The Evolution of Marketing Analytics in a Privacy First World
The numbers didn’t make sense. As the analytics lead for his e-commerce platform, Jesse found himself staring at a dashboard showing conversion rates that had plummeted by 30% overnight. After hours of investigation, he uncovered the culprit: Apple’s iOS update had restricted tracking capabilities, causing their attribution model to crumble. What followed was a frantic week of meetings with stakeholders, as Jesse explained why their previously reliable performance metrics could no longer be trusted. That crisis became a turning point in his career. He dove into researching alternative measurement approaches, connecting with industry experts, and experimenting with new modeling techniques. What began as a tactical response to a technical challenge evolved into a deep exploration of how analytics must transform in the privacy era. Jesse came to realize that this disruption wasn’t just a technical inconvenience—it was an opportunity to build more robust, ethical, and ultimately more insightful measurement frameworks. The journey changed not only how he approached analytics but his entire philosophy about the relationship between data, privacy, and marketing effectiveness.
Introduction
Marketing analytics stands at a historic inflection point. The convergence of privacy regulations (GDPR, CCPA), platform restrictions (Apple's App Tracking Transparency, Google's Privacy Sandbox), and evolving consumer expectations has disrupted traditional measurement approaches that relied heavily on cross-site tracking, persistent identifiers, and individual-level behavioral monitoring.
This transformation is not merely a technical challenge but a fundamental shift in how marketing effectiveness is conceptualized and measured. According to Gartner, 40% of all consumer data used for digital marketing will be obtained and processed with explicit permission by 2023, up from 10% in 2020. This seismic shift demands new measurement paradigms that balance marketing accountability with privacy protection.
This article explores how marketing analytics is evolving to meet these challenges, examining emerging methodologies, technologies, and frameworks that enable effective measurement in a privacy-first world.
1. From Deterministic to Probabilistic Attribution
Traditional multi-touch attribution models relied on deterministic user identification—tracking individuals across touchpoints with cookies or device IDs. As these identifiers disappear, probabilistic approaches are gaining prominence.
Probabilistic attribution uses statistical modeling, machine learning, and aggregate data patterns to infer likely customer journeys without tracking individuals. According to Forrester Research, organizations implementing advanced probabilistic models can recover up to 85% of the measurement accuracy lost through privacy restrictions.
Marketing science professor Dr. Catherine Tucker notes that "probabilistic approaches actually force marketers to think more rigorously about causality rather than just correlation," potentially leading to more accurate insights despite using less granular data.
Case Study: Beauty retailer Sephora shifted from traditional multi-touch attribution to a probabilistic modeling approach following iOS privacy changes. By combining aggregated campaign data, store visitation patterns, and first-party customer insights, they developed a mixed media model that recovered 78% of lost measurement capabilities while remaining fully privacy-compliant. This transition actually improved their media allocation efficiency by 15% by eliminating attribution biases inherent in their previous last-click model.
2. Incrementality Testing and Experimental Design
As continuous tracking becomes less viable, controlled experiments are reemerging as the gold standard for measuring marketing effectiveness. Incrementality testing—measuring the lift generated by a marketing intervention versus a control group—provides causal evidence of impact without requiring individual-level tracking.
Google's research shows that brands implementing robust experimental designs can identify 20-30% of marketing spend that generates no incremental value, even without granular user-level data. As measurement expert Jim Novo observes, "Privacy restrictions are driving a renaissance in experimental design, which has always been the most reliable way to prove causality."
Case Study: Streaming service Spotify developed a geo-matched market testing program to measure campaign effectiveness without relying on user-level tracking. By comparing subscriber growth in demographically similar markets with different advertising exposures, they identified which channels drove true incremental growth. This approach revealed that certain digital channels previously credited with high performance under their attribution model were actually delivering minimal incremental value, leading to a reallocation of 30% of their acquisition budget.
3. Aggregated Reporting and Privacy-Enhancing Technologies
Privacy-enhancing technologies (PETs) are enabling measurement capabilities while protecting individual privacy. Techniques like differential privacy, federated analytics, and aggregated reporting systems add statistical noise or compute insights on decentralized data without centralizing raw information.
