T-statistic
The t-statistic is a statistical measure used to evaluate the significance of the difference between the means of two groups. It is primarily utilized in hypothesis testing and constructing confidence intervals, especially when working with small sample sizes or when the population standard deviation is not known.
The formula for calculating the t-statistic is:
Where:
- Xˉ = Sample mean
- μ = Population mean
- s = Sample standard deviation
- n = Sample size
Table of Contents
- Understanding T-Statistics
- Applications of T-Statistics
- Importance of T-Statistics
- Implementation in Python
- Conclusion
Understanding T-Statistics
The T-statistic is a key component in statistical hypothesis testing, specifically within a T-test. It helps determine whether to accept or reject the null hypothesis by measuring the difference between sample means relative to the variability in the data. It functions similarly to a Z-score, where one compares a calculated value against a critical threshold. However, a T-test is typically applied when the sample size is small or when the population standard deviation is unknown.
Purpose of the T-Statistic
The T-statistic by itself does not provide much insight without context. Similar to how an "average" value only becomes meaningful when paired with relevant data (e.g., "The average height of students in a classroom is 5'6"), the T-statistic must be used within a hypothesis test to yield meaningful conclusions. This is done by comparing the T-statistic to a p-value, which helps determine the likelihood that observed differences in data occurred by chance.
For instance, suppose a group of friends scores an average of 205 in a bowling game, while the general population’s average score is 79.7. To determine whether their scores are significantly different from the population mean (and not just a random occurrence), a T-test is conducted. A higher T-value suggests stronger evidence of a significant difference, while a smaller T-value indicates no substantial deviation from the average.
If the p-value is greater than 5%, then the observed difference is likely due to chance. However, if the p-value is below 5%, it suggests a statistically significant difference—perhaps the team should consider taking bowling more seriously!
T-Score vs. Z-Score
Both T-scores and Z-scores help in determining whether a sample differs from a population mean. However, there are key differences:
T-Score | Z-Score |
---|---|
Used when population standard deviation is unknown | Requires population standard deviation to be known |
Preferred for small sample sizes (typically n < 30) | Suitable for large samples (typically n ≥ 30) |
Based on the T-distribution | Based on the Normal distribution |
Accounts for increased variability in small samples | Assumes less variability due to large sample size |
Since population parameters are often unknown, the T-test is generally used more frequently than the Z-test in real-world applications.
Applications of T-Statistics
T-statistics are widely used in various domains to analyze differences between sample means, particularly when sample sizes are small or population parameters are unknown. Common applications include:
1. Comparing Group Means
- Two-Sample T-Test: Determines whether there is a significant difference between the means of two independent groups.
- Example: Comparing student performance in two different teaching methods.
2. Quality Control in Manufacturing
- Process Monitoring: Helps assess whether production processes remain within expected parameters.
- Example: Evaluating variations in product weight or size across different batches.
3. Medical and Clinical Research
- Clinical Trials: Compares treatment effects between two patient groups to determine if a new medication has a significant impact.
- Example: Measuring blood pressure reduction in patients taking different medications.
These examples illustrate how T-statistics aid in hypothesis testing, ensuring that observed differences are statistically valid rather than random occurrences.
Importance of T-Statistics
The T-test plays a crucial role in statistical inference, allowing researchers to determine whether observed differences in data are meaningful. Key reasons for its significance include:
1. Hypothesis Testing
- Null Hypothesis (H₀): Assumes no significant difference between sample means.
- Alternative Hypothesis (H₁): Suggests a significant difference exists.
- The T-test helps decide whether the null hypothesis should be rejected in favor of the alternative.
2. Effective for Small Samples
- Unlike the Z-test, which requires a large sample size (n ≥ 30), the T-test is designed for smaller datasets.
- The T-distribution accounts for increased variability in small samples, making it more appropriate in many practical scenarios.
3. Consideration of Degrees of Freedom
- The T-test adjusts for sample size through degrees of freedom (df), improving the accuracy of statistical estimates.
- Degrees of freedom impact the critical values needed for hypothesis testing, making the T-test more adaptable to real-world data limitations.
4. Broad Applicability Across Disciplines
- Business & Economics: Evaluating employee performance before and after training programs.
- Social Sciences: Studying behavioral differences between demographic groups.
- Healthcare: Analyzing the effectiveness of medical treatments across patient populations.
By providing a reliable method for comparing means, the T-test enables data-driven decision-making across a wide range of industries and research fields.
Implementation in Python
import numpy as np
from scipy import stats
# Function to perform a two-sample t-test and explain results
def perform_t_test(group1, group2, alpha=0.05):
"""
Perform a two-sample t-test to compare the means of two groups.
Parameters:
group1 (array-like): Data for the first group.
group2 (array-like): Data for the second group.
alpha (float): Significance level for the test (default 0.05).
