Using Predictive Analytics to Improve Retention
The revelation came to Paul during a quarterly business review with a subscription software company where he served as an advisor. The CFO was presenting what appeared to be strong results—new customer acquisition had increased 24% year-over-year. Yet, the CEO's expression remained troubled. When the retention slides appeared, Paul understood why. Despite their acquisition success, they were losing existing customers faster than they could replace them, creating a costly leaky bucket. "We're always surprised by who leaves," the CEO confessed. "By the time we see the warning signs, it's too late." This conversation fundamentally shifted Paul's perspective on customer retention. He realized that in today's subscription economy, the ability to predict—rather than merely respond to—customer departure signals represents perhaps the most significant competitive advantage a company can develop. This experience launched Paul's exploration into how predictive analytics is transforming retention strategies across industries.
Introduction: The Retention Revolution
Traditional approaches to customer retention operated on reactive principles—identifying at-risk customers through explicit signals like service complaints, declining purchase frequency, or direct cancellation threats. While these methods helped recover some relationships, they focused primarily on customers already in active departure mode, when intervention success rates typically fall below 20%.
Research from Bain & Company reveals that increasing customer retention rates by just 5% increases profits by 25% to 95%, making retention improvement one of the highest ROI activities for most businesses. Meanwhile, Harvard Business Review reports that acquiring a new customer costs 5-25 times more than retaining an existing one, depending on industry and business model.
The emergence of predictive retention analytics—using data science to identify departure signals long before traditional warning signs appear—represents what the Journal of Marketing Research calls "a fundamental transition from reactive retention management to predictive relationship governance."
1. The Science of Departure Prediction
Modern retention prediction models have evolved well beyond simplistic rules-based approaches to incorporate sophisticated data science techniques:
- Machine learning algorithms detecting subtle behavioral patterns
- Natural language processing analyzing sentiment across interactions
- Advanced statistical methods like survival analysis and hazard modeling
- Ensemble approaches combining multiple prediction methodologies
- Deep learning systems identifying complex interaction signals
Streaming entertainment leader Netflix developed their "Retention Genome" project, using machine learning to analyze over 1,700 behavioral signals to predict subscriber departure risk 60-90 days before cancellation. This system identifies subtle viewing pattern changes, engagement shifts, and content engagement markers that predict future churn with 87% accuracy. The resulting early intervention program has reduced cancellations by 16%, representing hundreds of millions in preserved annual revenue.
2. From Single Signals to Behavioral Constellations
Early predictive retention efforts focused on individual warning indicators. Advanced approaches now recognize that departure risk manifests through complex behavioral patterns:
- Engagement velocity changes across multiple dimensions
- Interaction pattern shifts across channels and touchpoints
- Value perception indicators from multiple sources
- Relationship lifecycle stage-specific warning patterns
- Competitive exploration signals from digital footprints
Software company Adobe transformed their Creative Cloud retention strategy by developing "Behavior Constellation Analysis" that examines not just declining usage, but shifts in usage patterns, feature exploration trajectories, and learning engagement that indicate evolving creative needs. This methodology identifies at-risk customers 45-75 days earlier than traditional methods, allowing for targeted capability education that has improved renewal rates by 13.5% across identified segments.
3. Differentiated Intervention Architecture
Sophisticated retention systems match prediction with precisely calibrated interventions:
- Risk severity-appropriate response levels
- Persona-specific retention approaches
- Root cause-targeted intervention selection
- Timing optimization based on intervention receptivity
- Channel appropriateness for relationship context
Telecommunications provider Sprint revolutionized their retention approach through "Precision Intervention Mapping," which matches 14 distinct churn risk profiles to over 125 specific retention treatments delivered through the optimal channel at the optimal time. This precision approach increased retention program effectiveness by 36% while reducing retention marketing costs by 22% by eliminating ineffective broad-based retention offers.
4. The Economics of Early Intervention
Advanced retention analytics creates transformative economic opportunities:
- Intervention cost optimization through early engagement
- Retention resource allocation efficiency improvements
- Customer lifetime value-weighted prioritization
- Relationship salvage rate modeling by intervention timing
- Loyalty economics trajectory forecasting
Financial services leader American Express implemented their "Relationship Value Optimization" system, which combines predictive retention analytics with economic modeling to determine the optimal intervention timing, offer value, and delivery approach for each at-risk customer relationship. This methodology balances intervention costs against relationship salvage probability and future value potential, improving retention program ROI by 53% while maintaining the same retention budget.
5. Ethical Dimensions of Predictive Retention
As predictive capabilities advance, organizations face important ethical considerations:
- Transparency around data usage for retention purposes
- Equitable treatment across customer segments
- Privacy-preserving prediction methodologies
- Value sharing between company and customer
- Avoidance of manipulative retention tactics
Healthcare provider Kaiser Permanente developed their "Member Relationship Ethics Framework" to ensure their predictive retention program respects member autonomy while still delivering proactive care. Their system provides transparent explanations of how behavioral data informs preventative outreach and ensures that departure risk never impacts care quality or access. This ethical approach has increased member trust scores by 14% while still achieving a 9% improvement in voluntary disenrollment rates.
Conclusion: The Future of Relationship Intelligence
The evolution of predictive retention analytics represents more than incremental improvement in customer management—it signals a fundamental shift in how organizations understand and nurture customer relationships. As artificial intelligence and machine learning capabilities continue advancing, the depth and accuracy of relationship intelligence will expand exponentially.
Organizations that master predictive retention will increasingly differentiate themselves through what the Harvard Business Review terms "anticipatory relationship governance"—the ability to not just retain customers, but to proactively evolve relationships before dissatisfaction emerges, creating unprecedented levels of loyalty and lifetime value.
Call to Action
For organizations seeking to advance their predictive retention capabilities:
- Develop a comprehensive data strategy unifying behavioral, transactional, and sentiment signals
- Invest in analytic talent with expertise in predictive modeling and customer behavior
- Create clear ethical guidelines governing predictive retention practices
- Build testing frameworks to validate prediction accuracy and intervention effectiveness
- Establish cross-functional governance ensuring insights translate to coordinated action
- Evolve metrics from lagging indicators (churn rates) to leading indicators (risk detection accuracy)
The future belongs to organizations that transform retention from a reactive recovery function to a predictive relationship discipline, preserving and growing customer value before it becomes endangered.
