The Role of Data Co-ops in Privacy Compliant Audience Targeting
Jesse's fascination with data cooperatives began during a conference panel discussion when a retail marketing executive made a statement that stopped him in his tracks: "We're a mid-sized brand competing against giants with 100 times our customer data. Then we joined a retail data cooperative and suddenly had insights from 50 million shoppers instead of 500,000." What caught Jesse's attention wasn't just the scale but what she said next: "The magic wasn't that we could access more data—it was that we could derive insights from collective intelligence while never actually seeing anyone else's raw customer information." As someone who had spent years navigating the increasingly complex privacy landscape, this revelation sparked a profound curiosity in Jesse. Could data cooperatives offer the elusive middle path between the personalization consumers expect and the privacy they demand? His subsequent exploration into this model revealed a collaborative approach that might just represent the future of ethical audience targeting.
Introduction: Collaborative Data in a Privacy-First World
As third-party cookies fade into obsolescence and privacy regulations tighten globally, marketers face an unprecedented challenge: how to maintain audience targeting effectiveness while honoring heightened privacy expectations. This challenge is particularly acute for small and mid-sized organizations lacking the first-party data scale of digital giants.
Data cooperatives—collaborative arrangements where multiple entities contribute anonymized data to create collective intelligence—have emerged as a promising solution. Unlike third-party data aggregators, these cooperatives operate on principles of mutual value, explicit consent, and privacy by design. By pooling consented first-party data within secure frameworks, participants gain scale while maintaining higher ethical standards than traditional data marketplaces.
As the marketing ecosystem reconfigures around privacy, these collaborative models are evolving from niche experiments to mainstream infrastructure for privacy-compliant audience targeting.
1. The Structural Models: How Data Co-ops Work
Data cooperatives operate across a spectrum of models, each balancing data utility, privacy protection, and operational complexity:
a) Federated Analysis Systems The most privacy-preserving approach keeps raw data within organizational boundaries while sharing aggregated insights. Professor Lalana Kagal of MIT's Internet Policy Research Initiative explains, "Federated systems solve the fundamental privacy paradox—deriving collective intelligence without centralizing sensitive data."
LiveRamp's Data Marketplace implements this model, enabling retailers to access aggregated shopper insights without exposing individual transaction data.
b) Clean Room Cooperatives These models use secure computational environments where multiple organizations can analyze combined datasets without accessing underlying raw data. The Trade Desk's Unified ID 2.0 initiative exemplifies this approach, creating a collaborative identity framework with differential privacy guarantees.
c) Industry Data Commons Sector-specific cooperatives pool anonymized data to create industry-wide intelligence. The Trustworthy Accountability Group's Data Transparency initiative demonstrates this approach, enabling advertisers to collectively combat fraud while protecting proprietary data.
According to Winterberry Group research, participants in well-structured data cooperatives achieve 37% higher targeting accuracy compared to using their first-party data alone, while maintaining higher privacy standards than traditional third-party data.
2. Strategic Applications: Beyond Basic Targeting
Forward-thinking organizations leverage data cooperatives for applications beyond simple audience expansion:
a) Collaborative Customer Journey Mapping Cooperatives enable visibility into customer journeys across organizational boundaries. The UK's Open Banking initiative demonstrates this capability, allowing financial services providers to collaboratively map customer financial journeys while maintaining strict data separation.
b) Privacy-Preserving Look-alike Modeling Cooperatives enable more robust predictive modeling without exposing sensitive data. The Advertising ID Consortium uses this approach to help publishers identify valuable audiences without relying on third-party cookies.
c) Ethical AI Training By accessing diverse datasets, organizations can train more accurate, less biased AI models. As Professor Avi Goldfarb of the University of Toronto notes, "The artificial intelligence revolution is fundamentally a data revolution, and cooperatives democratize access to that essential resource."
Mars, Inc.'s participation in the Consumer Goods Forum data cooperative exemplifies this benefit, enabling the company to build more accurate demand forecasting models by incorporating anonymized data from complementary product categories.
