Why Multi-Touch Attribution is Dying & What's Replacing It
The evening Pedro's agency lost a major client still haunts him. They had delivered record-breaking campaign results, yet couldn't definitively prove which channels deserved credit. "Your attribution model shows social driving conversions, but Google Analytics says it's paid search," the client's CMO explained during their final call. "Meanwhile, our internal data suggests it's email. We need clarity, not contradictions." That night, Pedro dove into research on attribution's fundamental limitations, initially hoping to defend their approach. Instead, he discovered something unsettling—the attribution models they had trusted for years were built on increasingly shaky foundations. This realization sparked an eighteen-month journey exploring emerging measurement alternatives. What began as a professional crisis evolved into a fascination with how the marketing analytics landscape is being completely reimagined as traditional attribution crumbles under the weight of privacy changes, walled gardens, and fundamental mathematical limitations.
Introduction
For over a decade, multi-touch attribution (MTA) has represented the gold standard in digital marketing measurement—promising to reveal each touchpoint's precise contribution to conversion. This algorithmic approach to credit allocation became the foundation for budget optimization, channel strategy, and performance evaluation across the industry. Yet despite significant investment and sophistication, MTA now faces an existential crisis. Privacy regulations, browser restrictions, walled garden data policies, and cross-device fragmentation have collectively undermined the data foundation that attribution models require to function. More fundamentally, growing evidence suggests that even with perfect data, attribution's underlying assumptions about customer journeys may be fundamentally flawed. This disruption has forced a profound rethinking of marketing measurement, with organizations pivoting toward alternative frameworks that balance granular insight with practical reliability. Understanding this transformation is essential for marketers seeking to maintain analytical rigor in an increasingly complex, privacy-first digital ecosystem.
1. The Fundamental Limitations of Traditional Attribution
Multi-touch attribution has always contained inherent weaknesses that recent developments have simply exposed more clearly. According to research from Northwestern University's Kellogg School of Management, traditional attribution suffers from several mathematical limitations:
The Path Dependency Problem
Attribution models assume customer journeys follow consistent patterns, but research shows up to 76% of journeys involve non-linear interaction effects that attribution algorithms struggle to capture.
The Baseline Challenge
Traditional models cannot separate incremental impact from what would have happened anyway. Marketing scientist Rex Briggs estimates that between 30-60% of attributed conversions would occur regardless of advertising exposure.
Counterfactual Impossibility
True attribution requires knowing what would have happened without a particular touchpoint—information that's impossible to derive from observational data alone.
These limitations existed before privacy changes, but as marketing technologist Avinash Kaushik notes, "Third-party cookies and device identifiers simply masked these fundamental flaws by creating an illusion of precision through granularity."
2. Privacy Catalysts Accelerating Attribution's Decline
While attribution already faced structural challenges, several privacy developments have accelerated its decline:
Browser Tracking Prevention
Apple's Intelligent Tracking Prevention (ITP) and similar features in Firefox and Chrome have reduced cross-site tracking capabilities by up to 89%, according to Adobe Analytics benchmarks.
Mobile Attribution Limitations
Apple's App Tracking Transparency framework resulted in opt-in rates averaging just 24%, per Flurry Analytics, creating massive blind spots in user journeys.
Walled Garden Expansion
Platform-specific attribution systems from Google, Facebook, and Amazon now account for 74% of digital ad spend according to eMarketer, yet provide increasingly limited data exchange with external systems.
Regulatory Pressure
GDPR, CCPA, and emerging privacy regulations have reduced identifiable data availability by 42% in affected markets, per IAB Europe research.
Marketing professor Scott Galloway describes this as "the great identity fragmentation," where the unified customer view that attribution requires has become increasingly unattainable.
3. Emerging Measurement Alternatives
As attribution's limitations become more pronounced, several alternative approaches are gaining traction:
Marketing Mix Modeling Renaissance
This decades-old statistical approach analyzes the relationship between marketing inputs and business outcomes at an aggregate level. Modernized with machine learning capabilities, MMM solves many privacy challenges by not requiring user-level data.
Procter & Gamble has publicly discussed their shift toward "always-on MMM" using high-frequency data and automated modeling to provide insights in weeks rather than quarters. The approach has reportedly improved their marketing efficiency by 30% while eliminating privacy concerns.