The most sophisticated organizations are already progressing beyond merely predicting departure to forecasting relationship evolution needs—anticipating when customers will require new capabilities, experiences, or relationship dimensions before the customers themselves recognize these emerging needs.
Featured Blogs
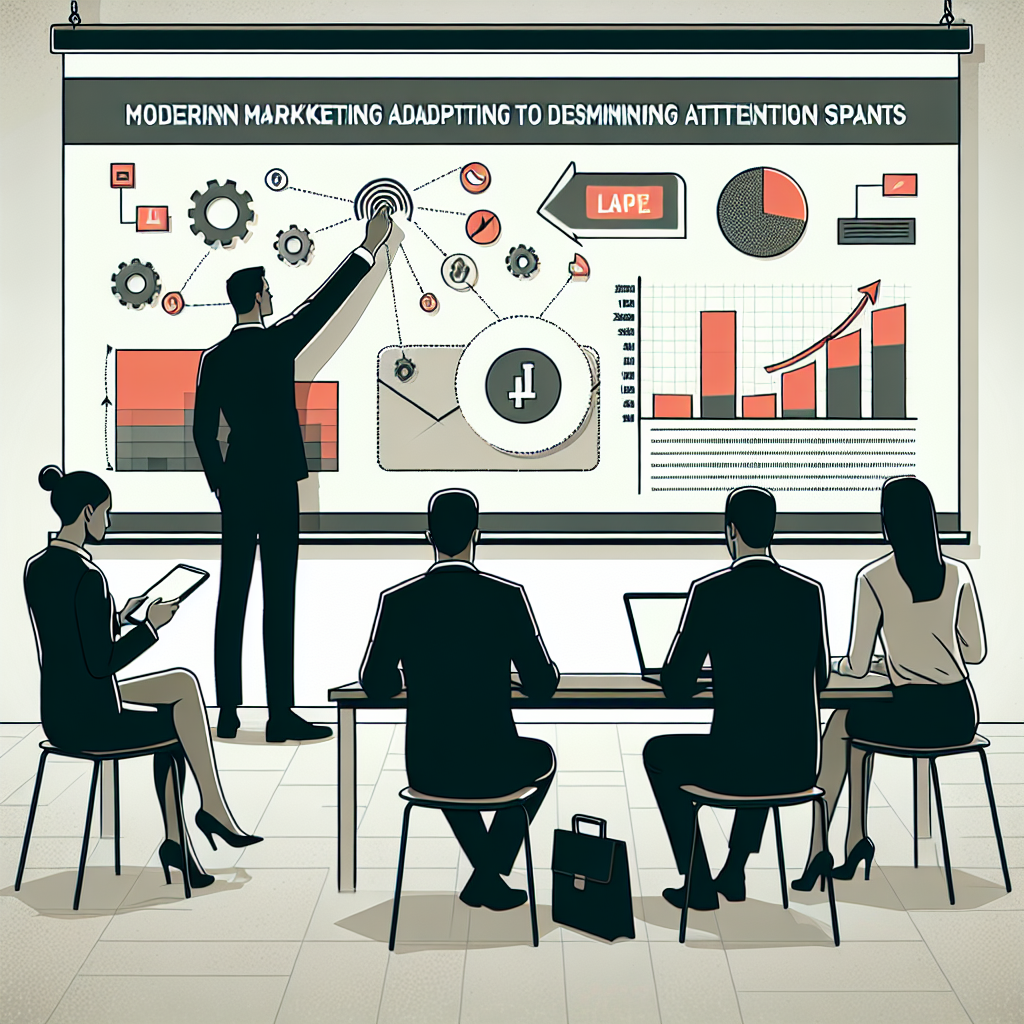
How the Attention Recession Is Changing Marketing