Facebook's Conversion API and Google's Aggregated Reporting API exemplify this approach, providing campaign performance data while adding privacy safeguards. According to McKinsey, organizations implementing PETs effectively can preserve up to 90% of the analytical value of raw data while dramatically reducing privacy risks.
Case Study: Financial services company American Express implemented a differential privacy framework for their marketing analytics that added calculated noise to datasets while maintaining statistical validity. This approach allowed them to continue personalizing offers and measuring campaign performance while enhancing privacy protections. The solution not only ensured regulatory compliance but actually improved targeting efficiency by 23% by focusing on patterns rather than individuals.
4. First-Party Data Ecosystems and Measurement Partnerships
As third-party measurement fragments, brands are developing first-party measurement infrastructures and strategic partnerships to close attribution gaps. Consumer data platforms (CDPs) that unify first-party interactions across touchpoints have seen 25% year-over-year growth according to Salesforce research.
Marketing technology expert Scott Brinker notes that "the winners in the privacy-first era will be organizations that build consensual data relationships directly with consumers rather than relying on intermediaries." These first-party relationships create the foundation for privacy-compliant measurement.
Case Study: Retailer Target built a comprehensive first-party measurement ecosystem through their Circle loyalty program, connecting online activity, in-store purchases, and app engagement with explicit customer consent. This unified system allowed them to continue measuring cross-channel customer journeys despite tracking restrictions. When combined with their clean room technology for secure data collaboration with advertisers, Target actually expanded their measurement capabilities while enhancing privacy protections, increasing both advertising revenue and customer trust metrics.
5. MMM Renaissance and AI-Enhanced Modeling
Marketing Mix Modeling (MMM)—a statistical analysis technique that measures the impact of marketing activities on sales outcomes—is experiencing a renaissance in the privacy era. Modern MMMs enhanced by machine learning and granular data inputs provide both strategic and tactical insights without individual tracking.
According to Analytic Partners, brands with mature MMM implementations are reallocating 20-30% of their marketing budgets annually based on effectiveness insights, driving 5-15% performance improvements. As analytics expert Avinash Kaushik observes, "The future of attribution isn't more granular tracking but smarter models that require less invasive data collection."
Case Study: CPG giant Unilever developed an AI-enhanced Marketing Mix Modeling system called "Demand Signal" that integrates traditional econometric modeling with machine learning and near real-time data ingestion. This approach provides both long-term strategic insights and tactical optimization guidance without relying on individual consumer tracking. The system identified $150 million in efficiency opportunities across their global portfolio while maintaining complete privacy compliance, demonstrating that sophisticated measurement doesn't require privacy compromises.
Conclusion
The privacy transformation is not the end of marketing analytics but its evolution. By embracing new methodologies—probabilistic modeling, experimental design, privacy-enhancing technologies, first-party ecosystems, and advanced marketing mix modeling—organizations can maintain or even enhance their measurement capabilities while respecting consumer privacy.
This shift may actually improve marketing effectiveness by eliminating the false precision of flawed attribution models and focusing on true incremental impact. As Harvard Business School professor John Deighton notes, "The most valuable insight is not which ad drove a specific purchase, but understanding how marketing collectively shapes consumer behavior over time."
The future of marketing analytics belongs to organizations that view privacy not as a constraint but as a catalyst for developing more robust, ethical, and ultimately more insightful approaches to measuring what matters.
Call to Action
For marketing leaders navigating the privacy-first measurement landscape:
- Audit your current analytics infrastructure to identify privacy vulnerabilities and measurement gaps
- Invest in experimental design capabilities and incrementality testing frameworks
- Develop a balanced measurement portfolio combining multiple methodologies rather than relying on a single attribution approach
- Build first-party data assets with explicit consent that enable owned measurement capabilities
- Prioritize analytical approaches that provide actionable insights rather than false precision
The organizations that transform their measurement approaches now will gain both competitive advantage and consumer trust in the privacy-first future, turning what appears to be a measurement crisis into an opportunity for more sophisticated marketing analytics.
