How Privacy Preserving Technologies Like Differential Privacy Work
Jesse's fascination with privacy-preserving technologies began with an uncomfortable revelation. During a marketing analytics conference, a presenter demonstrated how seemingly anonymized datasets could be re-identified through sophisticated matching techniques. As the presenter cross-referenced “anonymous” browsing data with public records to reveal specific individuals’ identities, Jesse noticed the audience shifting nervously in their seats. His own team had been using similar "anonymized" datasets for years, confidently assuring clients that their practices were privacy-compliant. That evening in his hotel room, Jesse began researching alternative approaches and stumbled upon a paper on differential privacy from Microsoft Research. The mathematical elegance of the solution captivated him—here was a technique that could offer valuable insights while providing provable privacy guarantees. What began as professional concern quickly evolved into intellectual fascination. Jesse realized they were witnessing the emergence of an entirely new approach to data—one that could fundamentally resolve the tension between analytical utility and privacy protection.
Introduction: Beyond the Privacy Paradox
For decades, marketers have operated within what Stanford privacy researcher Helen Nissenbaum calls "the privacy paradox"—the perceived tradeoff between data utility and privacy protection. Conventional wisdom held that meaningful analytics required unfettered access to raw data, while privacy protection necessarily diminished analytical capabilities.
Privacy-preserving technologies, particularly differential privacy, fundamentally challenge this assumption. These advances represent what the MIT Technology Review calls "the most significant innovation in data privacy since encryption"—enabling organizations to derive insights from sensitive data without exposing individual records.
As Professor Cynthia Dwork, differential privacy pioneer from Harvard University, explains: "These technologies don't merely offer incremental improvements to existing approaches—they represent a fundamental reconceptualization of privacy, transforming it from a qualitative goal to a quantitative, measurable property of algorithms."
For marketers navigating increasingly stringent privacy regulations and rising consumer expectations, these technologies offer a path to maintain analytical capabilities while demonstrating genuine privacy commitment.
1. The Technical Foundation: How Differential Privacy Actually Works
At its core, differential privacy introduces precisely calibrated statistical "noise" into data or queries. This noise masks individual contributions while preserving aggregate insights with mathematical guarantees of privacy protection.
Unlike traditional anonymization, which attempts to remove identifying information, differential privacy proactively adds noise in proportion to the sensitivity of the information. Professor Aaron Roth of the University of Pennsylvania explains the key distinction: "Anonymization asks 'what information should we remove?' while differential privacy asks 'how much mathematical noise should we add?'"
The approach provides a formal privacy guarantee: no query results will reveal whether any specific individual is included in the dataset. This guarantee is quantified through an "epsilon" value that precisely measures privacy loss—transforming privacy from a vague promise into a measurable property.
The technique's mathematical rigor led Apple's Chief Privacy Officer Jane Horvath to describe it as "the first privacy approach with a formal proof rather than a hope and a promise."
2. Real-World Applications: From Theory to Commercial Implementation
Differential privacy has rapidly evolved from theoretical concept to commercial application across industries:
Apple pioneered commercial implementation by using local differential privacy to gather usage statistics without exposing individual behavior. Their approach, which Apple Fellow Craig Federighi describes as "learning from the crowd while keeping individual users unidentifiable," enables product improvement while maintaining their privacy-first brand promise.
Similarly, LinkedIn developed a differential privacy framework called "Audience Engagements API" that allows advertisers to measure campaign performance without accessing individual-level data. This approach, according to LinkedIn's VP of Engineering Ravi Gummadi, "delivers 92% of the analytical value of raw data while providing mathematical privacy guarantees."
The Census Bureau's adoption of differential privacy for the 2020 census demonstrates its transition from specialized technique to mainstream methodology. As the Bureau's Chief Scientist John Abowd notes, "When constitutionally mandated activities adopt differential privacy as their standard, it signals a fundamental shift in how organizations must approach sensitive data."