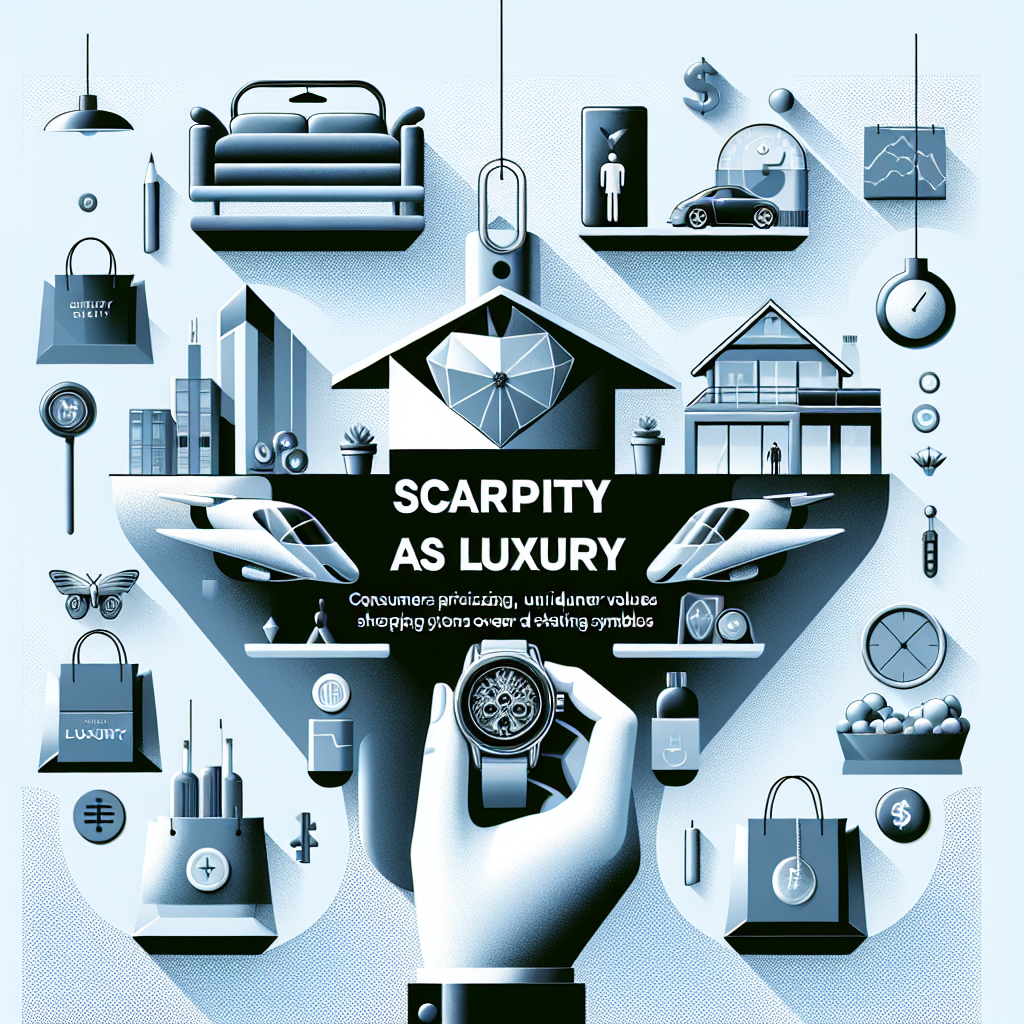
The New Luxury Why Consumers Now Value Scarcity Over Status
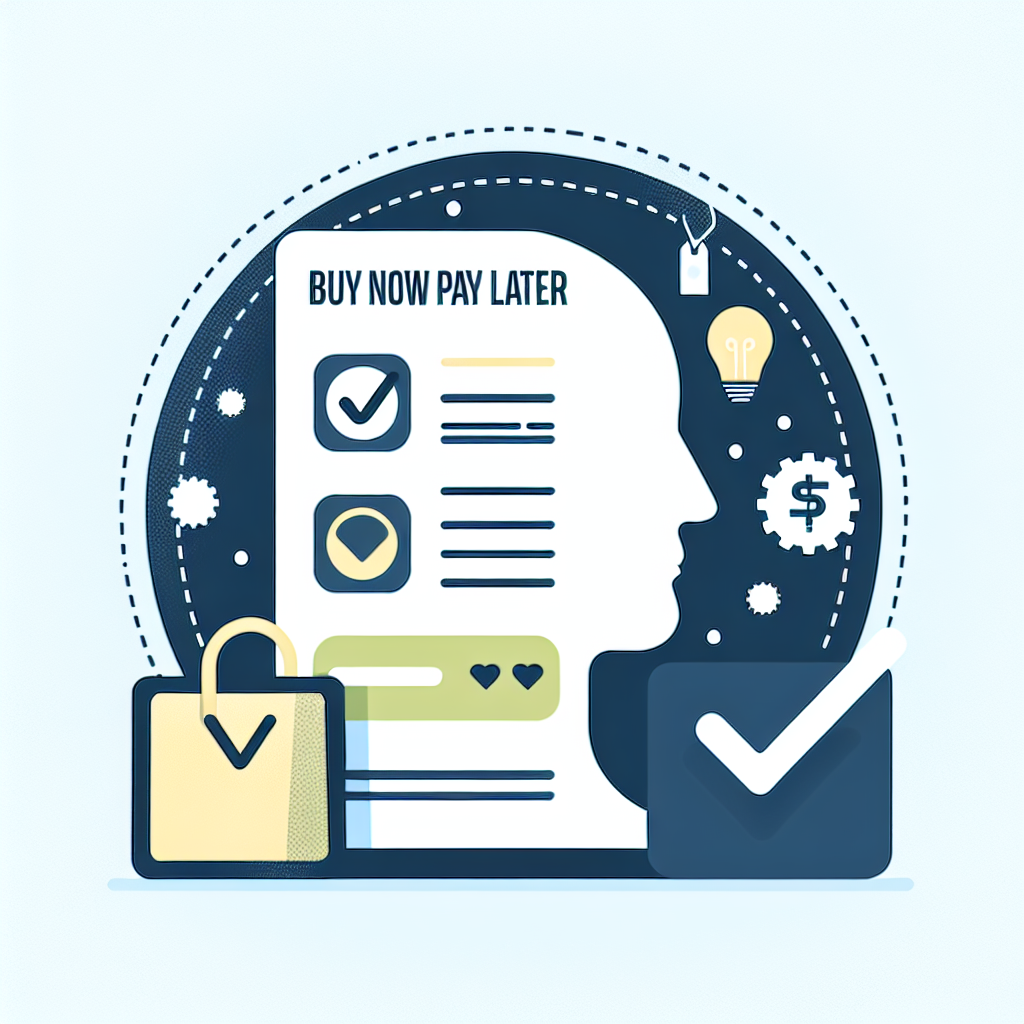
The Psychology Behind Buy Now Pay later
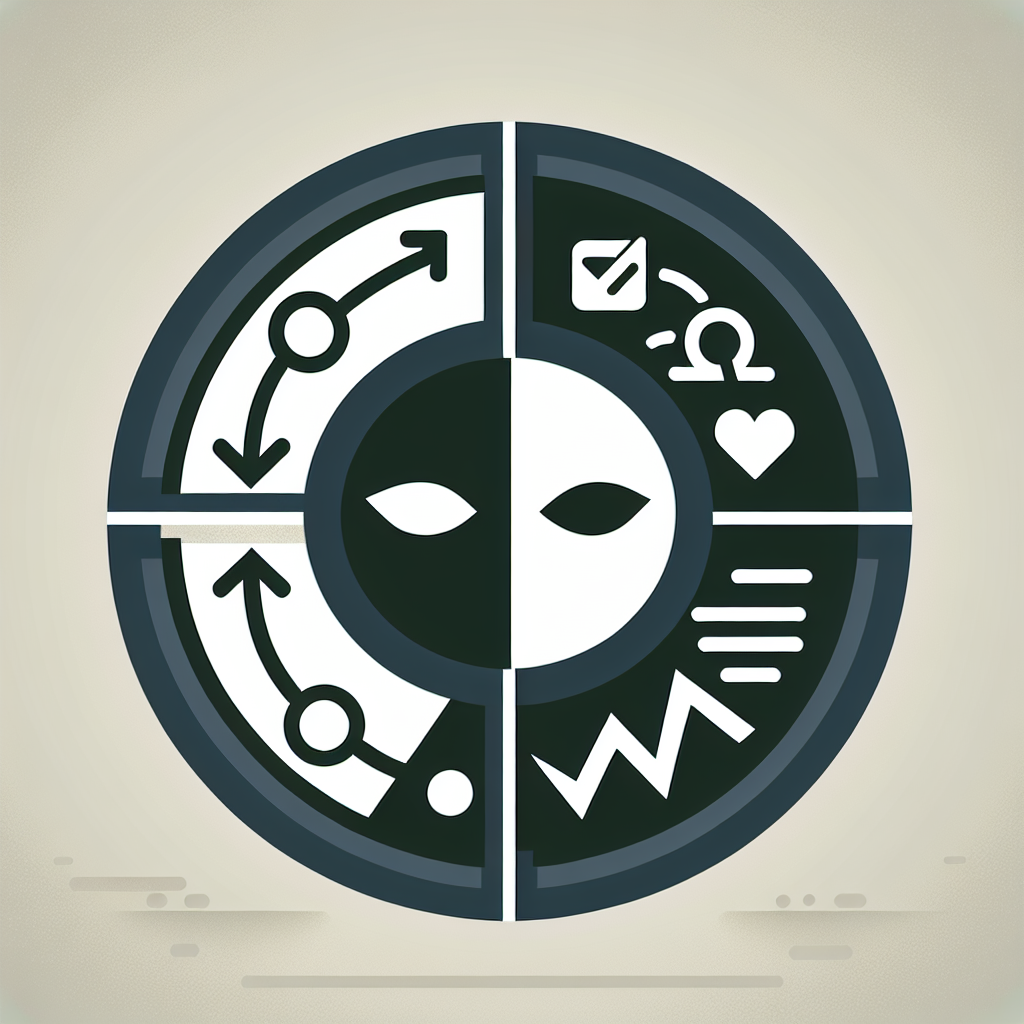
