Predictive Budgeting in Marketing Using AI
The quarterly planning meeting was reaching its predictable conclusion. The marketing team, led by Paul, had spent weeks preparing meticulous budget proposals, only to have the CFO question every assumption. "But how do you know this channel will perform next quarter? What happens if competitive pressure increases? Where's your contingency plan?" As Paul watched the CMO struggle to provide convincing answers, a notification appeared on his phone: their AI-powered forecast had just been updated with the previous day's results, suggesting a shift of 18% of their social budget to connected TV based on emerging performance patterns. The irony wasn't lost on Paul—while they debated last month's performance data, AI was already processing yesterday's results to predict tomorrow's outcomes. Six months later, the company had transformed its budget planning from quarterly PowerPoint debates to a continuous, AI-guided process that identified opportunities and risks before humans could even spot the patterns.
Introduction: The Transformation of Marketing Budgeting
Marketing budget planning is undergoing a fundamental transformation, shifting from periodic, backward-looking processes to continuous, forward-looking systems powered by artificial intelligence. According to research from Gartner, organizations using predictive AI for budget planning report 31% higher marketing ROI and 24% faster response to market changes compared to those using traditional methods.
This evolution comes at a critical time. Marketing environments are increasingly characterized by volatility, rapid channel proliferation, and compressed decision windows. Traditional budgeting approaches—annual plans with quarterly reviews based on historical performance—cannot keep pace with market dynamics. Meanwhile, the explosion of marketing data has created both opportunity and complexity, with the average enterprise marketing department now managing 23 distinct data sources according to Forrester.
AI-powered predictive budgeting represents what Deloitte has called "the third wave of marketing analytics"—moving beyond descriptive analytics (what happened) and diagnostic analytics (why it happened) to predictive intelligence that forecasts outcomes and prescribes optimal resource allocation.
1. Forecasting Spend and Outcomes
Advanced AI systems are revolutionizing marketing forecasting through sophisticated modeling techniques and unprecedented data integration capabilities.
Multi-Signal Forecasting Models
Modern predictive systems incorporate diverse signals:
- Historical marketing performance data
- Seasonal pattern recognition
- Macroeconomic indicator correlation
- Competitive activity monitoring
- Consumer sentiment analysis
Luxury retailer Nordstrom implemented a multi-signal forecasting system that predicted holiday season performance with 94% accuracy—three months in advance—by integrating traditional marketing metrics with economic indicators, weather patterns, and social media sentiment analysis.
Scenario Planning Automation
AI enables systematic exploration of alternative futures:
- Monte Carlo simulations of potential outcomes
- Sensitivity analysis across hundreds of variables
- Automated "what-if" scenario generation
- Confidence interval calculation for forecasts
Consumer packaged goods giant Procter & Gamble developed an AI-powered scenario planning platform that automatically generates 500+ potential market scenarios each quarter, identifying both opportunities and vulnerabilities that human analysts had consistently missed in traditional planning processes.
Channel Interaction Modeling
Advanced systems quantify complex relationships between channels:
- Cross-channel synergy prediction
- Cannibalization effect forecasting
- Diminishing returns threshold identification
- Attribution weight dynamic adjustment
When automotive marketplace TrueCar implemented AI-driven budget forecasting, they discovered previously unrecognized synergies between podcast advertising and paid search—the system predicted a 34% performance improvement by coordinating flight timing between these channels, a relationship too complex for human analysts to identify from historical data alone.
Example: Streaming platform Spotify developed "Maestro," an AI forecasting system that integrates marketing performance data with 150+ external variables to predict customer acquisition costs across 31 channels. This system forecasts CAC with 91% accuracy 60 days in advance, enabling proactive budget adjustments before performance changes materialize. The platform automatically generates weekly budget reallocation recommendations that have increased marketing efficiency by 26% compared to quarterly human-led planning.
2. Risk Modeling
AI-powered budget planning introduces sophisticated risk assessment capabilities that transform uncertainty from a threat to a manageable variable.
Volatility Prediction
Advanced models quantify performance variability:
- Channel-specific volatility scoring
- Seasonal risk factor calculation
- Competitive threat anticipation
- Black swan event simulation
Financial services company Capital One implemented AI-driven volatility prediction that categorizes marketing investments into stability tiers, establishing appropriate reserve requirements for each category. This approach enabled more aggressive investment in high-opportunity areas while maintaining appropriate risk controls.