3. Marketing Applications: Balancing Insight and Privacy
Forward-thinking marketing organizations have adapted differential privacy specifically for advertising and analytics applications:
Google's Privacy Sandbox initiatives implement differential privacy techniques for ad measurement, allowing marketers to understand conversion patterns without tracking individual journeys. Their approach, which Google's Engineering VP Vint Cerf describes as "privacy-safe attribution," demonstrates how these techniques can address core marketing needs without individual-level tracking.
Procter & Gamble's marketing science team adapted differential privacy for consumer research, developing what their Chief Analytics Officer Guy Peri calls "insight-rich, privacy-safe consumer understanding." Their approach enables behavior analysis without exposing individual activities, resolving long-standing tensions in consumer research.
Professor Catherine Tucker of MIT Sloan notes that these implementations represent a paradigm shift: "Rather than minimizing privacy considerations to maximize insight, differential privacy enables organizations to optimize both simultaneously."
4. Competitive Differentiation: Privacy as Innovation Strategy
Organizations adopting privacy-preserving technologies are discovering unexpected competitive advantages:
Microsoft's confidential computing initiative, which combines differential privacy with secure enclaves, enables what their CTO Kevin Scott calls "privacy-enhancing collaboration." Their approach allows multiple organizations to derive insights from combined datasets without exposing underlying records to each other—creating new partnership opportunities while maintaining data control.
The pharmaceutical industry's adoption of privacy-preserving clinical trial analytics demonstrates similar value. Novartis implemented differential privacy techniques that their Head of Data Science Luca Finelli describes as "enabling research collaboration while protecting patient privacy," accelerating research while addressing regulatory requirements.
Professor Jane Bambauer of the University of Arizona notes that privacy-preserving technologies increasingly function as competitive differentiators: "Organizations that master these techniques gain not only regulatory compliance but also expanded data utilization opportunities unavailable to competitors relying on traditional approaches."
5. The Evolving Landscape: From Single Techniques to Integrated Solutions
As privacy-preserving technologies mature, they increasingly combine into comprehensive platforms:
IBM's privacy-preserving data science suite integrates differential privacy with homomorphic encryption and federated learning. This approach, which their Chief Privacy Officer Christina Montgomery describes as "privacy by design operationalized," enables analysis of sensitive data while it remains encrypted.
The OpenDP Initiative, led by Harvard's Privacy Tools Project, is developing open-source implementations that democratize access to these techniques. Their director, Professor Salil Vadhan, emphasizes that "privacy-preserving analytics is transitioning from specialized expertise to standard practice—a transition that requires accessible tools and education."
Conclusion: The Privacy-Preserving Future
Privacy-preserving technologies, particularly differential privacy, represent not merely a technical response to regulation but a fundamental advancement in how organizations derive value from data. By replacing the false choice between privacy and utility with mathematical frameworks that optimize both simultaneously, these approaches enable what the Harvard Business Review calls "the resolution of the privacy paradox."
Organizations adopting these technologies position themselves not just for regulatory compliance but for sustainable competitive advantage in an increasingly privacy-conscious marketplace. As Apple CEO Tim Cook noted in his 2021 data privacy address, "Organizations that view privacy as innovation rather than constraint will define the next era of digital experiences."
Call to Action
For marketing leaders navigating the privacy-first transition, three priorities emerge:
- Initiate pilot implementations of privacy-preserving technologies, focusing on high-sensitivity analytics use cases
- Invest in technical education around differential privacy and related techniques for analytics and data science teams
- Communicate privacy-preserving approaches to consumers and partners, positioning privacy innovation as a brand commitment rather than merely regulatory compliance
The organizations that execute on these priorities will not merely adapt to privacy constraints—they will transform privacy investment into sustainable competitive advantage through superior data capabilities in the privacy-first era.