The Role of Dark Patterns in Digital Marketing and Ethical Concerns
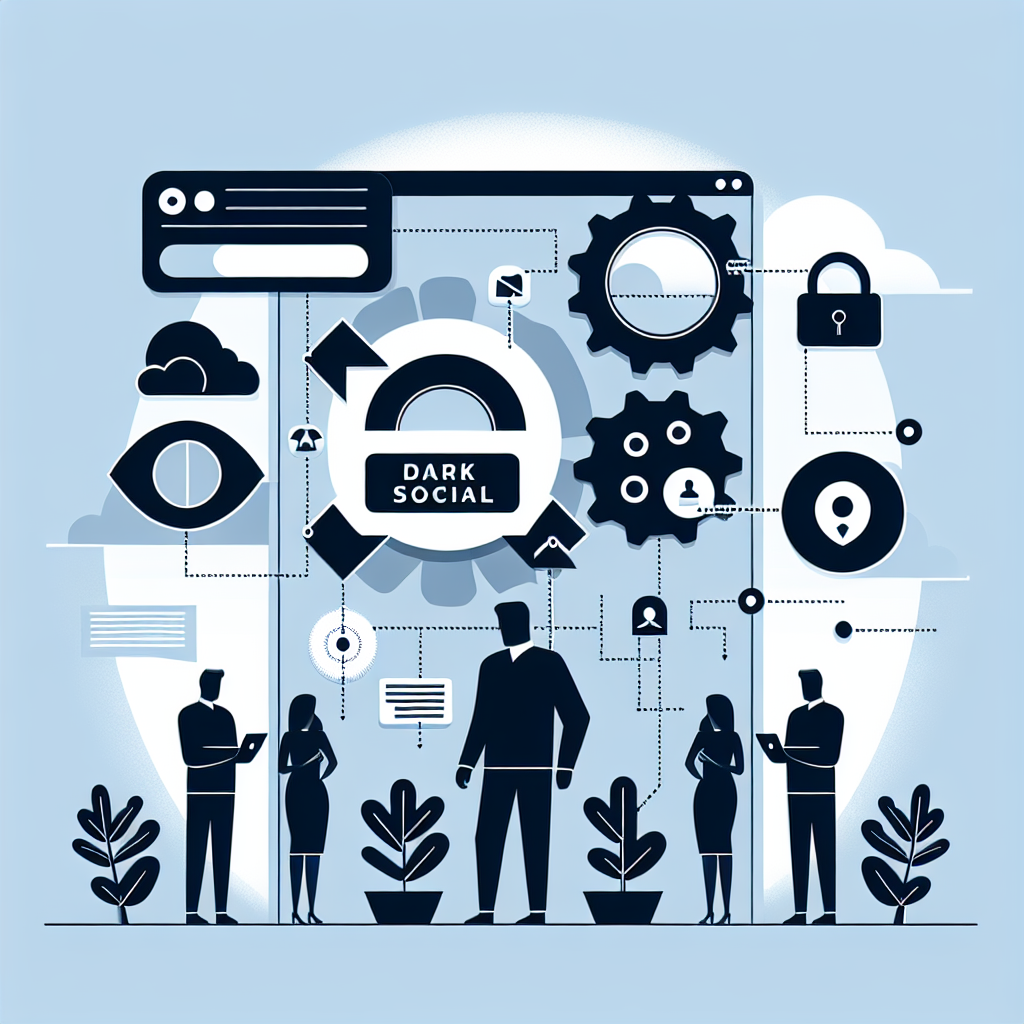
The Rise of Dark Social and Its Impact on Marketing Measurement
