The Rise of AI Driven Attribution Models in a Cookieless World
During a critical budget review last February, Jesse's CMO asked a question that left the entire marketing team momentarily speechless: "How confident are we that our attribution model will still work when third-party cookies disappear?" The uncomfortable silence that followed revealed a troubling reality—Jesse's entire measurement framework, the system determining where millions in marketing budget would flow, was built on foundations rapidly eroding beneath them. That evening, Jesse dove into researching alternatives and discovered an emerging field of AI-driven attribution models designed specifically for privacy-first environments. What began as a crisis response has evolved into a fascination with how artificial intelligence is fundamentally reimagining marketing measurement for a world where individual tracking is no longer the default.
Introduction: The Attribution Crisis
Marketing attribution—the science of determining which touchpoints influence consumer decisions—stands at a critical inflection point. For over a decade, multi-touch attribution models relying on third-party cookies have served as the gold standard for digital marketing measurement. Now, with Google Chrome phasing out third-party cookies, Apple's Intelligent Tracking Prevention limiting measurement windows, and privacy regulations restricting data collection, these traditional models are rapidly becoming obsolete.
According to Forrester Research, 82% of marketers report significant concerns about their ability to measure campaign effectiveness in a cookieless environment. Meanwhile, Gartner predicts that by 2025, over 60% of marketers will have transitioned to AI-driven attribution models that operate effectively without third-party identifiers.
This transition isn't merely a technical shift but a fundamental reimagining of how marketing effectiveness is measured. As Wharton professor Peter Fader notes, "We're witnessing marketing measurement evolve from tracking individuals to understanding patterns—a shift that may ultimately provide more valuable strategic insights than the pseudoprecision of cookie-based attribution."
1. The Limits of Traditional Attribution in a Privacy-First World
Traditional multi-touch attribution models face existential challenges in today's landscape:
Identity Fragmentation
With cookie deprecation and mobile identifier restrictions, customer journeys can no longer be comprehensively tracked across touchpoints. A Google study found that marketers can now observe only 68% of the digital customer journey on average, down from 92% in 2018.
Measurement Windows
Apple's privacy changes have reduced attribution windows from 28 days to just 7 days in many contexts, severely limiting visibility into longer purchase journeys. Research from Publicis Groupe showed this change alone caused a 20-35% decrease in reported conversions across affected channels.
Walled Garden Expansion
Major platforms like Facebook, Amazon, and TikTok increasingly restrict data access, creating measurement silos. According to eMarketer, 76% of digital ad spend now flows through environments with limited cross-platform tracking capabilities.
As marketing scientist Dr. Augustine Fou observes: "The attribution models that have guided digital marketing decisions for the past decade were built for a world that no longer exists—one with unfettered tracking capabilities and minimal privacy concerns."
2. AI-Driven Alternatives: The New Attribution Paradigms
In response to these challenges, several AI-powered approaches have emerged:
Media Mix Modeling (MMM) Renaissance
This decades-old econometric approach is experiencing a revival through AI enhancement. Unilever employed machine learning to modernize their MMM framework, reducing modeling time from months to weeks while increasing accuracy by 31%.
Incrementality Experimentation
Platforms like Measured and TVSquared use automated experimentation to determine true incremental impact. Meal kit service HelloFresh implemented this approach to discover that 40% of their attributed conversions would have occurred without advertising—a finding that reshaped their channel strategy.
Probabilistic Attribution
Rather than deterministic tracking, AI models identify patterns that suggest causal relationships. Sportswear brand Adidas utilizes probabilistic models that operate without personal identifiers, maintaining 82% of the accuracy of their previous cookie-based systems.
Unified Marketing Measurement (UMM)
These hybrid approaches combine multiple measurement techniques through AI orchestration. Cosmetics giant L'Oréal's UMM framework integrates top-down and bottom-up measurement, delivering attribution insights even in channels with zero identifiers.
Stanford professor and former Google chief measurement strategist Cassie Kozyrkov explains: "The most sophisticated AI attribution models don't just work around privacy limitations—they exploit them by focusing on patterns rather than individuals, often revealing insights that individual-level tracking missed entirely."