3. Governance Frameworks: Trust as Infrastructure
The effectiveness of data cooperatives depends on robust governance ensuring participant trust. Three governance models have proven effective:
a) Industry Consortium Approach Organizations in a sector establish collective standards for data sharing. The Partnership for Responsible Addressable Media (PRAM) exemplifies this model, creating industry-wide standards for identity resolution.
b) Third-Party Mediation Independent entities provide governance and technical infrastructure. InfoSum's data collaboration platform demonstrates this approach, serving as a neutral intermediary ensuring no raw data exchange.
c) Distributed Governance Blockchain and similar technologies enable trustless data cooperation. The Brave browser's Basic Attention Token ecosystem illustrates this model, using distributed ledger technology to enable privacy-preserving advertising without centralized control.
Deloitte's research indicates organizations participating in cooperatives with robust governance frameworks see 43% higher consumer trust scores and 27% higher consent rates than those using traditional data-sharing approaches.
4. The Emerging Ecosystem: From Competition to Coopetition
The cooperative data landscape continues to evolve through novel partnership structures:
a) Vertical Collaborations Organizations across supply chains form data alliances. Carrefour and Unilever's data partnership shows how retailers and manufacturers can collaboratively derive insights while respecting consumer privacy.
b) Competitive Cooperatives Even direct competitors collaborate on data where mutual benefit exists. The Advertiser Protocol initiative allows competing brands to collectively combat ad fraud without exposing strategic data.
Professor Marshall Van Alstyne of Boston University, known for platform economics research, observes that "data cooperatives represent a fundamental shift from pure competition to 'coopetition,' where organizations compete on services but cooperate on data infrastructure."
5. Future Directions: Technological Enablers
Emerging technologies are expanding the potential of data cooperatives:
a) Homomorphic Encryption This technology allows computation on encrypted data without decryption. Google's Privacy Sandbox incorporates these techniques to enable targeting without exposing individual data.
b) Zero-Knowledge Proofs These cryptographic methods prove statements without revealing underlying data. Mastercard's Tourism Insights platform uses this approach to share tourism trends without exposing sensitive transaction data.
c) Synthetic Data Generation AI creates statistically representative but non-real user data. Microsoft and the NHS's cooperative synthetic health data initiative demonstrates how sensitive data can be utilized while protecting individual privacy.
Conclusion: Collaborative Intelligence in the Privacy Era
As the marketing ecosystem navigates the complex balance between personalization and privacy, data cooperatives offer a promising middle path. By enabling organizations to derive collective intelligence without centralizing sensitive data, these models transform privacy from a constraint into a catalyst for collaboration.
As Professor Shoshana Zuboff of Harvard Business School observes, "The next era of digital marketing won't be defined by who has the most data, but rather by who can create the most trusted frameworks for collaborative intelligence while respecting individual autonomy."
Organizations that embrace cooperative approaches will discover that privacy and personalization need not be opposing forces—they can be complementary aspects of a more sustainable, ethical approach to audience targeting.
Call to Action
For marketing leaders navigating the privacy-first landscape: • Assess potential cooperative data opportunities within your industry or across complementary sectors • Evaluate governance frameworks that would provide sufficient trust guarantees for your organization and customers • Consider pilot programs with trusted partners before full-scale implementation • Invest in technical and legal expertise specific to privacy-preserving data collaboration
The organizations that approach data not as proprietary assets to be hoarded but as collective resources to be responsibly leveraged will establish sustainable competitive advantages in the privacy-first future—advantages built on trust, collaboration, and mutual value rather than surveillance and exploitation.