Incrementality Testing
Experimental approaches that measure true causal impact are replacing correlational attribution. Incrementality testing uses randomized controlled trials to measure the true lift provided by a marketing intervention.
Booking.com has implemented an incrementality-focused framework they call "Causal Marketing Analytics," applying experimental design across channels. According to their published case studies, this approach revealed that 35% of their previously attributed conversions would have occurred regardless of advertising exposure.
Conversion Modeling
AI-based approaches that fill measurement gaps through probabilistic modeling rather than direct tracking. Google's implementation uses federated learning to preserve privacy while maintaining analytical utility.
Unified Marketing Measurement
Hybrid frameworks that combine aggregate econometric techniques with available user-level data. According to Forrester Research, organizations implementing UMM approaches are achieving 15-30% higher marketing ROI compared to those relying solely on attribution.
Marketing economist Peter Field notes that these approaches represent "a return to first principles—measuring what advertising actually changes rather than simply what it precedes."
4. AI's Transformative Impact on New Measurement Approaches
Artificial intelligence is fundamentally reshaping measurement capabilities beyond traditional attribution:
Machine Learning for Signal Recovery
Advanced algorithms can now identify patterns in sparse, anonymized data that would be invisible to traditional analysis. Pinterest has developed what they call "Aggregated Event Measurement" using machine learning to recover measurement accuracy despite data limitations.
Causal AI
New approaches like causal inference and structural equation modeling provide a more sophisticated understanding of marketing influence than traditional attribution's correlational approach. According to research from MIT's Digital Economy Initiative, causal AI models can improve prediction accuracy by up to 40% compared to traditional attribution.
Synthetic Data Generation
AI can create privacy-compliant synthetic datasets that maintain the statistical properties of original data without individual identity. Microsoft's Azure Synthetic Data Suite demonstrates how this approach enables analysis without privacy compromise.
The most advanced organizations are combining these AI techniques to create what Deloitte Digital terms "privacy-preserving analytics ecosystems" that maintain measurement capabilities despite data restrictions.
5. Implementation Strategies for the Post-Attribution Era
Forward-thinking organizations are adopting several strategies to navigate the transition from traditional attribution:
Measurement Diversification
Leading brands like Adidas have implemented what their measurement lead calls "analytical triangulation"—using multiple complementary methodologies rather than relying on a single source of truth.
First-Party Data Infrastructure
Companies like Disney have invested heavily in customer data platforms that maximize the value of consensual first-party data while reducing dependence on third-party signals.
Experimentation Culture
Organizations like Booking.com and Airbnb have restructured their analytics approach around continuous experimentation rather than passive measurement, making causal understanding a core capability.
Data Clean Rooms
Collaborative environments that enable analysis across datasets without exposing raw data. Unilever has pioneered this approach with major media partners, allowing cross-platform analysis without privacy compromise.
According to Gartner research, organizations implementing these strategies are 2.3 times more likely to report high confidence in their marketing measurement despite attribution limitations.
Conclusion
The decline of multi-touch attribution represents not simply a technical challenge but a fundamental opportunity to reimagine marketing measurement. As statistician George Box famously noted, "All models are wrong, but some are useful." Attribution models have become less useful as their underlying data foundation erodes, but emerging approaches offer potentially greater insight into true marketing effectiveness.
This transition requires marketers to embrace a more scientific mindset—acknowledging uncertainty, prioritizing causal understanding over correlation, and combining multiple analytical lenses rather than seeking a single perfect measurement solution. The organizations that successfully navigate this shift will gain not just more reliable measurement but deeper insight into what truly drives consumer behavior.
Call to Action
For marketing leaders navigating the post-attribution landscape:
- Audit your current measurement approach to identify attribution dependencies and potential blind spots created by privacy changes.
- Invest in developing mixed-method measurement capabilities that combine the granularity of available user-level data with the causal rigor of experimental and econometric approaches.
- Foster cross-functional collaboration between marketing, analytics, and privacy teams to ensure measurement approaches remain both insightful and responsible as the privacy landscape continues to evolve.
The end of attribution as we've known it doesn't mean the end of marketing accountability—rather, it marks the beginning of a more mature, scientifically grounded approach to understanding marketing's true impact.
