A/B Testing: Optimizing Marketing Campaigns
1. Introduction: The Evolution of Data-Driven Decision Making in Marketing
In today's hyper-competitive digital landscape, intuition-based marketing decisions have given way to empirical, evidence-driven strategies. At the forefront of this transformation stands A/B testing—a methodical approach to optimizing marketing campaigns through controlled experimentation. By systematically comparing two or more variants of a marketing element, organizations can determine which version drives superior performance across key metrics like conversion rates, engagement, and ROI.
The digital transformation has elevated A/B testing from a periodic exercise to an ongoing, integral component of marketing operations. According to a study by Conversion XL, companies that implement regular A/B testing see an average 223% ROI on their testing programs. As marketing professor Scott Brinker notes, "In a world of infinite possibilities but finite resources, A/B testing provides the empirical compass to navigate marketing decisions." This article explores the strategic implementation of A/B testing, its applications across marketing channels, methodological best practices, and how emerging technologies are reshaping experimental marketing in the AI era.
2. The Science of A/B Testing: Methodology and Statistical Foundations
A/B testing operates on sound statistical principles that transform subjective marketing decisions into objective, data-backed strategies:
a) Hypothesis Development: The Foundation of Effective Testing
Every meaningful A/B test begins with a clear hypothesis based on:
- Customer behavior analysis and friction points in the conversion funnel
- User feedback and qualitative insights
- Competitive analysis and industry benchmarks
- Previous test results and iterative learning
b) Test Design and Implementation
The testing architecture incorporates several critical elements:
- Random audience segmentation to ensure statistical validity
- Sample size calculation to achieve statistical significance
- Control variables to isolate the impact of the tested element
- Multivariate testing when examining multiple variables simultaneously
c) Data Collection and Analysis
Modern A/B testing platforms offer sophisticated analytical capabilities:
- Real-time data collection and performance monitoring
- Bayesian vs. frequentist statistical approaches
- Segmentation analysis to identify performance variations across user cohorts
- Confidence intervals and p-values to validate results
According to research by Harvard Business School professor Stefan Thomke, organizations that adopt rigorous A/B testing methodologies achieve 5-25% improvements in conversion rates compared to those relying on intuition-based decision-making.
3. Strategic Applications Across Marketing Channels
A/B testing has evolved beyond basic website optimization to encompass the entire marketing ecosystem:
a) Email Marketing Optimization
Email remains a high-ROI channel where incremental improvements drive significant value:
- Subject line testing can increase open rates by 10-15% (Campaign Monitor)
- Call-to-action variations can lift click-through rates by 25-45% (Mailchimp)
Example: Booking.com runs over 25,000 email tests annually, resulting in personalized messaging that has improved conversion rates by 30% and contributed to their market leadership position.
b) Landing Page and Website Conversion Optimization
The digital storefront represents a critical conversion point:
- Headline and value proposition testing
- Form field optimization and friction reduction
- Social proof placement and visual hierarchy testing
Example: Shopify implemented systematic A/B testing across their trial signup flow, resulting in a 20% increase in merchant acquisition—translating to millions in additional annual revenue.
c) Paid Media and Ad Creative Optimization
With rising acquisition costs, optimizing ad performance is essential:
- Ad copy and creative element testing
- Audience targeting and segmentation experiments
- Bid strategy and budget allocation optimization
Example: Dollar Shave Club attributes their rapid growth to rigorous A/B testing of video ad creative, identifying messaging that resonated with their target demographic and reducing customer acquisition costs by 23%.