Featured Blogs
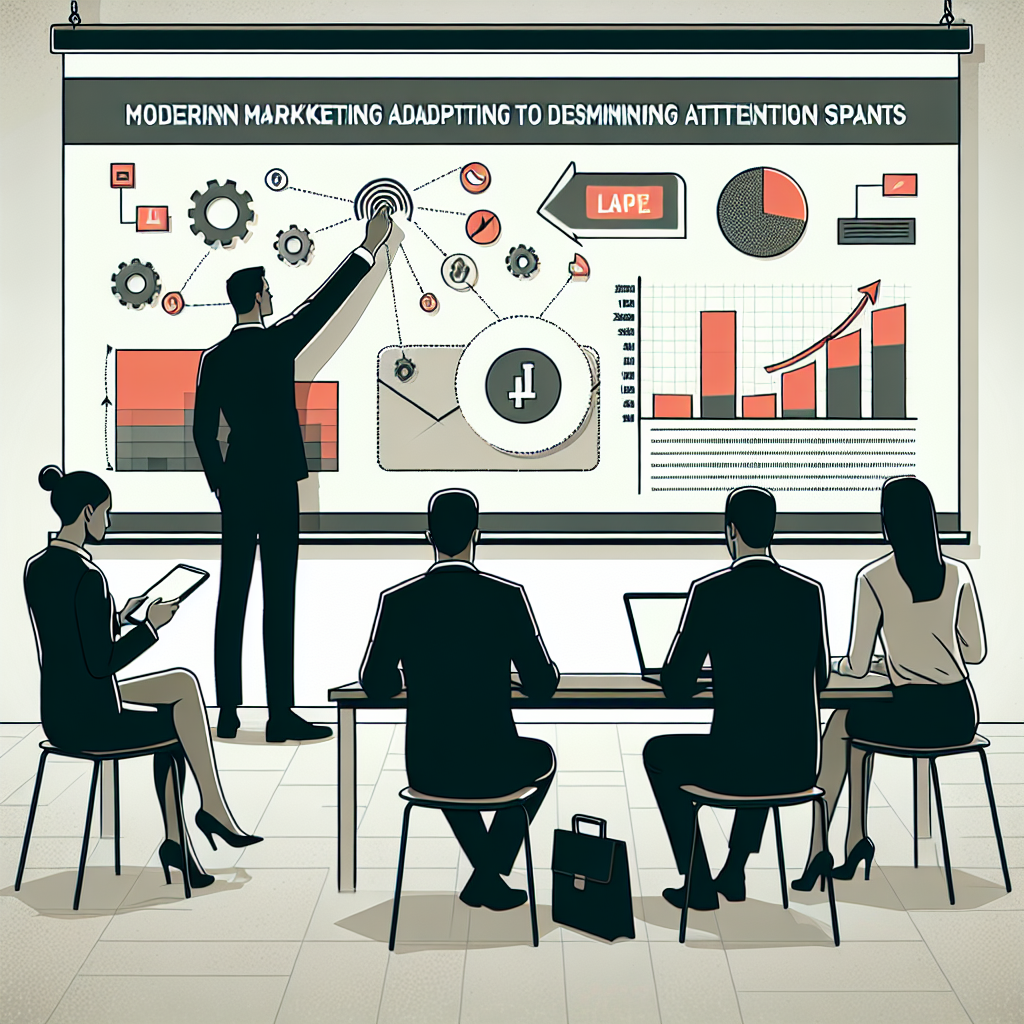
How the Attention Recession Is Changing Marketing
