Predictive Analytics in Marketing
1. Introduction: The Shift Toward Data-Driven Marketing
Marketing has always relied on understanding consumer behavior, but traditional methods—historical data analysis, intuition-based decision-making, and broad demographic segmentation—are no longer sufficient in an era of hyper-personalization and real-time decision-making. Predictive analytics, powered by machine learning and artificial intelligence (AI), is transforming marketing by forecasting consumer behavior with unprecedented accuracy.
By analyzing past interactions, real-time signals, and external data sources, predictive models enable businesses to anticipate customer needs, optimize marketing spend, and enhance engagement. Companies that integrate predictive analytics into their marketing strategies achieve higher customer retention, improved conversion rates, and greater ROI. This article explores how predictive analytics is reshaping marketing, its core applications, challenges, and the future of AI-driven consumer forecasting.
2. How Predictive Analytics Works: The Science Behind Forecasting Consumer Behavior
At its core, predictive analytics leverages statistical models and AI to identify patterns in consumer data and predict future actions. The process follows a structured approach:
a) Data Collection: The Foundation of Prediction
Predictive analytics relies on diverse data sources, including:
- First-party data: Customer purchase history, website/app interactions, CRM data.
- Third-party data: External market trends, social sentiment, competitive intelligence.
- Real-time signals: Clickstream data, IoT interactions, customer feedback.
b) Machine Learning Algorithms: Transforming Data into Insights
Predictive models range from traditional statistical techniques to advanced AI-driven approaches:
- Regression models: Identify relationships between variables (e.g., income and purchase likelihood).
- Decision trees & clustering: Segment customers based on behaviors and attributes.
- Neural networks & deep learning: Analyze complex, non-linear relationships for more accurate predictions.
- Bayesian models: Continuously update predictions based on new data.
c) Model Training and Optimization
Predictive algorithms are trained on historical data and continuously refined through feedback loops, ensuring they remain adaptive to changing consumer behaviors. Companies that successfully integrate these models into their marketing strategy gain a competitive advantage through:
- More precise audience targeting.
- Real-time personalization at scale.
- Optimized media and campaign performance.
3. Key Applications of Predictive Analytics in Marketing
Predictive analytics is revolutionizing marketing across multiple domains:
a) Customer Segmentation & Personalization
Traditional segmentation is based on static attributes like age and income. Predictive analytics, however, segments users dynamically based on behavioral patterns, enabling hyper-personalized engagement.
Example: Netflix leverages predictive modeling to personalize recommendations, increasing user retention. Amazon’s predictive engines drive product recommendations, contributing to 35% of its revenue.
b) Demand Forecasting & Inventory Optimization
By predicting future demand, companies optimize stock levels, reducing waste and lost sales.
Example: Zara uses real-time sales data and AI-driven demand forecasting to adjust production and distribution, minimizing excess inventory while ensuring product availability.
c) Churn Prediction & Retention Strategies
Predictive analytics identifies at-risk customers before they churn, enabling targeted retention efforts.
Example: Spotify analyzes listening behavior to predict potential churn and proactively offers personalized incentives to retain users.
d) Dynamic Pricing & Real-Time Bidding
AI-driven pricing models adjust prices based on demand elasticity, competition, and consumer behavior.
Example: Uber’s surge pricing and Amazon’s dynamic pricing strategies optimize revenue while maintaining competitiveness.
e) Marketing Spend Optimization
Predictive analytics optimizes budget allocation across channels, ensuring maximum ROI.
Example: Google’s Smart Bidding automatically adjusts ad bids in real-time, maximizing conversions.
4. The Business Impact: Quantifying the Value of Predictive Analytics
The adoption of predictive analytics yields measurable business outcomes:
- 5-15% revenue uplift through improved targeting and conversion optimization.
- 10-30% reduction in marketing costs by eliminating inefficient spend.
- Higher customer lifetime value (CLV) through better retention strategies.
Case Study: A Global Consumer Brand
A leading FMCG company integrated predictive analytics to optimize media spend across digital channels. By shifting budgets dynamically based on AI-driven insights, they achieved:
- 18% increase in conversion rates.