Risk-Adjusted Return Optimization
Sophisticated systems balance opportunity with uncertainty:
- Modern portfolio theory application to channel mix
- Efficient frontier identification for budget allocation
- Correlation analysis between channel performances
- Optimal diversification modeling
Travel marketplace Expedia adopted a portfolio theory approach to marketing investment, using AI to identify the optimal balance of high-return/high-volatility channels with stable-performing channels. This risk-adjusted methodology increased overall marketing ROI by 18% while reducing performance volatility by 24%.
Dynamic Budget Contingency Planning
AI enables automated risk mitigation approaches:
- Triggered reallocation rules based on early warning signals
- Automated budget throttling in underperforming scenarios
- Opportunity sensing for outperforming channels
- Competitive response modeling and countermeasures
Technology company Microsoft implemented "dynamic guardrails" in their AI budgeting system that automatically adjusts investment levels when performance deviates from forecasts by predefined thresholds. This approach reduced negative performance variance by 62% compared to standard quarterly planning cycles.
Example: E-commerce giant Shopify developed "ResiliencePlanner," an AI system that conducts continuous stress testing of marketing budgets against 1,000+ simulated market scenarios. The platform quantifies the Value-at-Risk (VaR) for each channel investment, automatically adjusting allocations to maintain optimal risk-return profiles as market conditions change. This approach has enabled the company to maintain consistent customer acquisition targets despite highly volatile market conditions.
3. Integration with CRM/CDPs
The integration of predictive budgeting with customer data platforms creates closed-loop systems that continuously optimize marketing investment against customer lifetime value.
Customer-Centric Budget Optimization
Advanced systems allocate resources based on customer value:
- CLV-optimized channel investment
- Segment-specific budget allocation
- Acquisition/retention balance modeling
- High-value persona targeting investment
Subscription software company Adobe implemented customer-centric budget optimization that allocates investment based on predicted lifetime value rather than immediate conversion metrics. This approach shifted 28% of budget toward channels that acquired higher-value customer segments, increasing customer lifetime value by 34% while maintaining stable acquisition volumes.
Closed-Loop Learning Systems
AI enables continuous performance adaptation:
- Automated feedback loops between outcomes and forecasts
- Self-correcting model adjustment
- Continuous hypothesis testing through micro-experiments
- Machine learning model performance improvement over time
Food delivery platform DoorDash developed a closed-loop budget optimization system that automatically generates and tests hundreds of micro-investment hypotheses each month across geographical markets. These automated experiments continuously refine the system's predictive models, improving forecast accuracy by 6-8% quarterly.
First-Party Data Activation
CDP integration enhances budget optimization:
- Lookalike audience performance forecasting
- Customer journey stage investment optimization
- Cross-sell/upsell opportunity identification
- Retention risk prediction and intervention
Telecommunications company T-Mobile integrated their CDP with predictive budget planning to forecast the impact of marketing investments on specific customer microsegments. This approach enabled precision investment in high-potential segments while reducing spending on poor-performing audiences, increasing marketing ROI by 31%.
Example: Hospitality company Marriott International implemented "ValueMapper," an AI system that integrates CDP data with their marketing budget platform. The system continuously forecasts the expected lifetime value impact of every marketing dollar across 230+ customer microsegments and 45 channels. This approach transformed budget planning from channel-centric to customer-centric, increasing customer lifetime value by 28% while maintaining stable marketing investment levels.
Conclusion: The Future of Marketing Budget Planning
The integration of artificial intelligence into marketing budget planning represents more than an incremental improvement—it fundamentally transforms the process from periodic resource allocation to continuous optimization. Organizations at the forefront of this evolution have reimagined budgeting as a dynamic system that continuously forecasts performance, assesses risk, and reallocates resources based on emerging opportunities and threats.
As these technologies mature, we can expect several developments:
- Real-time budget optimization across channels and campaigns
- Autonomous marketing systems that adjust tactics within strategic guardrails
- Advanced creative performance prediction to guide content investment
- Competitive response simulation to anticipate market dynamics
While technology enables these capabilities, successful implementation requires organizational transformation—breaking down silos between analytics, finance, and marketing teams while developing new competencies in data science, algorithmic thinking, and scenario planning.
The future belongs not to marketers who create perfect annual plans, but to those who build adaptive systems that continuously reallocate resources to maximize return in ever-changing environments.