Featured Blogs
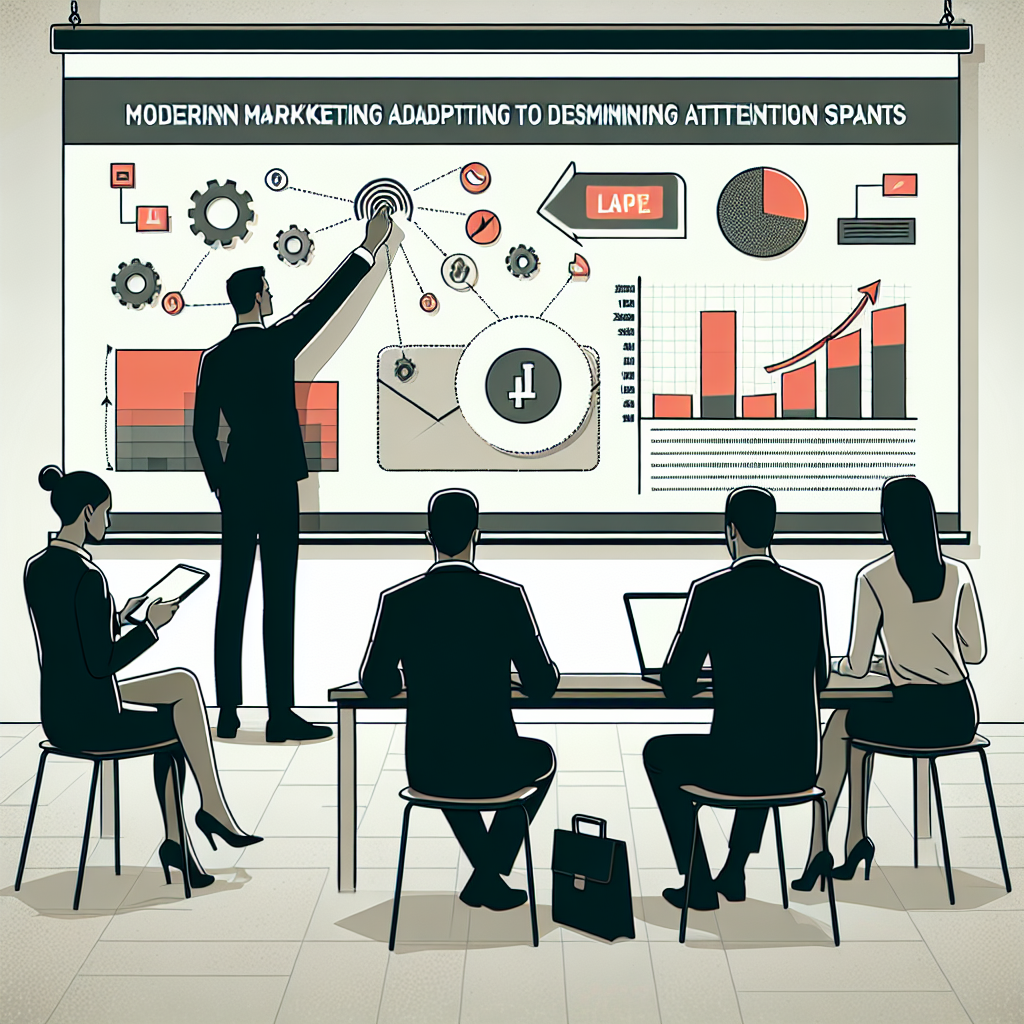
How the Attention Recession Is Changing Marketing
