Privacy-Preserving Attribution: How to Measure Marketing Without Cookies
Last summer, Jesse’s digital marketing team was celebrating a seemingly successful campaign when their analytics specialist walked in with troubling news: "Our attribution data is increasingly unreliable. Third-party cookies are disappearing, and we can't accurately track customer journeys anymore." That moment sparked Jesse’s journey into exploring privacy-preserving attribution methods. Like many marketers, he had grown dependent on cookies for years, but the digital landscape was transforming beneath his feet, forcing him to innovate or risk flying blind.
Introduction: The End of an Era
The digital marketing ecosystem stands at a critical inflection point. With Google's planned deprecation of third-party cookies in Chrome, Apple's Intelligent Tracking Prevention, and stringent regulations like GDPR and CCPA, the foundation of digital measurement is crumbling. According to eMarketer, 72% of marketers believe that the loss of third-party cookies will harm their attribution capabilities. This seismic shift demands a fundamental rethinking of how we measure marketing effectiveness while respecting consumer privacy.
The cookie's demise isn't merely a technical challenge—it represents a paradigm shift in the relationship between brands and consumers. As Harvard Business School professor John Deighton notes, "We're witnessing the end of surveillance marketing and the beginning of permission marketing 2.0." This article explores the emerging attribution methodologies that balance measurement accuracy with privacy protection in a cookieless world.
1. First-Party Data Activation
The cornerstone of privacy-preserving attribution lies in first-party data—information collected directly from customer interactions with brand touchpoints. Unlike third-party cookies, first-party data is both privacy-compliant and higher quality.
Nestlé demonstrates this approach through its unified customer data platform (CDP) that aggregates first-party signals across owned touchpoints. By implementing rigorous consent management protocols, Nestlé maintains measurement capabilities while respecting privacy preferences. Their digital engagement increased by 30% through personalization powered by consensual first-party data.
The strategic framework for first-party activation follows what McKinsey calls the "Data Value Chain"—collection, integration, activation, and governance—with privacy embedded throughout each stage. Brands must invest in robust data infrastructure that captures valuable signals while maintaining transparent data practices.
2. Privacy Sandbox and Aggregate Measurement
Google's Privacy Sandbox initiative represents a fundamental shift toward aggregate measurement through proposed APIs like Attribution Reporting. These solutions use differential privacy techniques to measure conversion data without tracking individuals across sites.
The Washington Post has been among the early adopters testing these frameworks. By implementing on-device processing that sends only anonymized, aggregated signals to their analytics systems, they've maintained 93% of their measurement capability while eliminating individual-level tracking. The approach exemplifies what Stanford privacy researcher Jonathan Mayer calls "data minimization in practice."
The key innovation is moving computation to the device rather than centralizing user data—a technique known as edge computing. This approach creates what academic research terms "privacy by design," where privacy protection is built into systems rather than added afterward.
3. Machine Learning and Conversion Modeling
When direct measurement becomes impossible, probabilistic modeling fills the gaps. Advanced machine learning techniques can predict conversion likelihood even when the complete customer journey isn't visible.
Adidas implemented conversion modeling to address measurement gaps in their cross-device customer journeys. By training ML models on anonymized, aggregated data patterns, they maintained 95% accuracy in conversion attribution despite losing 40% of traditional tracking signals. As Adidas's Chief Digital Officer explained, "We're shifting from tracking every step to understanding the overall path."
The methodology follows what statisticians call "Bayesian inference"—updating probability estimates as new data becomes available. This approach allows marketers to make informed decisions despite incomplete information, a capability becoming increasingly valuable in privacy-first environments.
4. Clean Rooms and Privacy-Enhancing Technologies
Data clean rooms provide secure environments where first-party data from multiple sources can be analyzed without sharing or exposing raw customer information. These environments use cryptographic techniques like secure multi-party computation to derive insights while protecting underlying data.