Call to Action
To strengthen your organization's predictive budgeting capabilities:
- Conduct an AI readiness assessment focusing on data quality, integration capabilities, and organizational processes
- Develop a phased implementation roadmap that balances immediate wins with long-term capability building
- Create cross-functional alignment by educating stakeholders about the potential and limitations of AI-driven budgeting
- Implement continuous testing processes that compare AI recommendations with traditional approaches
- Establish governance frameworks that balance algorithmic efficiency with strategic guidance
The organizations that thrive in tomorrow's marketing landscape will be those that effectively combine human strategic insight with AI-powered continuous optimization, creating marketing budget systems that learn, adapt, and improve over time.
Featured Blogs
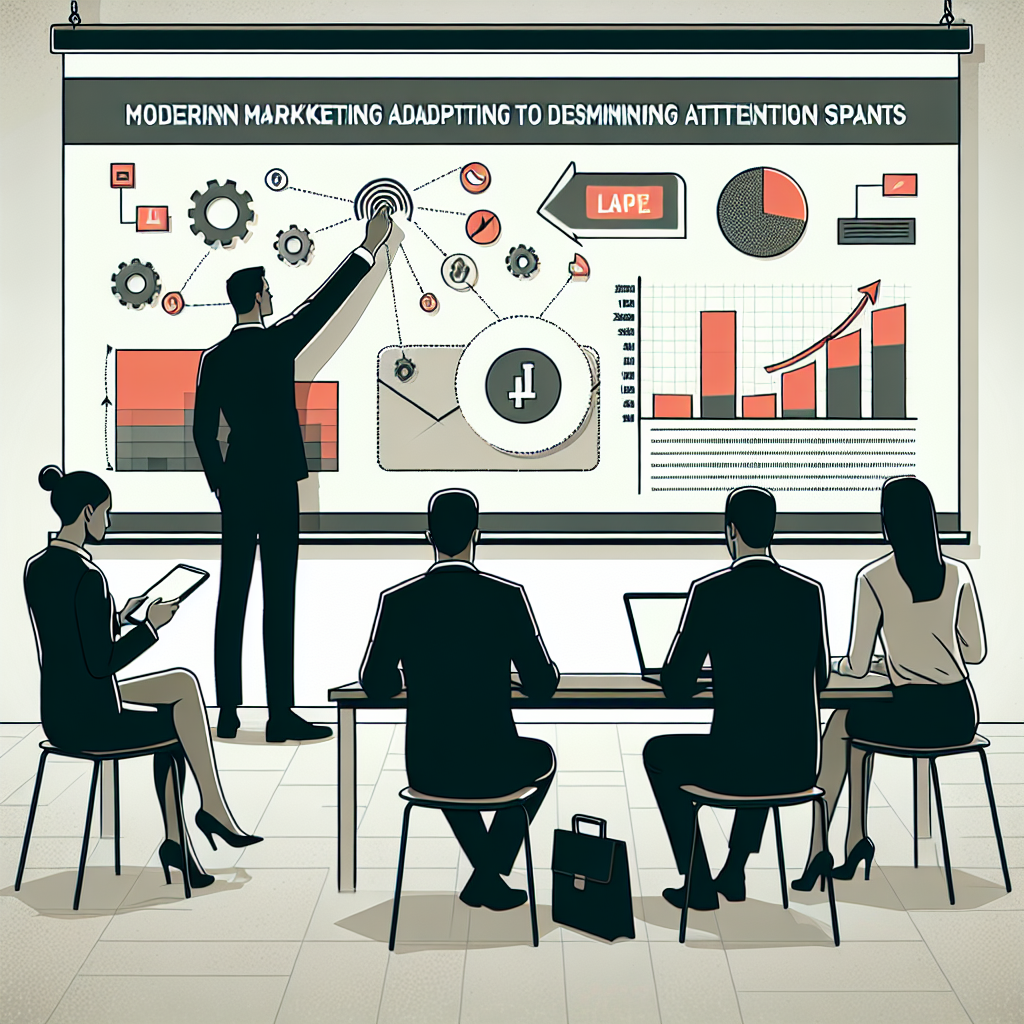
How the Attention Recession Is Changing Marketing
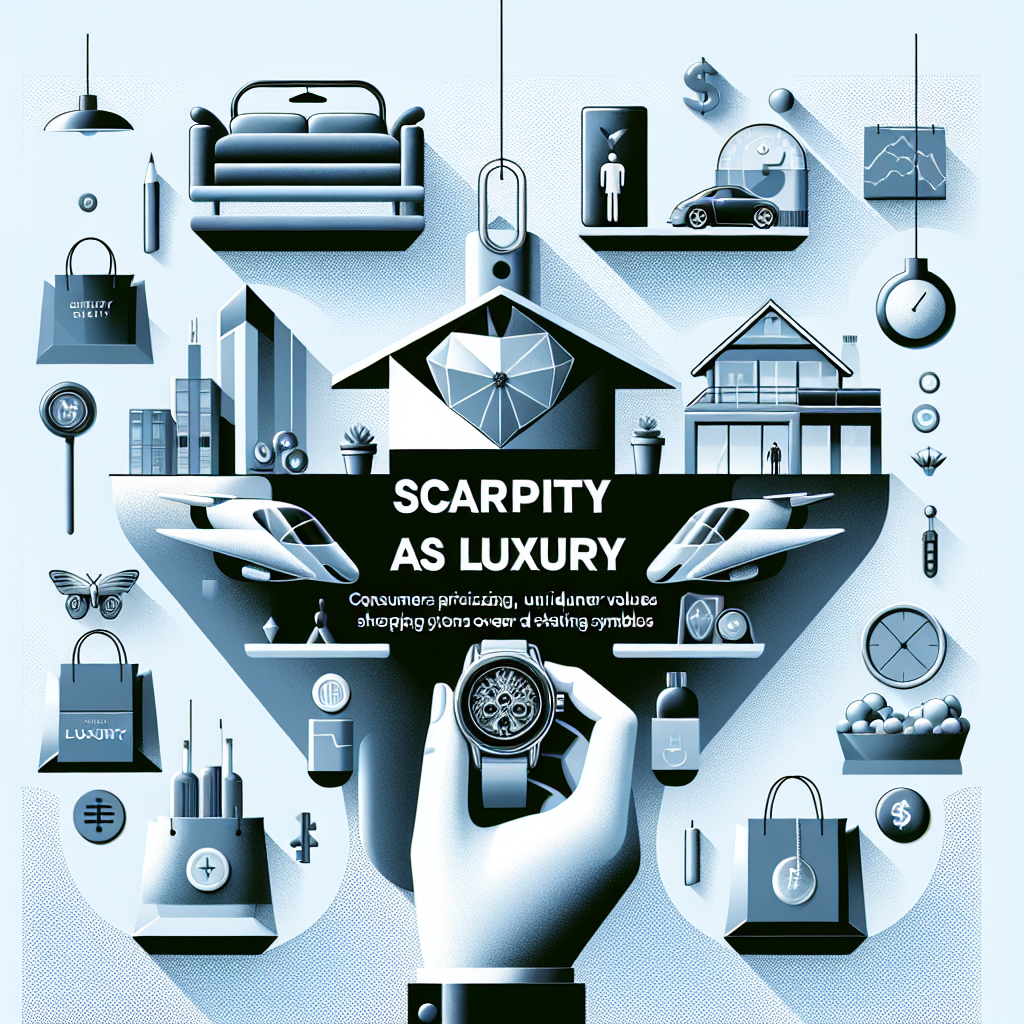
The New Luxury Why Consumers Now Value Scarcity Over Status
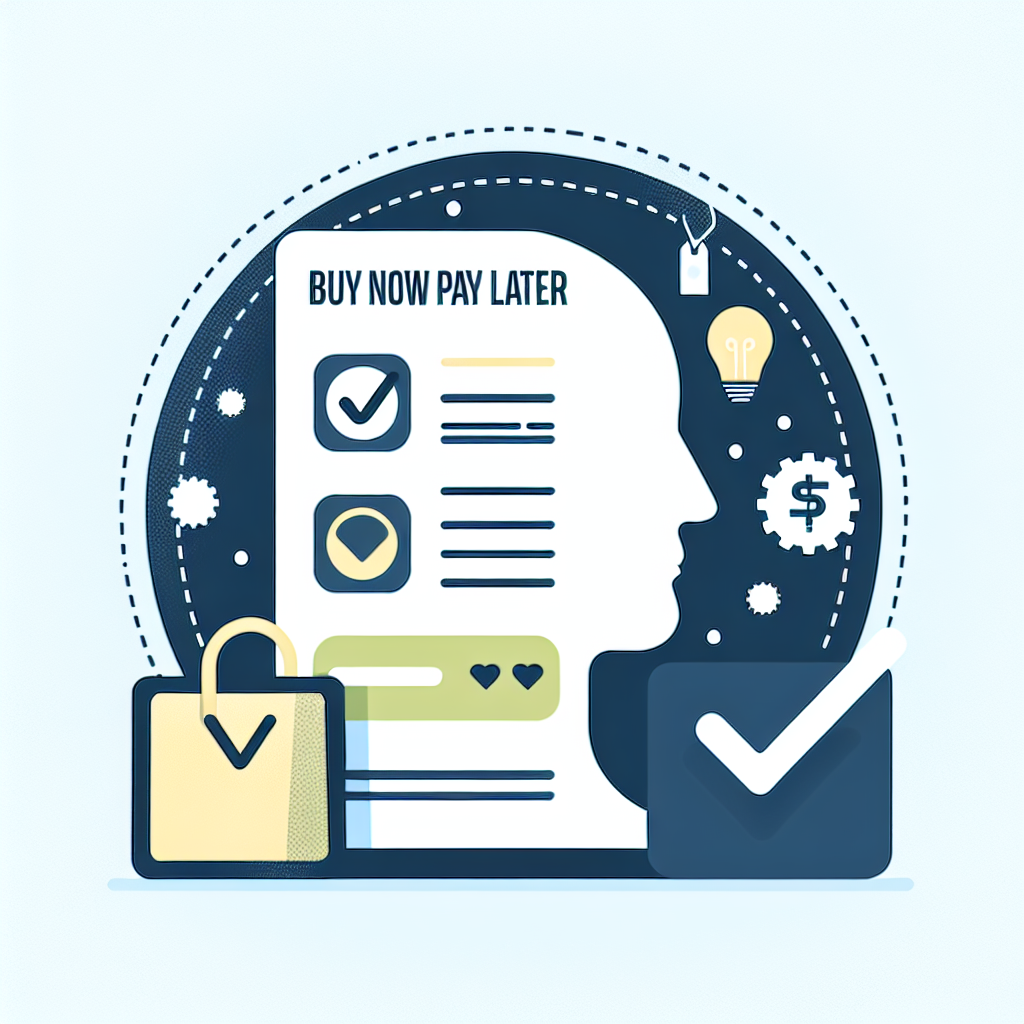