Featured Blogs
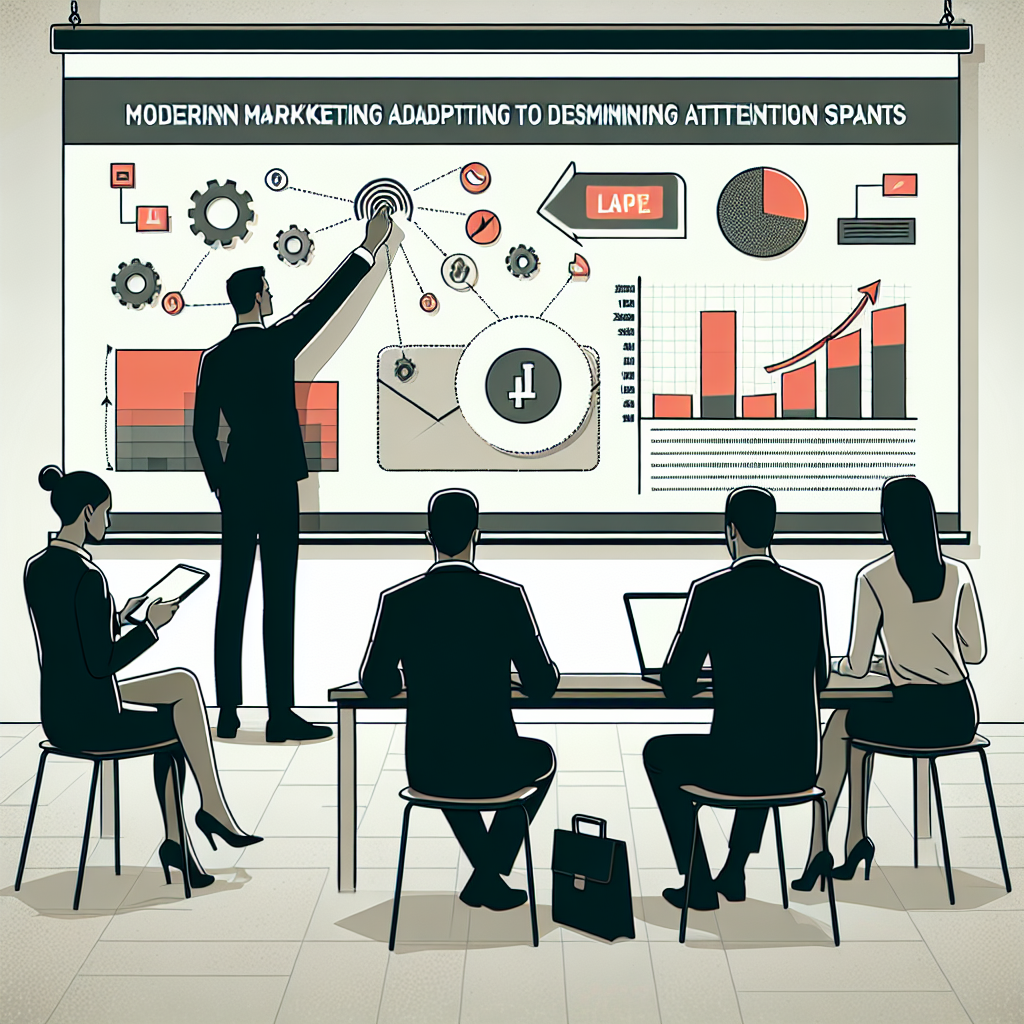
How the Attention Recession Is Changing Marketing
