables: Inclusion of unnecessary variables that correlate highly with each other or with existing predictors.
- Data Collection Methods: Using hierarchical data or repeated measures that inherently generate correlated variables.
- Polynomial Terms: Transformations or powers of variables (such as quadratics) that naturally correlate with the original variables.
- Over specified Models: Including too many variables relative to the number of observations, often due to overfitting.
Identifying Multicollinearity
Detecting the Multicollinearity is integral to understanding its impact on a regression model. Key methods include:
- Correlation Matrix: Examining pairwise correlations can provide preliminary signals of multicollinearity.
- Variance Inflation Factor (VIF): A statistical measure that quantifies how much the variance of an estimated regression coefficient increases due to multicollinearity. Typically, a VIF value above 10 indicates serious multicollinearity.
- Tolerance: The reciprocal of VIF, offering another perspective on collinearity.
- Eigenvalue Analysis: Small eigenvalues close to zero can signal multicollinearity issues.
Consequences of Multicollinearity
Multicollinearity can lead to a variety of concerns:
- Inflated Standard Errors: With correlated predictors, the model struggles to attribute variations in the dependent variable to each specific predictor, thus increasing uncertainty around coefficient estimates.
- Unstable Coefficients: Small changes in data can cause large fluctuations in the estimated coefficients.
- Reduced Model Interpretability: Difficulty in understanding which predictor variables are truly influencing the dependent variable.
- Overestimation of Fit: Can result in high R-squared values that are misleading in terms of actual predictive power.
Addressing Multicollinearity
Approaches to mitigate multicollinearity include:
- Removing Variables: Eliminating one or more correlated predictors can immediately reduce multicollinearity.
- Combining Variables: Aggregating correlated variables into a single composite index or mean score.
- Centering: Subtracting the mean from the variables to reduce correlation without changing the model's basic structure.
- Principal Component Analysis (PCA): Dimensionality reduction technique that transforms correlated variables into a set of linearly uncorrelated variables (principal components).
Significance of Multicollinearity in Regression Analysis
Multicollinearity, a common concern in regression analysis, refers to the situation where independent variables in a model are highly correlated. Understanding its significance is critical for several reasons:
1. Coefficient Interpretation
In the context of multicollinearity, the main difficulty lies in evaluating the individual influence of each predictor variable on the dependent variable. When predictors are highly correlated, it becomes challenging to determine the unique effect that each has on the outcome. This is because:
- Inflated Standard Errors: The standard errors of the coefficients increase, making them less reliable. This inflation is because the model has difficulty distinguishing the unique contribution of each correlated predictor.
- Unstable Estimates: Regression coefficients can vary significantly with slight changes in the model or data, leading to erratic interpretability.
2. Statistical Significance Testing
Multicollinearity can have a profound impact on statistical inference:
- Reduced Power: With larger standard errors, hypothesis tests for individual coefficients (e.g., t-tests) have reduced power, making it harder to determine whether a predictor is significantly different from zero.
- Non-significance: Variables might appear non-significant simply because their effects are obscured by the correlation with other variables, even if they are relevant.
3. Model Selection and Prediction
Multicollinearity affects model-building strategies and the predictive performance of models:
- Variable Selection: It complicates the selection of relevant variables for the model. The presence of multicollinearity might lead to the inclusion of redundant variables, potentially overfitting the model.
- Prediction Accuracy: While multicollinearity does not affect the overall predictive power of the model when considering all predictors together, it does hinder the interpretability of individual predictor effects, which can be misleading in some applications.
4. Diagnostic and Corrective Actions
Understanding and diagnosing multicollinearity is essential to refine the model and improve its utility:
- Variance Inflation Factor (VIF): A key diagnostic tool, VIF helps identify which variables are excessively correlated with others, guiding necessary model adjustments.
- Techniques to Address: Recognizing its presence enables analysts to take corrective actions such as combining variables, removing redundant predictors, or applying dimensionality reduction techniques like Principal Component Analysis (PCA).
5. Impact on Decision-Making
In domains where regression models inform policy or business decisions, understanding multicollinearity is vital:
- Informed Decisions: Ensuring model reliability through proper handling of multicollinearity leads to more informed and accurate decision-making based on clear interpretations of variable impacts.
- Risk Mitigation: By addressing multicollinearity, analysts reduce the risk of drawing incorrect conclusions from regression models, which can have significant implications in fields like finance, healthcare, and economics.
Applications of Multicollinearity in Regression
Multicollinearity plays a crucial role in various fields where regression analysis is utilized. Here are some common applications and considerations:
1. Economics
- Macroeconomic Models: Economic indicators like GDP, inflation, and unemployment rates are often interrelated. Understanding multicollinearity helps in creating models that accurately capture these complex relationships.
- Policy Evaluation: When analysing the impact of policy changes, distinguishing the effect of different variables can guide more effective decision-making.
2. Finance
- Asset Pricing Models: Variables such as interest rates, market indices, and economic indicators are typically correlated. Multicollinearity handling ensures models reflecting stock prices or bond yields remain stable.
- Risk Assessment: Financial risk models often deal with correlated inputs, such as different types of market risks. Addressing multicollinearity improves risk evaluation strategies.
3. Marketing
- Consumer Behaviour Analysis: Understanding customer preferences requires modelling various influencing factors like demographics, purchasing history, and campaign exposure, which often exhibit multicollinearity.
- Sales Forecasting: Variables like pricing, advertising spend, and competitor actions are interlinked. Effective multicollinearity management can enhance forecasting accuracy.
4. Healthcare and Medicine
- Clinical Research: In studies exploring the relationship between different medical indicators or treatment effects, multicollinearity can obscure true associations, impacting research conclusions.
- Epidemiology: Models predicting health outcomes based on lifestyle, genetic, and environmental factors benefit from managing multicollinearity to clarify contributing influences.
5. Social Sciences
- Sociological Studies: Multiple predictors such as income, education, and social mobility are often correlated. Addressing multicollinearity helps in studying their distinct effects on societal trends.
- Psychological Research: Psychological assessments often involve correlated variables like stress, anxiety, and cognitive functions. Proper handling enhances clarity in interpreting psychological phenomena.
6. Environmental Science
- Climate Modelling: Weather and climate models involve numerous correlated variables, like temperature, humidity, and CO2 levels. Efficient multicollinearity management is key to accurate climate prediction.
- Environmental Impact Studies: Analysing the effects of human activities on ecosystems often requires models that can separate correlated environmental variables.