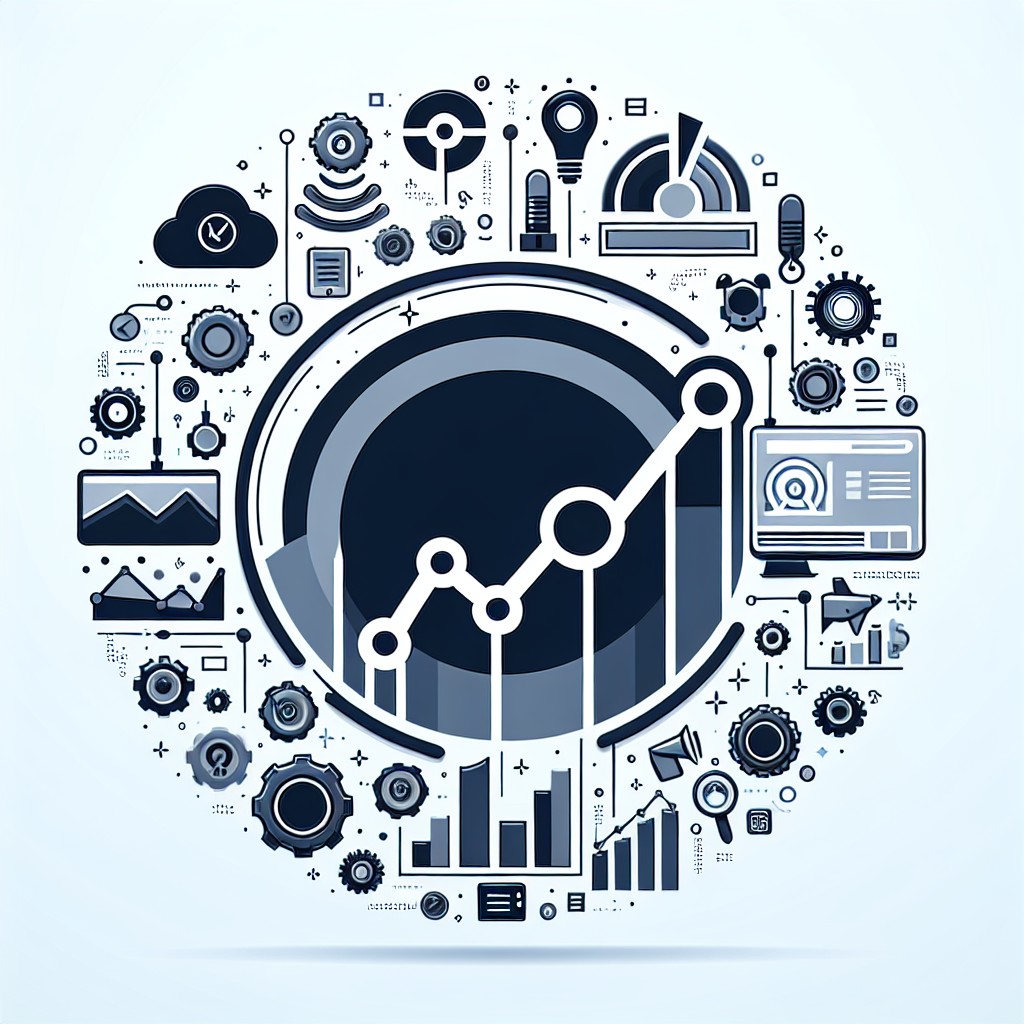
The Future of Retail Media Networks and What Marketers Should Know
Recent Blogs
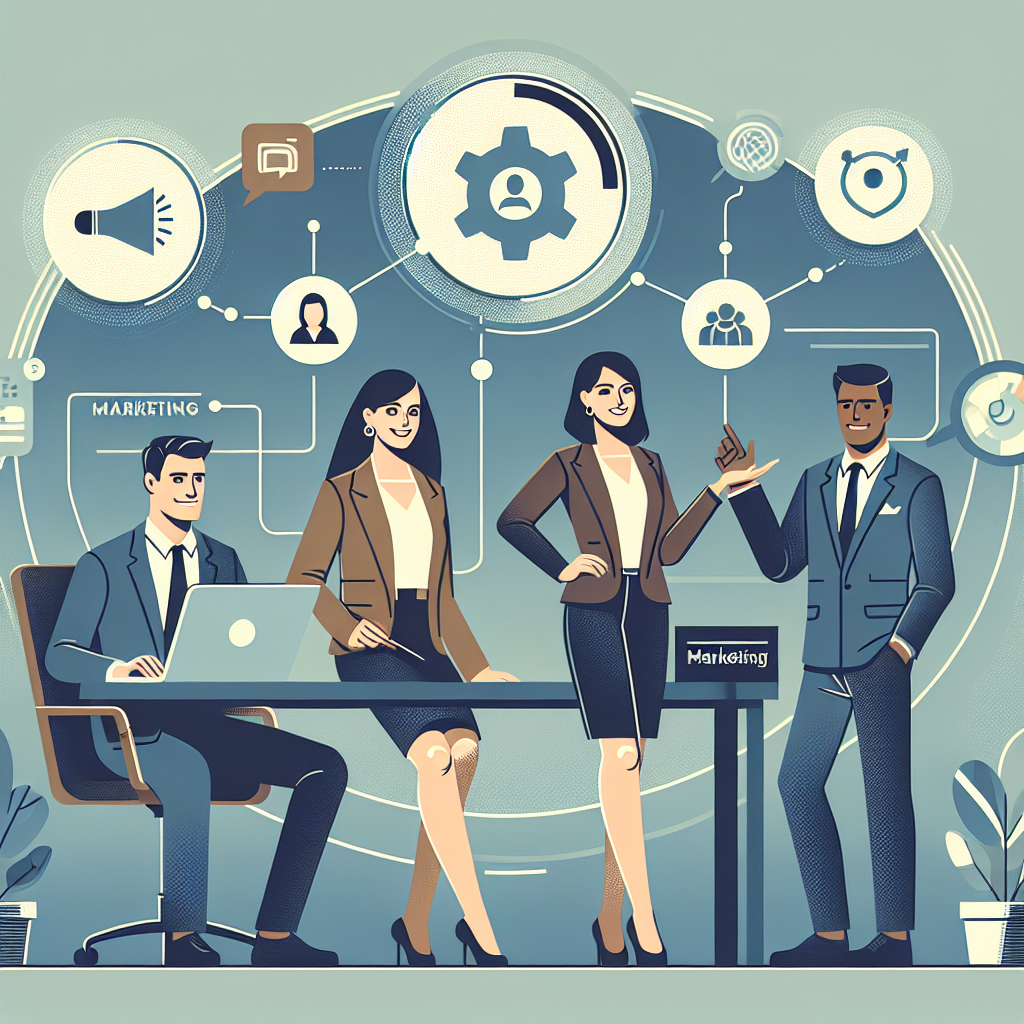
From Service to Experience - Training Teams for the Shift