3. The Technological Foundations: How AI Makes It Possible
Several AI technologies are enabling this measurement revolution:
Machine Learning for Signal Processing
Advanced algorithms identify meaningful patterns amid noisy and incomplete data. Meta's Robyn framework employs gradient boosting and Bayesian optimization to extract attribution signals from aggregated data without individual-level tracking.
Time Series Modeling
Techniques like LSTM (Long Short-Term Memory) networks analyze temporal relationships between marketing activities and business outcomes. Google's Privacy Sandbox initiative leverages these approaches to provide conversion insights while respecting privacy boundaries.
Causal Inference AI
Beyond correlation, these models apply rigorous causal analysis frameworks. Microsoft's DoWhy library implements causal inference algorithms that help brands distinguish between correlation and true marketing impact.
Federated Learning
This approach trains algorithms across decentralized data sources without centralizing sensitive information. Mastercard's attribution system employs federated learning to analyze purchase patterns while keeping individual transaction data secure.
4. Implementation Frameworks: From Theory to Practice
Organizations successfully implementing AI-driven attribution follow structured approaches:
Multi-Signal Integration
Rather than relying solely on user-level tracking, successful models incorporate diverse signals. Consumer packaged goods leader Procter & Gamble combines first-party data, market research, and aggregated publisher data to create "signal graphs" that power attribution without cross-site tracking.
Experimentation Infrastructure
Companies like Airbnb have built robust experimentation platforms that continuously test marketing effectiveness through controlled experiments rather than passive tracking, generating causal insights regardless of cookie availability.
Incrementality Focus
Moving beyond last-click attribution, brands like StitchFix measure true incremental impact through geo-matched market tests automated by machine learning, revealing that previous attribution models had overvalued some channels by up to 40%.
Direct-to-Consumer Insights
Apparel brand Patagonia leverages first-party purchase data combined with AI-driven marketing mix models to understand channel effectiveness without cross-site tracking.
As Jim Sterne, founder of the Digital Analytics Association, emphasizes: "The most successful measurement frameworks aren't trying to recreate cookie-based attribution—they're reimagining measurement for a new reality."
5. Future Directions: Beyond Current Capabilities
The next frontier of AI-driven attribution is already emerging:
Synthetic Data Modeling
Privacy-enhancing techniques generate synthetic user journeys that statistically mirror real behavior patterns without exposing individual data. Netflix employs this approach to test attribution models against realistic but non-identifiable consumer pathways.
Multi-Agency Measurement
Consortium approaches like the World Federation of Advertisers' Cross-Media Measurement Initiative use privacy-preserving techniques to combine measurement signals across publishers and platforms while protecting user privacy.
Real-Time Attribution Calibration
Rather than static models, systems that continuously recalibrate based on market shifts and campaign performance. Multinational consumer electronics company Samsung implemented self-tuning attribution models that automatically adjust to changing market conditions.
Conclusion: The Measurement Evolution
The demise of third-party cookies, while initially viewed as a measurement crisis, is catalyzing a long-overdue evolution in marketing attribution. AI-driven approaches don't merely work around privacy limitations—they leverage different types of data and statistical techniques to deliver more strategic insights than their cookie-dependent predecessors.
Organizations embracing these new paradigms aren't just maintaining measurement capabilities—they're gaining deeper understanding of true marketing effectiveness while simultaneously respecting consumer privacy. As the industry transitions from tracking individuals to modeling behavior, marketing measurement may paradoxically become both more privacy-friendly and more accurate.
Call to Action
For marketing leaders navigating this attribution transformation:
- Audit your current measurement stack to identify dependencies on soon-to-be-deprecated tracking technologies
- Invest in AI and data science capabilities specifically focused on privacy-preserving measurement techniques
- Build first-party data infrastructure that can provide owned signals to power new attribution models
- Implement clean room technologies that enable analysis across platforms without exposing individual-level data
- Develop experimentation frameworks that reveal causal relationships between marketing activities and business outcomes
The organizations that embrace AI-driven attribution today won't just survive the deprecation of third-party tracking—they'll establish new competitive advantages through superior measurement capabilities in the privacy-first era.