Disney's collaboration with major advertisers through its clean room technology demonstrates the potential. By matching campaign exposure data with conversion information in a protected environment, they maintained attribution accuracy while ensuring no personal data was exposed. Disney reported a 20% improvement in measurement precision across previously challenging channels like connected TV.
As MIT Technology Review highlighted, these solutions represent practical applications of "privacy-enhancing technologies" (PETs)—cryptographic innovations that enable computation on encrypted data without decryption.
5. Media Mix Modeling Renaissance
The constraints of user-level tracking have revitalized media mix modeling (MMM)—a technique that uses aggregate data and statistical methods to determine marketing effectiveness across channels.
Unilever's modern approach to MMM combines traditional econometric techniques with machine learning and high-frequency data inputs. By analyzing aggregate sales patterns against marketing investments while incorporating external variables like seasonality and competitor activity, they measure channel effectiveness without tracking individuals. This methodology allowed them to optimize their marketing mix, resulting in an 11% efficiency gain despite reduced tracking capabilities.
The approach aligns with what Deloitte terms "augmented MMM"—traditional statistical methods enhanced by AI and broader data signals to provide faster, more accurate insights without privacy compromises.
Conclusion: Balancing Measurement and Privacy
The future of marketing measurement doesn't require choosing between attribution accuracy and privacy protection. By combining these emerging methodologies into a holistic measurement framework, marketers can