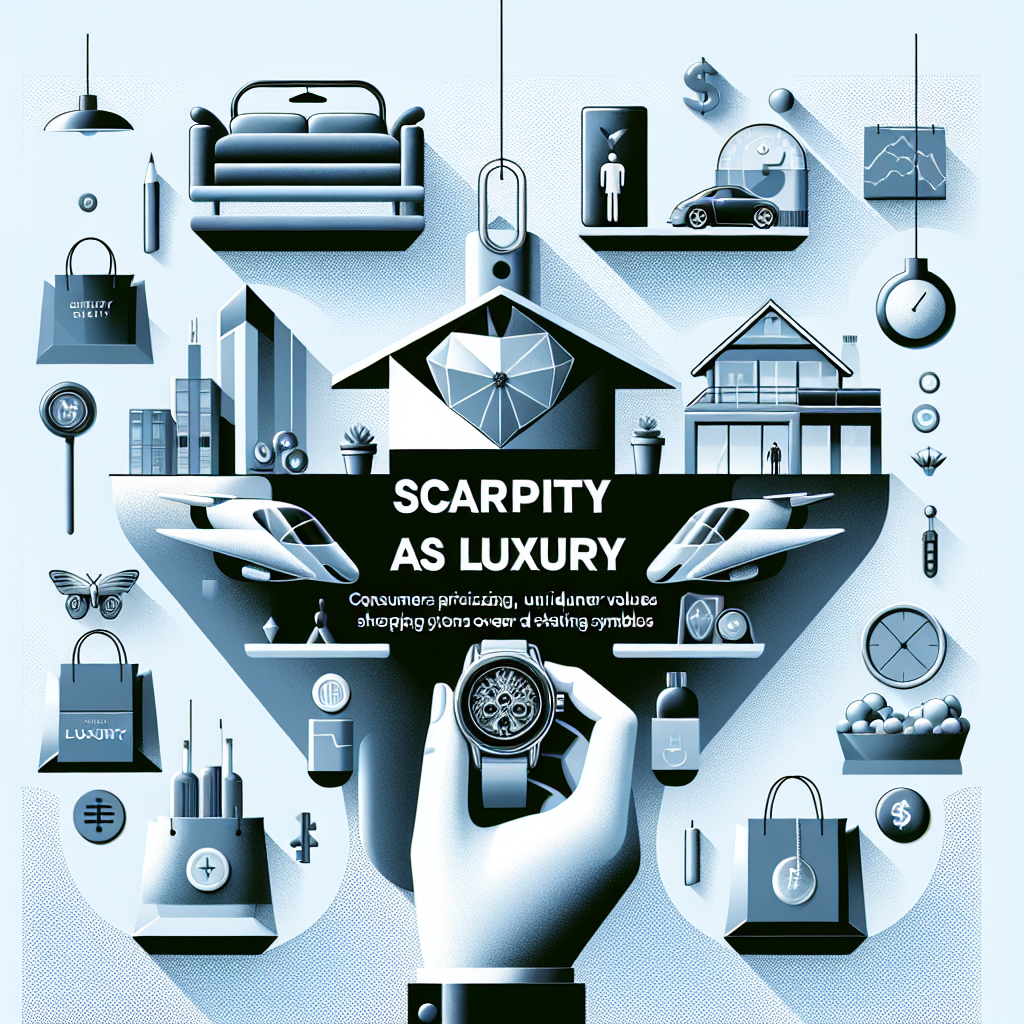
The New Luxury Why Consumers Now Value Scarcity Over Status