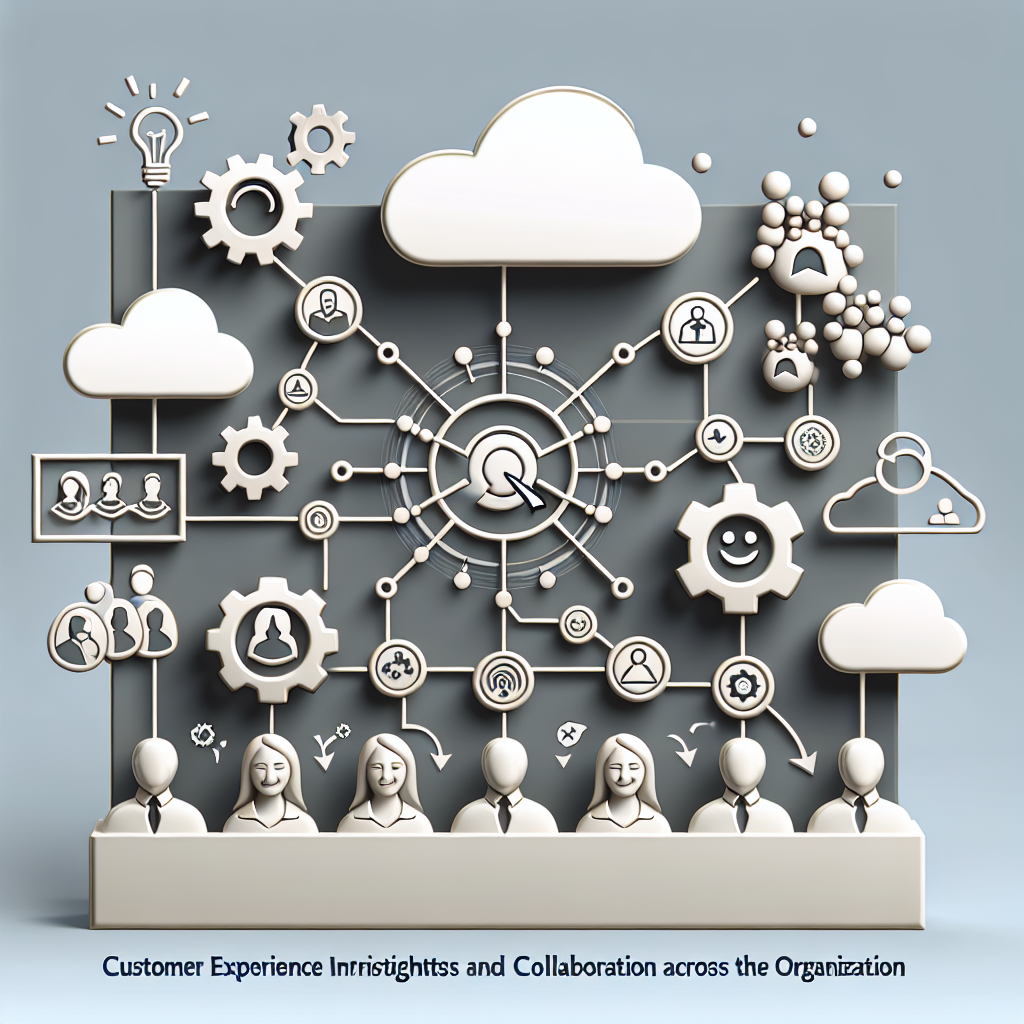
How to Democratize CX Insights Across the Org
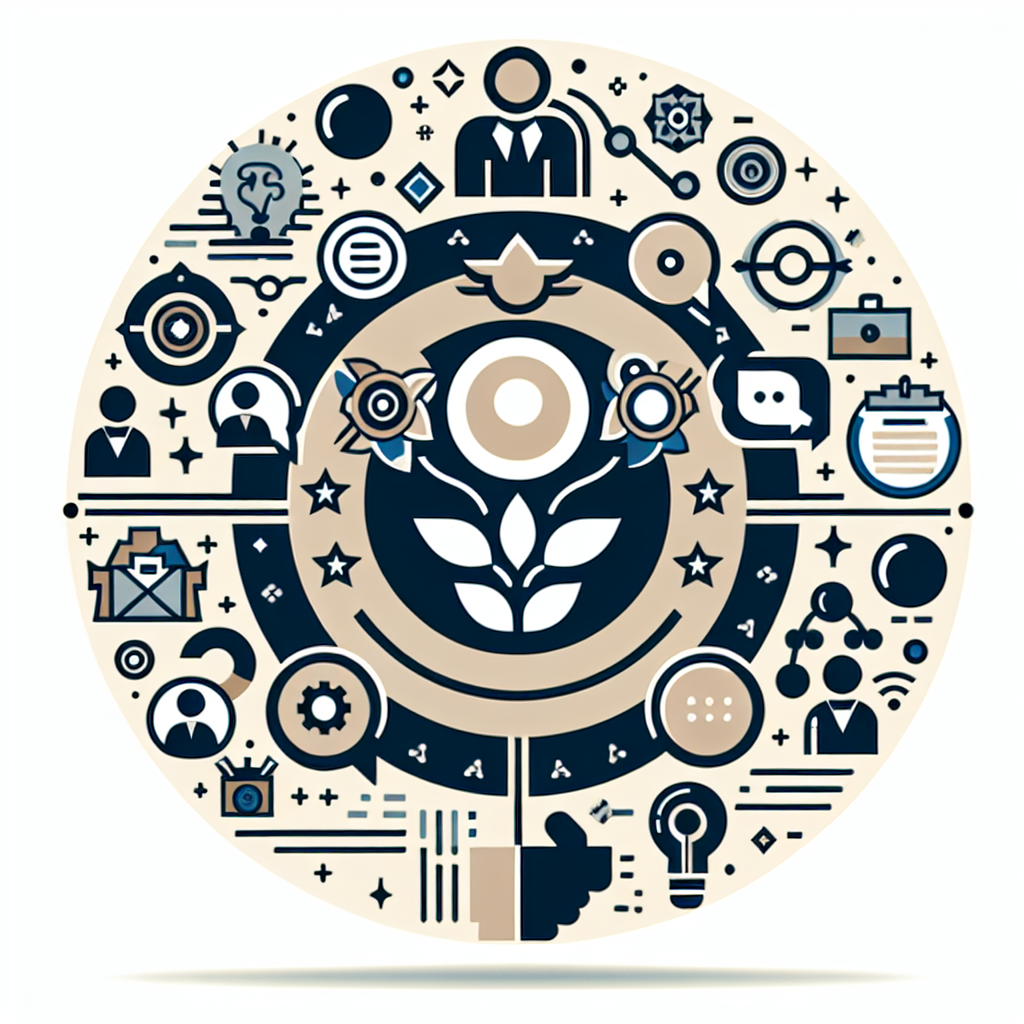
CX is Everyone's Job Building a Customer-Centric Culture
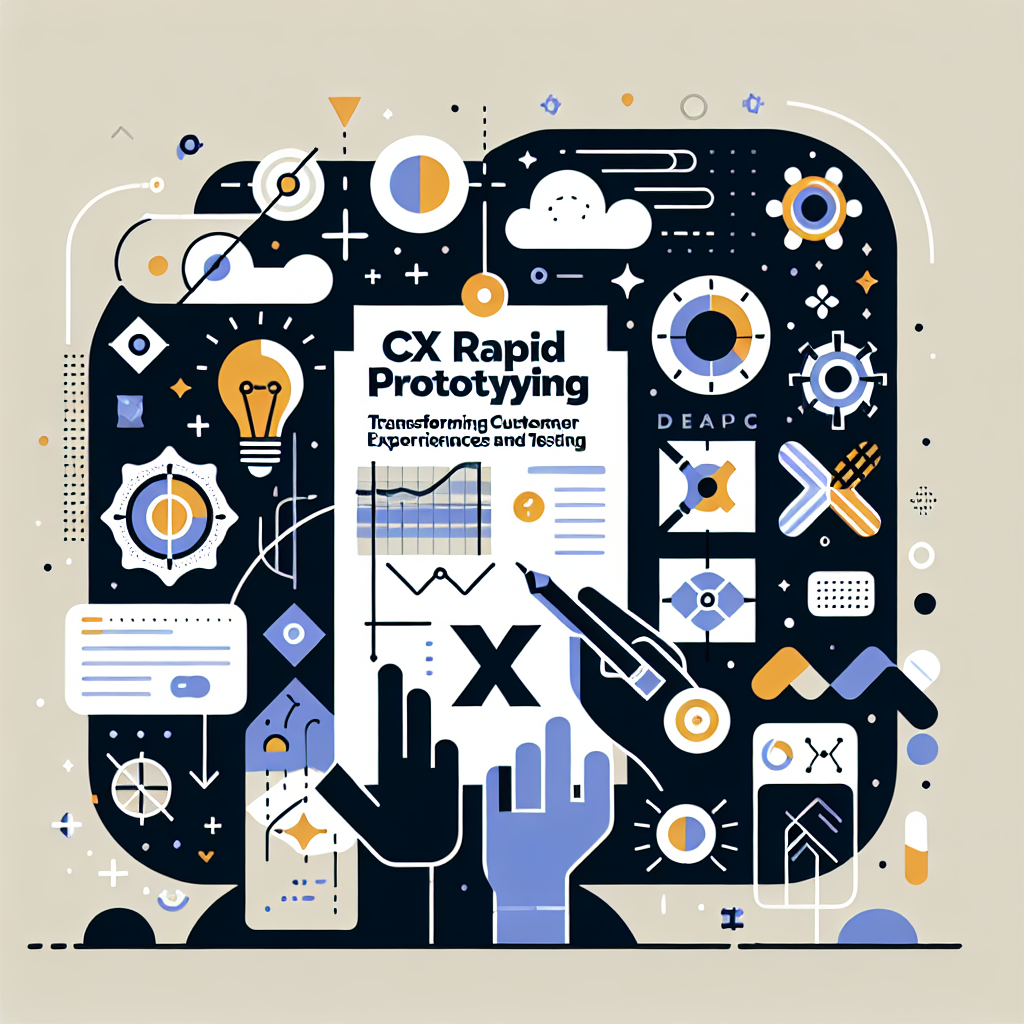