Featured Blogs
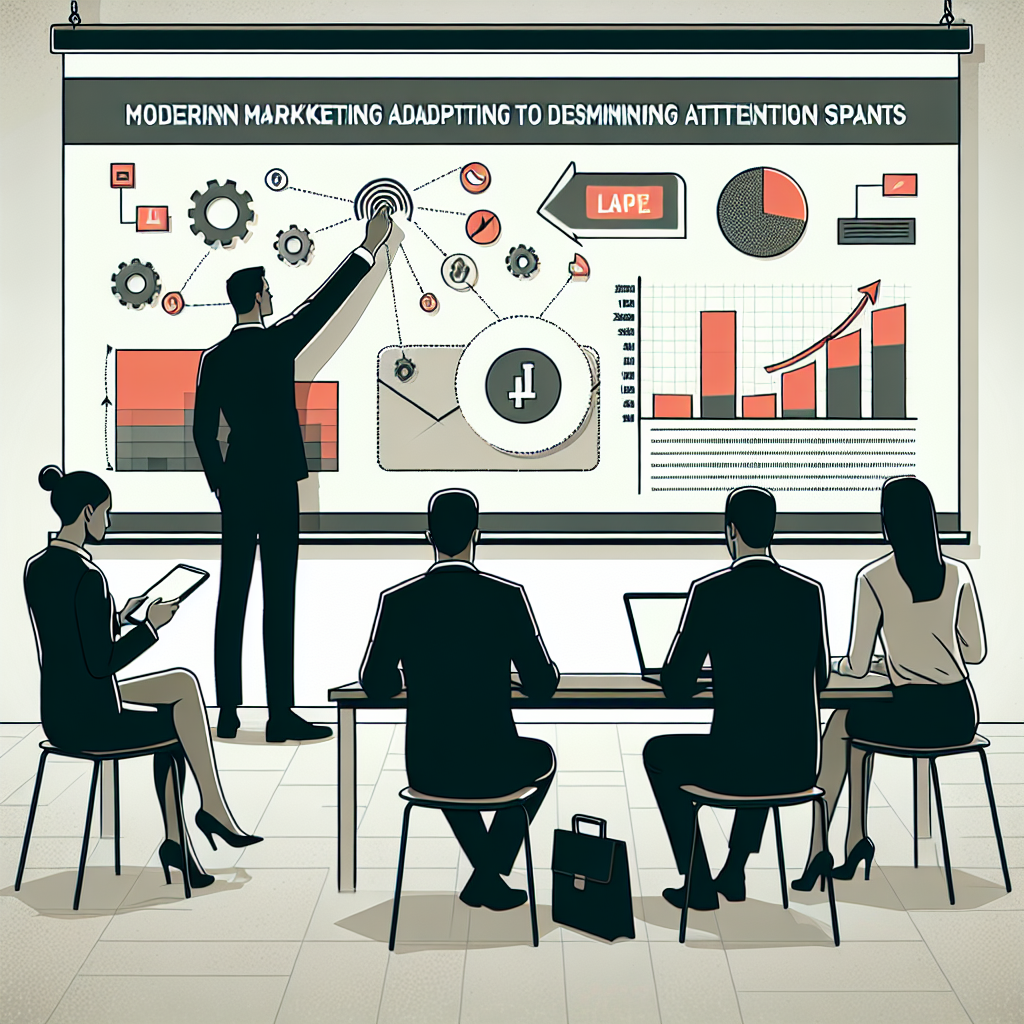
How the Attention Recession Is Changing Marketing
