Generative AI in Market Research: Opportunities & Pitfalls
Introduction: A New Era of Market Intelligence
Market research has evolved from traditional surveys and focus groups to sophisticated digital methodologies, but generative AI represents a paradigm shift in how organizations gather consumer insights. As McKinsey reports, generative AI could add $2.6-4.4 trillion annually to the global economy across various sectors. In market research specifically, AI systems capable of generating human-like text, images, and simulations are transforming how brands understand consumers, test concepts, and forecast trends. Unlike traditional analytics that interprets existing data, generative AI creates new data patterns and insights, enabling researchers to explore previously inaccessible dimensions of consumer behavior. As Professor Avinash Collis of MIT notes, "Generative AI doesn't just analyze what consumers have done; it helps predict what they might do in previously unimagined scenarios." This article examines how generative AI is reshaping market research, balancing its revolutionary potential against significant ethical and methodological considerations.
1. Core Applications of Generative AI in Market Research
a) Synthetic Consumer Personas & Behavioral Simulation
Generative AI creates detailed, realistic consumer personas that simulate how different segments might respond to products or campaigns.
Example: Unilever's "AI Consumer Lab" uses generative models to create thousands of synthetic consumer profiles that interact with product concepts in simulated environments, reducing traditional research timelines by 60% and enabling more iterative testing.
b) Unstructured Data Analysis & Insight Generation
Generative AI transforms vast unstructured data (social conversations, reviews, customer service interactions) into actionable insights.
Example: Procter & Gamble deployed generative AI systems to analyze millions of product reviews across 20+ markets, uncovering nuanced sentiment patterns that traditional NLP missed and identifying emerging consumer needs that informed R&D priorities.
c) Concept Testing & Rapid Prototyping
AI generates multiple campaign or product variations for testing without expensive production costs.
Example: Samsung's marketing team uses generative AI to create dozens of ad concept variations, testing messaging effectiveness before investing in full production, resulting in 25% higher engagement metrics.
d) Predictive Trend Analysis
By synthesizing patterns from diverse data sources, generative AI identifies emerging trends earlier than traditional methods.
Example: LVMH's trend forecasting unit combines generative AI analysis of fashion runway imagery, social media content, and historical sales data to predict micro-trends 3-6 months before they become apparent through conventional methods.
2. Strategic Advantages: The Transformative Impact
a) Speed & Scale Amplification
Generative AI dramatically accelerates research cycles, enabling real-time insight generation and continuous consumer understanding.
Research from Gartner indicates companies using generative AI in market research reduce time-to-insight by 40-70% compared to traditional methodologies.
b) Exploration of Counterfactual Scenarios
Perhaps the most revolutionary aspect is the ability to simulate "what if" scenarios that would be impossible to test in real-world environments.
Example: Coca-Cola uses generative AI to simulate consumer response to packaging innovations across diverse cultural contexts before committing to actual production changes.
c) Democratization of Consumer Insights
Generative AI makes sophisticated market research accessible to smaller organizations lacking extensive research budgets.
Professor Thomai Serdari of NYU Stern observes that "Generative AI is leveling the playing field, allowing smaller brands to access consumer intelligence previously exclusive to market leaders."
3. Critical Pitfalls & Ethical Considerations
a) Data Biases & Representational Concerns
Generative models reflect biases in their training data, potentially perpetuating or amplifying market blind spots.
Example: A major retailer's generative AI research system initially produced insights skewed toward urban consumers, missing critical rural market opportunities until researchers identified and corrected the training data imbalance.
b) The Authenticity Paradox
Synthetic insights may lack the nuanced authenticity of direct consumer engagement.
Research by the Market Research Society shows 68% of senior insights professionals express concern about losing "human intuition" in AI-generated research conclusions.
c) Methodological Transparency Challenges
"Black box" generative systems make it difficult to audit methodological rigor.
