How AI Can Enhance Retail Shelf Optimization & Shopper Engagement
Introduction: The Transformation of Physical Retail Spaces
Traditional retail shelf management—based on planograms, category captain recommendations, and periodic resets—increasingly falls short in today's dynamic retail environment. With 85% of purchase decisions still made in-store (POPAI) despite e-commerce growth, physical shelf space remains a critical but underoptimized asset. Artificial intelligence is revolutionizing retail merchandising by transforming static shelves into dynamic, responsive selling environments that adapt to shopper behavior in real-time. As Professor Katherine Cullen, Senior Director of Consumer Insights at the National Retail Federation notes, "We're witnessing the evolution of shelves from passive product displays to active selling platforms powered by intelligence." This transformation coincides with the convergence of computer vision, IoT sensor networks, and advanced analytics capabilities. By leveraging machine learning to optimize product placement, detect engagement patterns, and personalize the shopping experience, retailers can significantly enhance both operational efficiency and shopper engagement. This article examines how AI is reimagining retail shelf optimization, its key applications, implementation approaches, challenges, and the future of intelligent merchandising systems.
1. AI-Driven Shelf Intelligence: Beyond Traditional Planogramming
Artificial intelligence fundamentally transforms shelf management methodology:
a) Computer Vision & Real-Time Monitoring AI analysis of shelf conditions enables continuous optimization:
- Automated out-of-stock detection reducing lost sales opportunities
- Planogram compliance verification through image recognition
- Shopper interaction analysis revealing engagement patterns
b) Dynamic Space Allocation & Product Positioning Machine learning models optimize product placement continuously:
- Sales velocity algorithms adjusting facings based on performance
- Cross-purchase pattern analysis for complementary positioning
- Temporal demand forecasting for anticipatory merchandising
c) Personalized Shelf Experiences AI creates individualized shopping environments:
- Digital shelf labels adapting messaging to detected demographics
- Attention pattern analysis optimizing key product visibility
- Environmental factor correlation with purchase behavior
Dr. Herb Sorensen, retail scientist and author of "Inside the Mind of the Shopper," observes that "AI-powered shelf optimization acknowledges the fundamental reality that physical retail is not about selling things to people, but about people buying things."
2. Key Applications Across Retail Environments
AI shelf optimization manifests across multiple strategic approaches:
a) Intelligent Category Management Data-driven optimization of category performance: Example: Kroger's implementation of Microsoft's AI-powered "Connected Store" technology optimizes shelf space allocation based on real-time sales data and shopper movement patterns, increasing category performance by 18% and reducing stockouts by 30%.
b) Interactive Shelf Systems Technology-enhanced shelf engagement: Example: Lowe's deployment of smart shelf solutions with real-time product comparison capabilities and personalized recommendations resulted in a 24% increase in high-margin product selection and 12% higher average basket size.
c) Dynamic Pricing Integration Shelf-level pricing optimization based on contextual factors: Example: Carrefour's AI shelf system analyzes traffic patterns, inventory levels, and competitive pricing to adjust digital shelf labels, delivering 7.2% margin improvement while maintaining price perception.
d) Personalized Discovery Experiences Tailored product highlighting based on shopper profiles: Example: Sephora's Beauty Hub uses facial recognition to identify customer demographics and preferences, then highlights recommended products through subtle illumination changes, increasing trial rates by 32%.
3. The Business Impact: Quantifying AI Shelf Optimization Value
Organizations implementing AI-powered shelf systems report significant improvements:
- 23% average reduction in out-of-stock situations (McKinsey)
- 15% increase in sales for optimally positioned products
- 27% improvement in promotion effectiveness through placement optimization
- Substantial enhancements in inventory turns and space productivity
Case Study: Walgreens' Smart Shelf Implementation Walgreens' cooler doors with embedded AI demonstrated:
- 18% lift in featured product sales through dynamic merchandising
- 11% reduction in energy costs through traffic-based door illumination
- Enhanced cross-category purchase connections through path analysis
According to Alex Gourlay, former co-COO of Walgreens Boots Alliance, "AI-powered shelf optimization has transformed our approach to merchandising from periodic resets to continuous optimization, fundamentally altering the economics of physical retail."