Featured Blogs
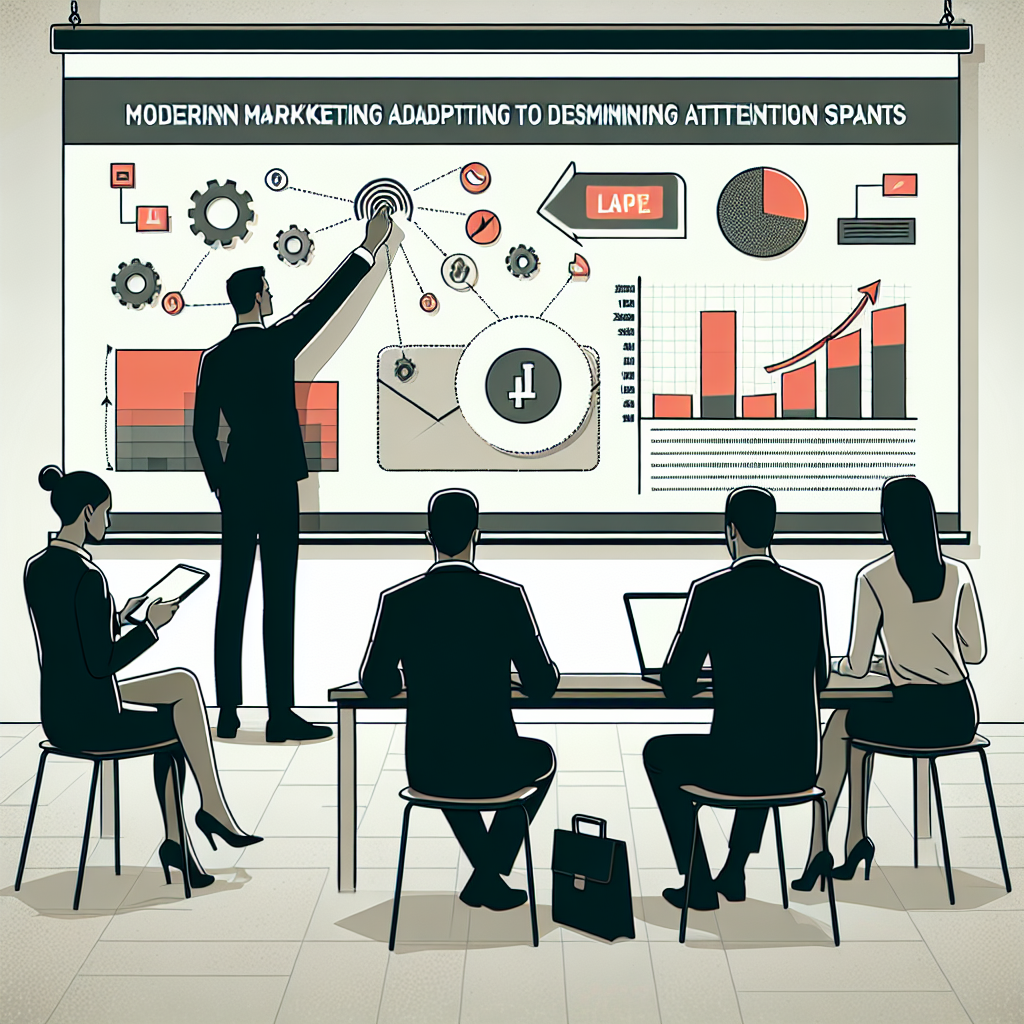
How the Attention Recession Is Changing Marketing