- 12% reduction in cost per acquisition (CPA).
- Improved cross-channel attribution, enhancing marketing efficiency.
For enterprises, the question is no longer whether to adopt predictive analytics but how to scale it effectively across marketing functions.
5. Challenges in Implementing Predictive Analytics
Despite its potential, predictive analytics comes with challenges:
a) Data Quality & Integration
- Incomplete, outdated, or biased data can lead to inaccurate predictions.
- Legacy IT systems may struggle with integrating real-time predictive capabilities.
b) Privacy & Compliance
- With GDPR, CCPA, and evolving data privacy regulations, companies must balance personalization with ethical data usage.
- First-party data strategies (e.g., zero-party data collection) are becoming critical in a post-cookie world.
c) Explainability vs. Black-Box AI
- Many AI-driven models operate as “black boxes,” making it difficult for marketers to interpret predictions and take action.
- The need for transparent AI and interpretable models is increasing.
d) Organizational Alignment
- Predictive analytics requires collaboration across marketing, data science, and IT teams.
- Resistance to change and lack of AI expertise can slow adoption.
Companies that address these challenges proactively can maximize the value of predictive analytics while minimizing risks.
6. The Future of Predictive Analytics in Marketing
Predictive analytics is evolving beyond static forecasting into real-time, AI-driven decision-making:
a) The Rise of Autonomous Marketing AI
- AI will not only predict but autonomously execute marketing decisions (e.g., automated ad optimization, real-time content customization).
b) Generative AI & Predictive Insights
- AI will generate content dynamically based on predictive models, personalizing messaging in real-time.
c) Zero-Party Data & Predictive Social Listening
- Brands will rely on direct consumer interactions (polls, surveys, engagement signals) to refine predictions.
- Social media sentiment analysis will be integrated into real-time predictive marketing models.
d) AI-Driven Customer Journey Orchestration
- Predictive analytics will power seamless, real-time customer journeys, automatically adjusting messaging and offers at each touchpoint.
Businesses that invest in these emerging capabilities will redefine competitive advantage in marketing.
7. Conclusion: Turning Prediction into Action
Predictive analytics is no longer a futuristic concept—it is a critical enabler of modern marketing. Companies that leverage AI-driven forecasting gain a strategic edge through:
- Enhanced customer insights and personalization.
- Optimized marketing spend and higher ROI.
- Proactive churn reduction and increased retention.
- Real-time, automated decision-making for marketing execution.
However, successful implementation requires more than just technology—it demands a data-driven culture, cross-functional collaboration, and an agile approach to marketing transformation. The future belongs to organizations that can not only predict consumer behavior but also translate those insights into dynamic, automated, and highly personalized marketing strategies.
Call to Action
For marketing leaders looking to scale predictive analytics, the focus should be on:
- Investing in AI-driven marketing platforms.
- Building strong data infrastructure and governance.
- Creating cross-functional teams that bridge marketing and data science.
The organizations that execute on these priorities will not just forecast the future—they will shape it.