Experimenting with CX Rapid Prototyping for New Journeys
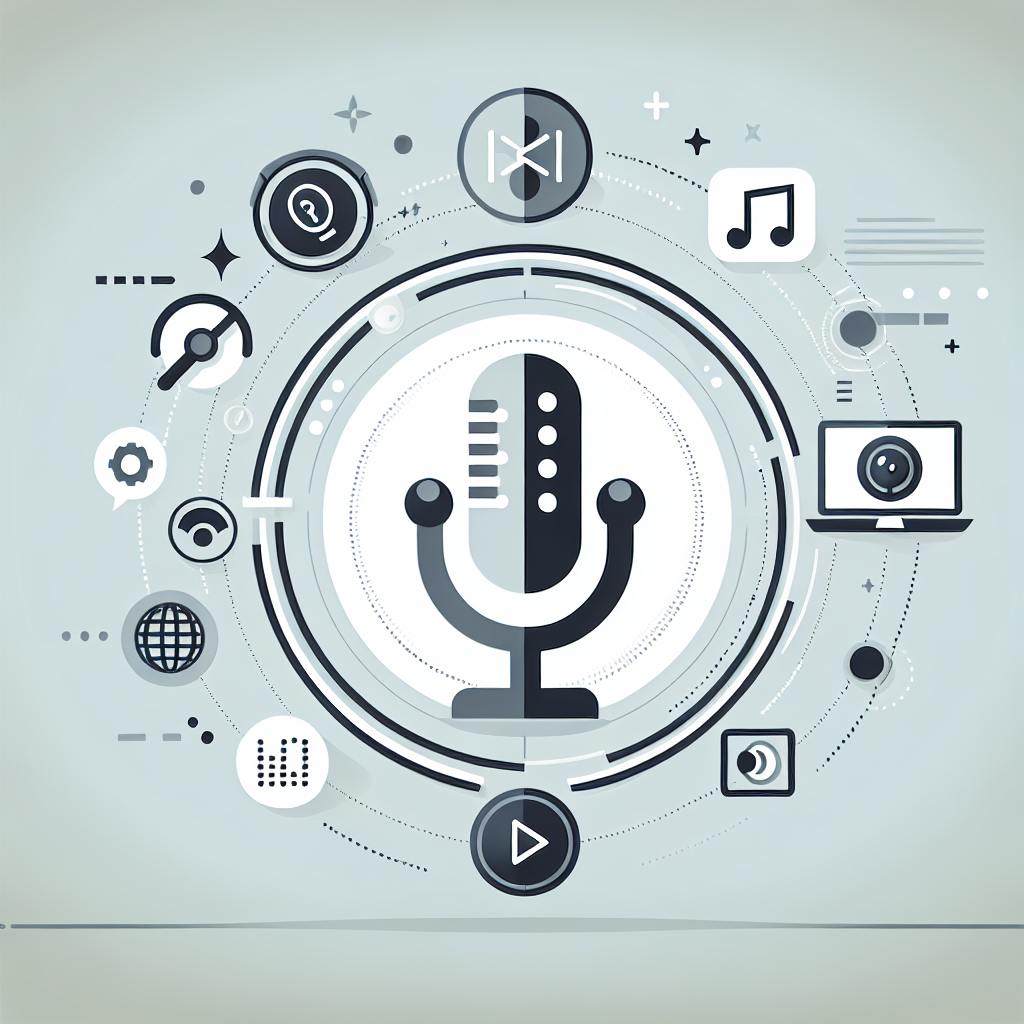
Voice, Video, and Beyond The Multimodal CX Future
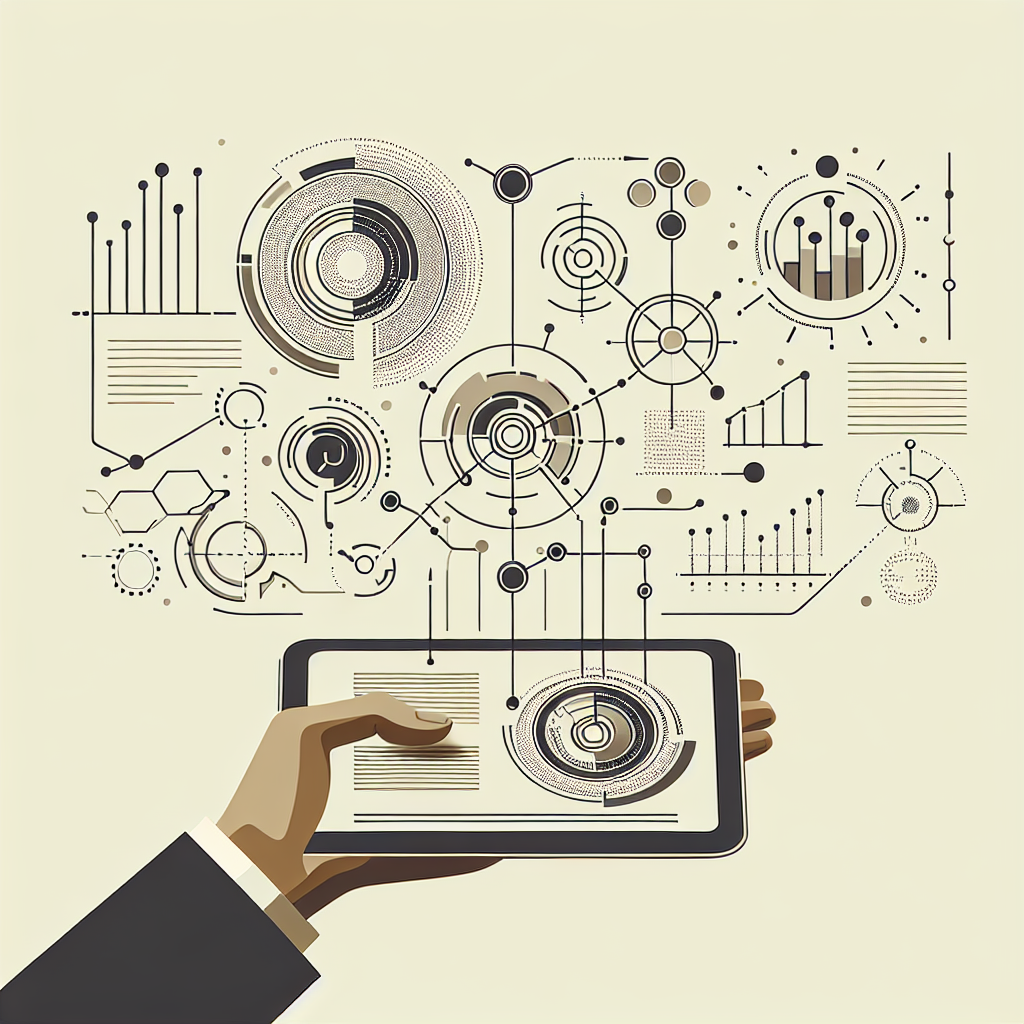