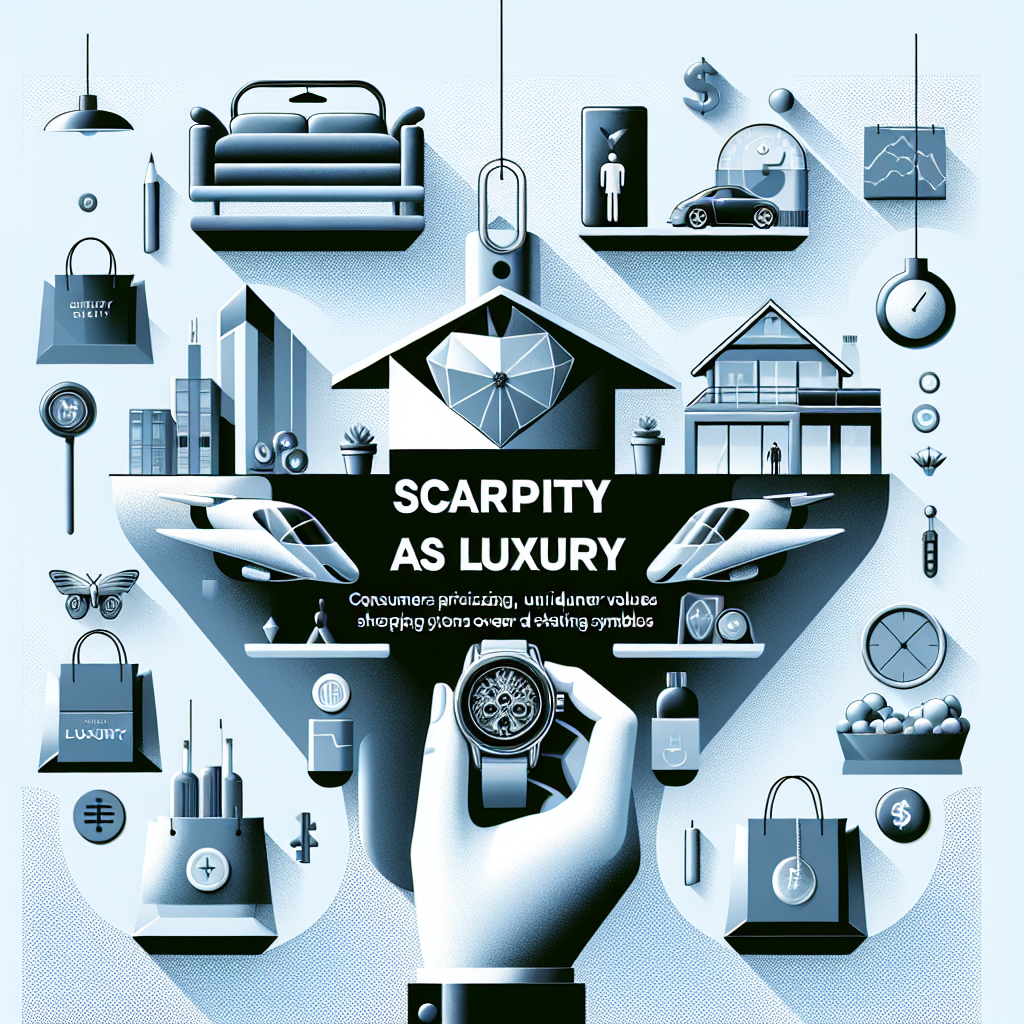
The New Luxury Why Consumers Now Value Scarcity Over Status