Example: Facebook's attempt to use generative AI for cross-cultural consumer research faced criticism when researchers couldn't explain contradictory findings across different markets.
d) Ethical & Privacy Implications
Generating synthetic consumer data raises novel ethical questions about consent and representation.
The recent EU AI Act specifically addresses concerns about synthetic data generation in consumer research, requiring disclosure when generative AI creates or augments consumer profiles.
4. Implementation Framework: Responsible Integration
a) Hybrid Research Models
The most effective implementations combine generative AI with traditional human-centered research methods.
Example: Nestlé's "Augmented Insights" program pairs generative AI analysis with traditional ethnographic research, using each approach to validate and enhance the other.
b) Bias Detection & Mitigation Systems
Leading organizations implement continuous monitoring for potential biases in generative research.
Professor Sandra Matz of Columbia Business School recommends "automated bias detection systems that continuously evaluate generative outputs against demographic and psychographic benchmarks."
c) Transparency & Explainability Protocols
Establishing clear documentation of generative AI methodologies ensures research integrity.
Example: Microsoft's market research division has implemented a "generative audit trail" system that documents all AI-generated insights, including training data sources and model parameters.
Conclusion: Balancing Innovation & Integrity
Generative AI represents both the most significant opportunity and challenge for market research in decades. Organizations that thoughtfully integrate these technologies—maintaining methodological