Featured Blogs
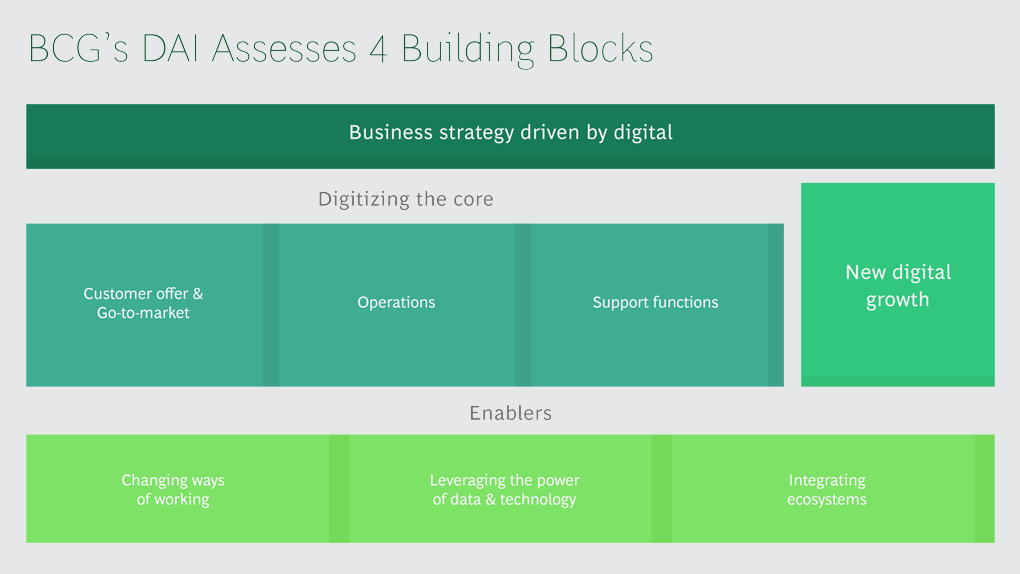
BCG Digital Acceleration Index
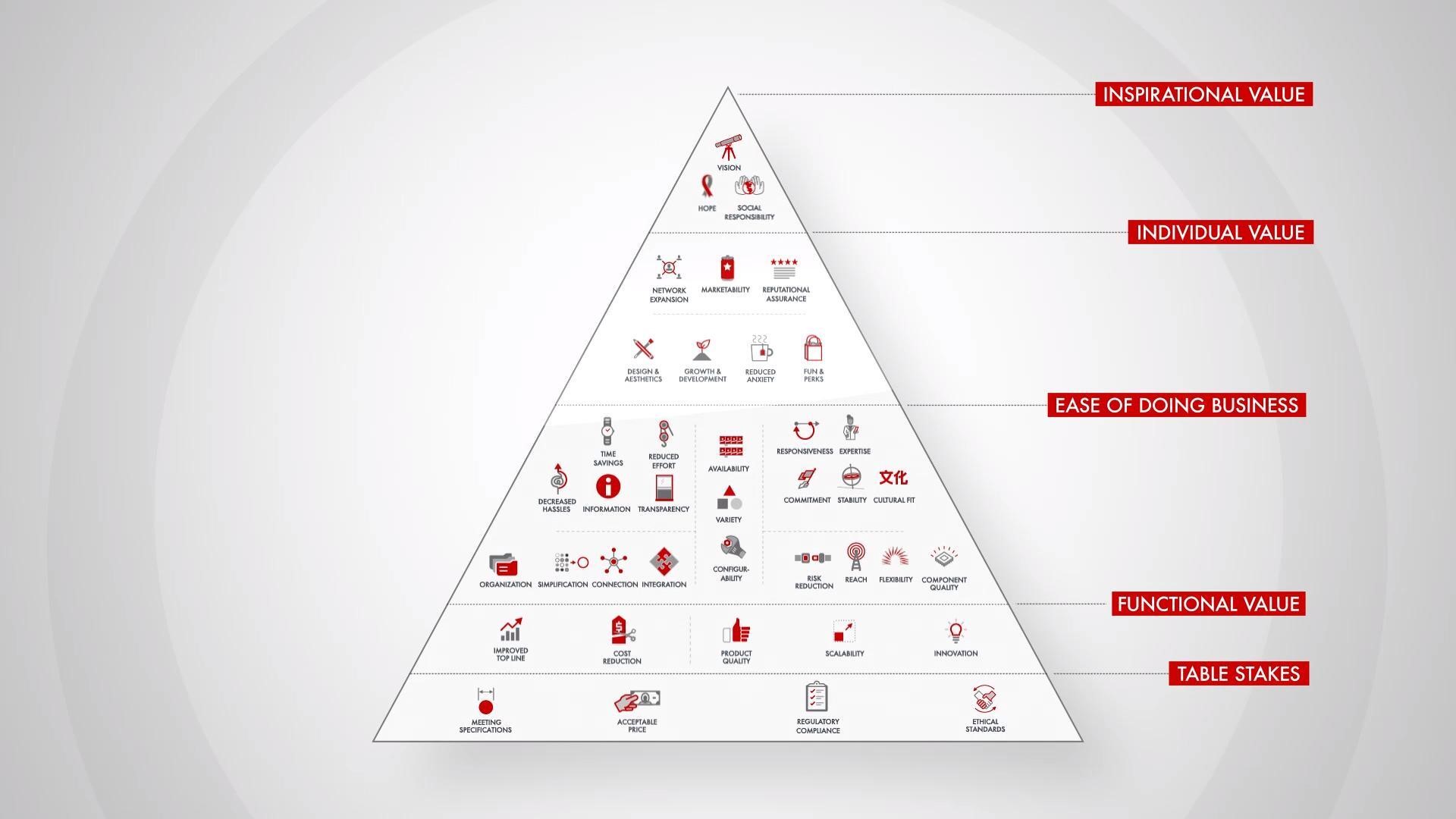