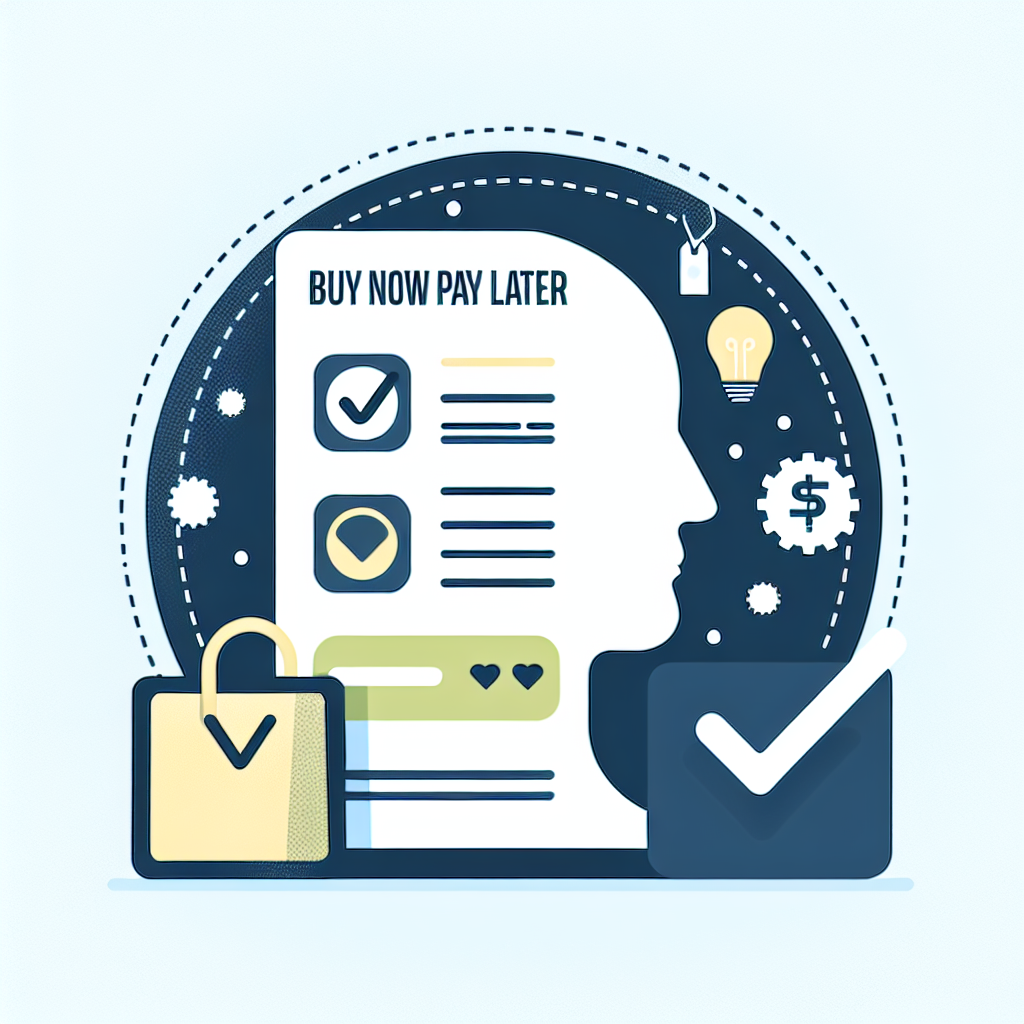
The Psychology Behind Buy Now Pay later
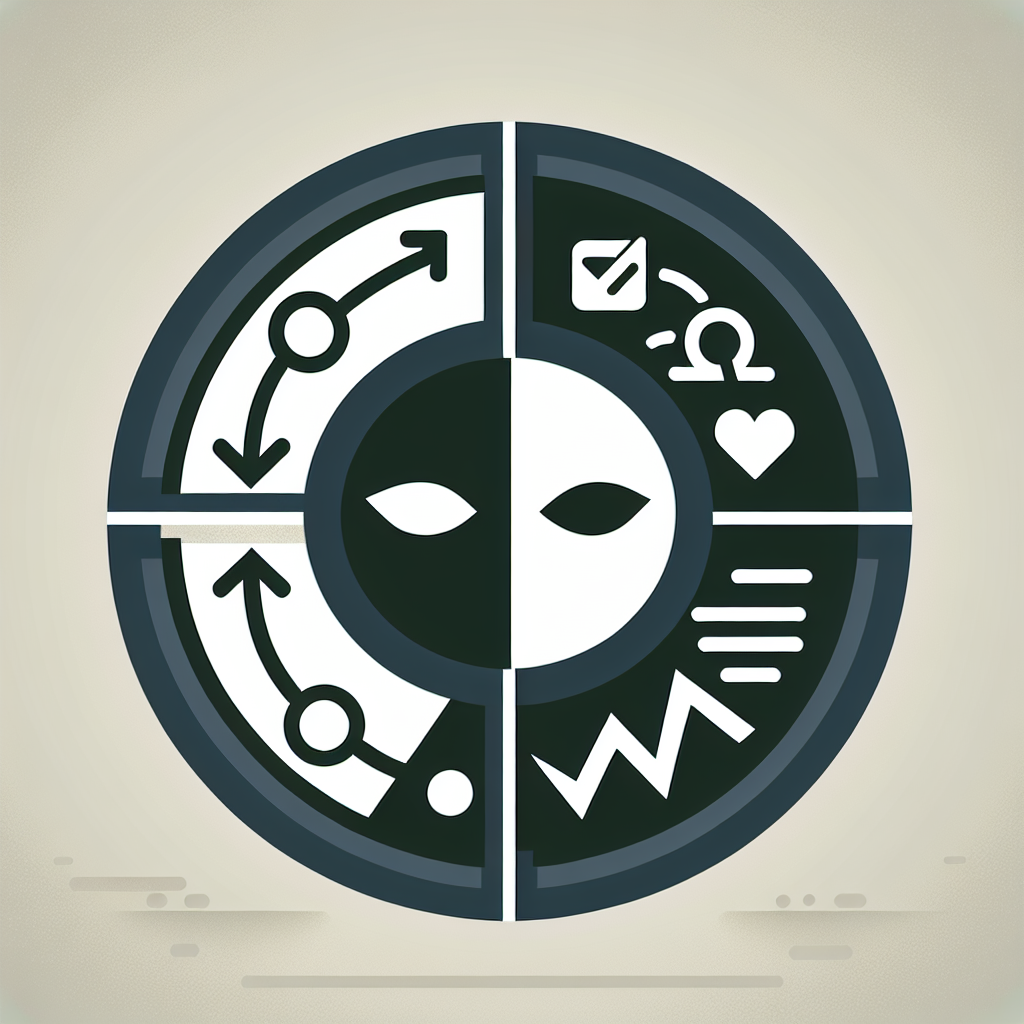