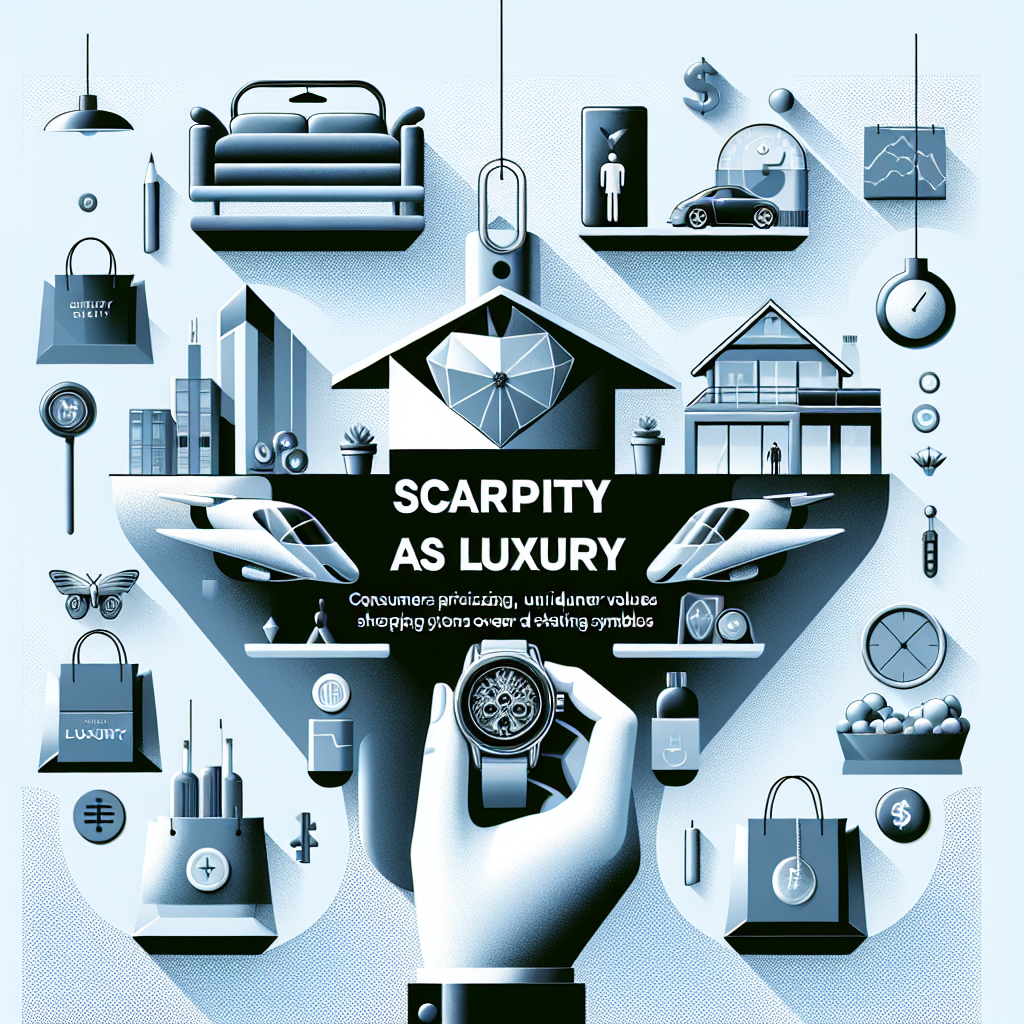
The New Luxury Why Consumers Now Value Scarcity Over Status