Multicollinearity Strategies in Applications:
- Dimensionality Reduction: Techniques like PCA aggregate correlated variables, providing a clearer insight into data patterns and reducing noise.
- Regularization Methods: Applying Lasso or Ridge regression helps mitigate multicollinearity effects by penalizing the size of coefficients.
- Model Refinement: By carefully selecting and testing variables, researchers and analysts can build models that reflect true relationships, enhancing their practical applicability.
Implementation of Multicollinearity in Python
import pandas as pd
import numpy as np
from statsmodels.stats.outliers_influence import variance_inflation_factor
from sklearn.preprocessing import StandardScaler
# Create a DataFrame with potential multicollinearity
data = pd.DataFrame({
'X1': np.random.rand(100),
'X2': np.random.rand(100) * 0.5 + np.random.rand(100) * 0.5,
'X3': np.random.rand(100) * 0.1 + np.random.rand(100) * 0.9
})
# Intentionally create high correlation
data['X4'] = data['X1'] + np.random.rand(100) * 0.1
# Standardize the dataset
scaler = StandardScaler()
data_scaled = scaler.fit_transform(data)
# Calculate VIF to detect multicollinearity
vif_data = pd.DataFrame()
vif_data["Variable"] = data.columns
vif_data["VIF"] = [variance_inflation_factor(data_scaled, i) for i in range(data_scaled.shape[1])]