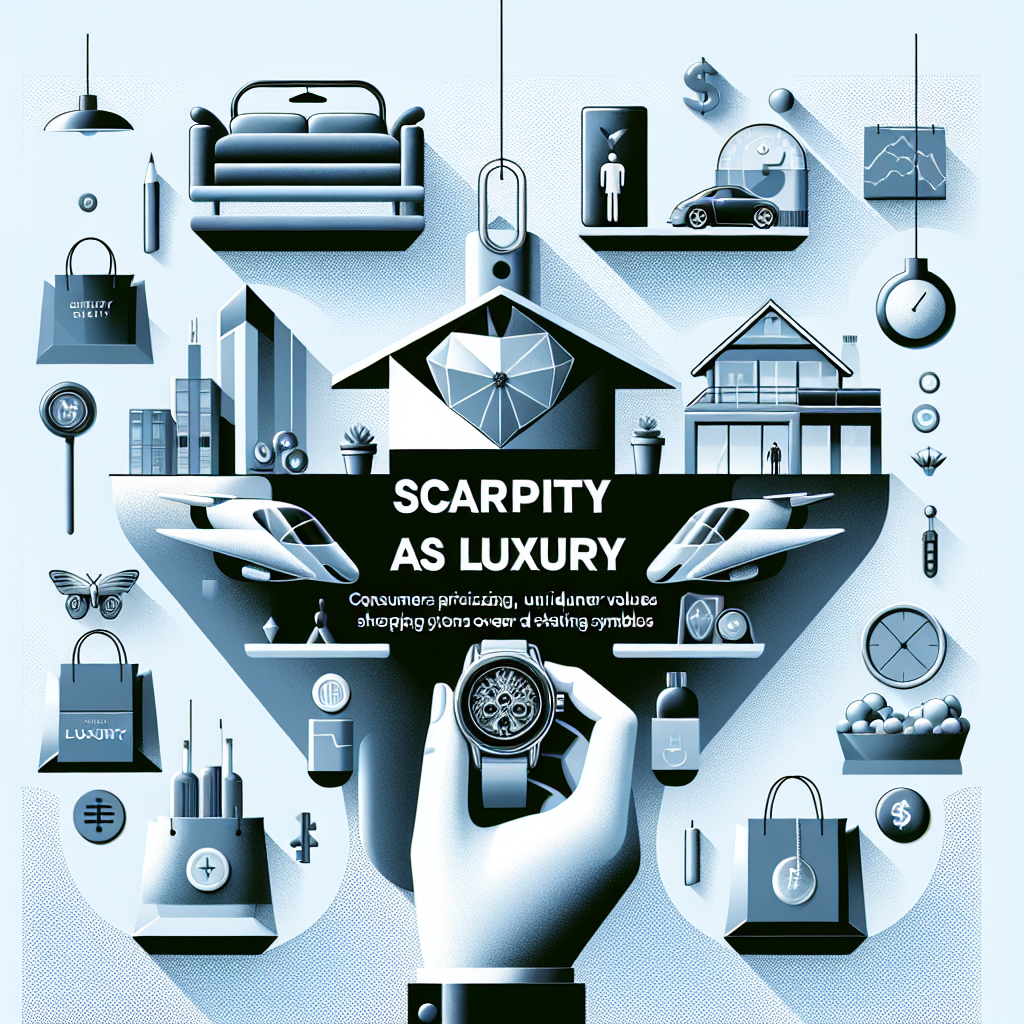
The New Luxury Why Consumers Now Value Scarcity Over Status