The Role of Dark Patterns in Digital Marketing and Ethical Concerns
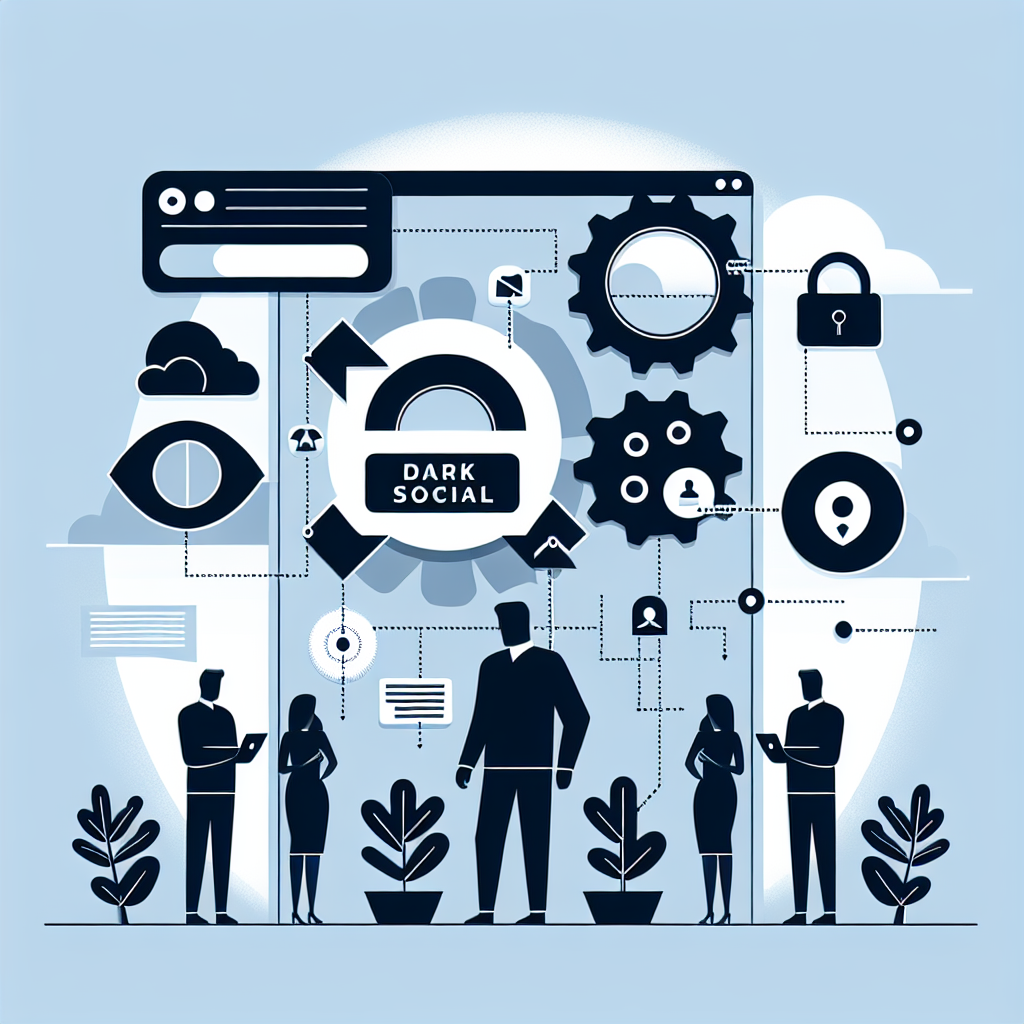