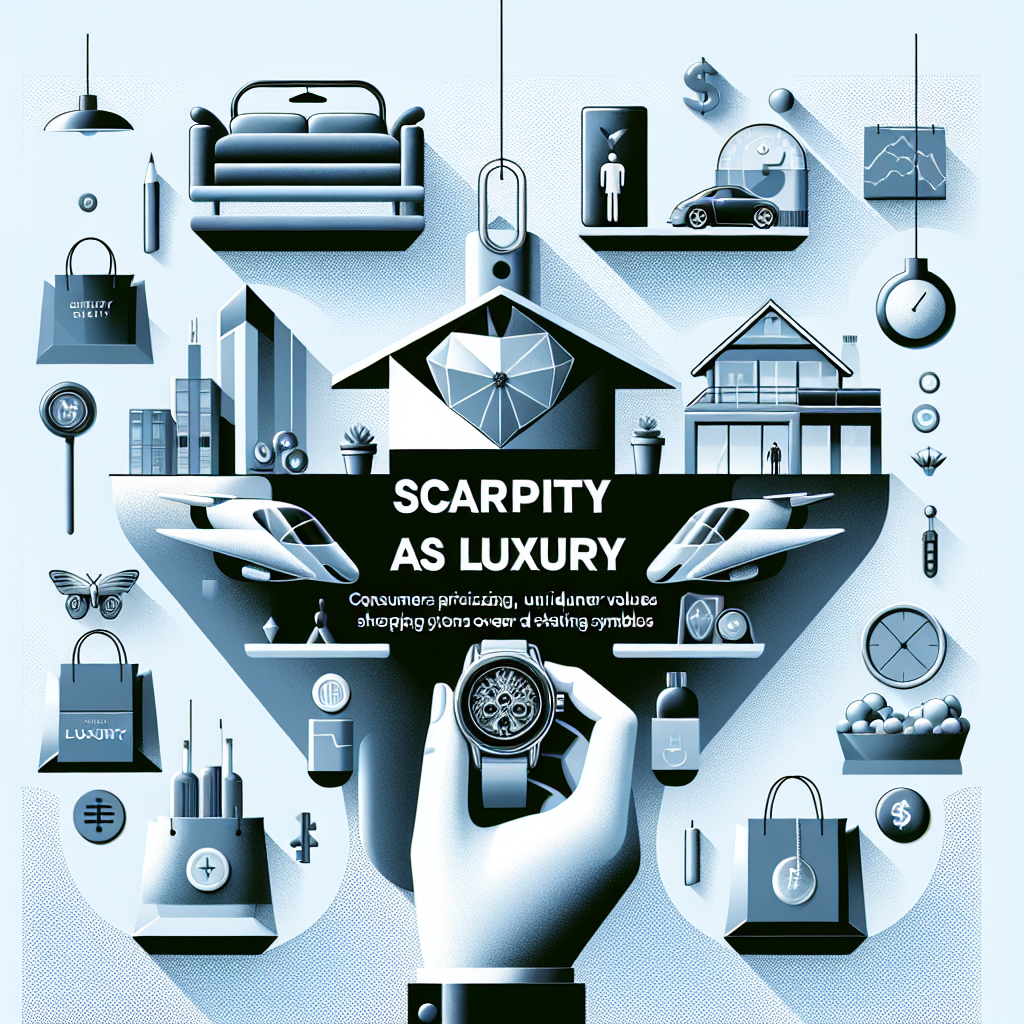
The New Luxury Why Consumers Now Value Scarcity Over Status