4. Implementation Challenges and Considerations
Despite compelling results, AI shelf optimization faces obstacles:
a) Technical Infrastructure Requirements
- Camera and sensor network installation costs
- Edge computing capabilities for real-time analysis
- Integration complexity with existing inventory systems
b) Data Integration & Analysis Complexity
- Unifying online and offline shopper behavior data
- Attribution modeling in multi-touchpoint environments
- Data quality challenges in busy retail environments
c) Organizational Adaptation Needs
- Merchant team workflow transformation
- Supplier relationship management in dynamic environments
- Cross-functional alignment between operations and merchandising
d) Privacy and Shopper Experience Balancing
- Transparent data collection practices
- Non-intrusive monitoring technologies
- Value exchange clarity for personalization features
5. The Future of AI-Enhanced Shelf Optimization
Intelligent shelf systems are evolving beyond current implementations toward:
a) Autonomous Merchandising Systems
- Self-organizing shelves adjusting to performance metrics
- Robotic restocking based on real-time demand signals
- Continuous experimentation driving ongoing optimization
b) Immersive Mixed-Reality Shelf Experiences
- Augmented reality overlays enhancing physical products
- Virtual product extensions beyond physical constraints
- Gesture-based interaction with intelligent shelf systems
c) Emotional Response Integration
- Sentiment analysis informing product positioning decisions
- Mood-responsive merchandising adapting to emotional contexts
- Subconscious response measurement influencing placement
d) Ecosystem Connectivity & Omnichannel Synchronization
- Shelf-mobile integration creating seamless experiences
- Consistent merchandising logic across physical and digital
- Total ecosystem optimization beyond individual stores
Conclusion: Navigating the Intelligent Shelf Revolution