Bain’s Elements of Value Framework
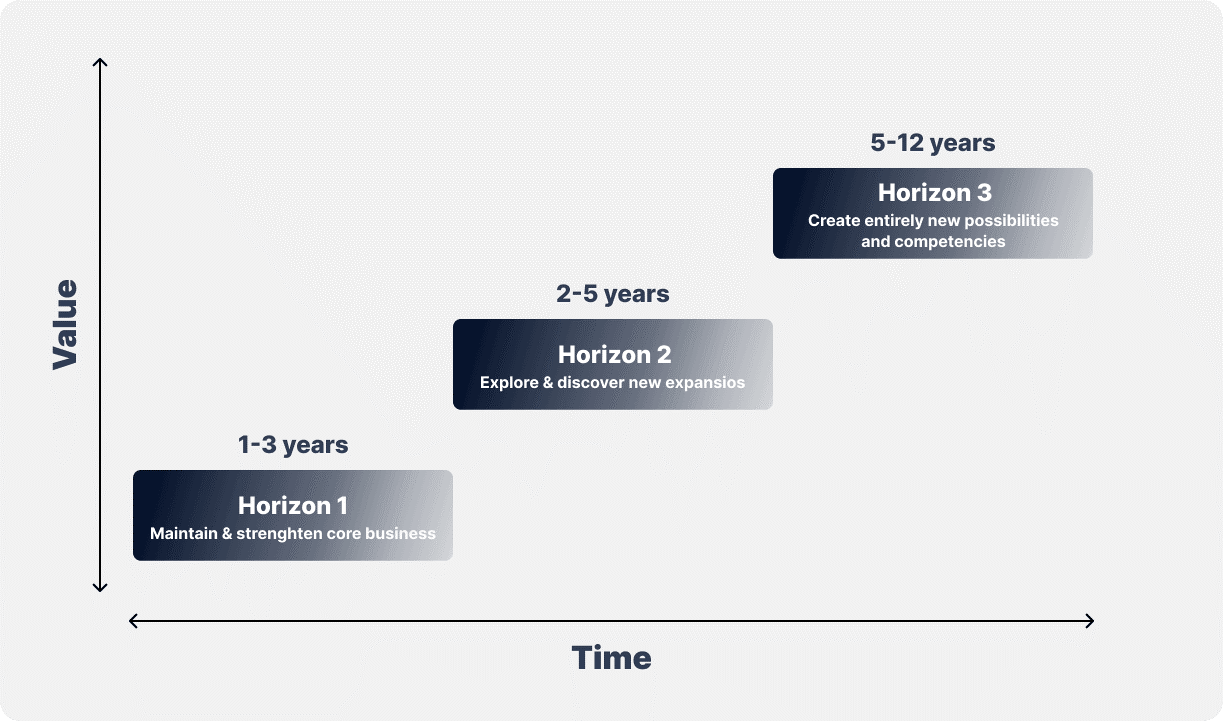
McKinsey Growth Pyramid