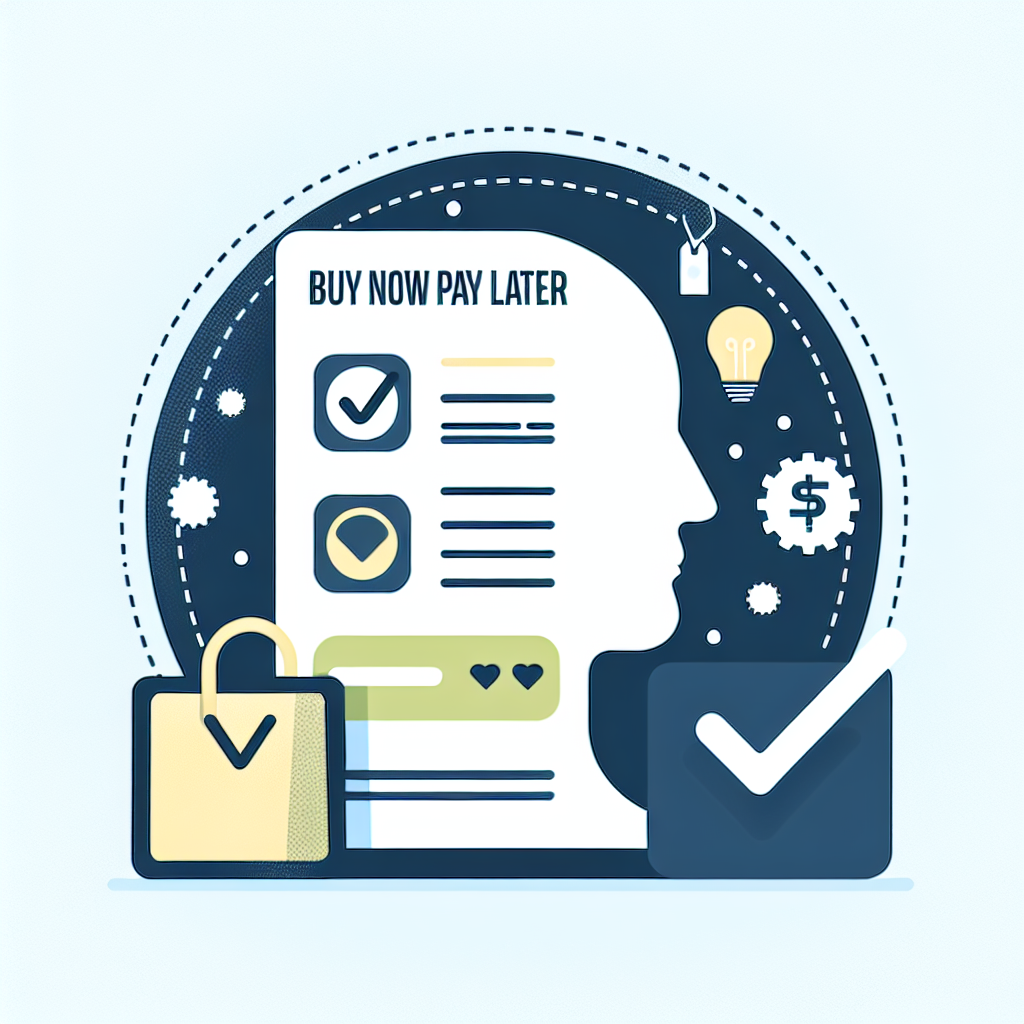
The Psychology Behind Buy Now Pay later
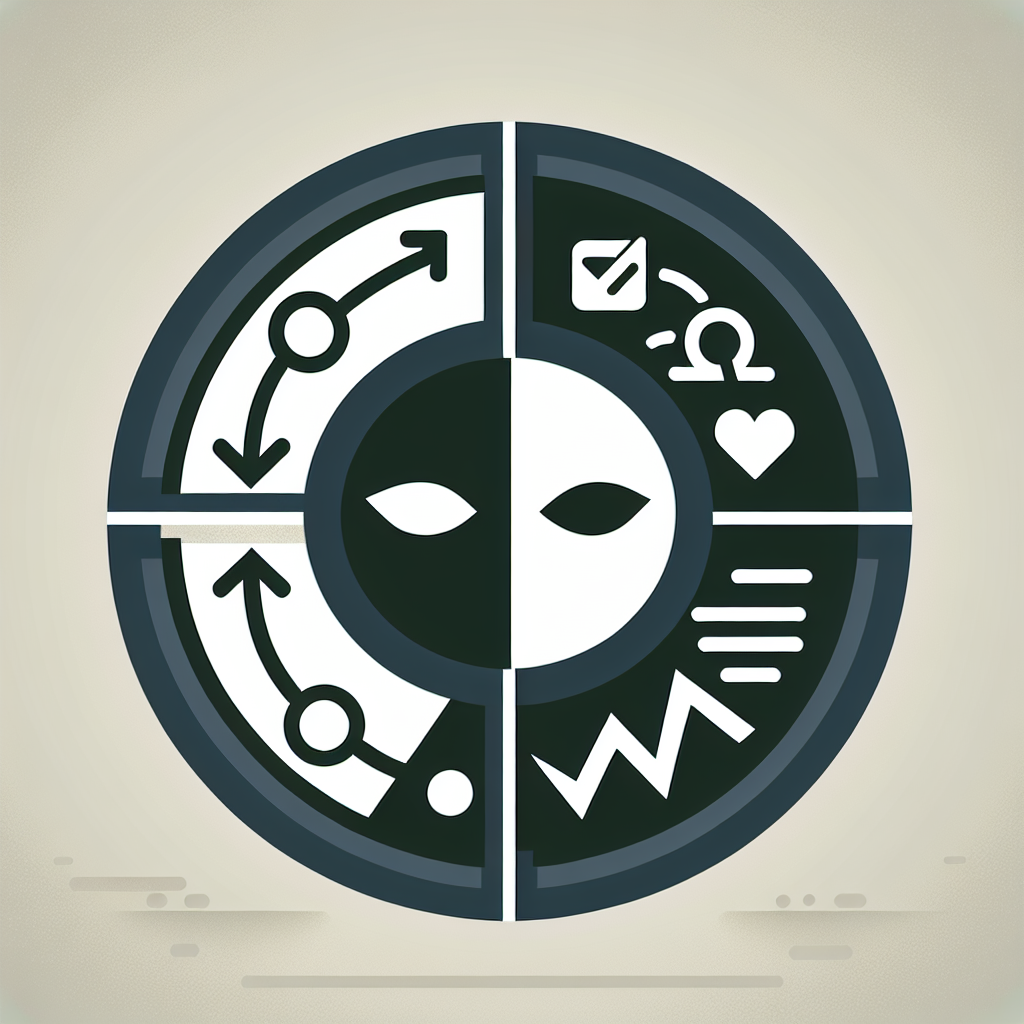