print(vif_data)
Conclusion
Multicollinearity is a critical aspect of regression analysis, presenting unique challenges in model specification and interpretation. By effectively diagnosing and addressing multicollinearity, analysts can enhance the robustness of their models, ensuring clearer insights and more reliable conclusions. This, in turn, aids in effective decision-making and strategic planning across various disciplines and industries. Understanding the intricacies of multicollinearity empowers researchers to refine their models, paving the way for more meaningful and actionable results.
Featured Blogs
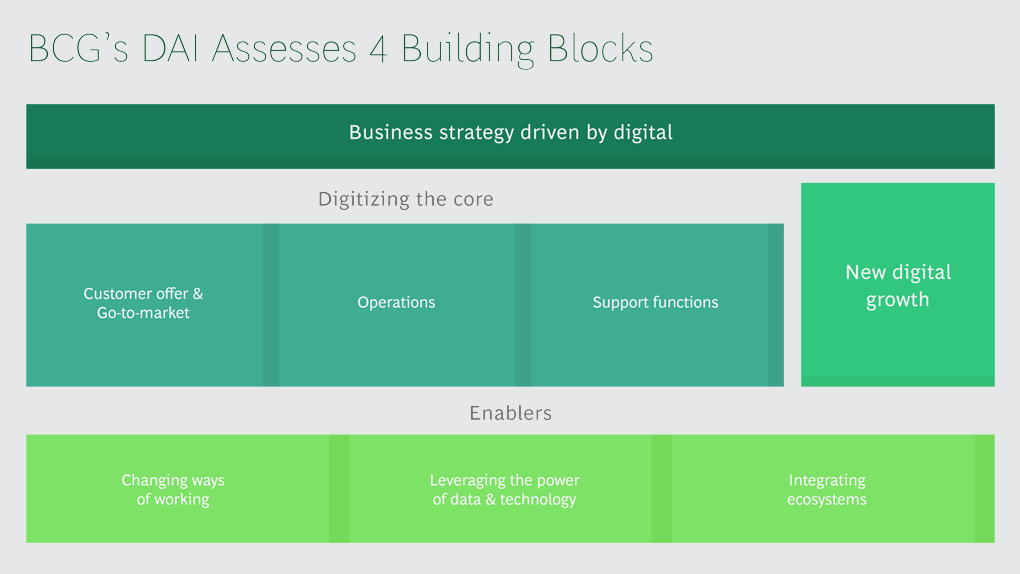
BCG Digital Acceleration Index
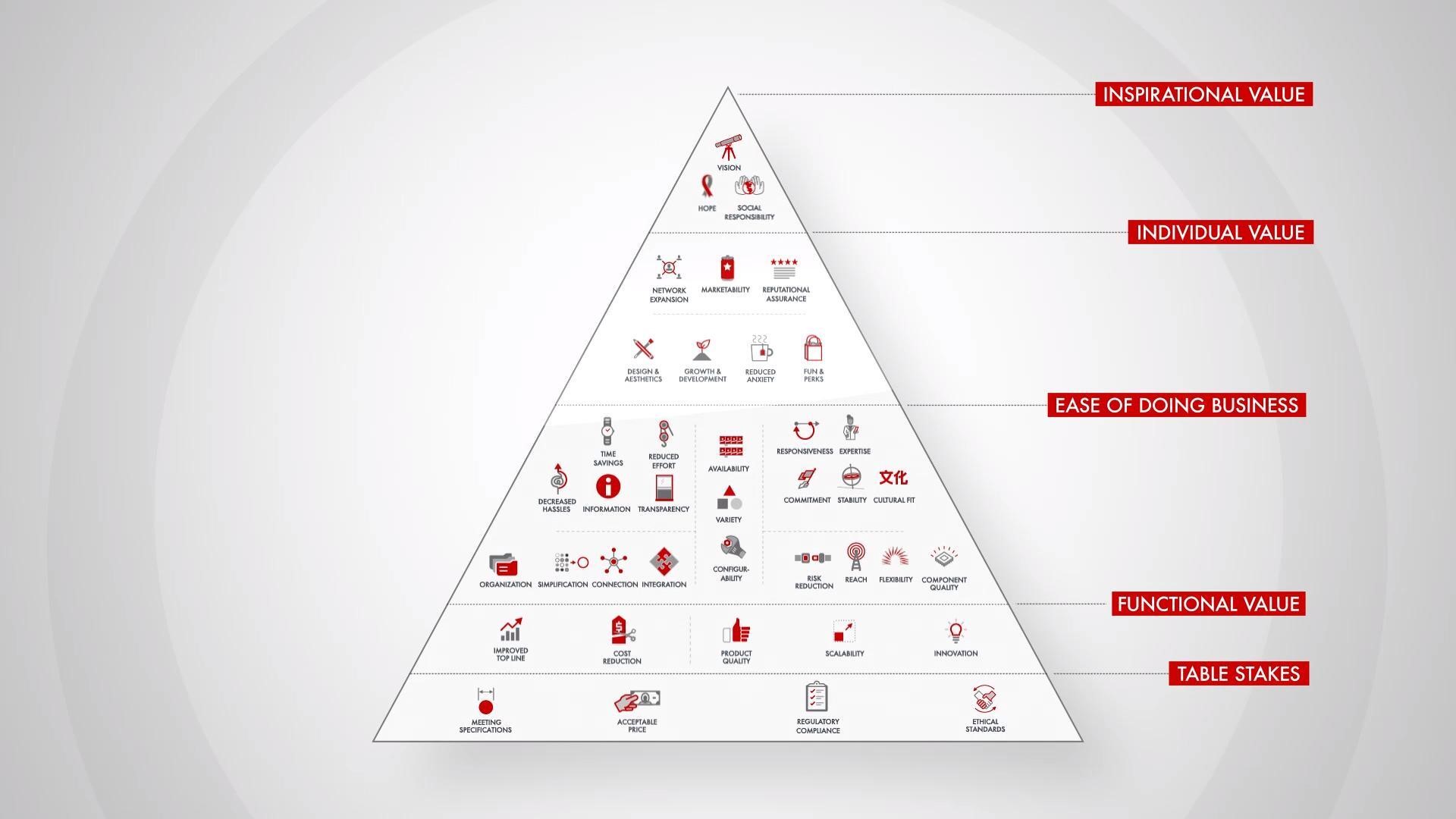
Bain’s Elements of Value Framework
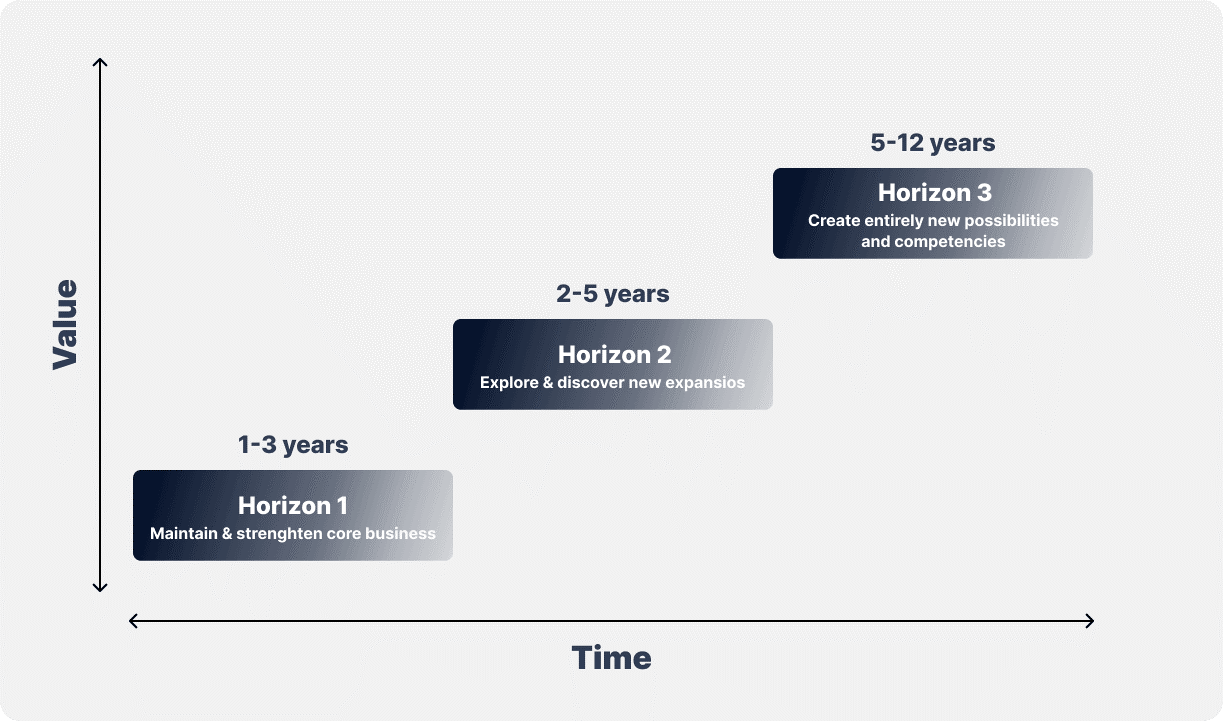
McKinsey Growth Pyramid
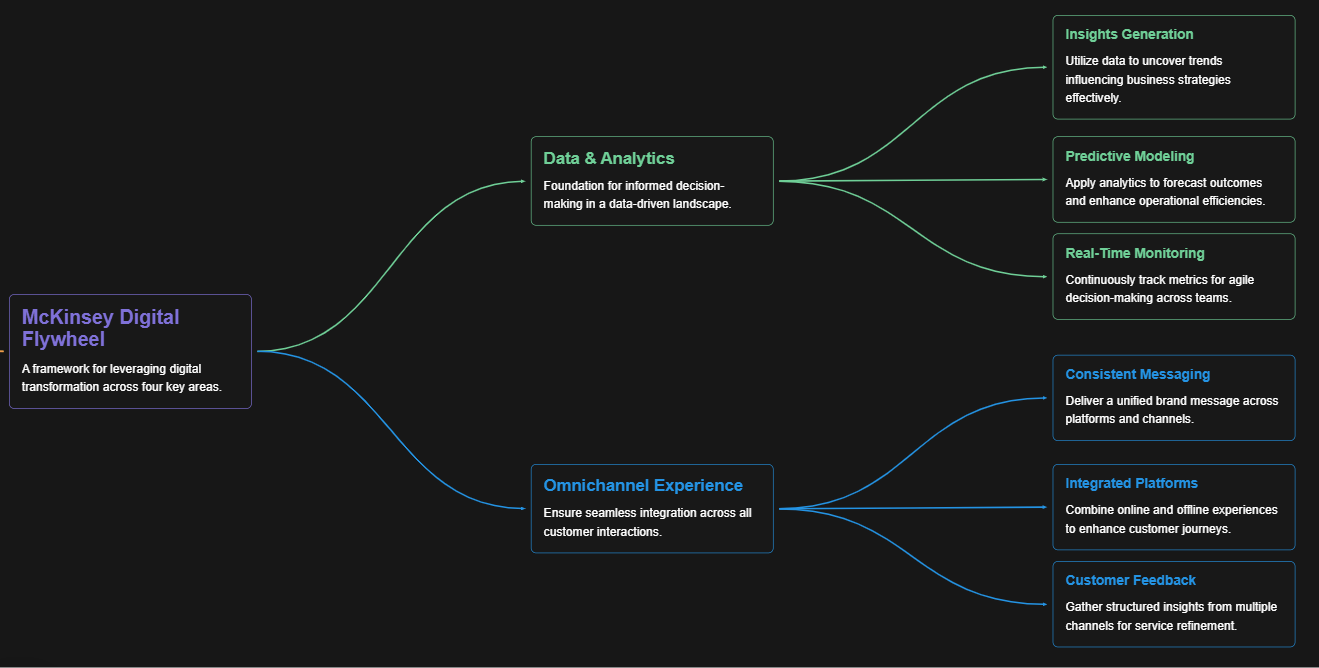
McKinsey Digital Flywheel
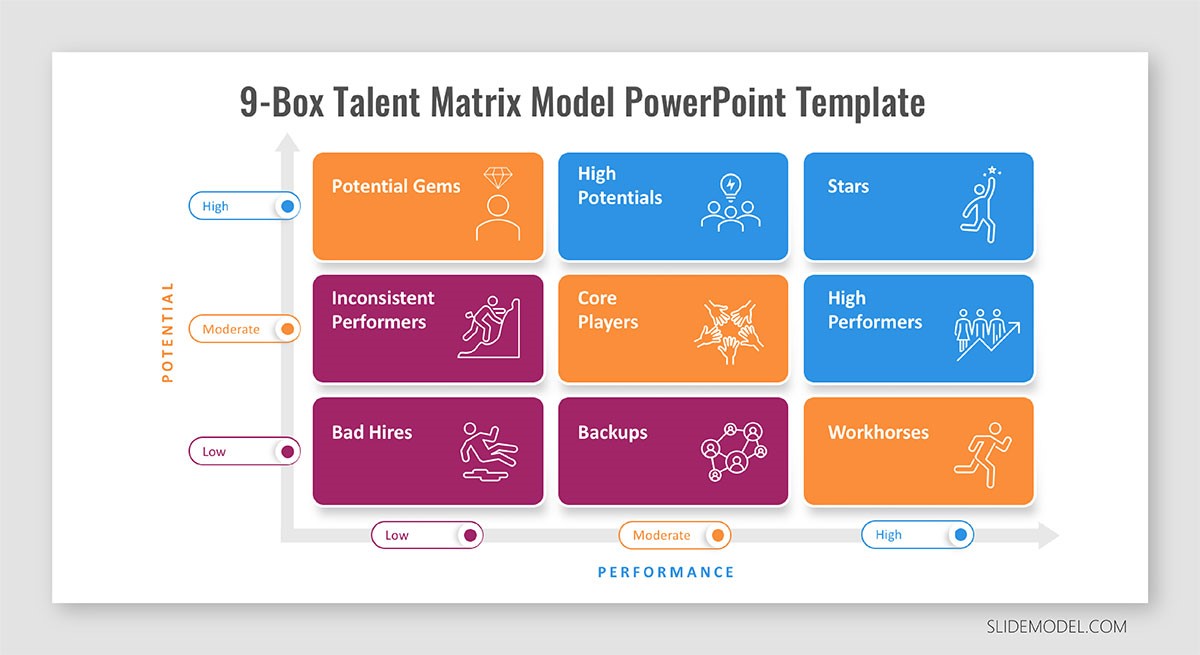
McKinsey 9-Box Talent Matrix
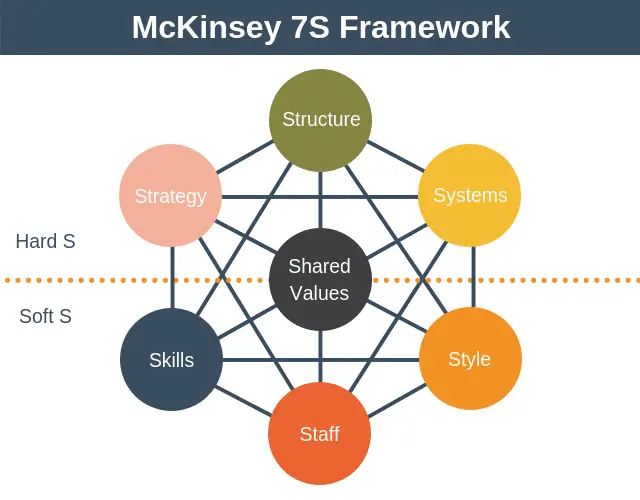
McKinsey 7S Framework
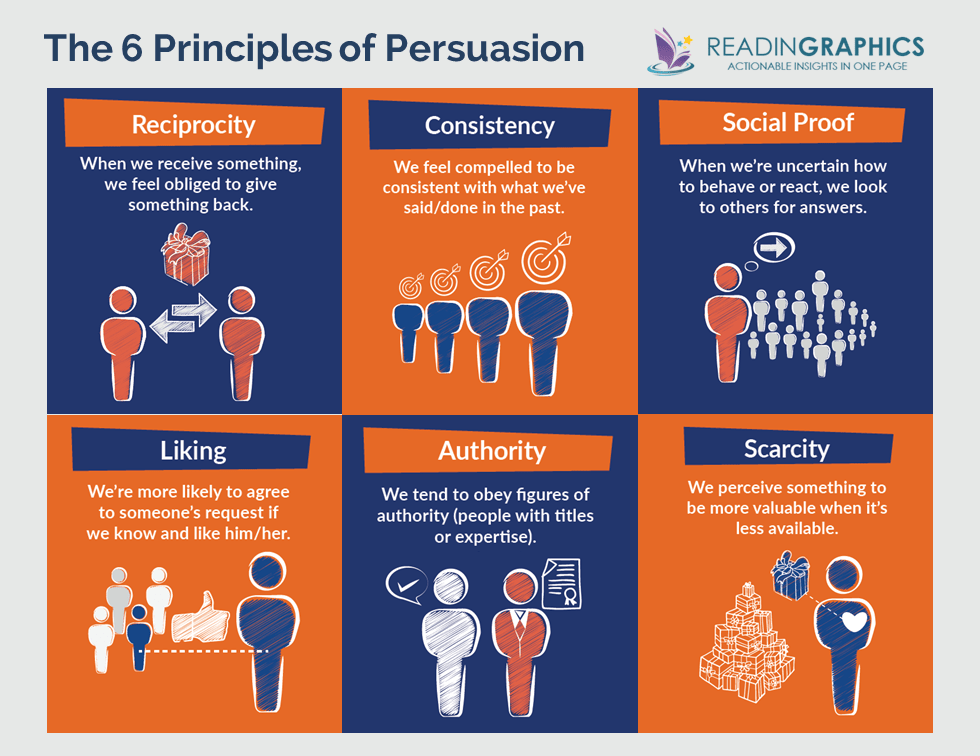
The Psychology of Persuasion in Marketing
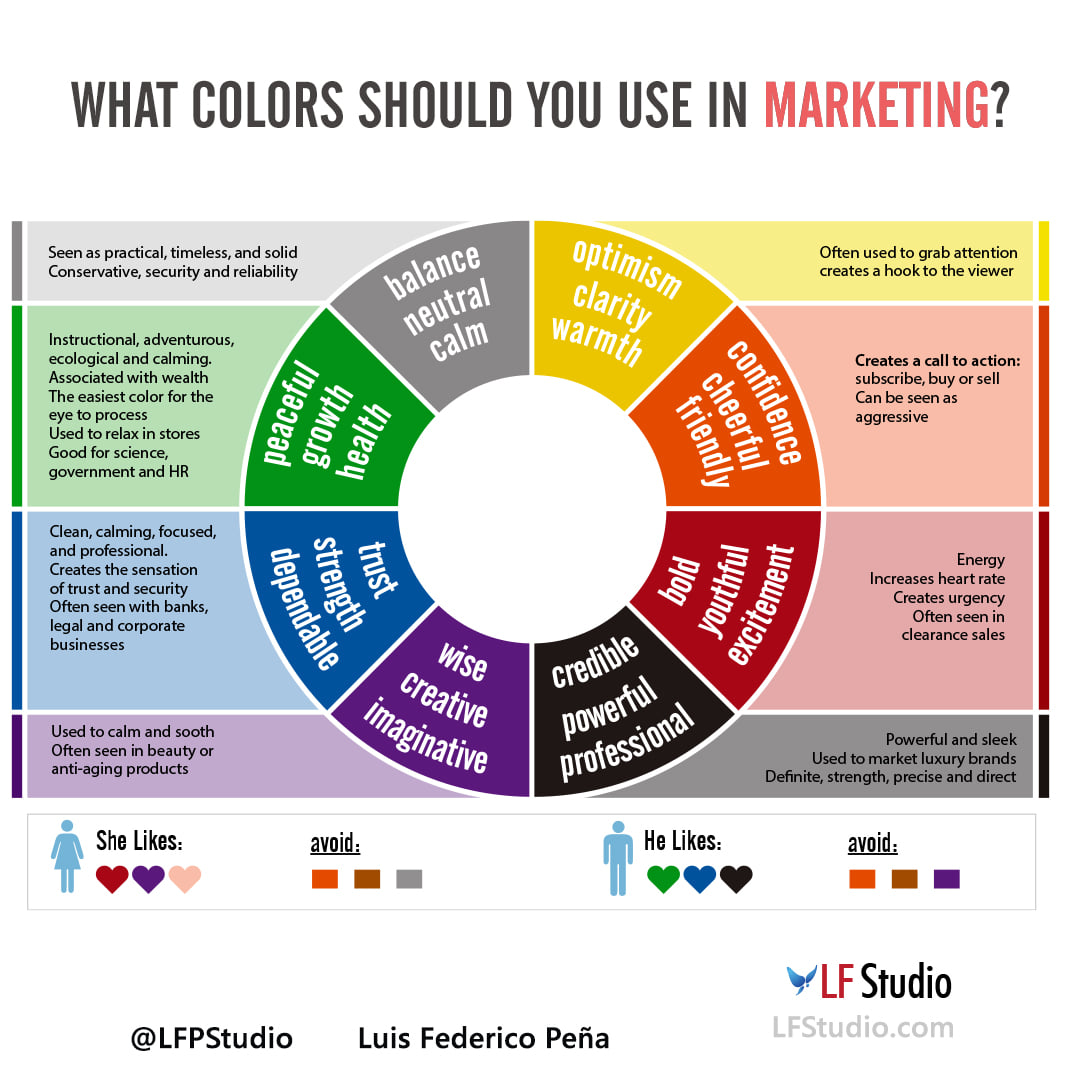
The Influence of Colors on Branding and Marketing Psychology
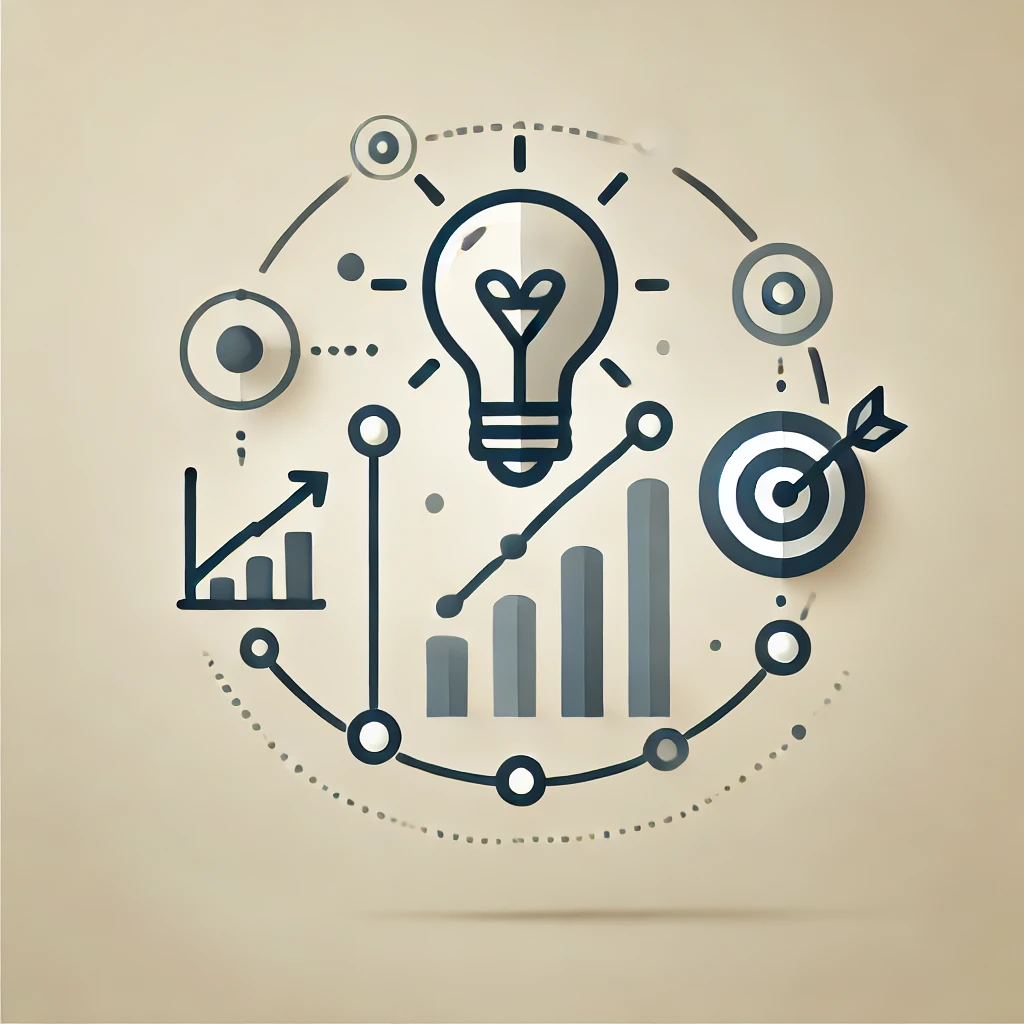
What is Marketing?