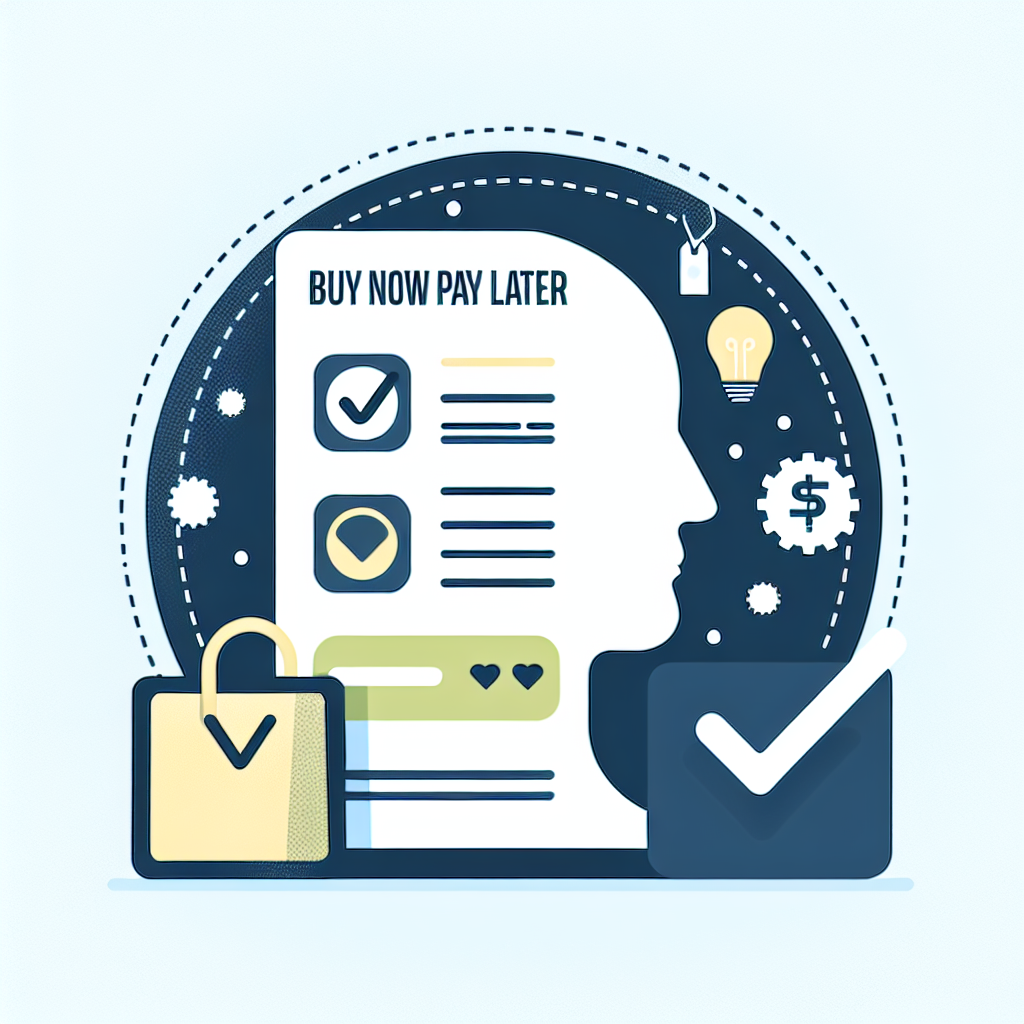
The Psychology Behind Buy Now Pay later
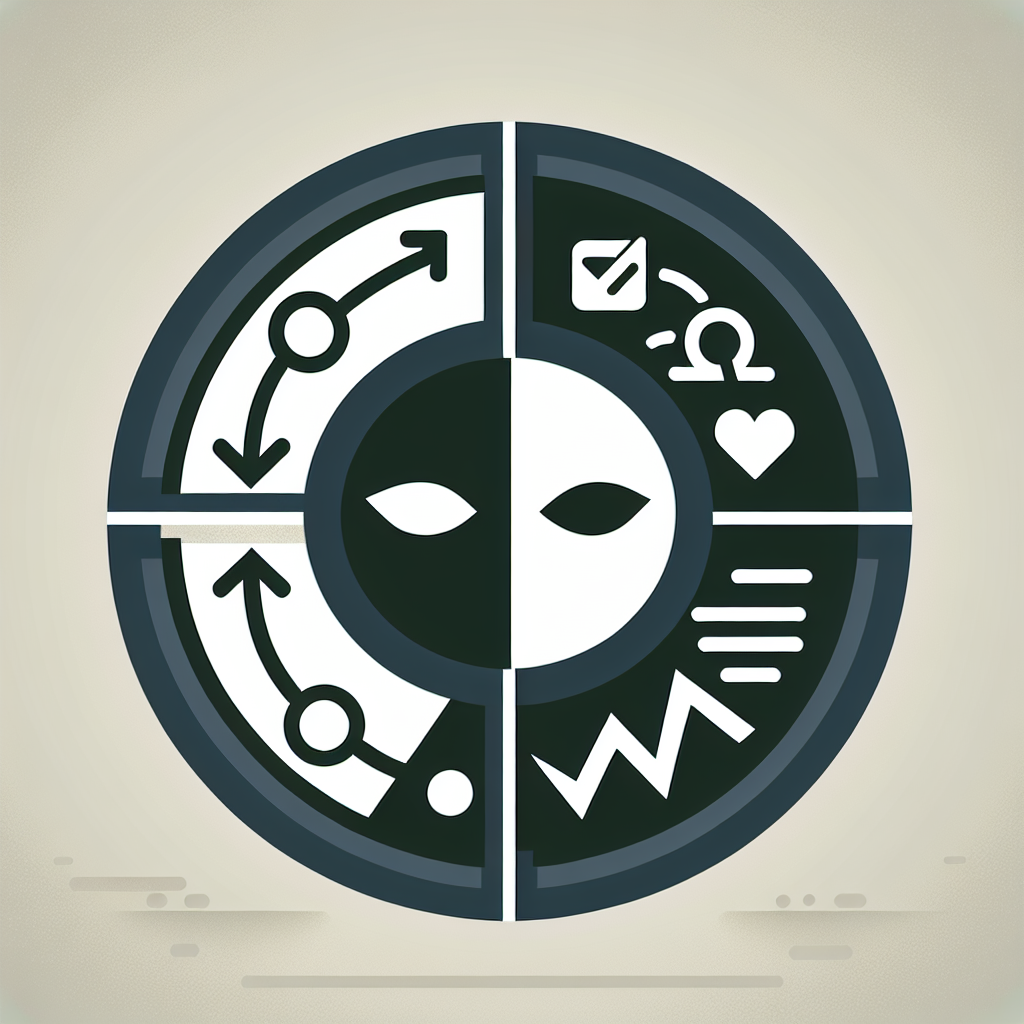