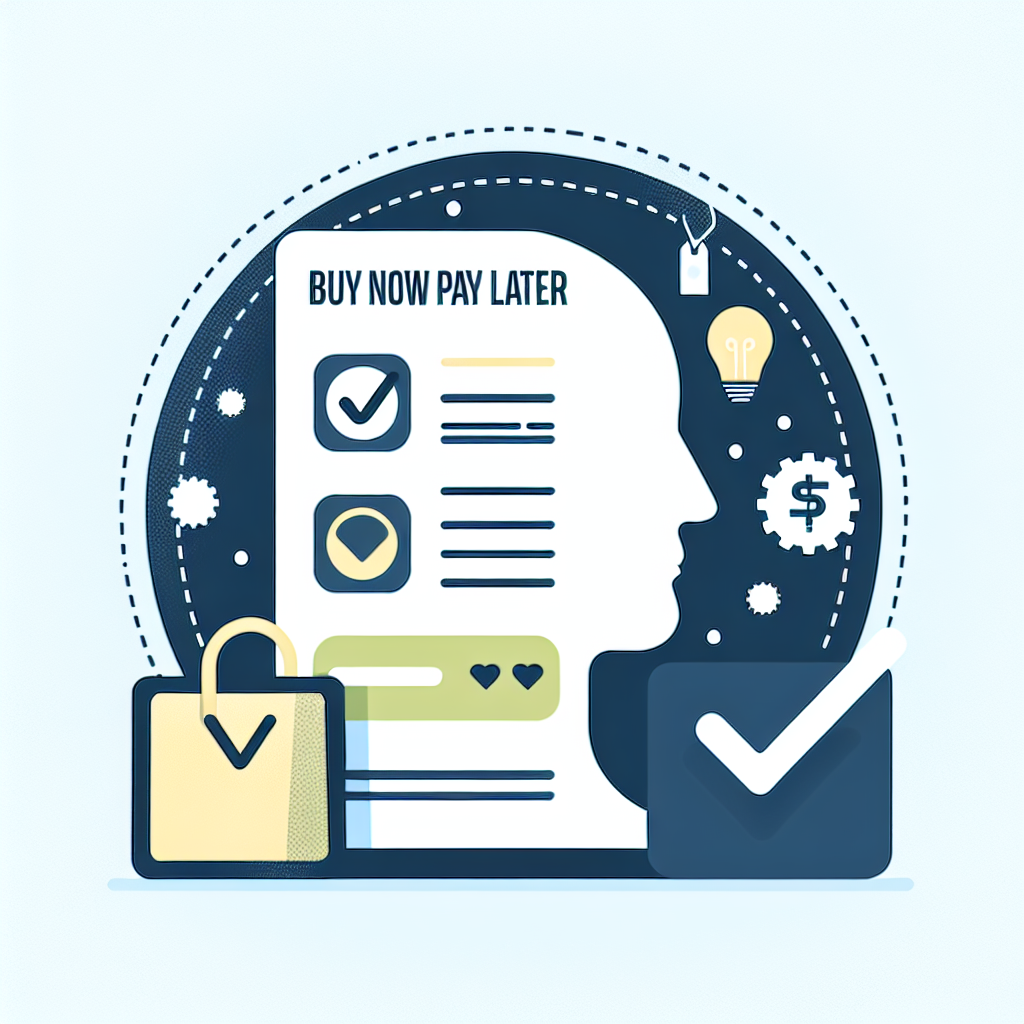
The Psychology Behind Buy Now Pay later
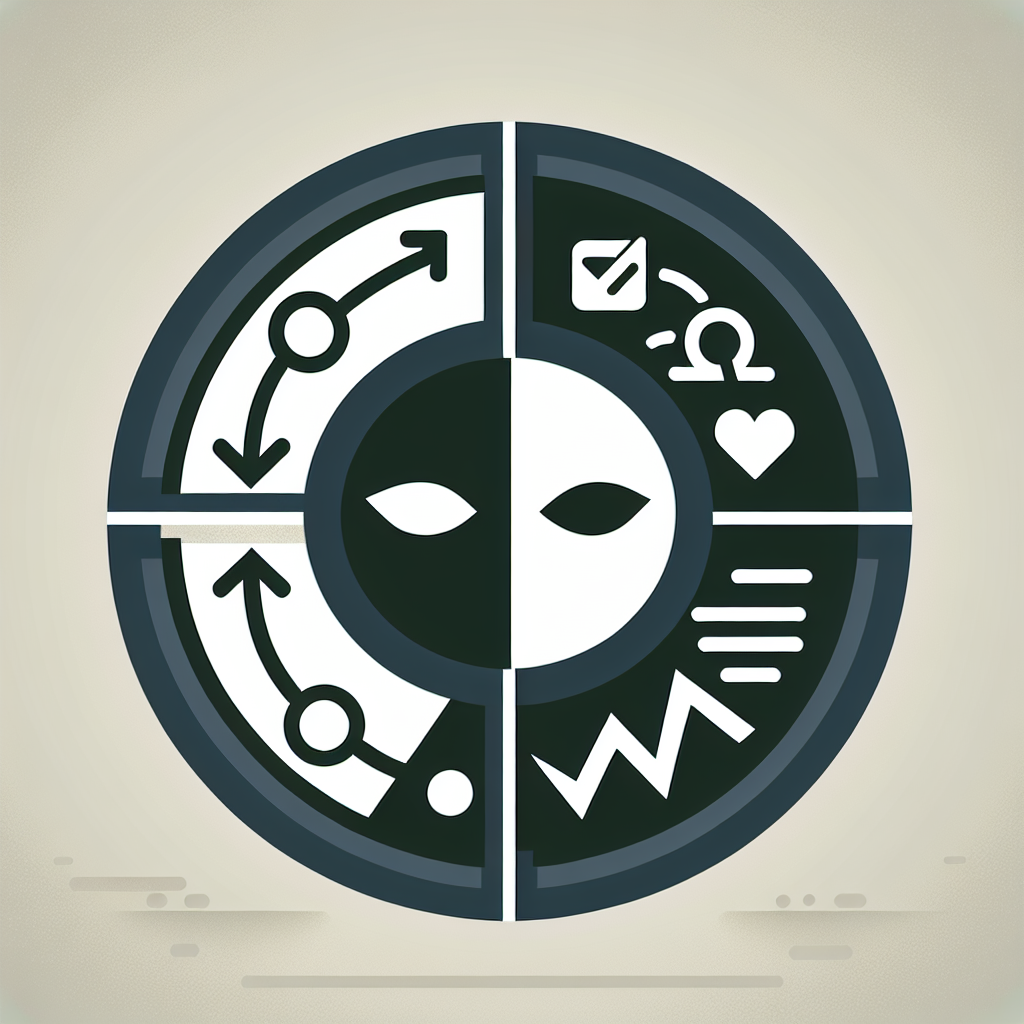