Recent Blogs
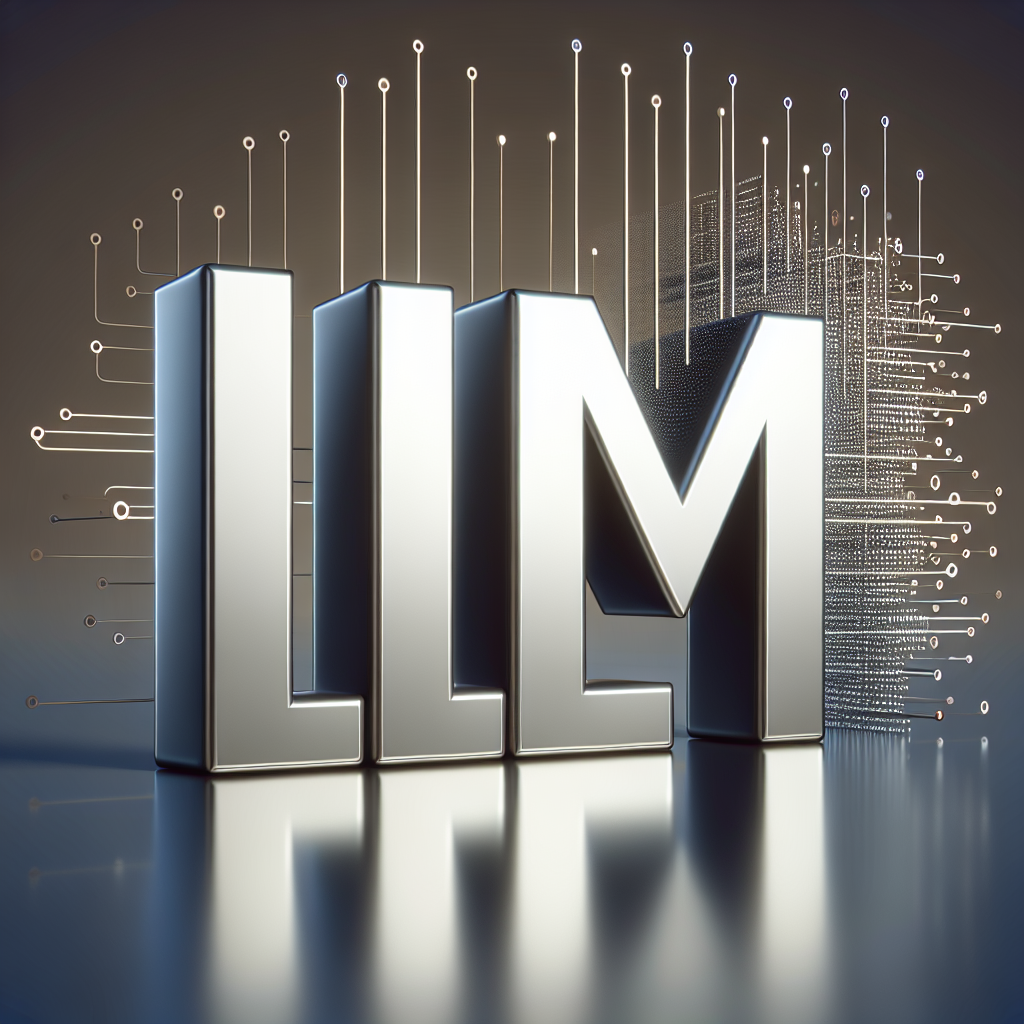
Part 8: From Blocks to Brilliance – How Transformers Became Large Language Models (LLMs) of the series - From Sequences to Sentience: Building Blocks of the Transformer Revolution
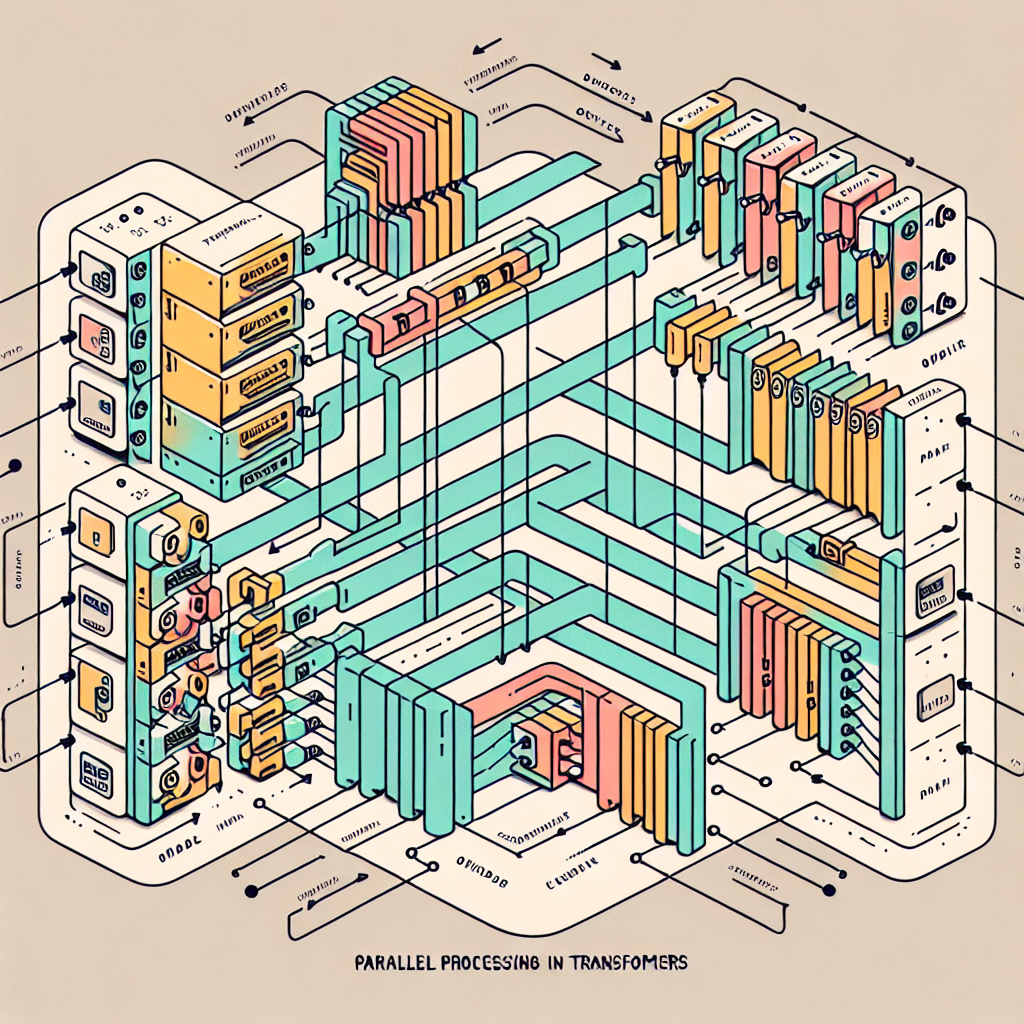
Part 7: The Power of Now – Parallel Processing in Transformers of the series - From Sequences