The Role of Dark Patterns in Digital Marketing and Ethical Concerns
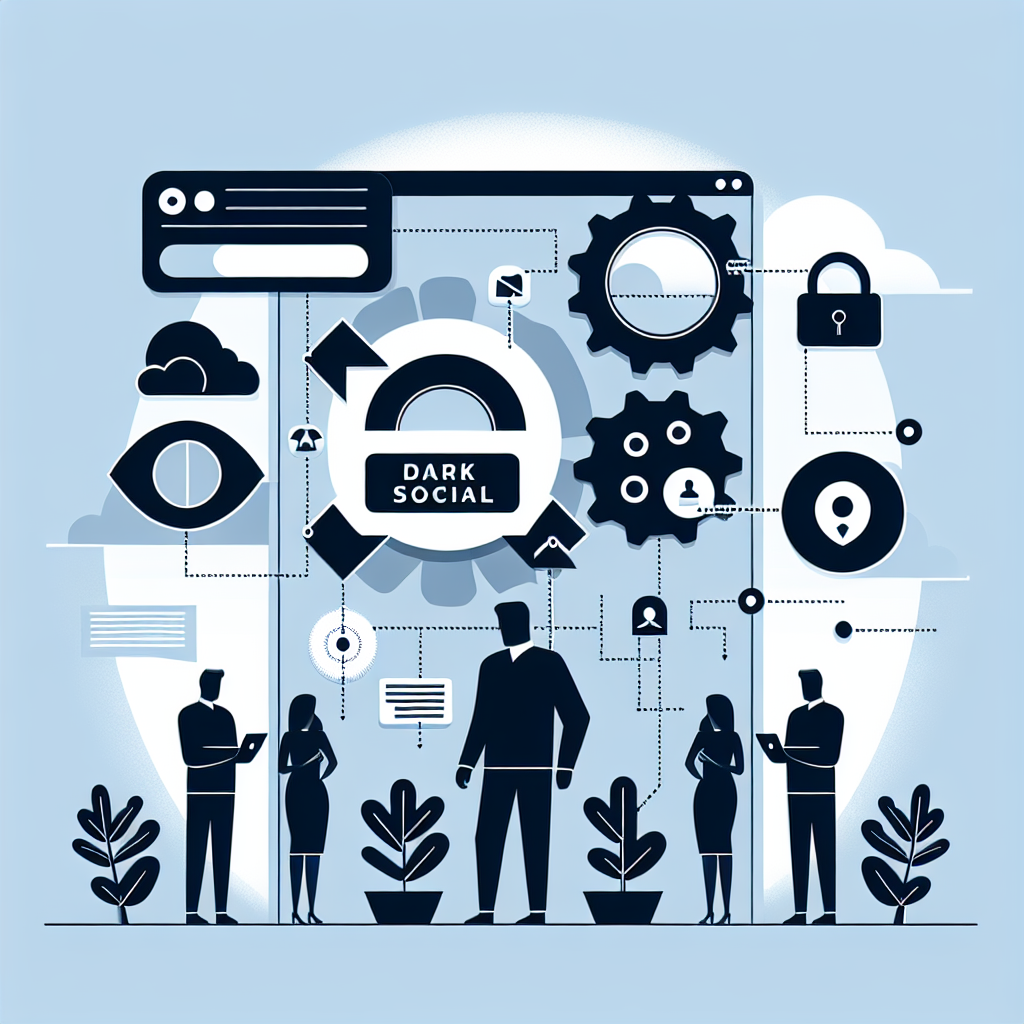