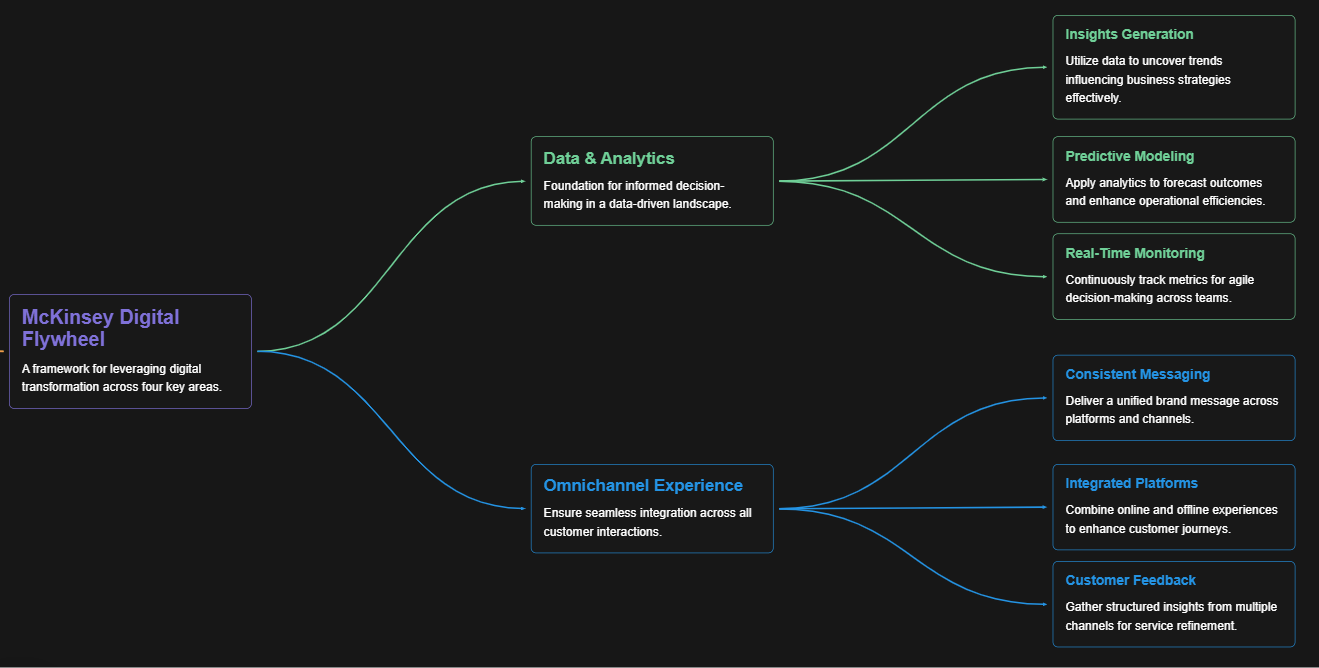
McKinsey Digital Flywheel
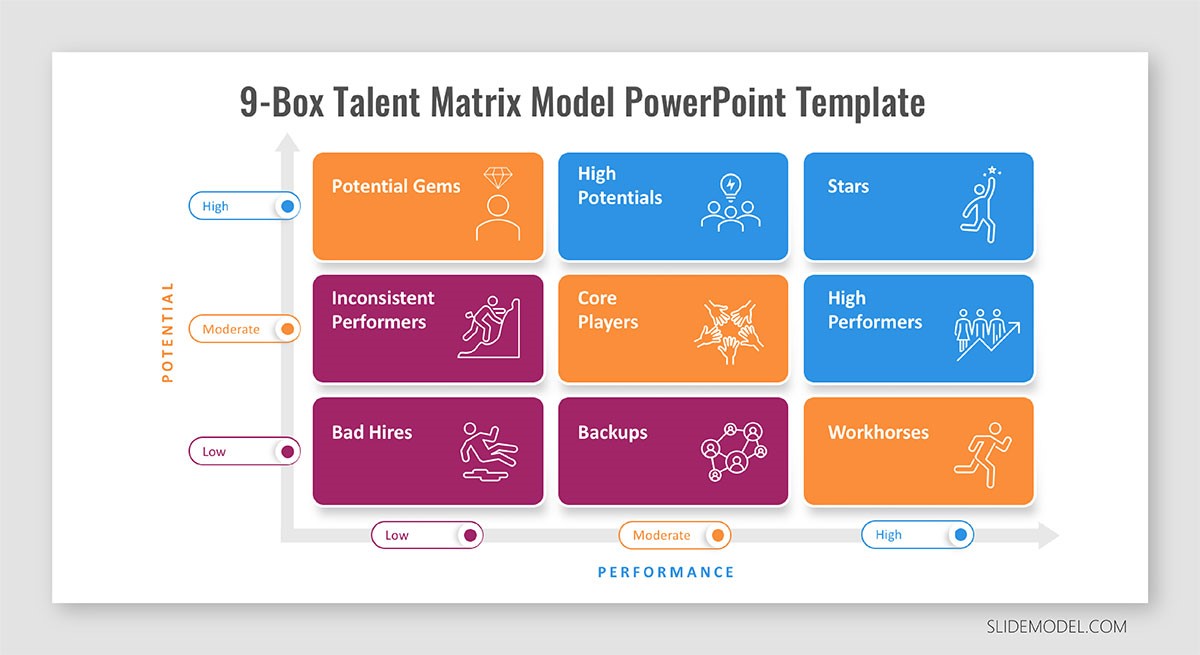