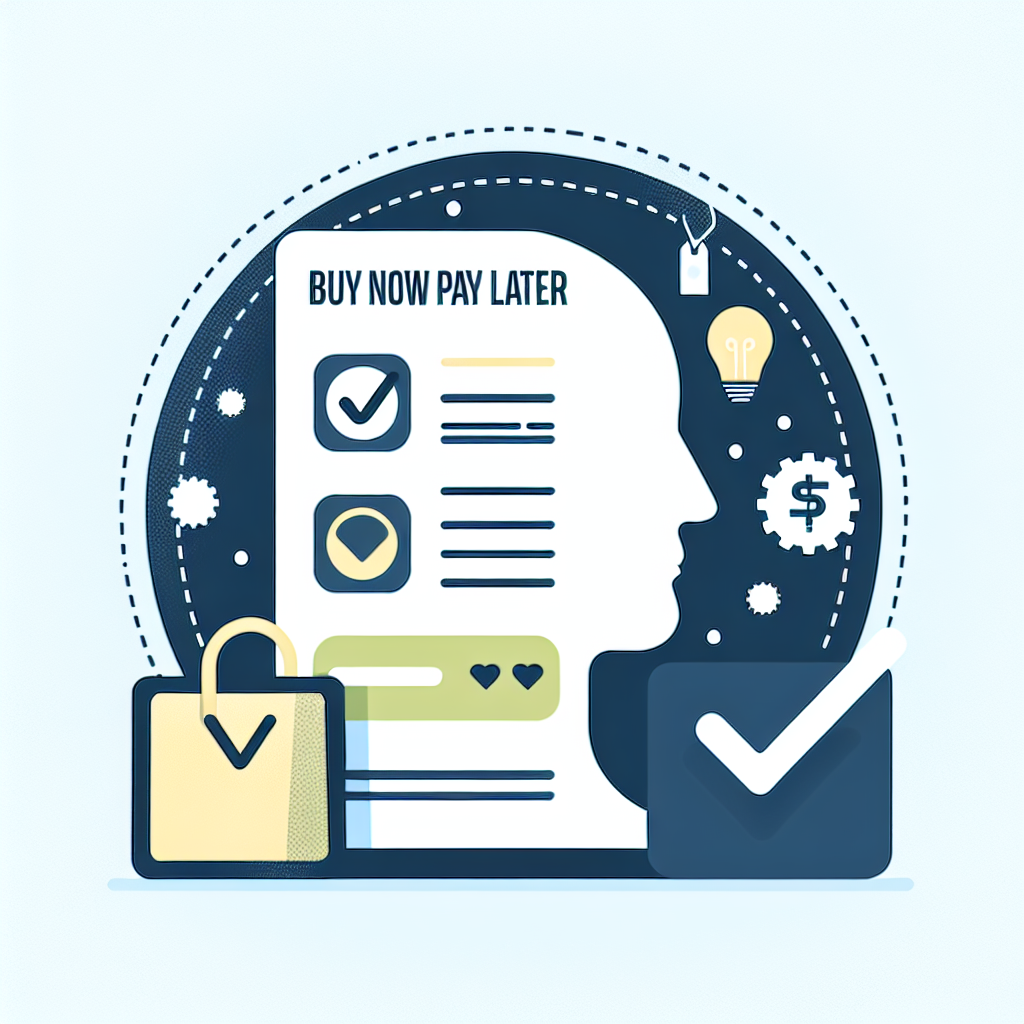
The Psychology Behind Buy Now Pay later
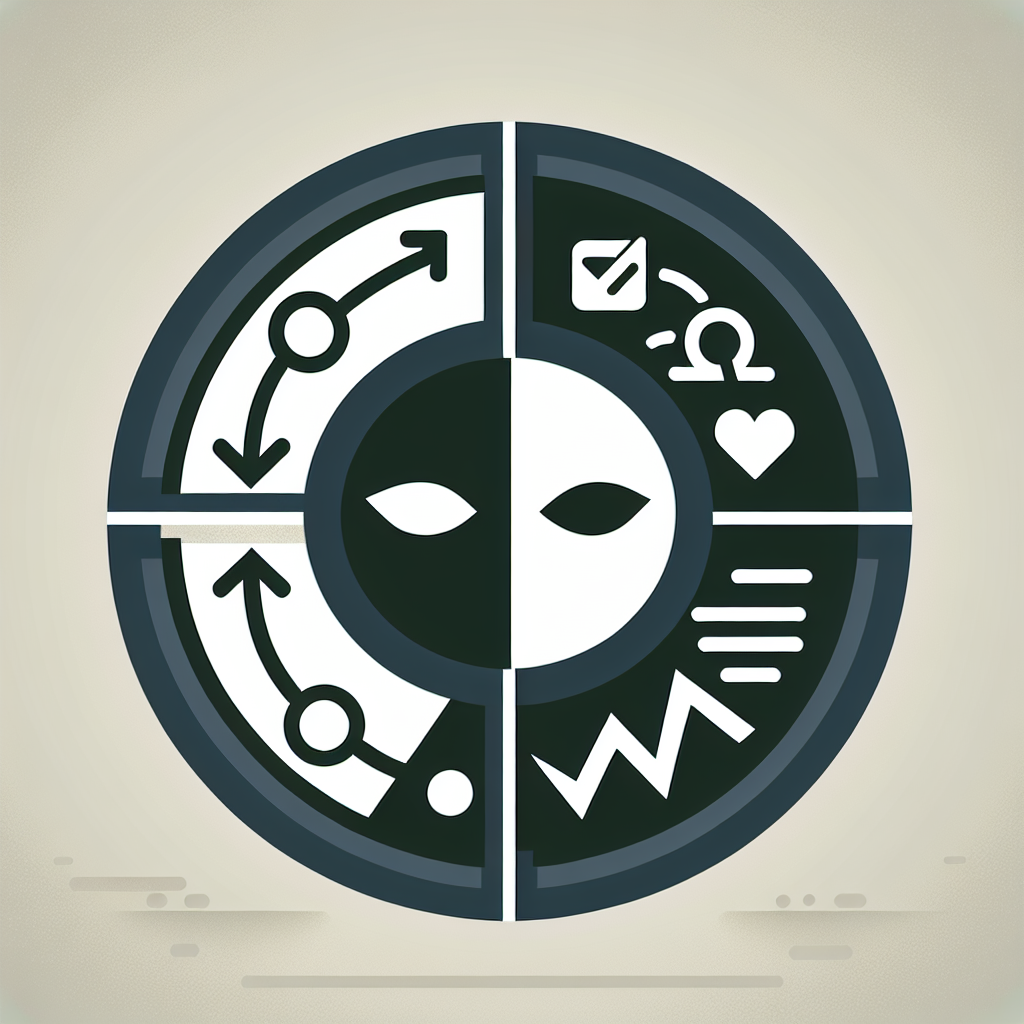