maintain visibility while respecting evolving privacy expectations. The most successful organizations will develop what Forrester Research calls "adaptive measurement systems"—flexible approaches that evolve alongside the regulatory and technical landscape.
As cookies crumble, the opportunity arises to build more sustainable, respectful relationships with consumers based on transparency and value exchange. The path forward isn't about replicating cookie-based tracking through alternative means, but fundamentally rethinking how we measure marketing effectiveness in a privacy-conscious world.
Call to Action
For marketing leaders navigating this transition, the time for action is now:
- Audit your current attribution systems to identify cookie dependencies
- Invest in first-party data infrastructure and consent management
- Experiment with privacy-preserving measurement techniques before cookies disappear completely
- Collaborate with technology and privacy teams to develop integrated approaches
- Join industry initiatives developing privacy-preserving standards
By embracing these strategies today, you won't just survive the cookieless future—you'll gain competitive advantage in the privacy-first digital ecosystem of tomorrow.
Featured Blogs
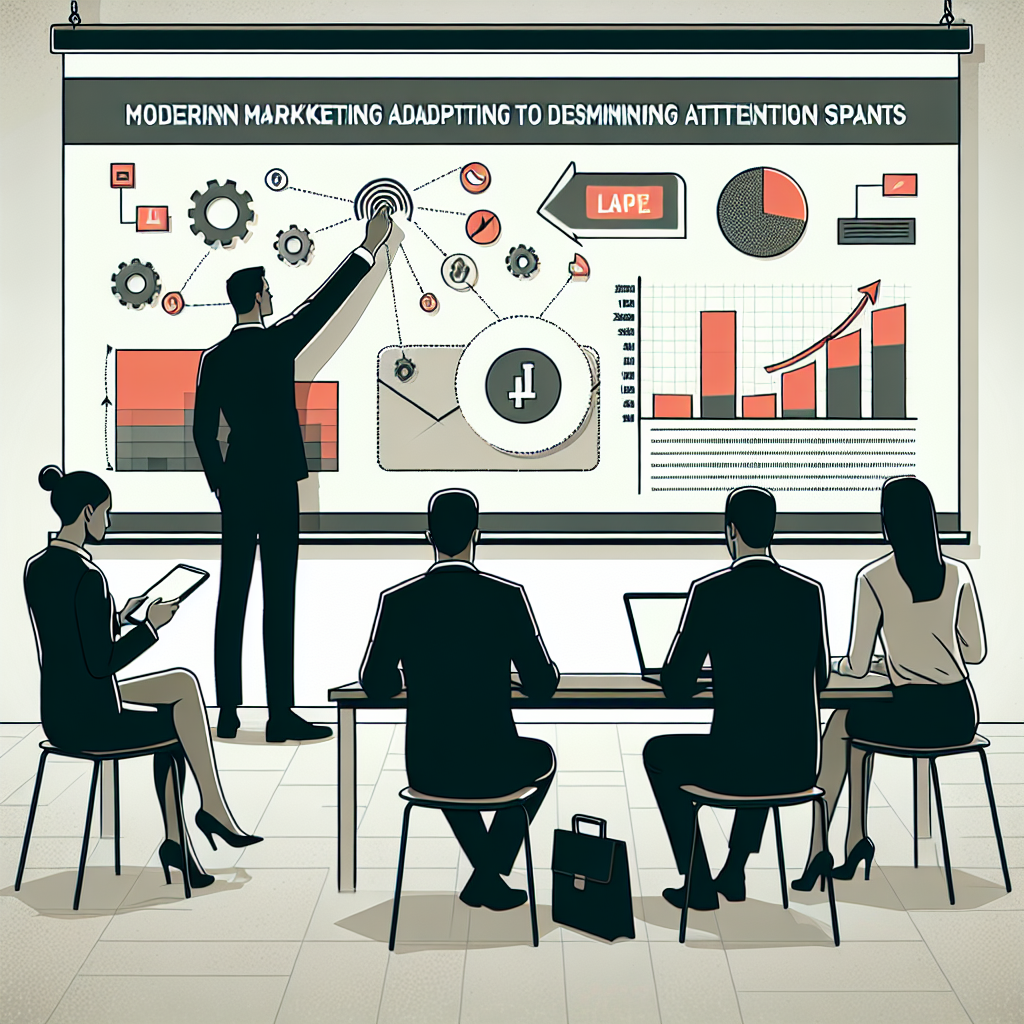
How the Attention Recession Is Changing Marketing
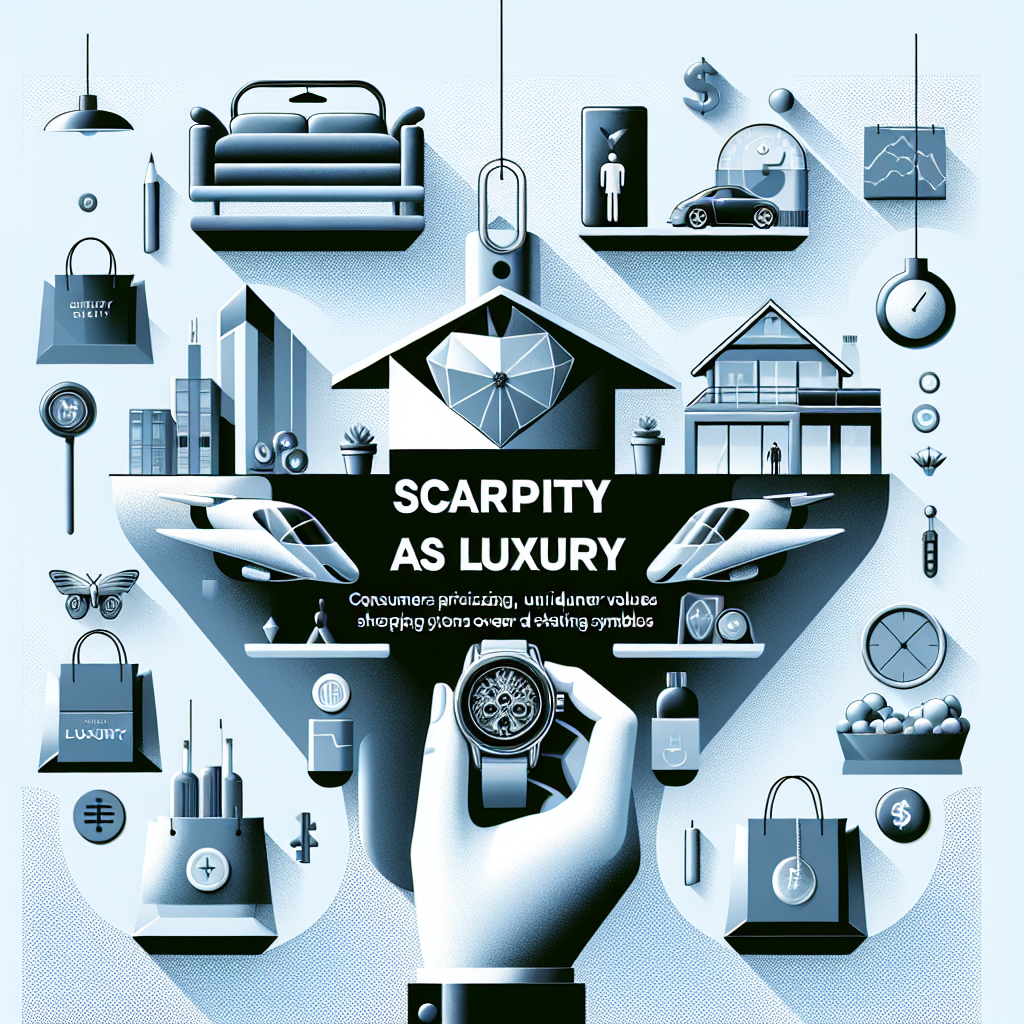
The New Luxury Why Consumers Now Value Scarcity Over Status
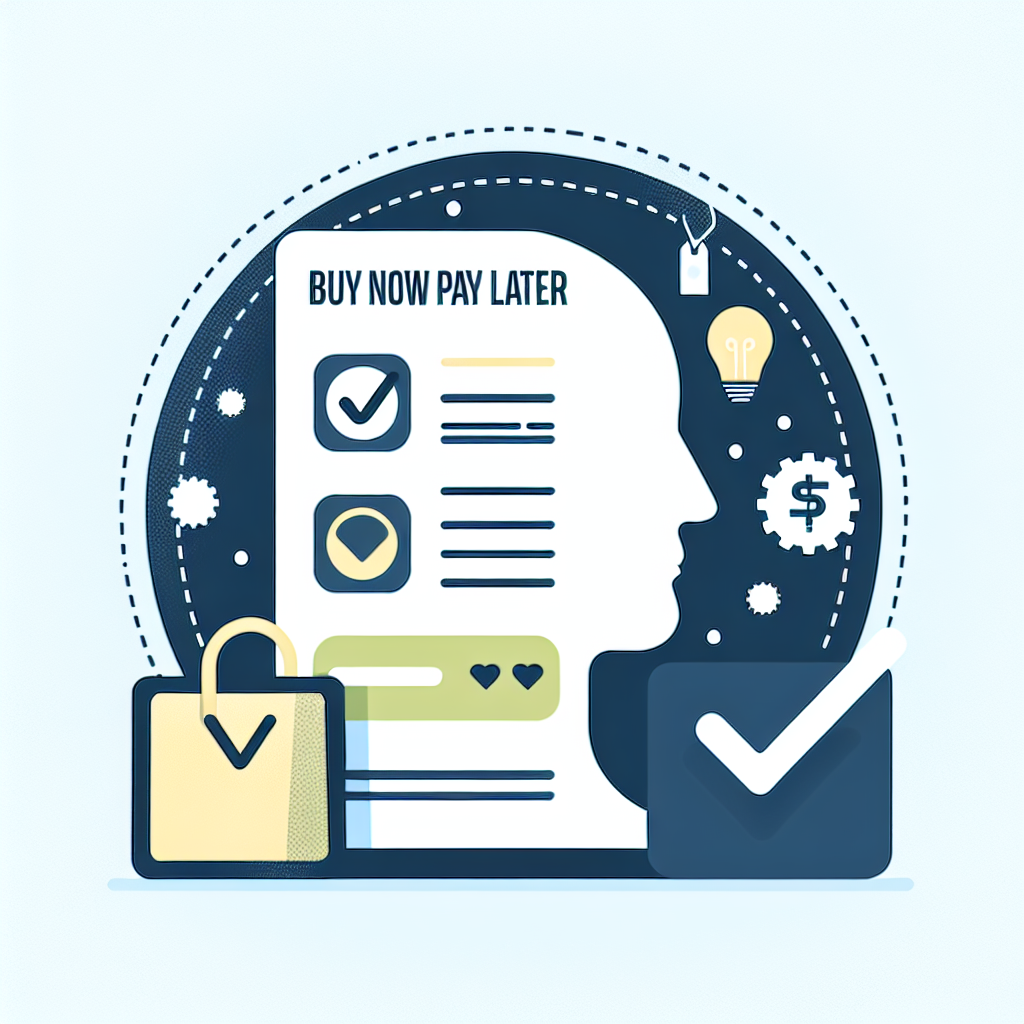
The Psychology Behind Buy Now Pay later
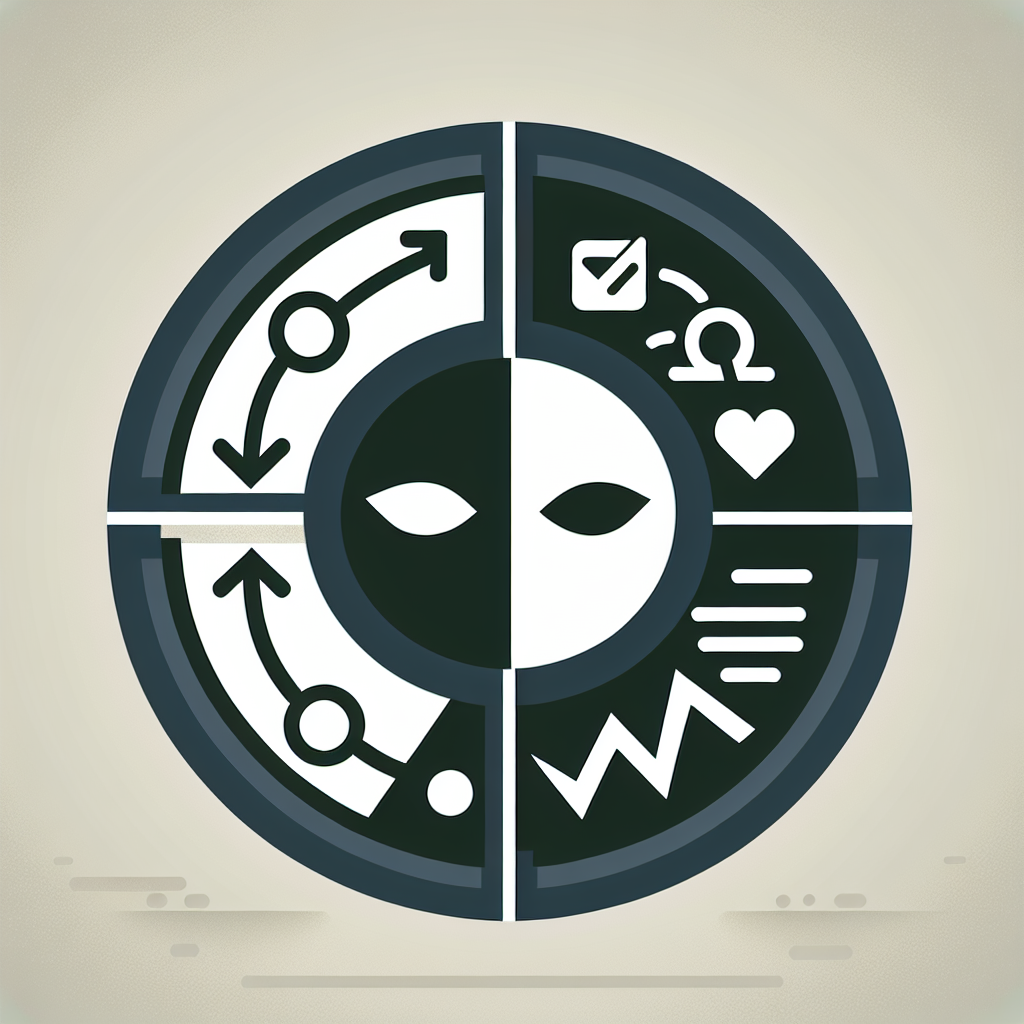
The Role of Dark Patterns in Digital Marketing and Ethical Concerns
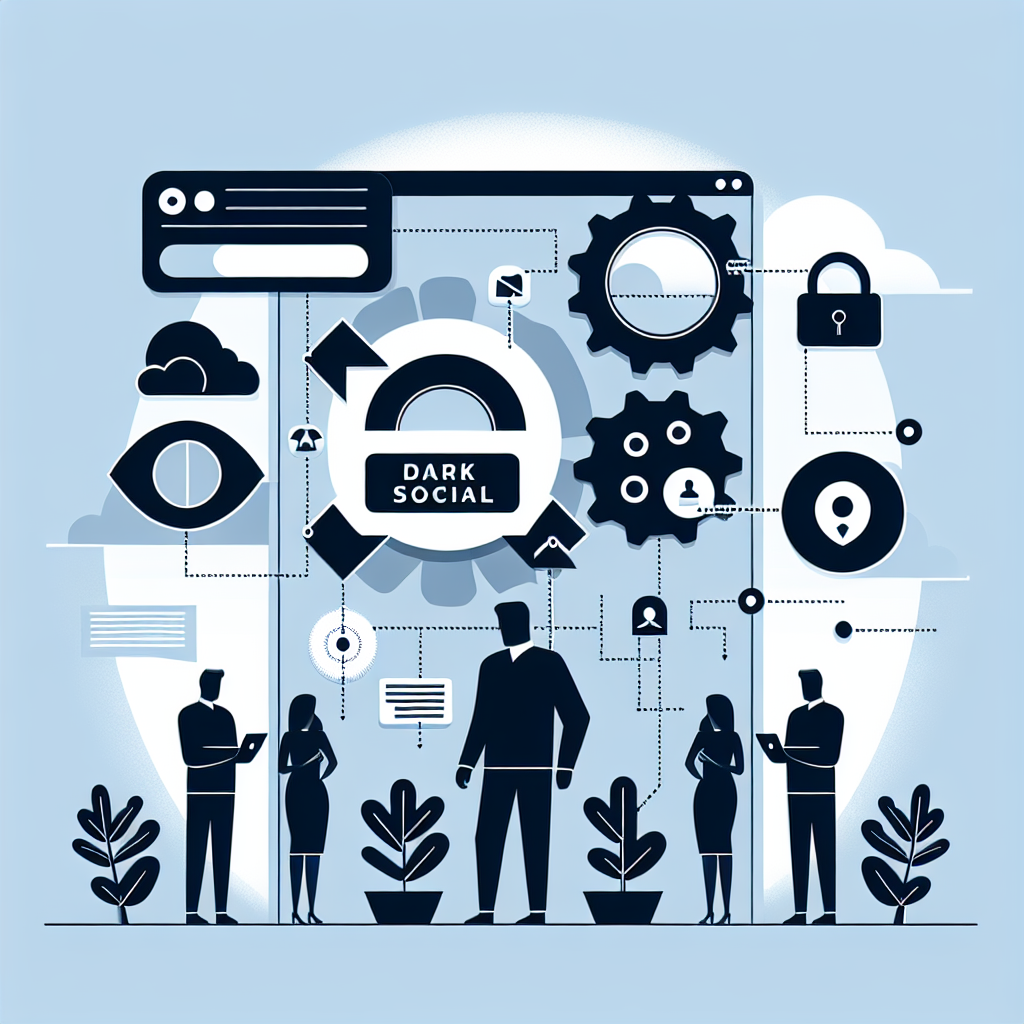
The Rise of Dark Social and Its Impact on Marketing Measurement
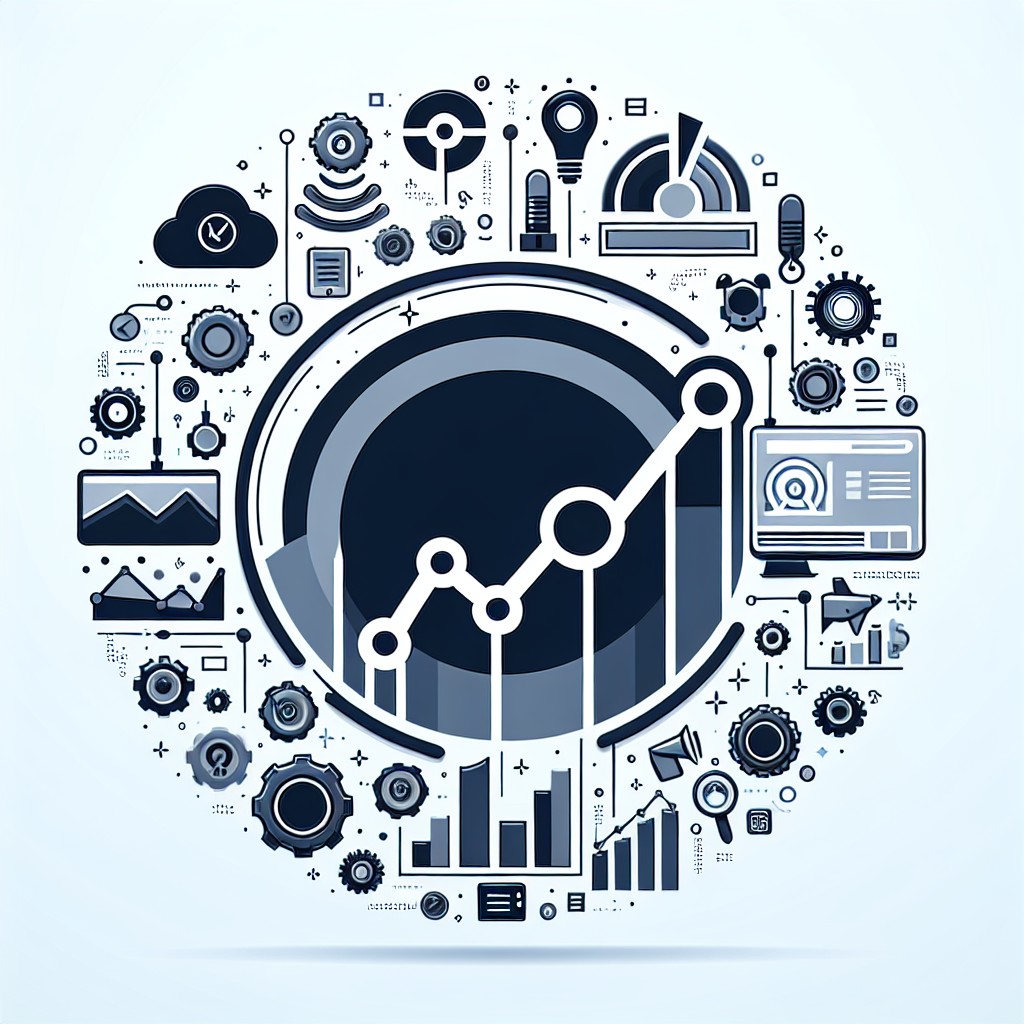