McKinsey 9-Box Talent Matrix
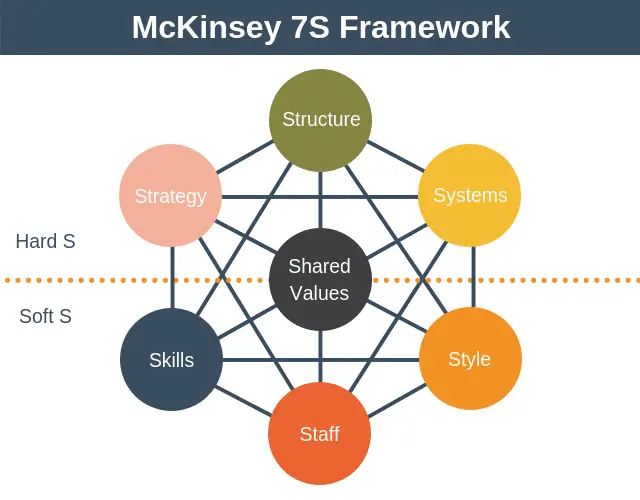
McKinsey 7S Framework
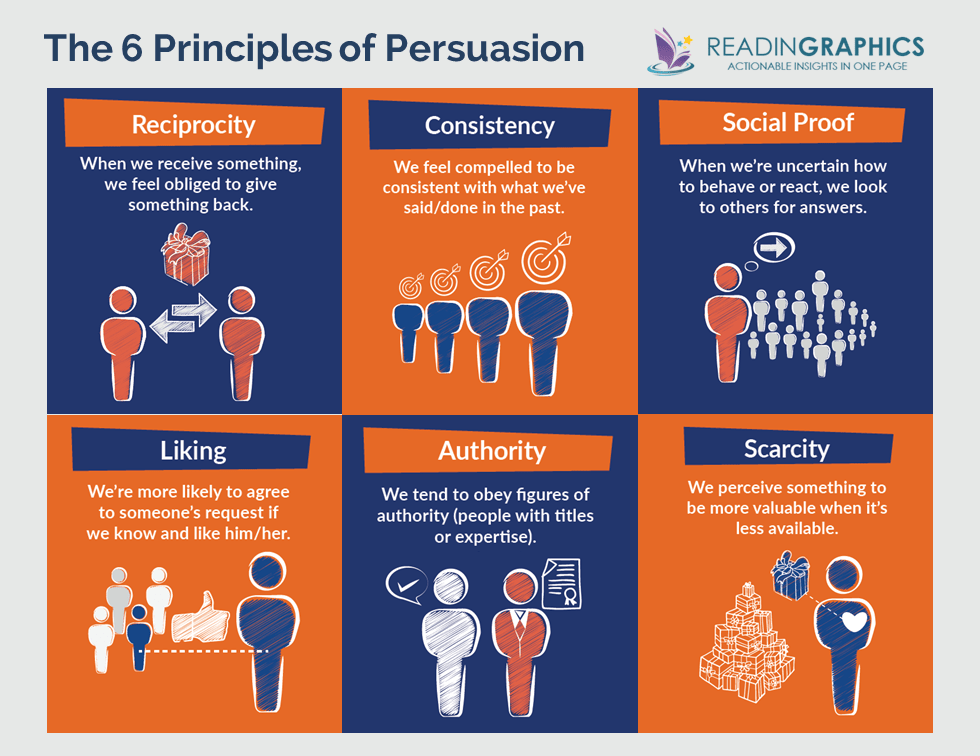
The Psychology of Persuasion in Marketing
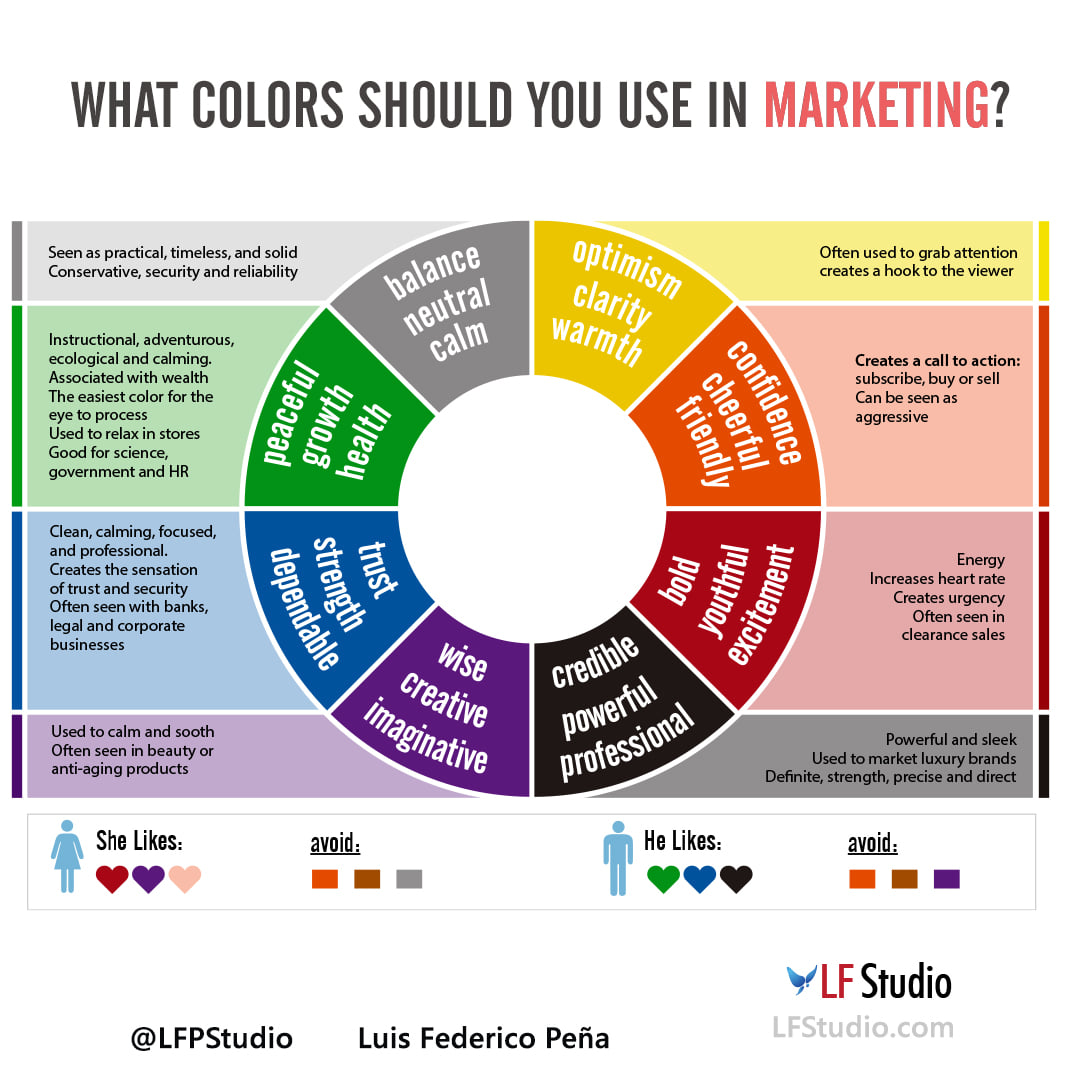