The Psychology Behind Buy Now Pay later
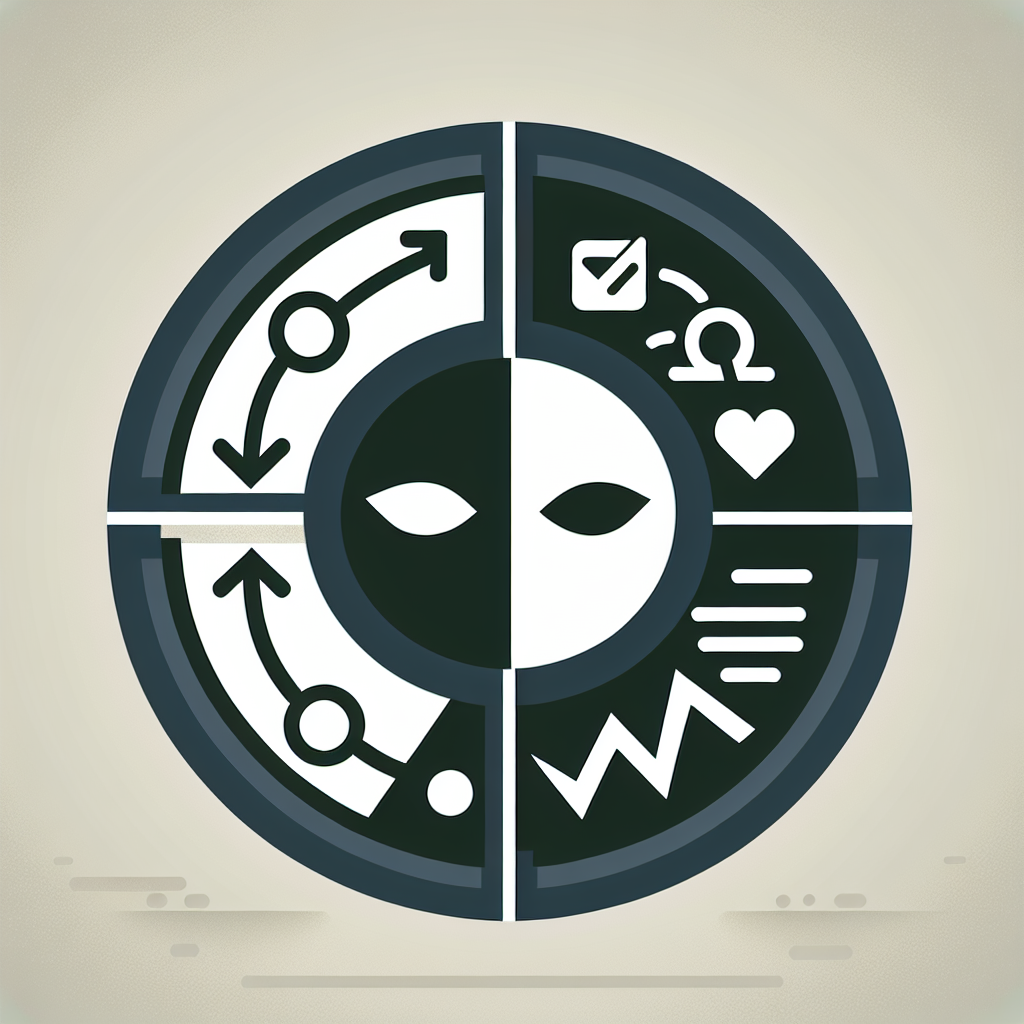
The Role of Dark Patterns in Digital Marketing and Ethical Concerns
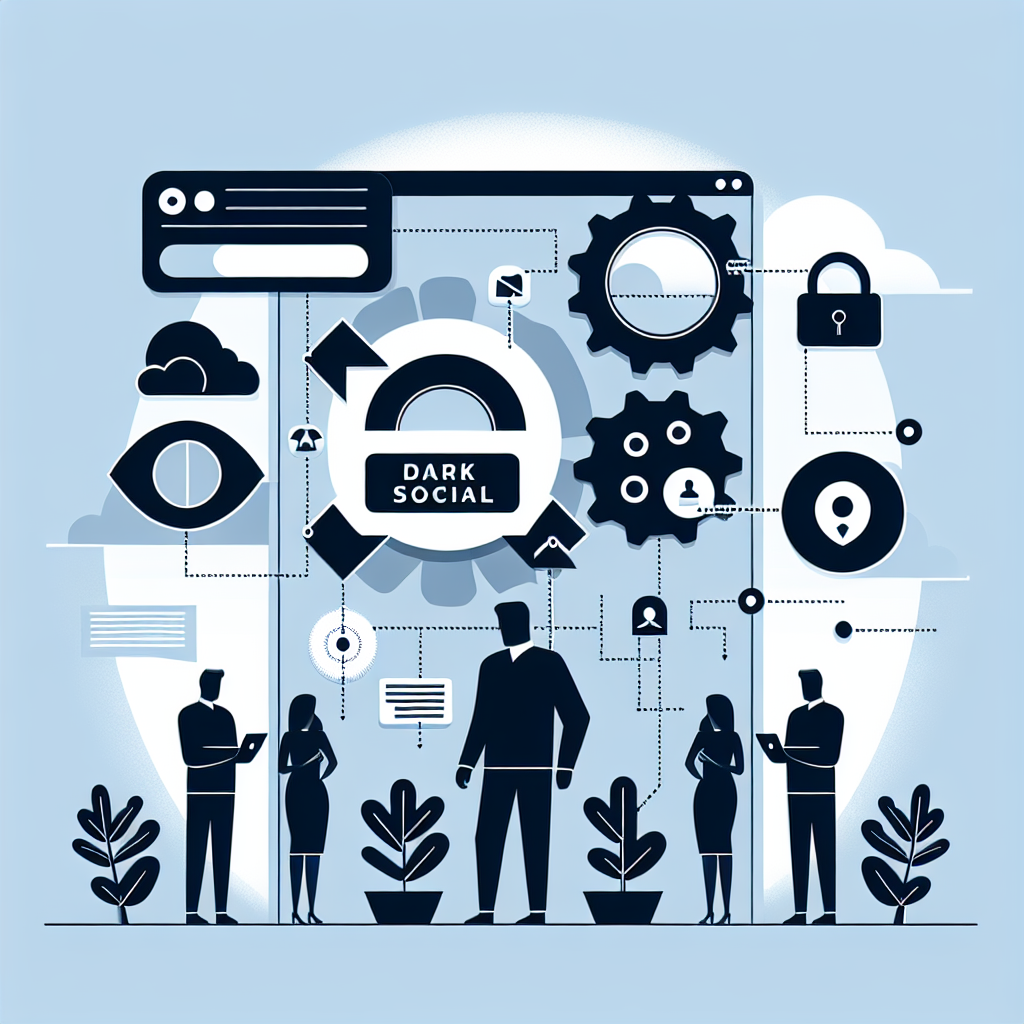
The Rise of Dark Social and Its Impact on Marketing Measurement
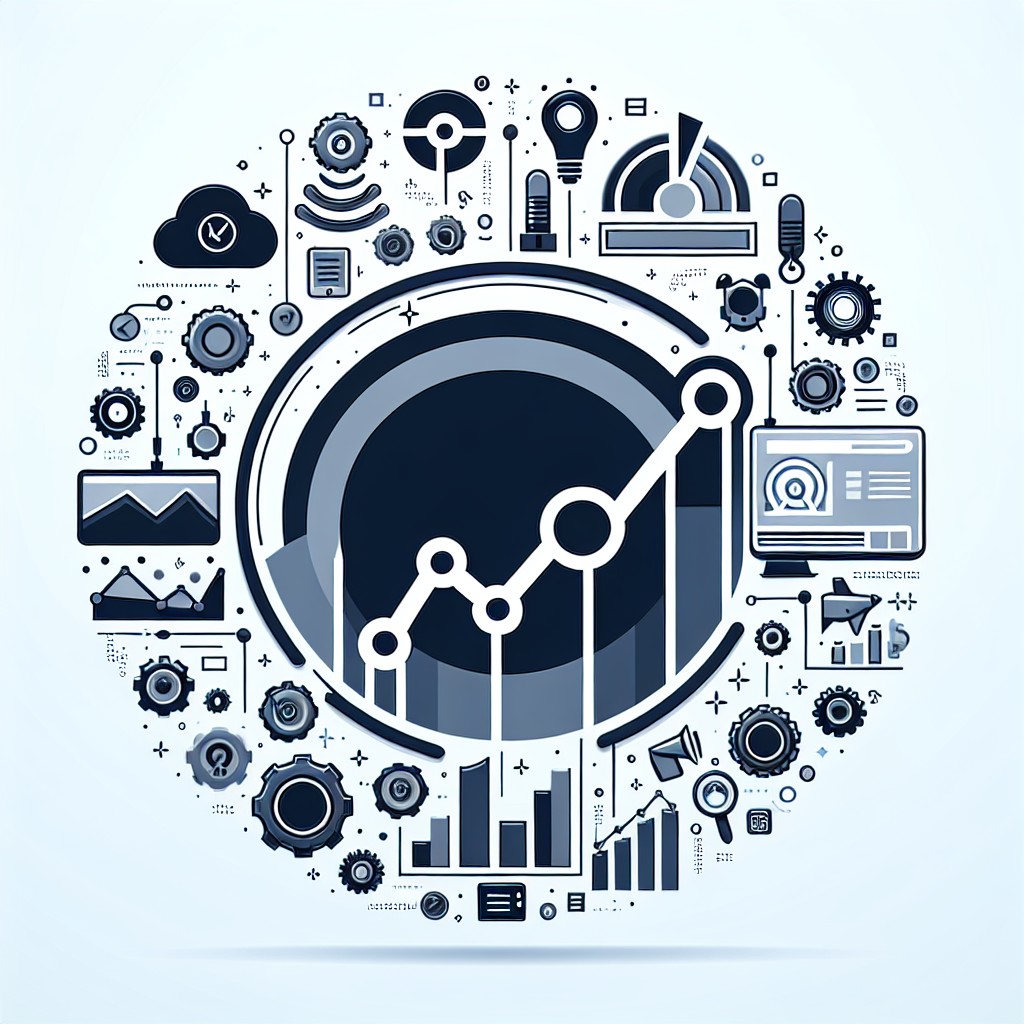