The Role of Dark Patterns in Digital Marketing and Ethical Concerns
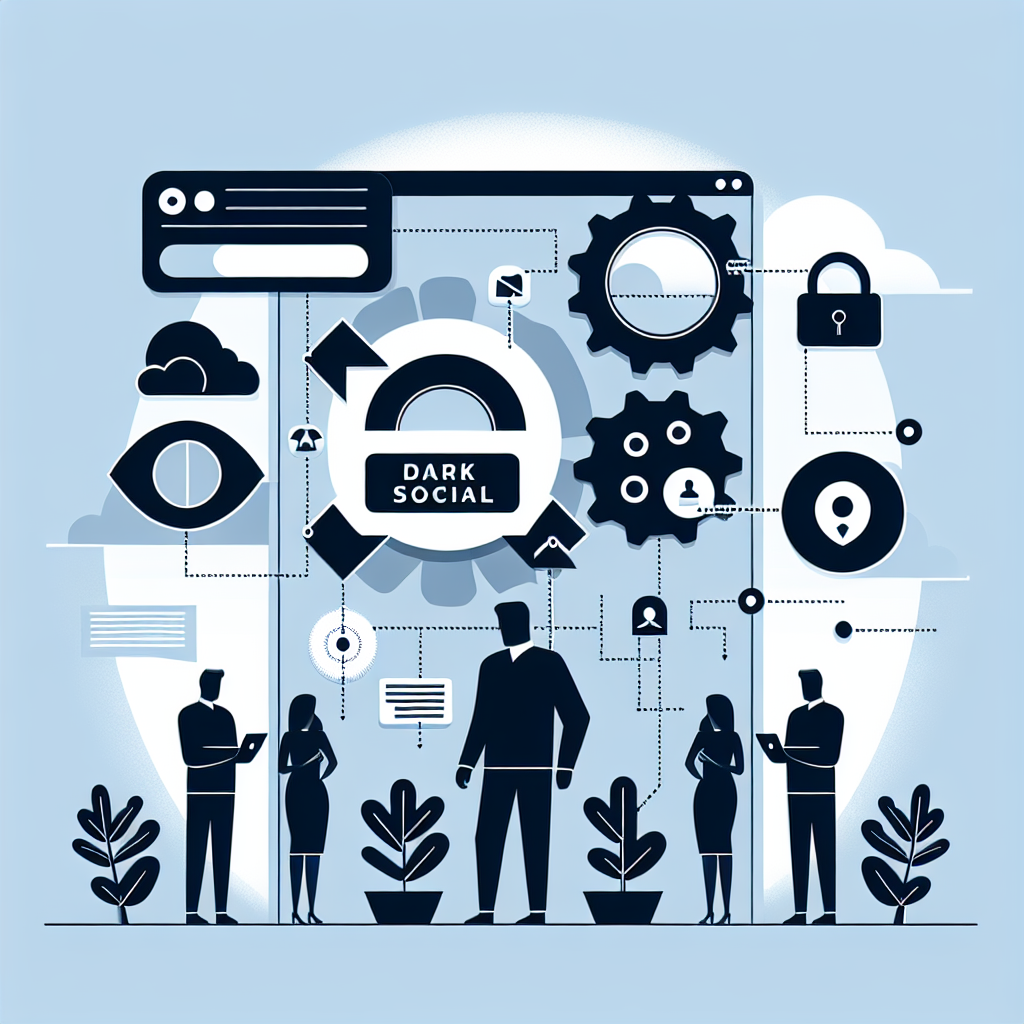