The Influence of Colors on Branding and Marketing Psychology
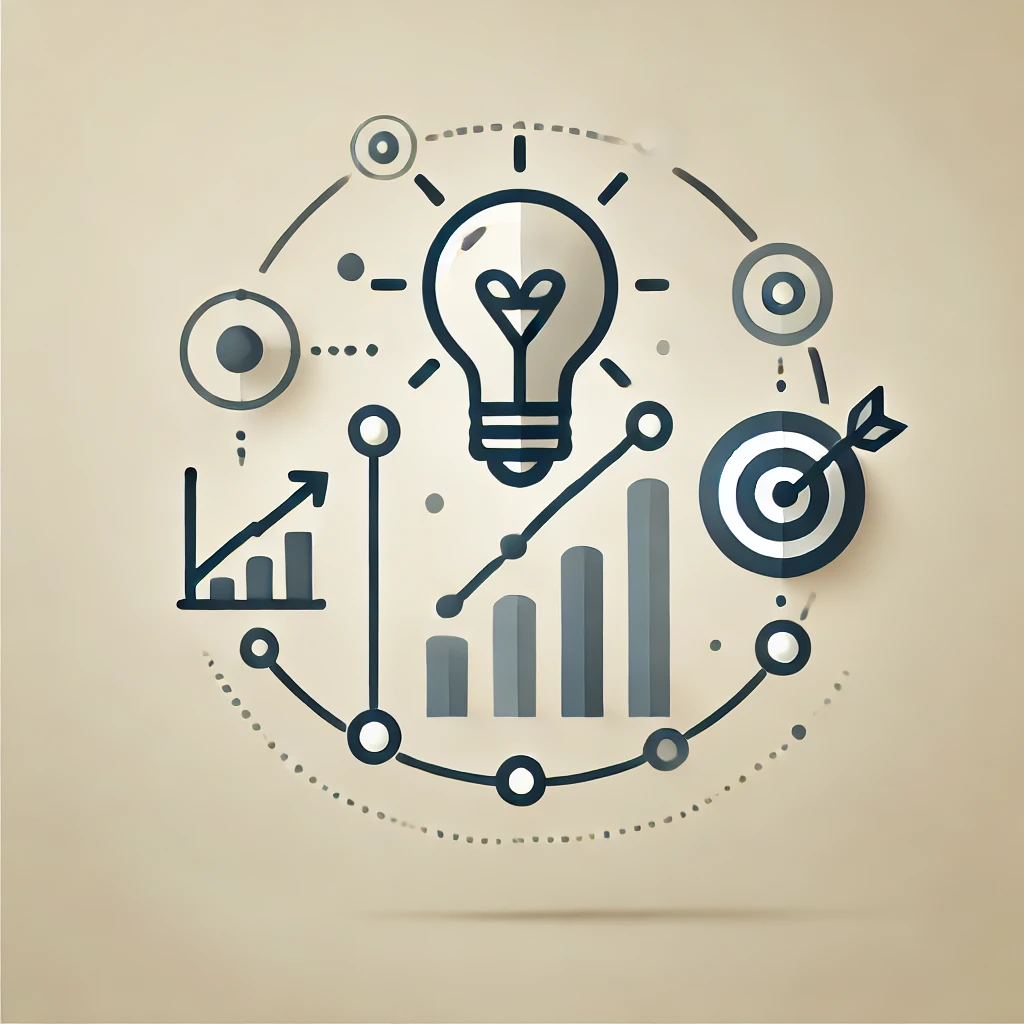