4. Implementation Challenges and Best Practices
Despite its potential, effective A/B testing faces several implementation hurdles:
a) Organizational and Cultural Barriers
- Moving from intuition to evidence-based decision making requires cultural transformation
- Testing programs often face resource constraints and organizational resistance
- Cross-functional alignment between marketing, analytics, and technology teams is essential
b) Methodological Pitfalls
- Sampling bias and premature test conclusion
- Multiple testing problems leading to false positives
- Ignoring external validity when generalizing results
c) Best Practices for Sustainable Testing Programs
- Implementing a test-and-learn culture with executive sponsorship
- Prioritizing tests based on potential impact and implementation effort
- Documenting and democratizing learnings across the organization
- Maintaining a balanced portfolio of incremental and disruptive tests
According to McKinsey, companies with mature testing programs achieve 3-8x higher ROI on their marketing initiatives compared to less sophisticated competitors.
5. The Future of A/B Testing: AI-Driven Experimentation
The next frontier of A/B testing is being shaped by artificial intelligence and machine learning:
a) Automated Experimentation
- AI-powered testing platforms that autonomously generate hypotheses
- Continuous multivariate testing with dynamic traffic allocation
- Reinforcement learning algorithms that optimize in real-time
b) Predictive Analytics and Simulation
- Predictive models that forecast test outcomes before full implementation
- Digital twins and simulation environments for risk-free experimentation
- Automated personalization based on individual user behavior models
Example: Spotify's recommendation system conducts thousands of simultaneous experiments using multi-armed bandit algorithms, continuously optimizing user engagement without waiting for traditional A/B test completion.