The Rise of Dark Social and Its Impact on Marketing Measurement
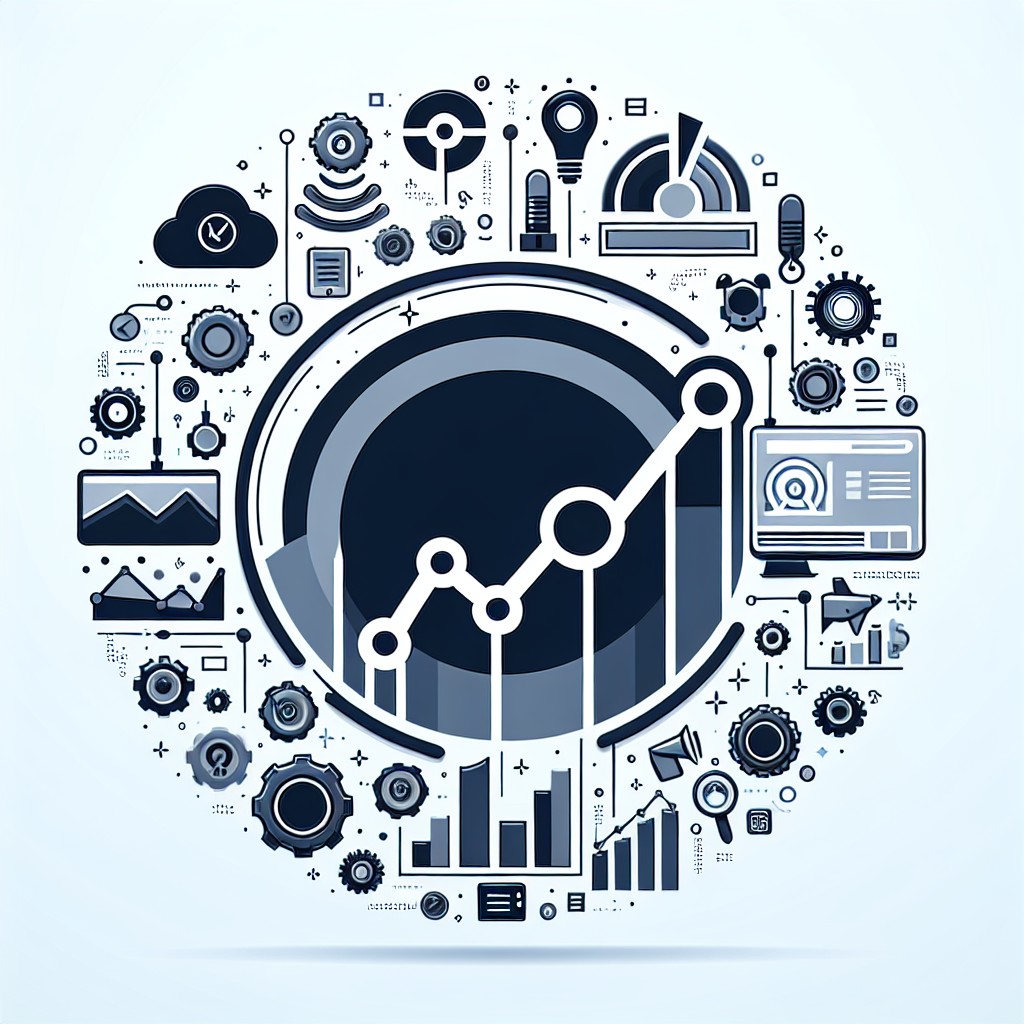