The Role of Dark Patterns in Digital Marketing and Ethical Concerns
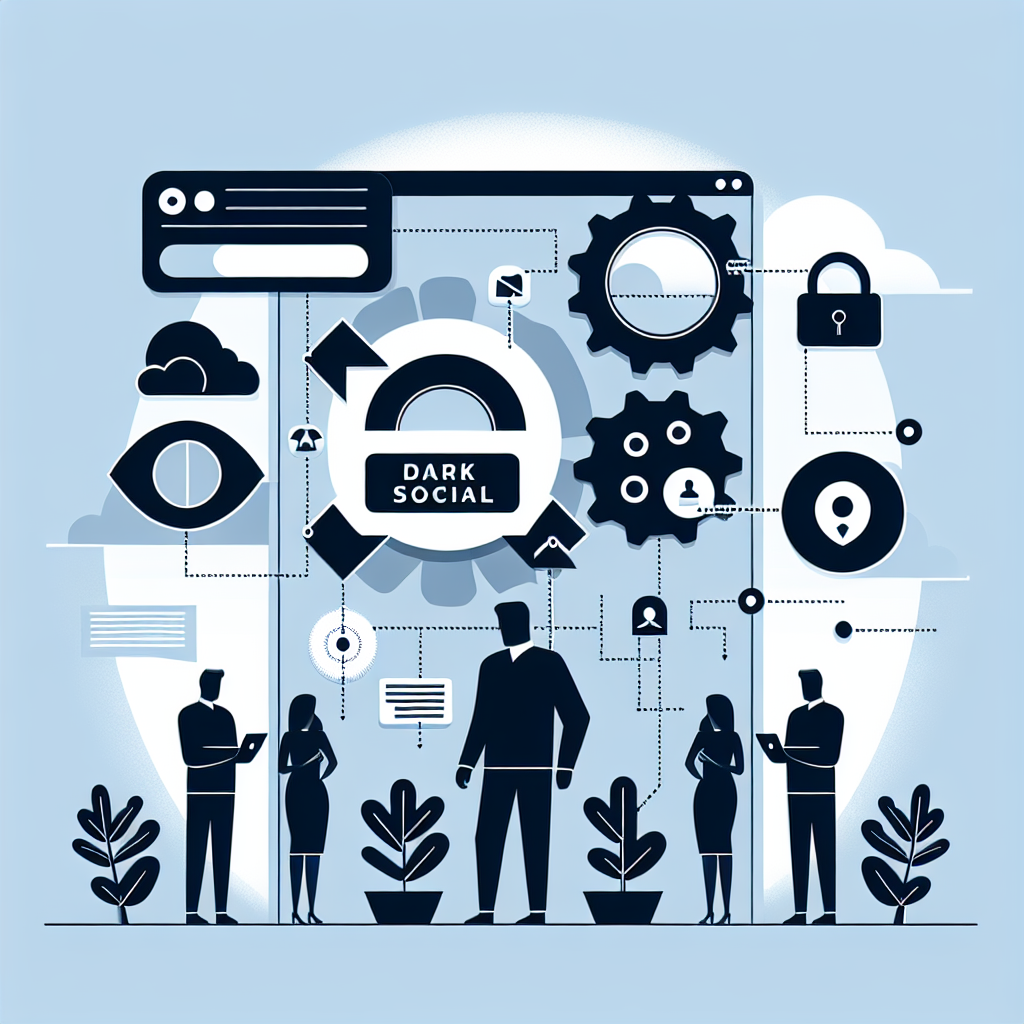