6. Conclusion: From Testing to Transformation
A/B testing has evolved from a tactical website optimization technique to a strategic capability that drives competitive advantage. Organizations that embed systematic experimentation into their marketing DNA gain:
- Data-driven decision-making capabilities that reduce marketing waste
- Deeper customer insights that inform broader marketing strategy
- Continuous improvement cycles that compound over time
- An empirical foundation for marketing innovation
However, the true power of A/B testing lies not in isolated experiments but in building a comprehensive experimentation ecosystem where testing becomes the default approach to marketing decisions. As marketing technologist Avinash Kaushik states, "The goal is not to be good at testing; the goal is to be good at learning through testing."
Call to Action
For marketing leaders looking to elevate their A/B testing capabilities:
- Audit your current testing maturity and develop a roadmap for advancement
- Invest in both technology infrastructure and team capabilities
- Establish clear KPIs and governance for your testing program
- Create cross-functional testing teams that bridge marketing creativity with analytical rigor
The organizations that master the science of experimentation will not merely optimize campaigns—they will transform marketing from an expense into a precision instrument for sustainable growth.
Featured Blogs
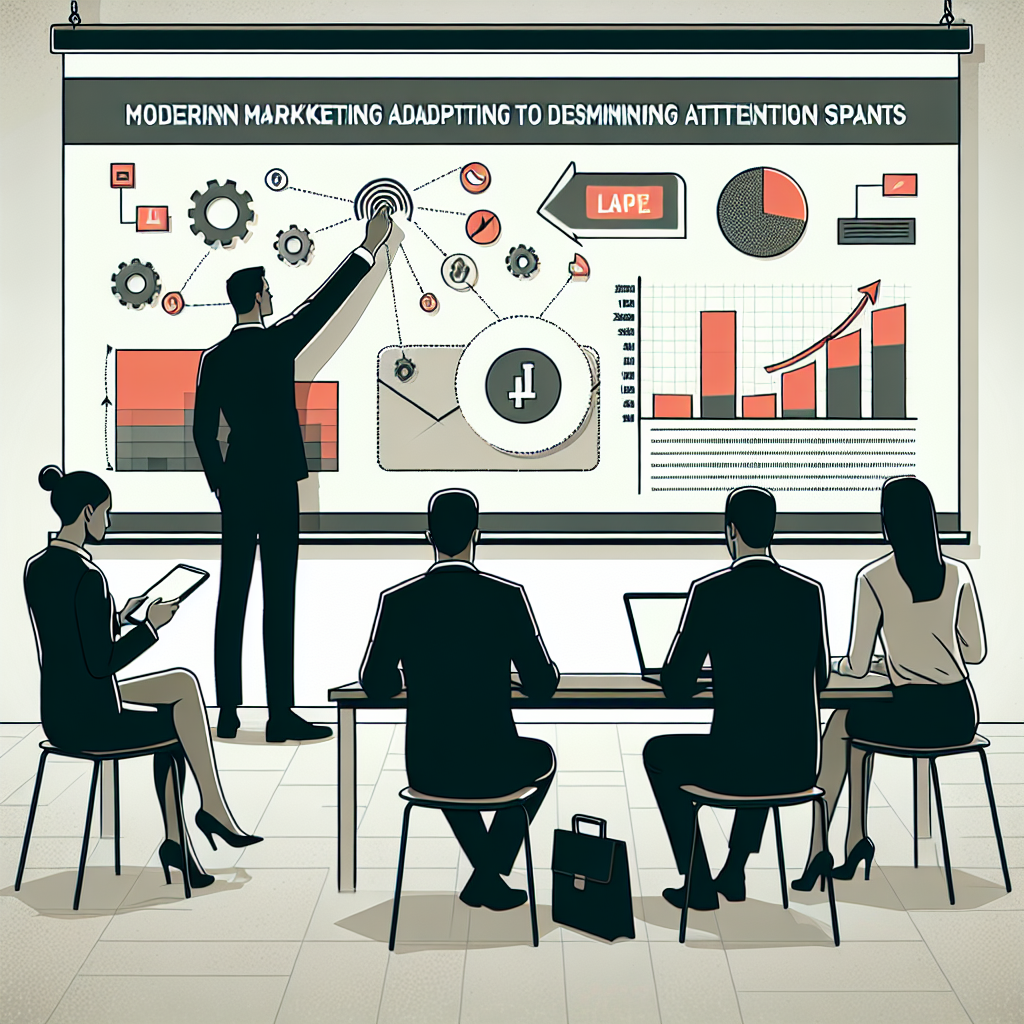
How the Attention Recession Is Changing Marketing
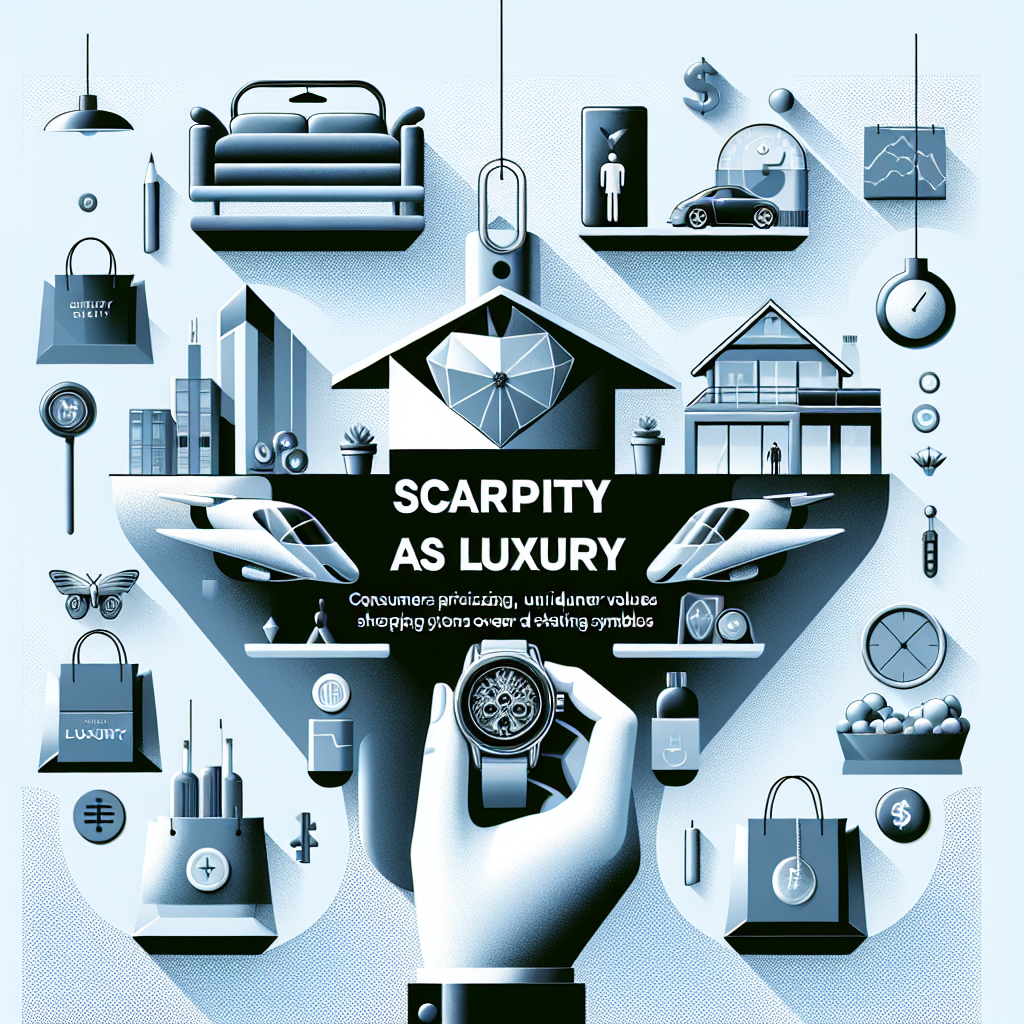
The New Luxury Why Consumers Now Value Scarcity Over Status
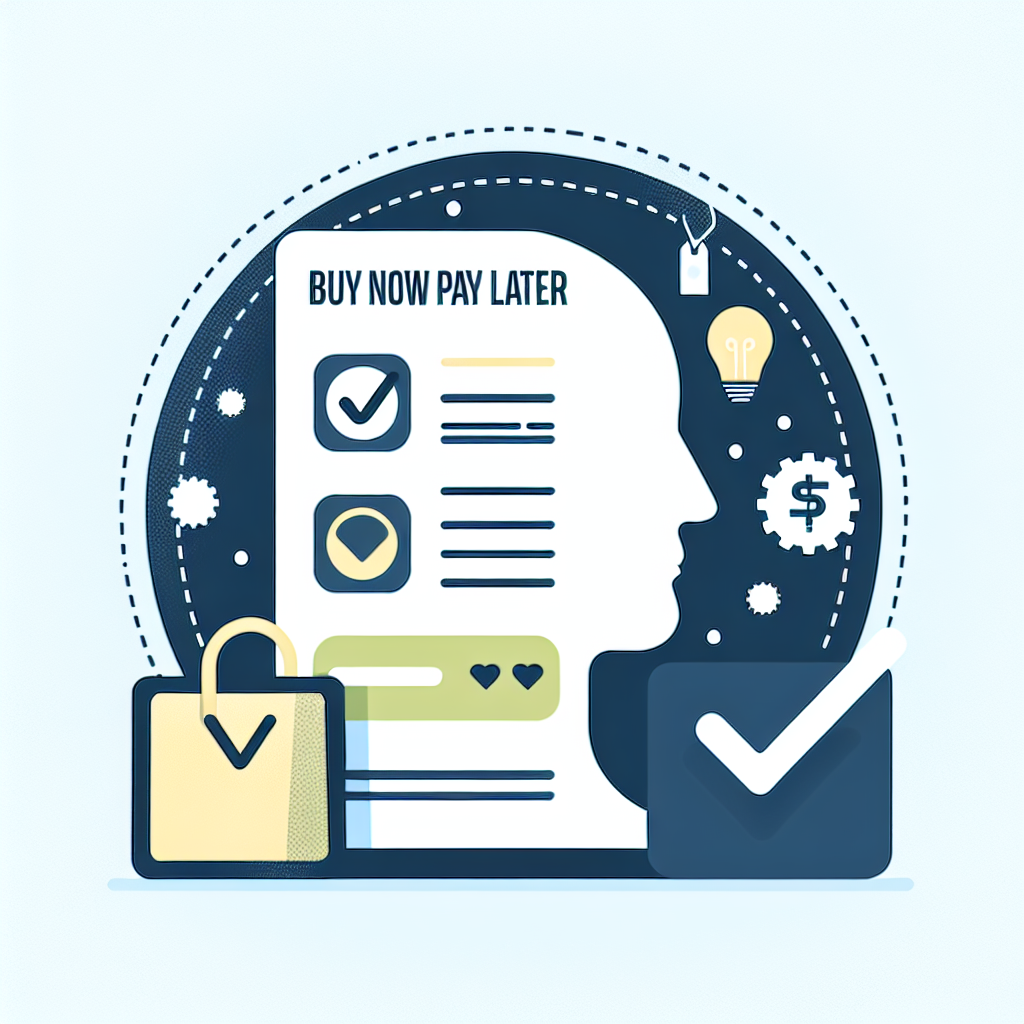
The Psychology Behind Buy Now Pay later
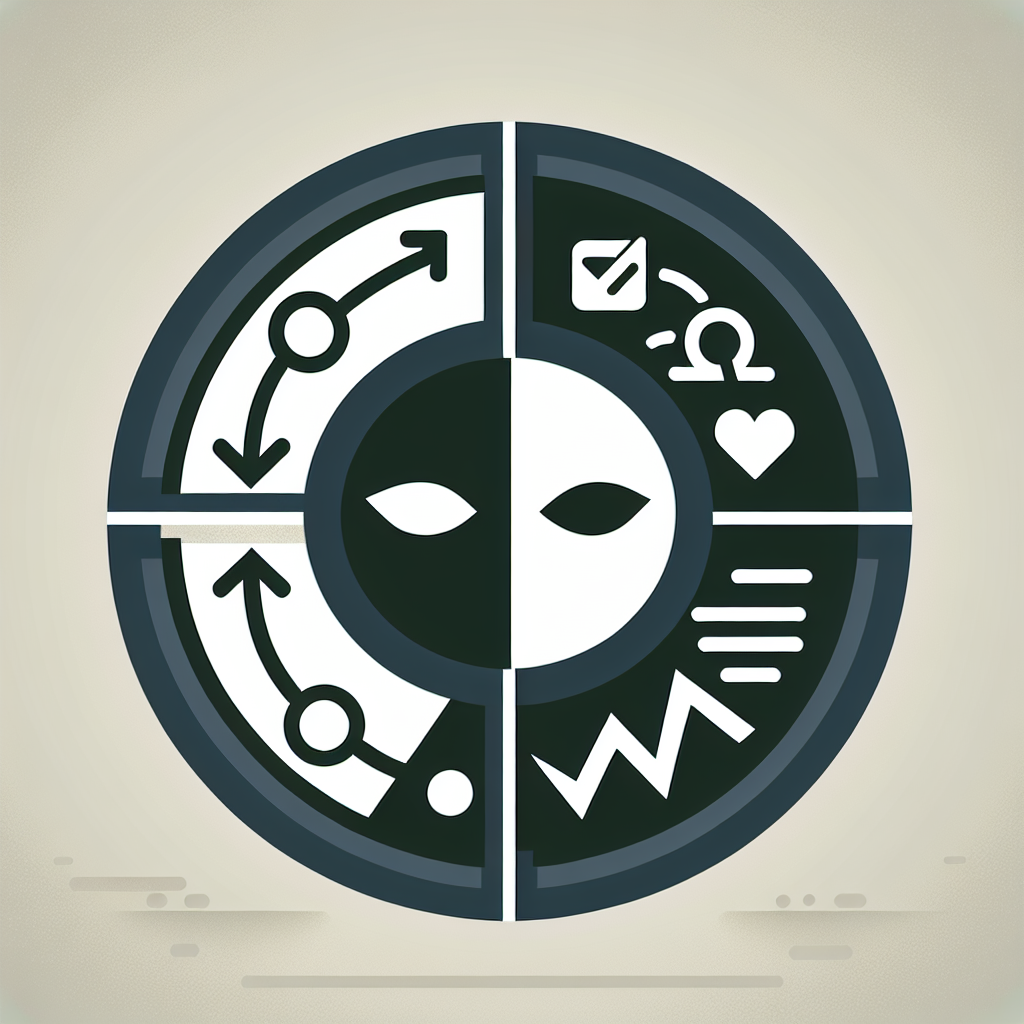
The Role of Dark Patterns in Digital Marketing and Ethical Concerns
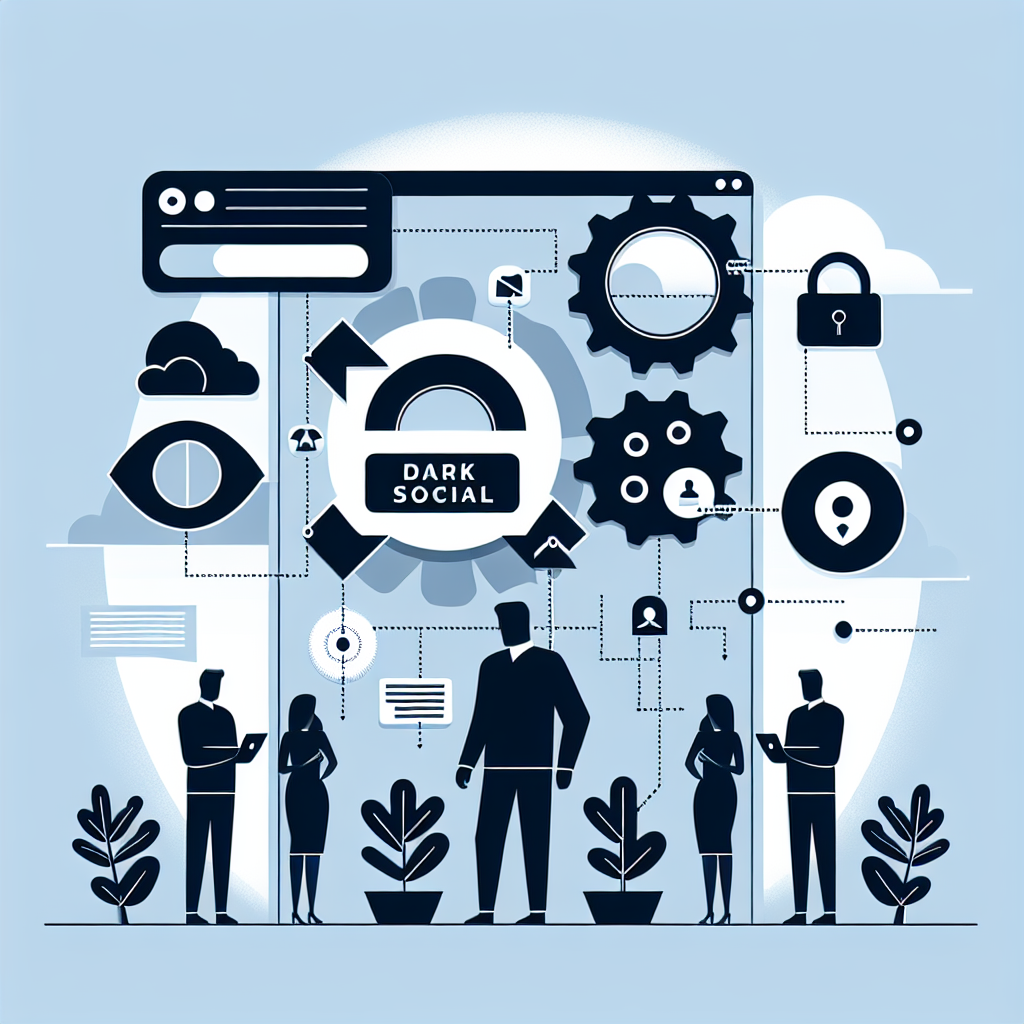
The Rise of Dark Social and Its Impact on Marketing Measurement
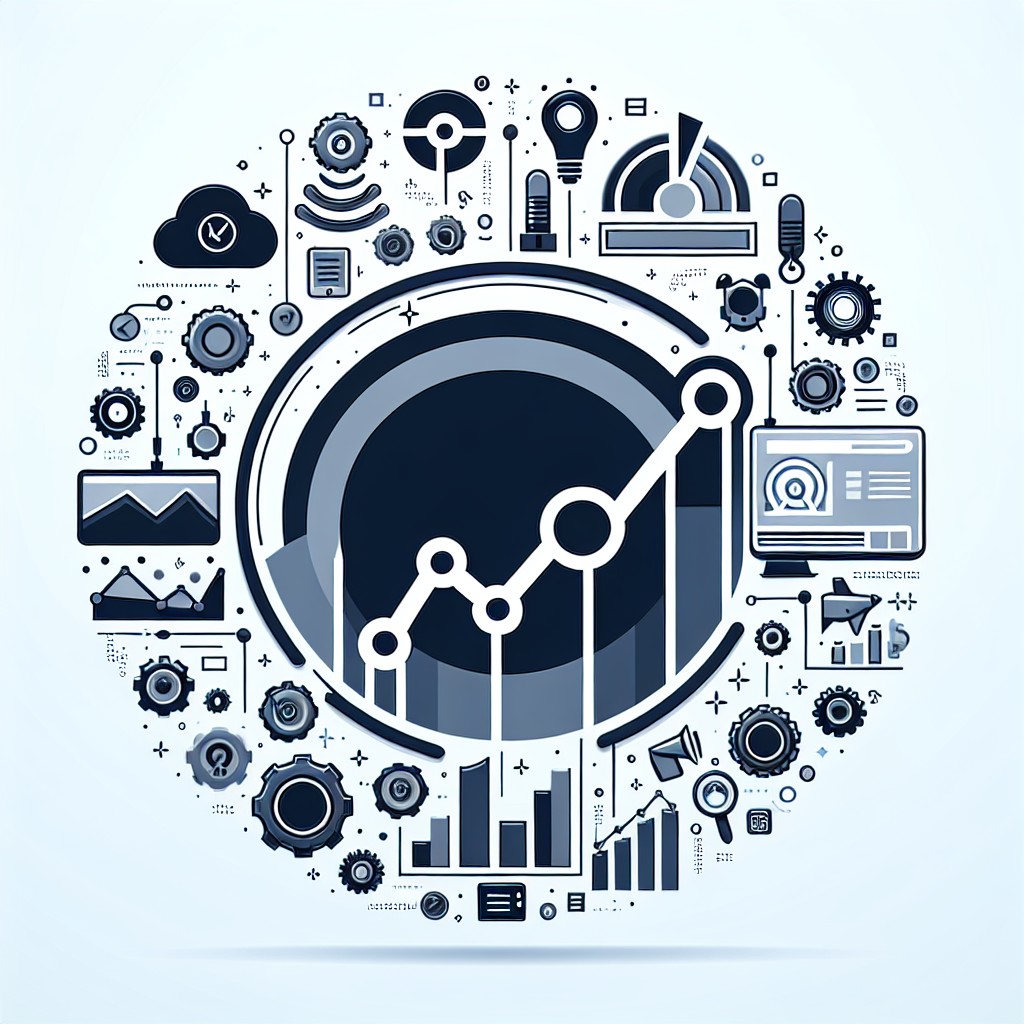