The Role of Dark Patterns in Digital Marketing and Ethical Concerns
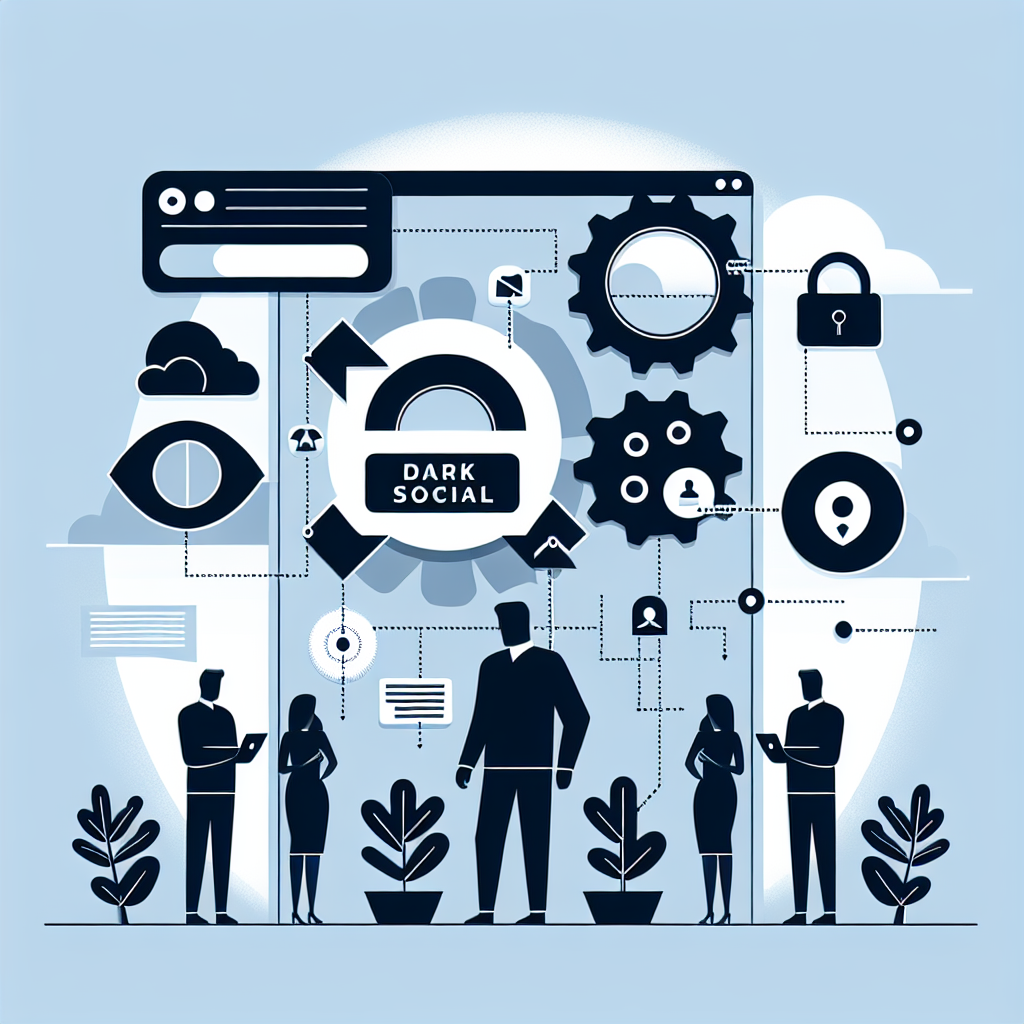