Returns:
None
"""
# Calculate the t-statistic and p-value using a two-sample t-test
t_statistic, p_value = stats.ttest_ind(group1, group2)
# Output the t-statistic and p-value
print(f"T-statistic: {t_statistic:.4f}")
print(f"P-value: {p_value:.4f}")
# Explain significance based on the p-value
if p_value < alpha:
print(f"Since the p-value ({p_value:.4f}) is less than the significance level ({alpha}), "
"we reject the null hypothesis. There is a statistically significant difference between the two groups.")
else:
print(f"Since the p-value ({p_value:.4f}) is greater than the significance level ({alpha}), "
"we fail to reject the null hypothesis. There is no statistically significant difference between the two groups.")
# Example usage:
np.random.seed(42) # For reproducibility
# Generate example data for two groups with normal distributions
group1 = np.random.normal(loc=30, scale=10, size=30) # Group 1: Mean=30, SD=10
group2 = np.random.normal(loc=35, scale=12, size=30) # Group 2: Mean=35, SD=12
# Perform the t-test and explain the result
perform_t_test(group1, group2)
T-statistic: -2.0720
P-value: 0.0427
Since the p-value (0.0427) is less than the significance level (0.05), we reject the null hypothesis. There is a statistically significant difference between the two groups.
Null Hypothesis (H₀): The assumption that there is no difference between the two groups. In this case, the null hypothesis assumes that the means of the two groups are equal.
Alternative Hypothesis (H₁): The assumption that there is a difference between the two groups. If we reject the null hypothesis, we accept that the means of the groups are different.
T-statistic: The t-statistic measures the difference between the means of two groups relative to the variation in the data. The larger the t-statistic, the greater the evidence that there is a significant difference between the groups.