The Rise of Dark Social and Its Impact on Marketing Measurement
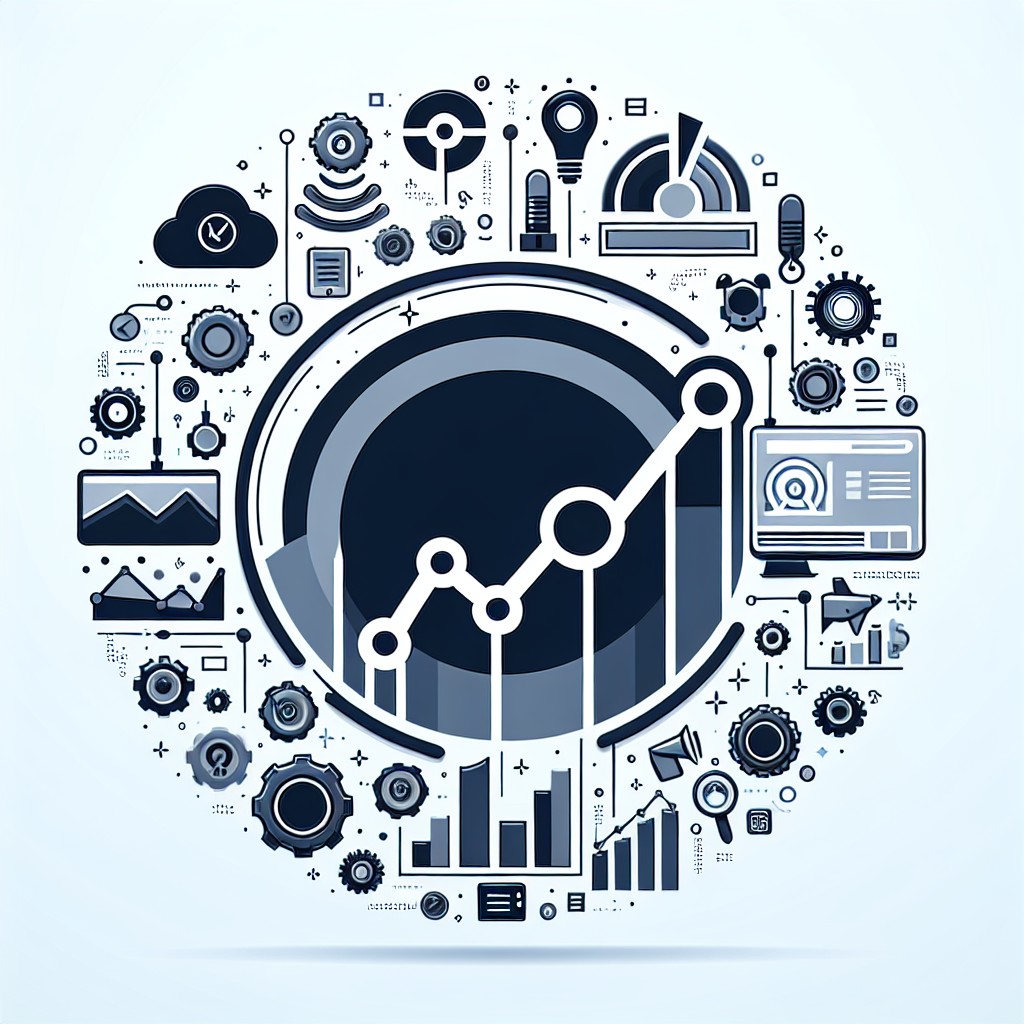