The Rise of Dark Social and Its Impact on Marketing Measurement
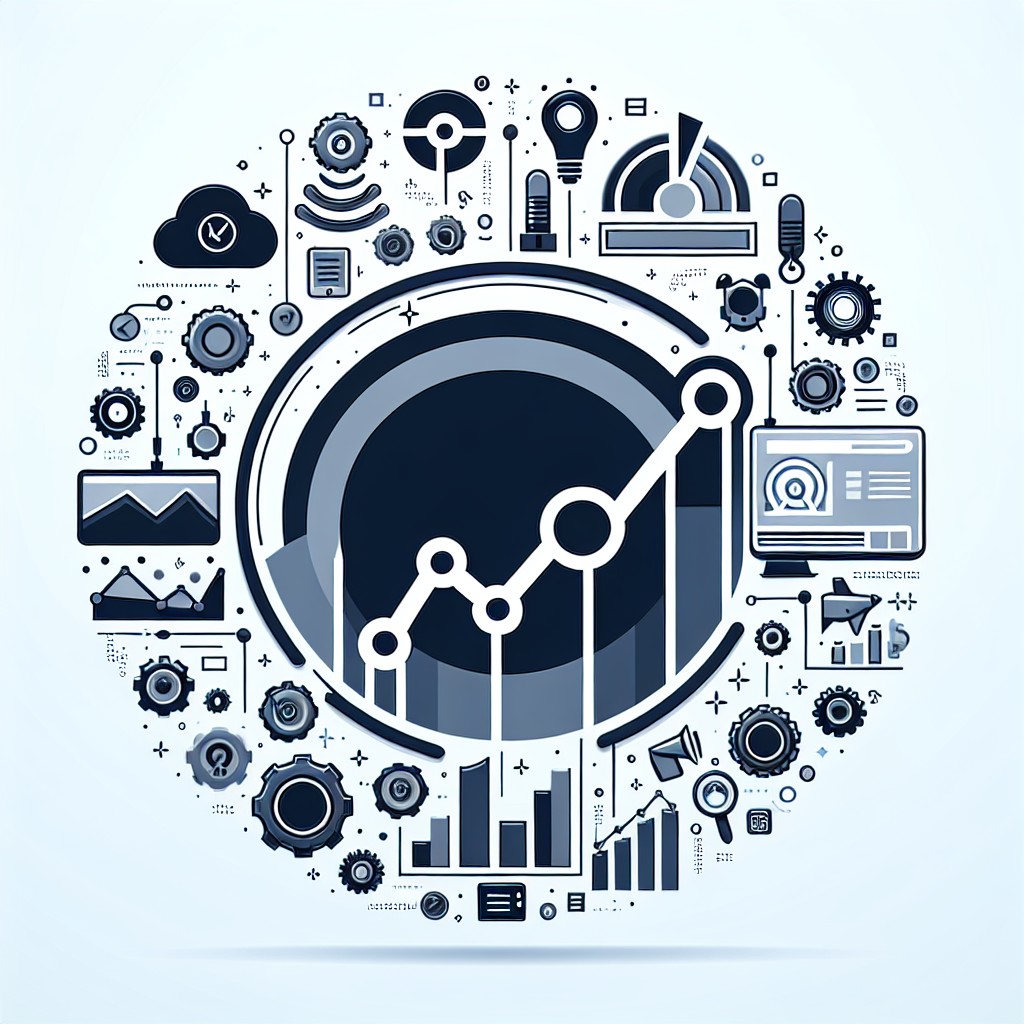