to Sentience: Building Blocks of the Transformer Revolution
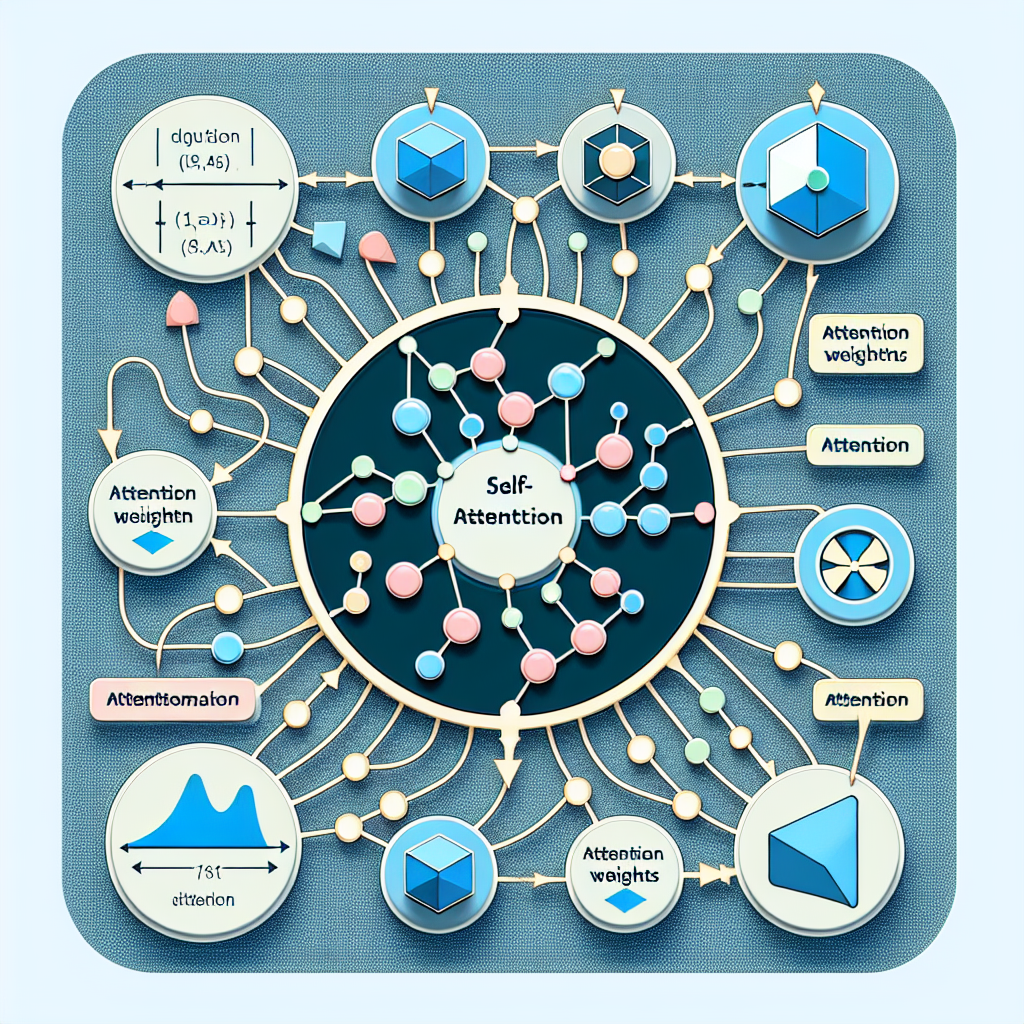
Part 6: The Eyes of the Model – Self-Attention of the series - From Sequences to Sentience: Building Blocks of the Transformer Revolution
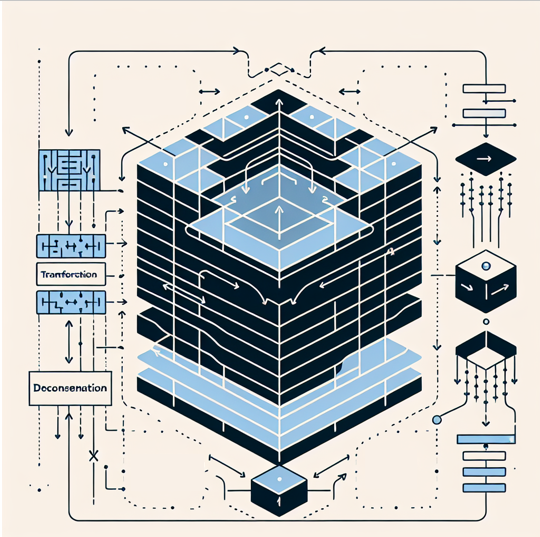
Part 5: The Generator – Transformer Decoders of the series - From Sequences to Sentience: Building Blocks of the Transformer Revolution