AI-powered shelf optimization represents not merely an incremental improvement in retail merchandising but a fundamental reimagining of how physical retail spaces function. By transforming static shelves into responsive, intelligent selling platforms, retailers can create more engaging shopping experiences while simultaneously improving operational efficiency. Organizations that develop comprehensive AI shelf strategies gain competitive advantages through enhanced product visibility, improved category performance, and more memorable shopper experiences. However, successful implementation requires thoughtful consideration of technical requirements, organizational readiness, and shopper experience design. As intelligent shelf technology evolves from basic applications to sophisticated merchandising systems, retailers must develop new competencies in real-time analytics, experience design, and continuous optimization.
Call to Action
For retail leaders exploring AI-powered shelf optimization:
- Conduct an audit of current merchandising performance metrics and pain points
- Develop pilot programs with clearly defined success metrics in high-opportunity categories
- Create cross-functional implementation teams connecting merchandising, operations, and technology
- Invest in customer education to drive adoption and comfort with intelligent shelf features
Featured Blogs
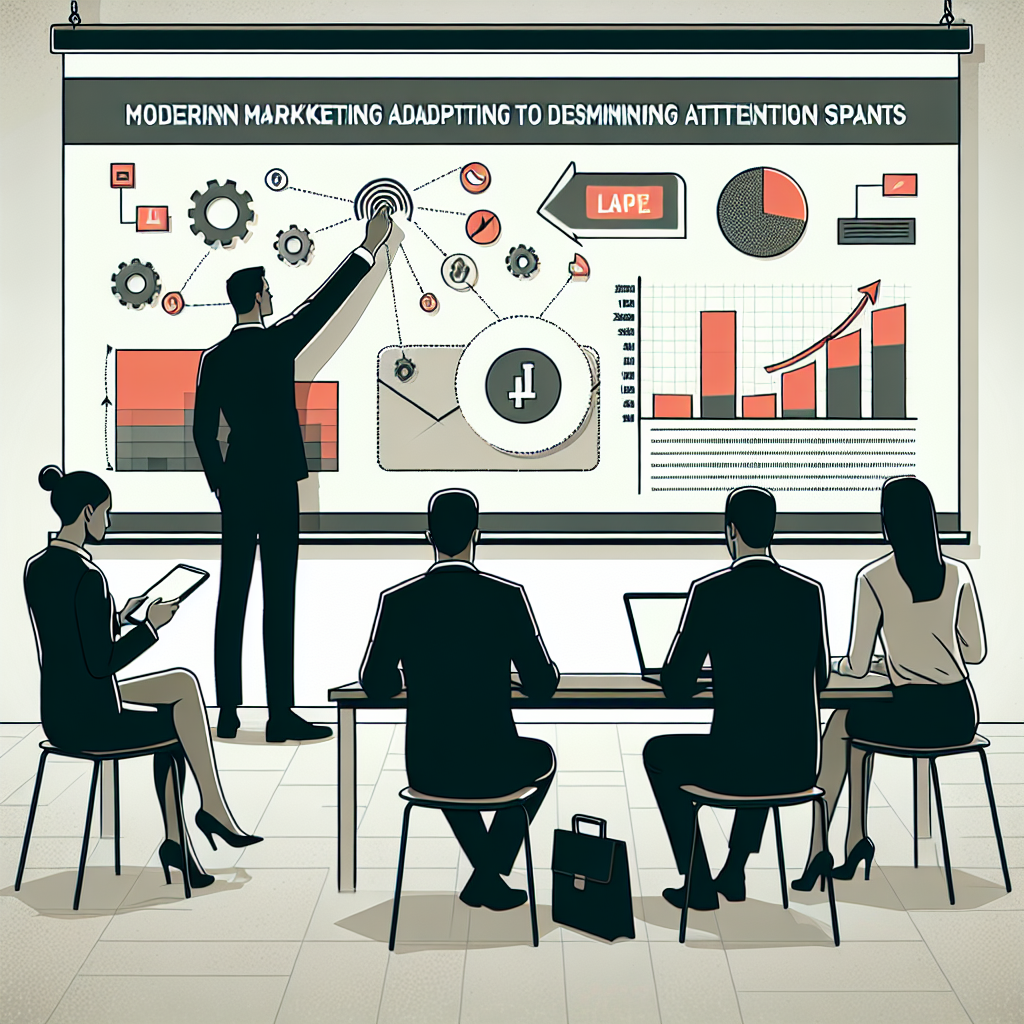
How the Attention Recession Is Changing Marketing
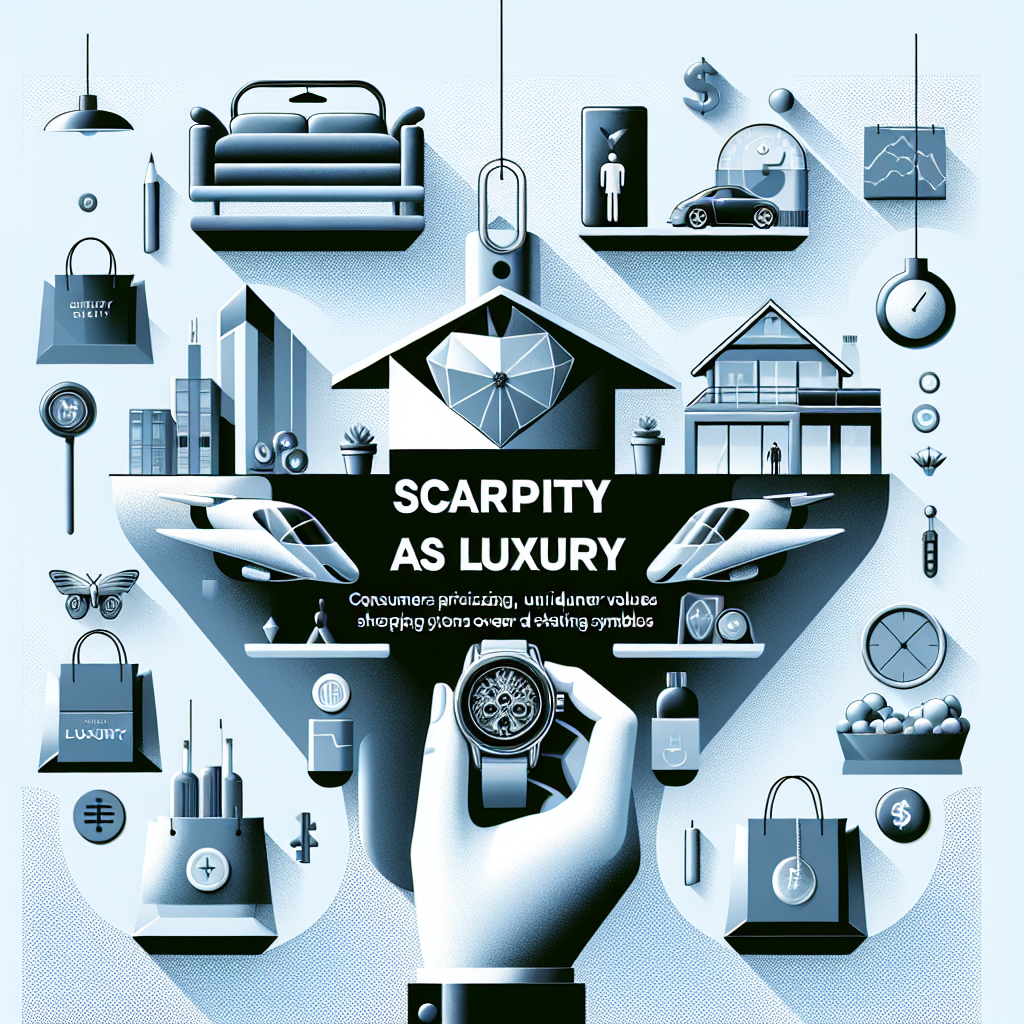
The New Luxury Why Consumers Now Value Scarcity Over Status
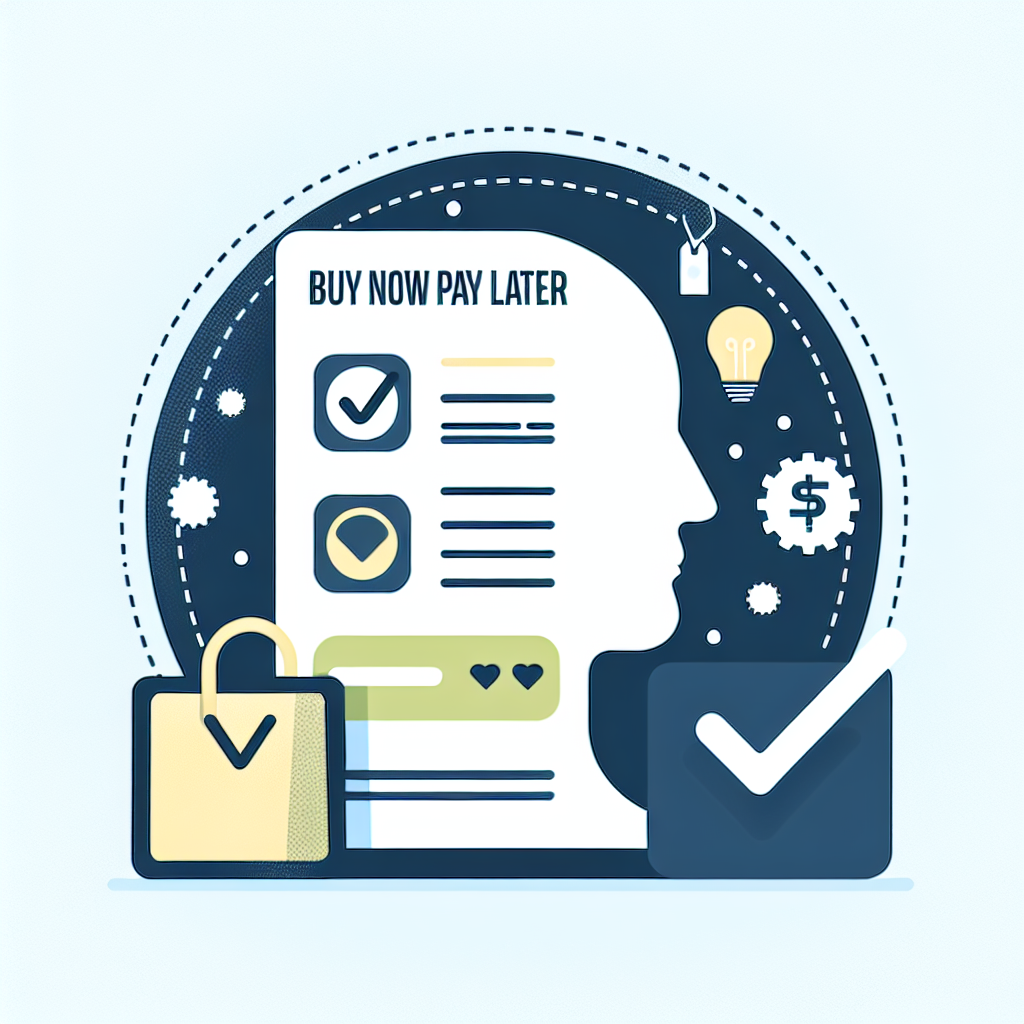
The Psychology Behind Buy Now Pay later
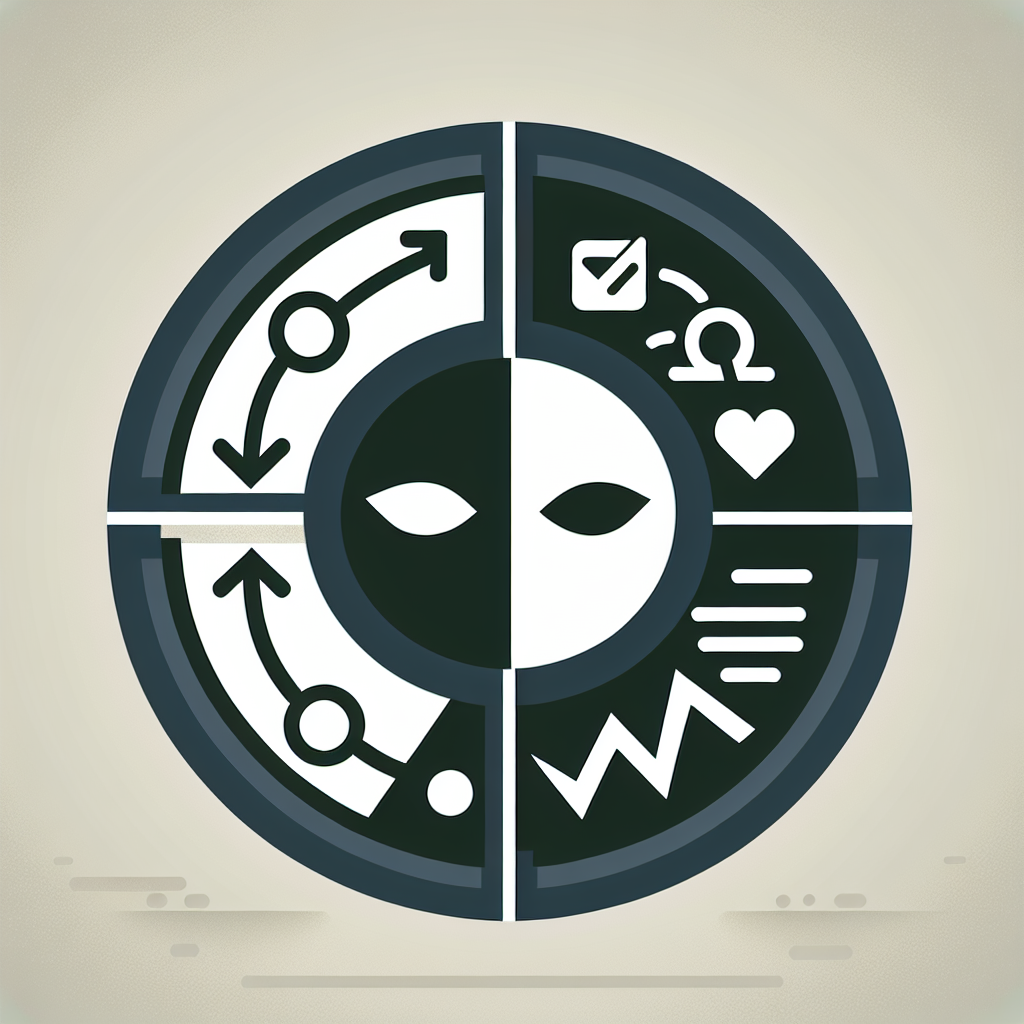
The Role of Dark Patterns in Digital Marketing and Ethical Concerns
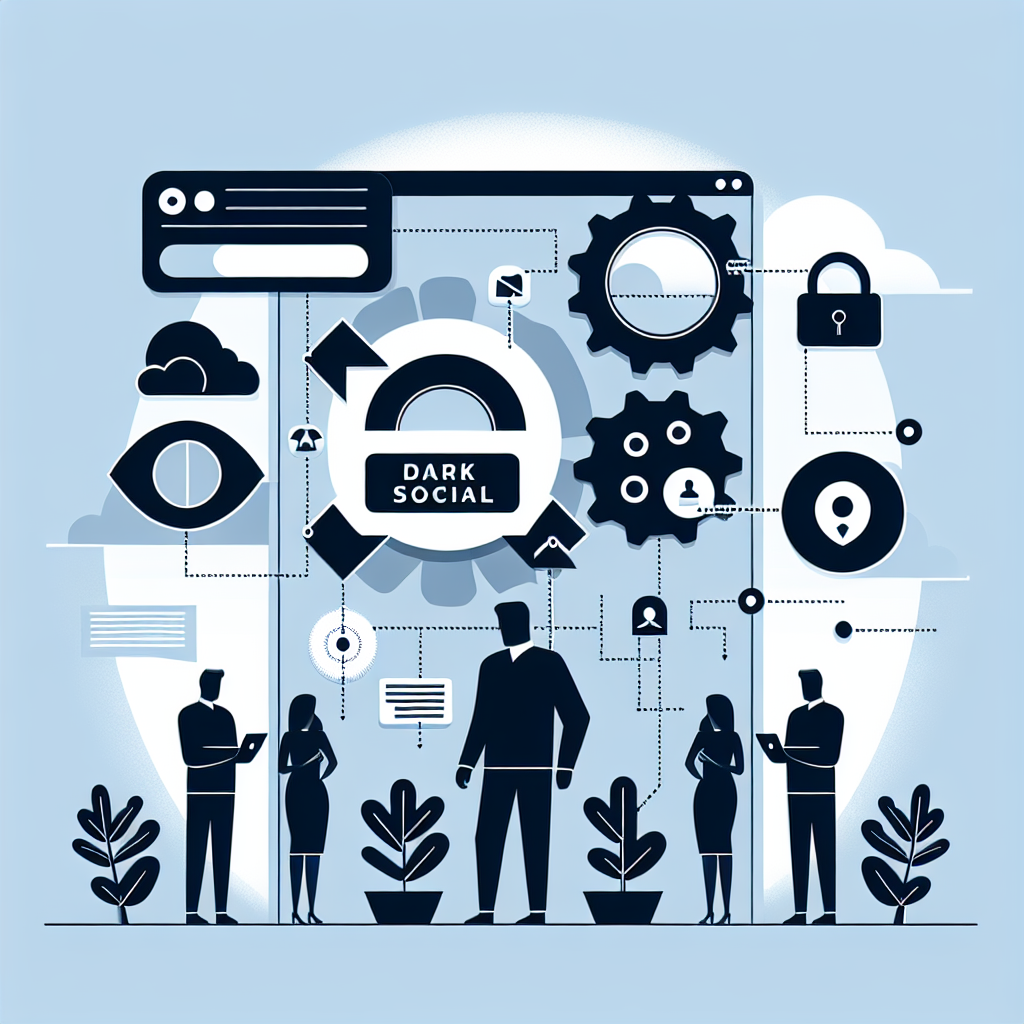
The Rise of Dark Social and Its Impact on Marketing Measurement
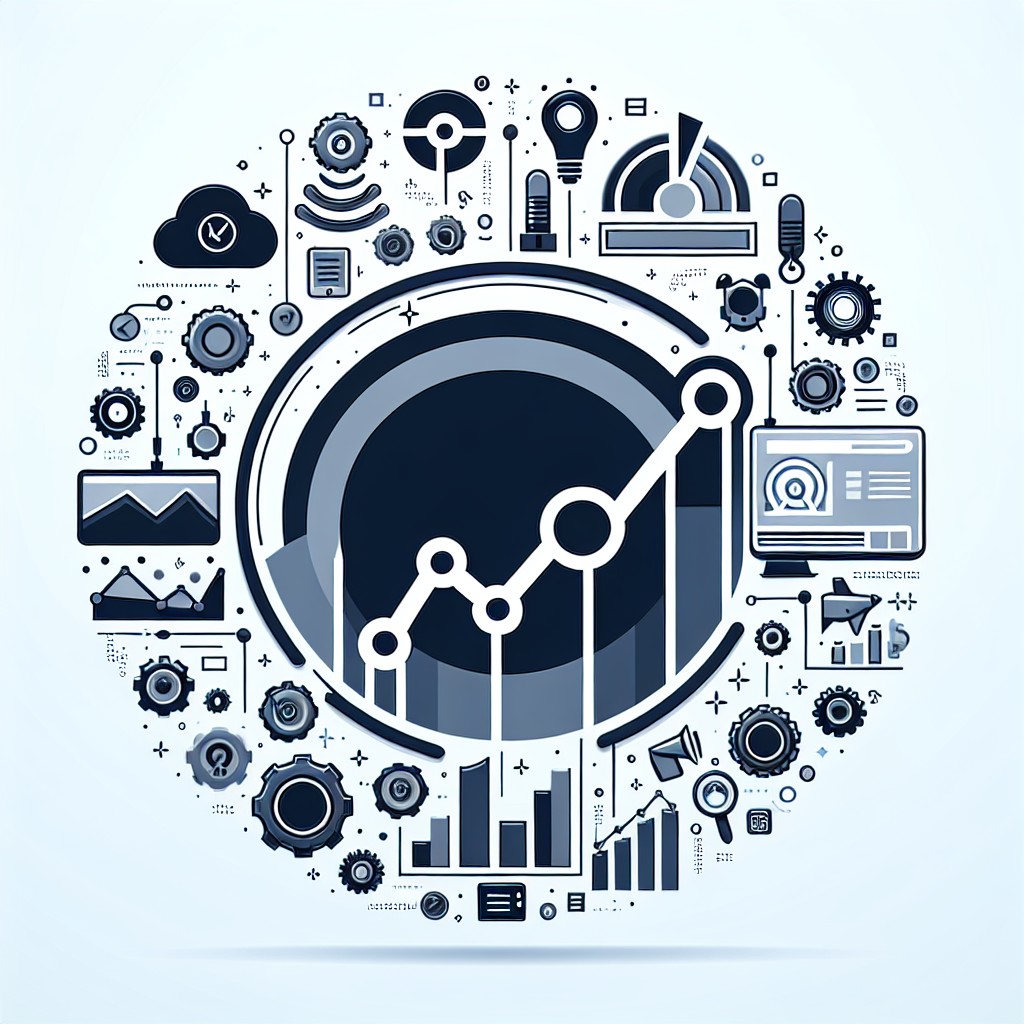