rigor while embracing new capabilities—will gain substantial competitive advantages through deeper, faster, and more nuanced consumer understanding. However, this integration must be approached with careful attention to potential biases, transparency limitations, and ethical considerations. As Professor Mark Ritson observes, "The future of market research isn't about replacing human insight with AI, but rather augmenting researchers with tools that expand their capacity to understand consumer complexity." The organizations that strike this balance will transform their market intelligence capabilities while maintaining the human-centered understanding essential to meaningful consumer connections.
Call to Action
For research and insights leaders navigating this new landscape:
- Develop clear governance frameworks for generative AI research methods, including bias monitoring protocols and transparency requirements.
- Invest in training programs that equip researchers to effectively collaborate with generative AI systems while maintaining methodological expertise.
- Create cross-functional teams that combine data scientists, traditional researchers, and ethicists to guide generative AI implementation.
Featured Blogs
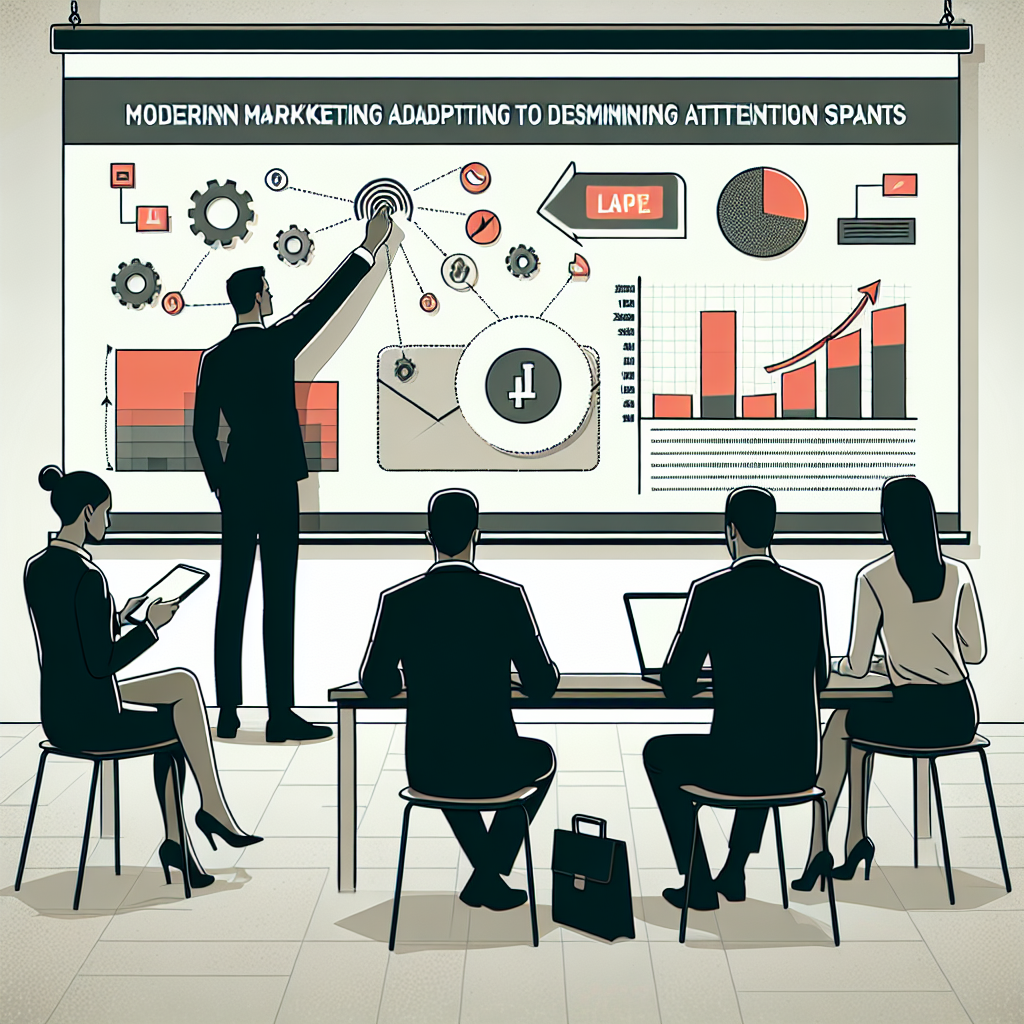
How the Attention Recession Is Changing Marketing
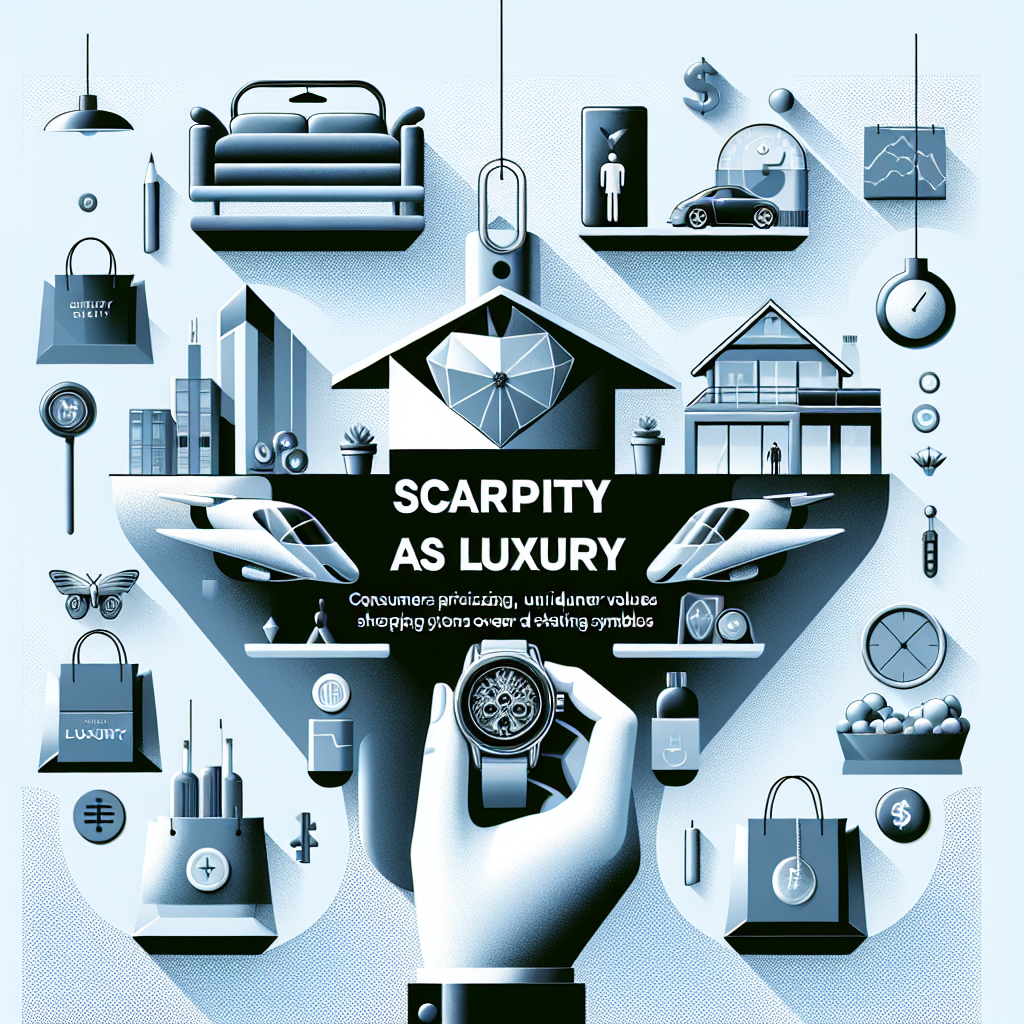
The New Luxury Why Consumers Now Value Scarcity Over Status
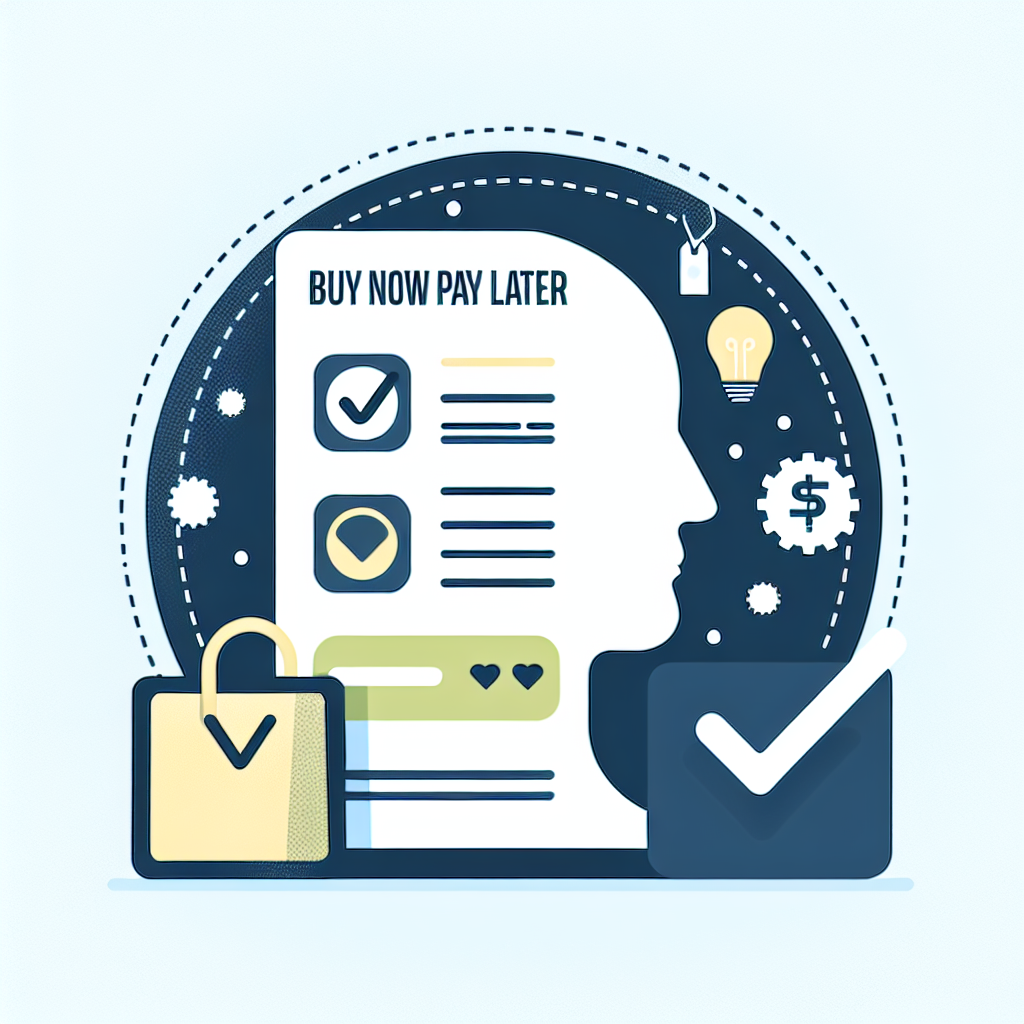
The Psychology Behind Buy Now Pay later
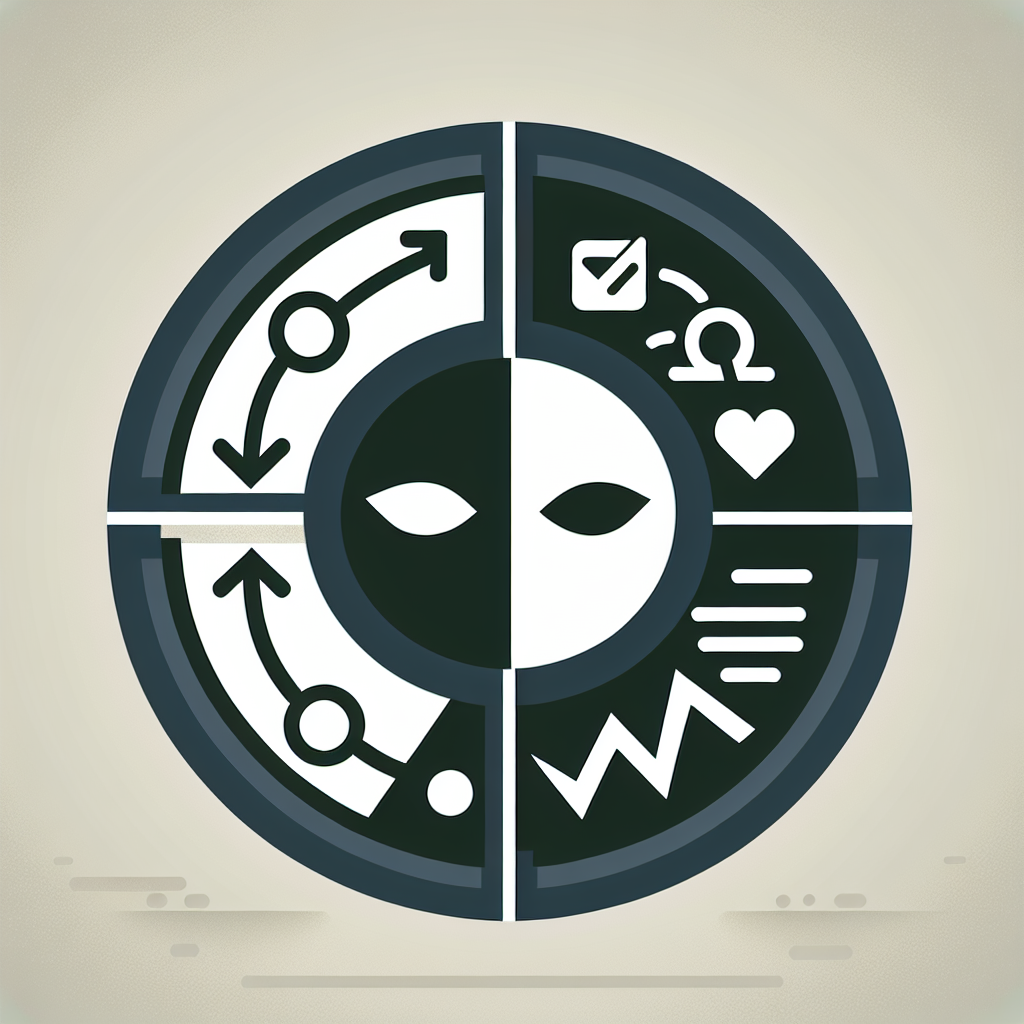
The Role of Dark Patterns in Digital Marketing and Ethical Concerns
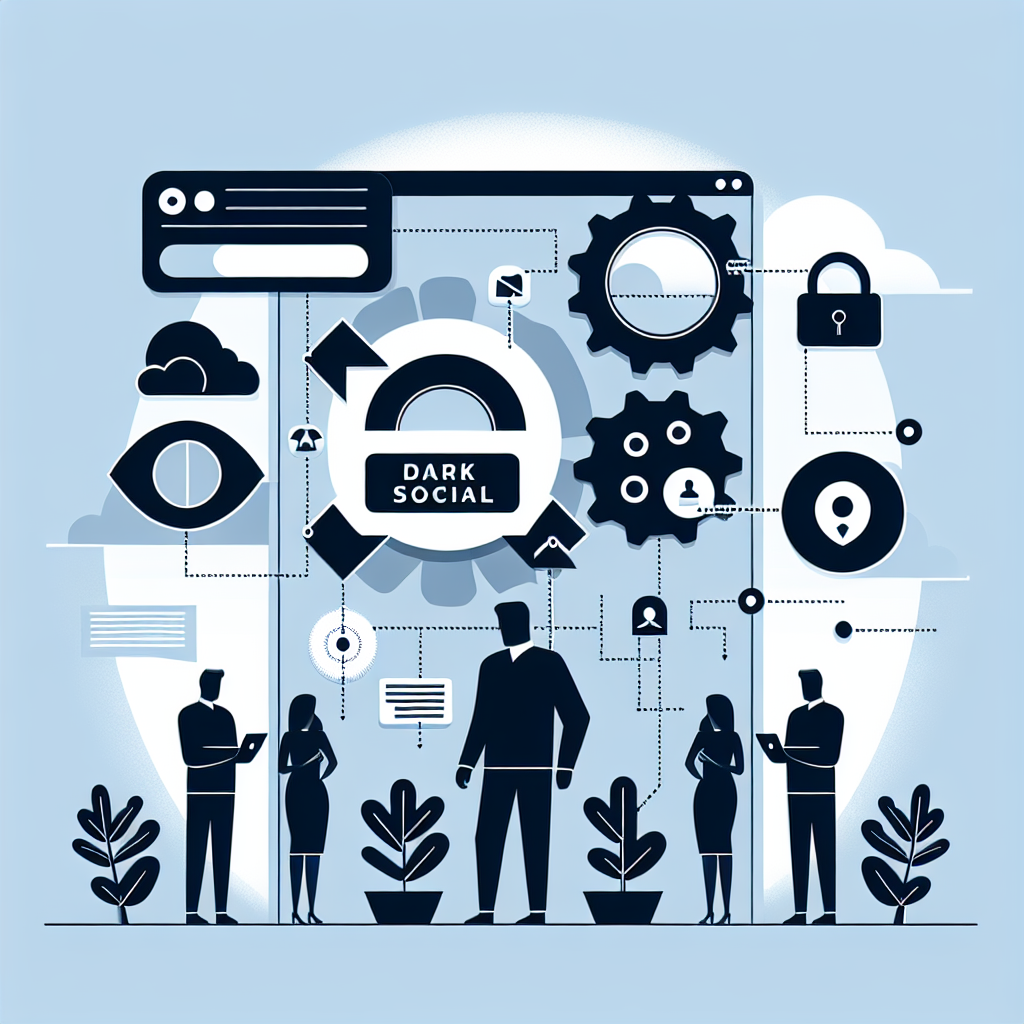
The Rise of Dark Social and Its Impact on Marketing Measurement
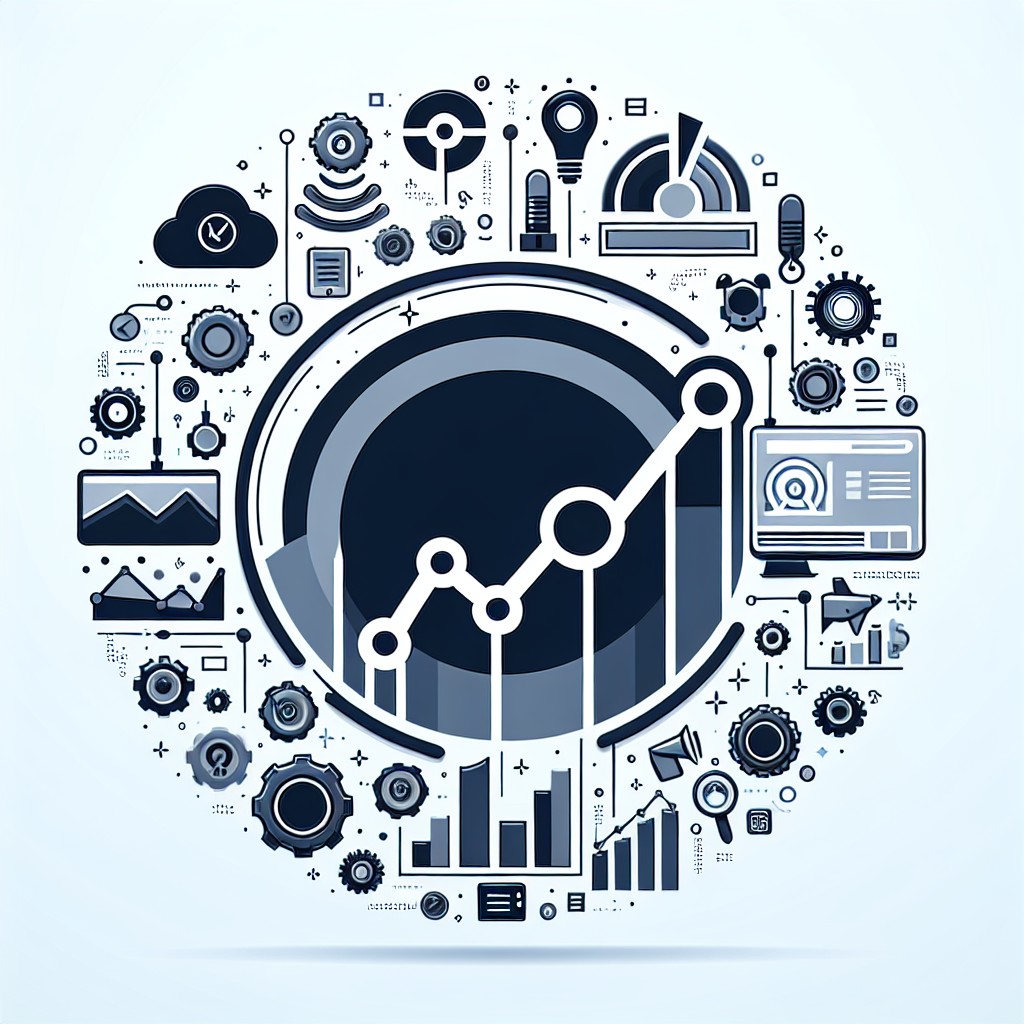