The Rise of Dark Social and Its Impact on Marketing Measurement
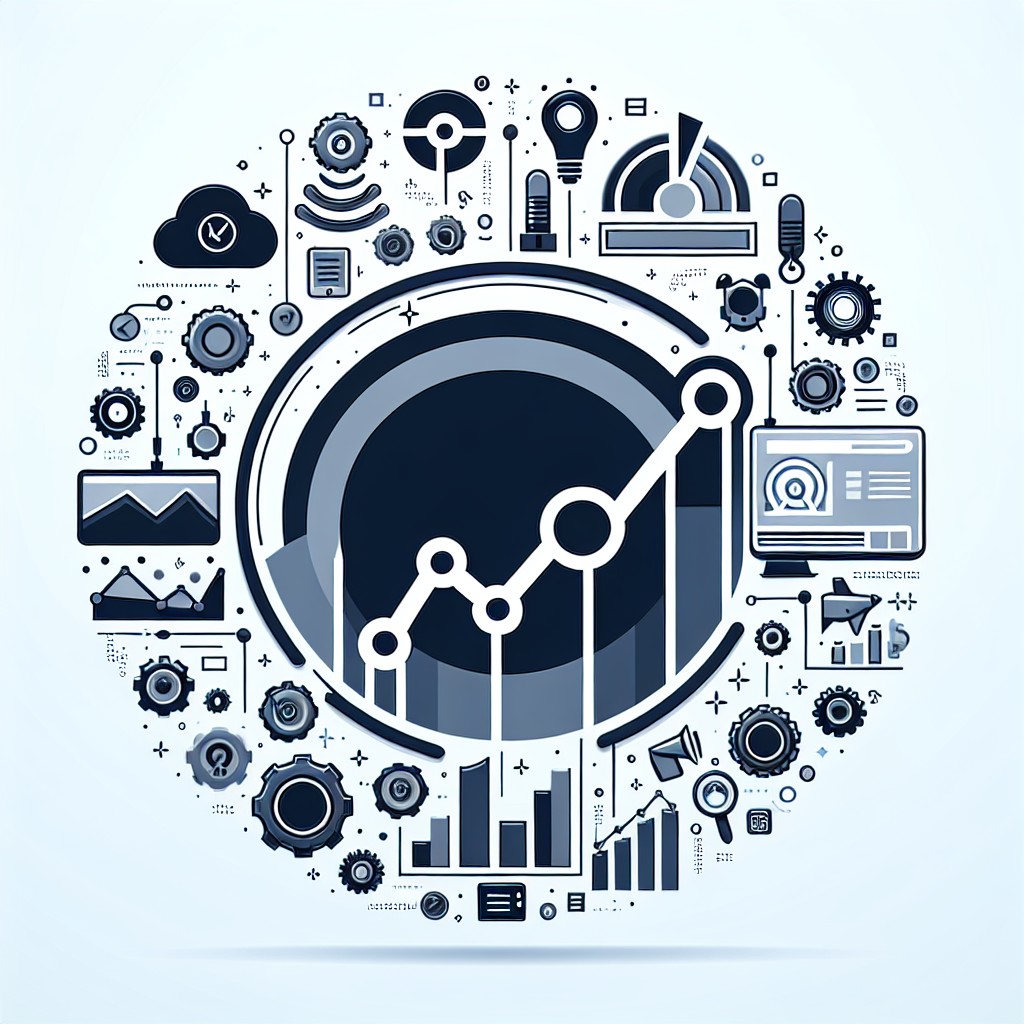