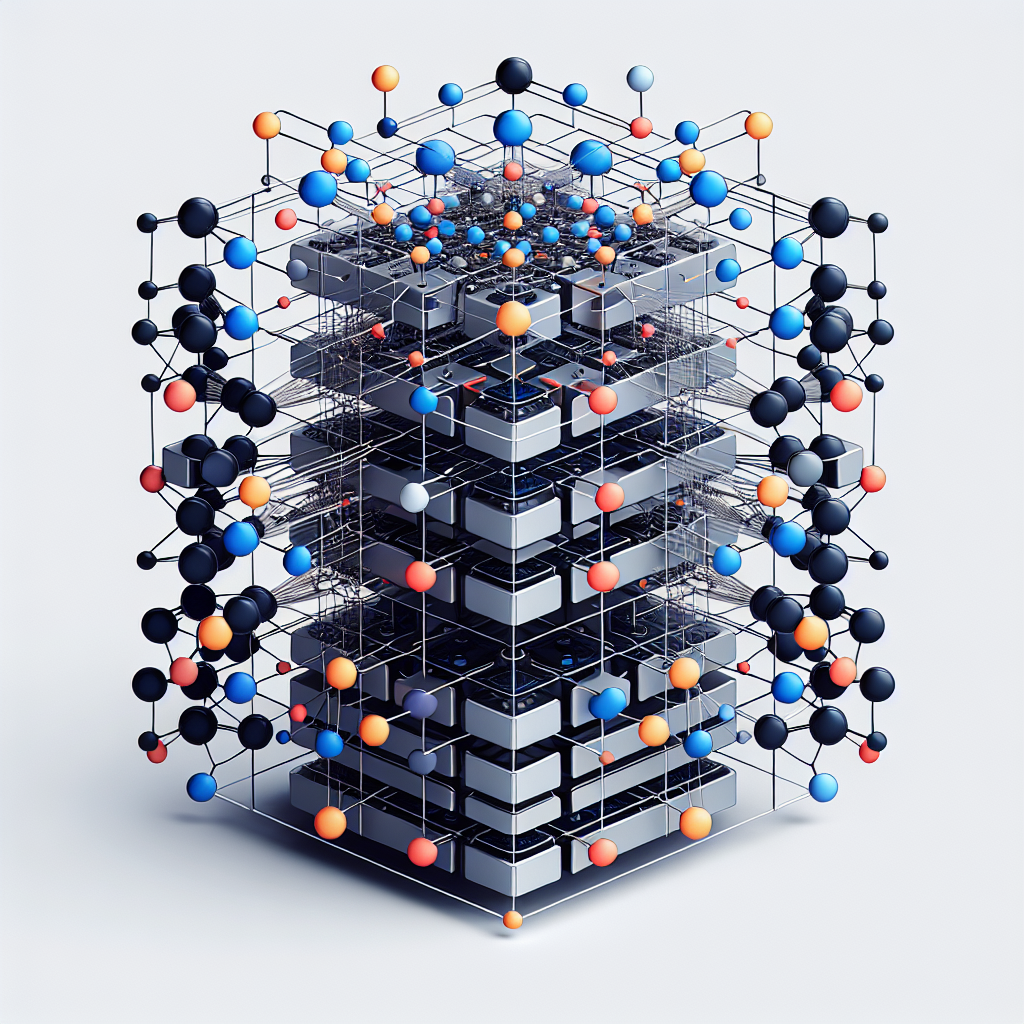
Part 4: The Comprehender – Transformer Encoders of the series - From Sequences to Sentience: Building Blocks of the Transformer Revolution of the series - From Sequences to Sentience: Building Blocks of the Transformer Revolution
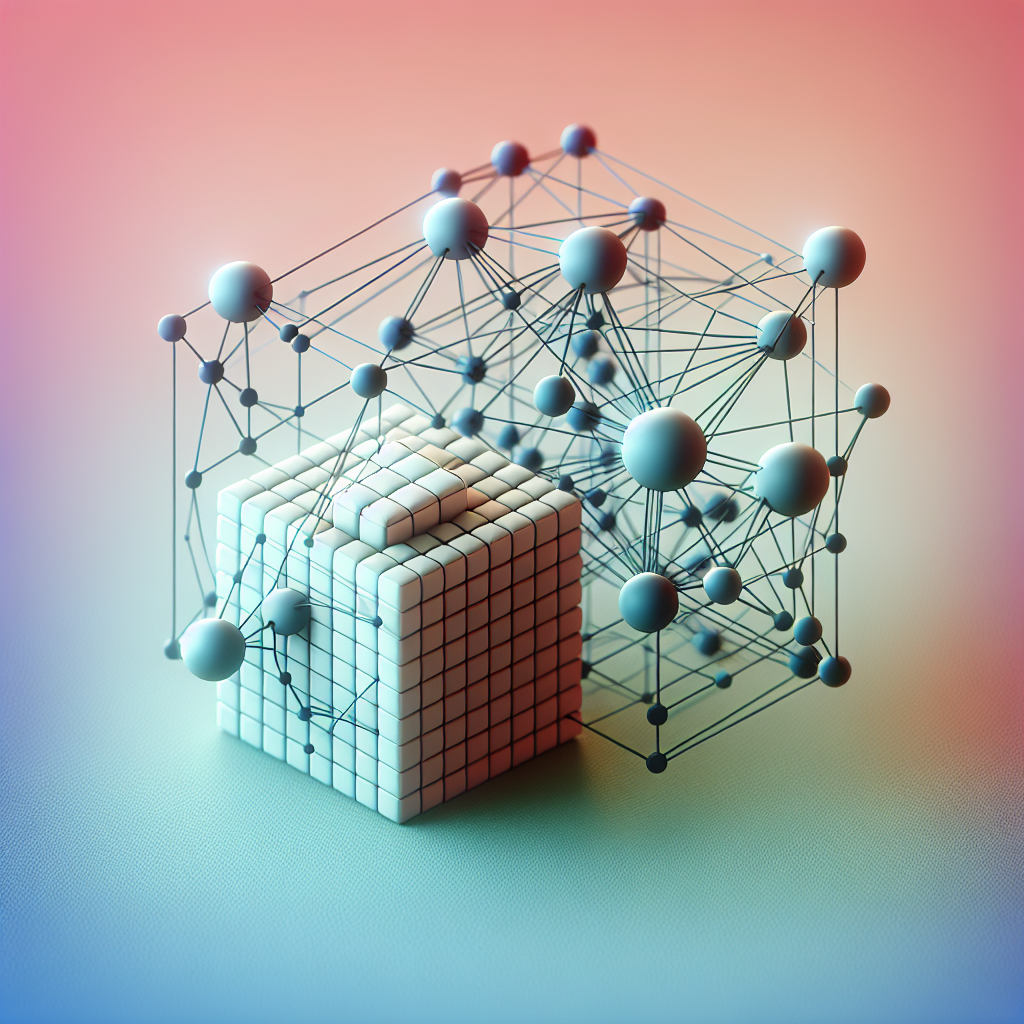