The Rise of Dark Social and Its Impact on Marketing Measurement
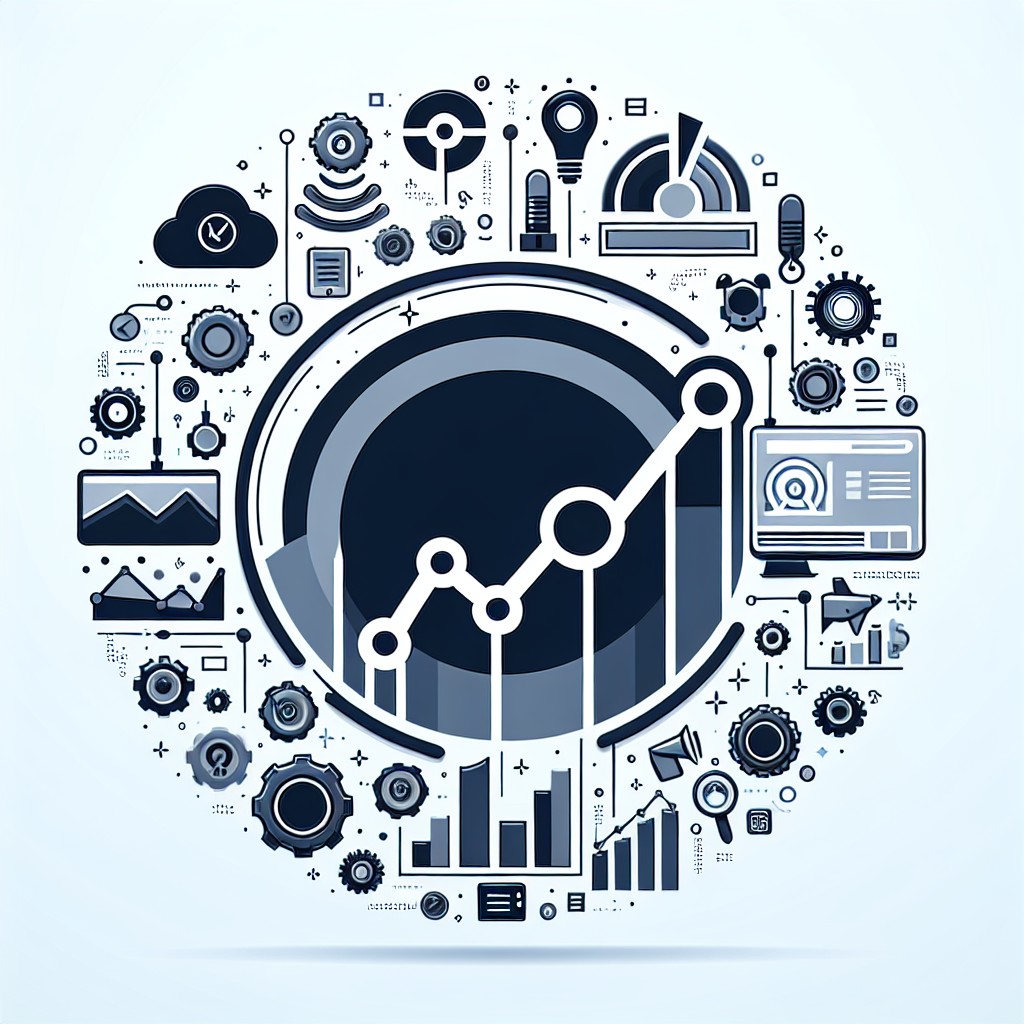