P-value: The p-value tells us the probability of observing the data if the null hypothesis is true. A small p-value (typically < 0.05) indicates that the difference between groups is statistically significant.
Conclusion
Utilizing T-statistics in Python enables efficient and reliable hypothesis testing and mean comparisons. Below are the key takeaways regarding the application of T-statistics in Python:
- Convenient Access to Statistical Libraries
- Python provides well-established libraries such as NumPy and SciPy, which offer built-in functions for performing T-tests and other statistical computations with ease. These tools simplify statistical analysis, making it more accessible and efficient.
- User-Friendly Implementation
- With Python’s intuitive syntax and well-documented statistical functions, T-tests can be conducted effortlessly, even by individuals with limited statistical knowledge. The straightforward implementation enhances usability and reduces the learning curve.
- Support for Various T-Test Variants
- Python accommodates different types of T-tests, including one-sample, two-sample, and paired-sample T-tests. This versatility makes it a valuable tool for analyzing data across diverse research scenarios and experimental setups.
Final Thoughts
Python’s rich ecosystem of statistical libraries provides a powerful, flexible, and accessible framework for conducting T-tests and broader statistical analyses. Its open-source nature and integration with data science tools make it an ideal choice for researchers, analysts, and data scientists seeking efficient, scalable, and insightful statistical evaluations.
Featured Blogs
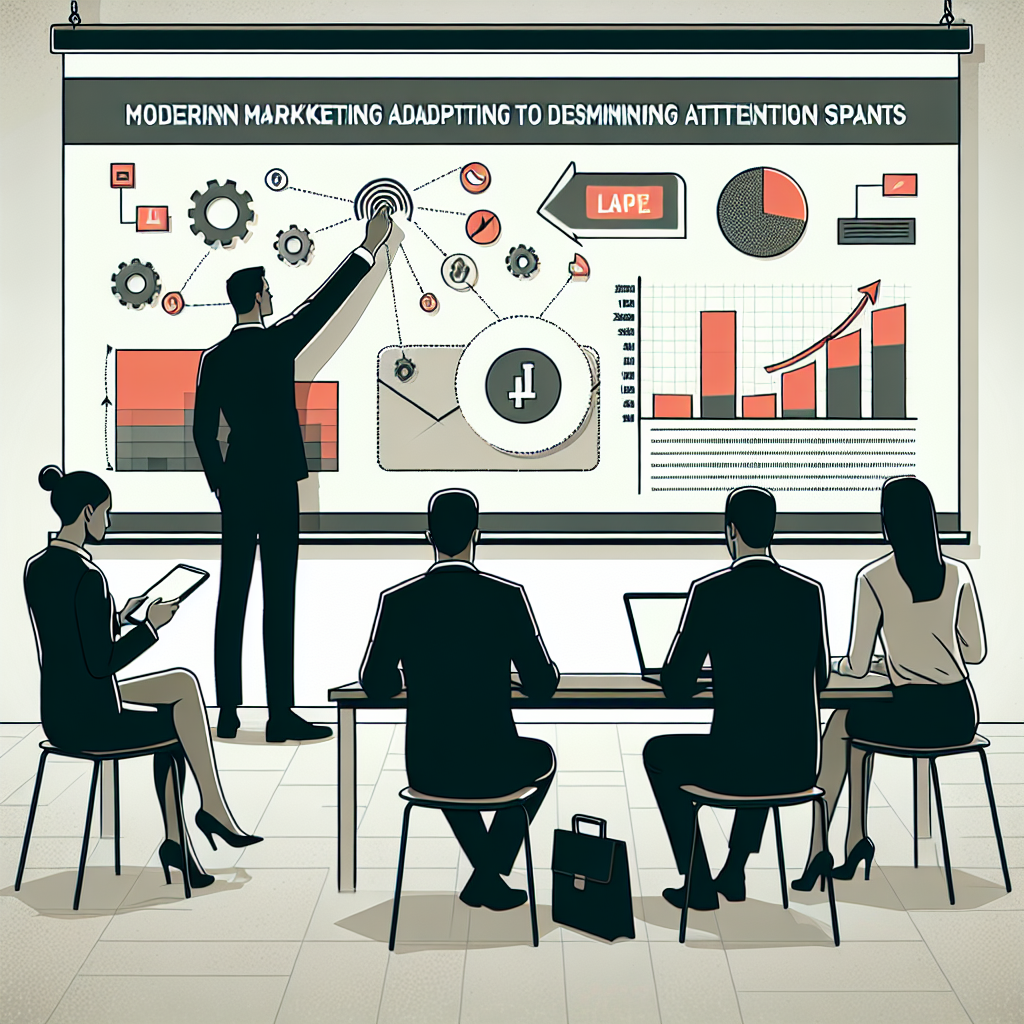
How the Attention Recession Is Changing Marketing
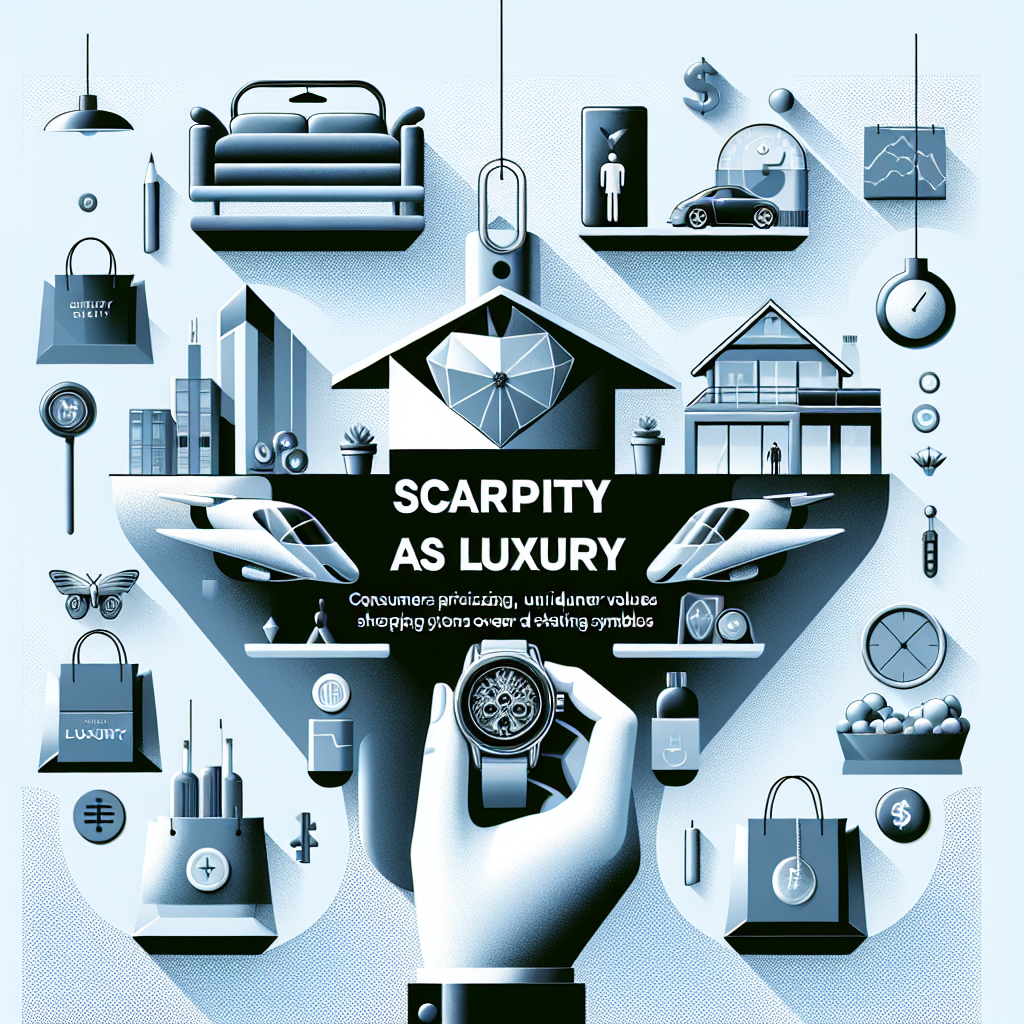
The New Luxury Why Consumers Now Value Scarcity Over Status
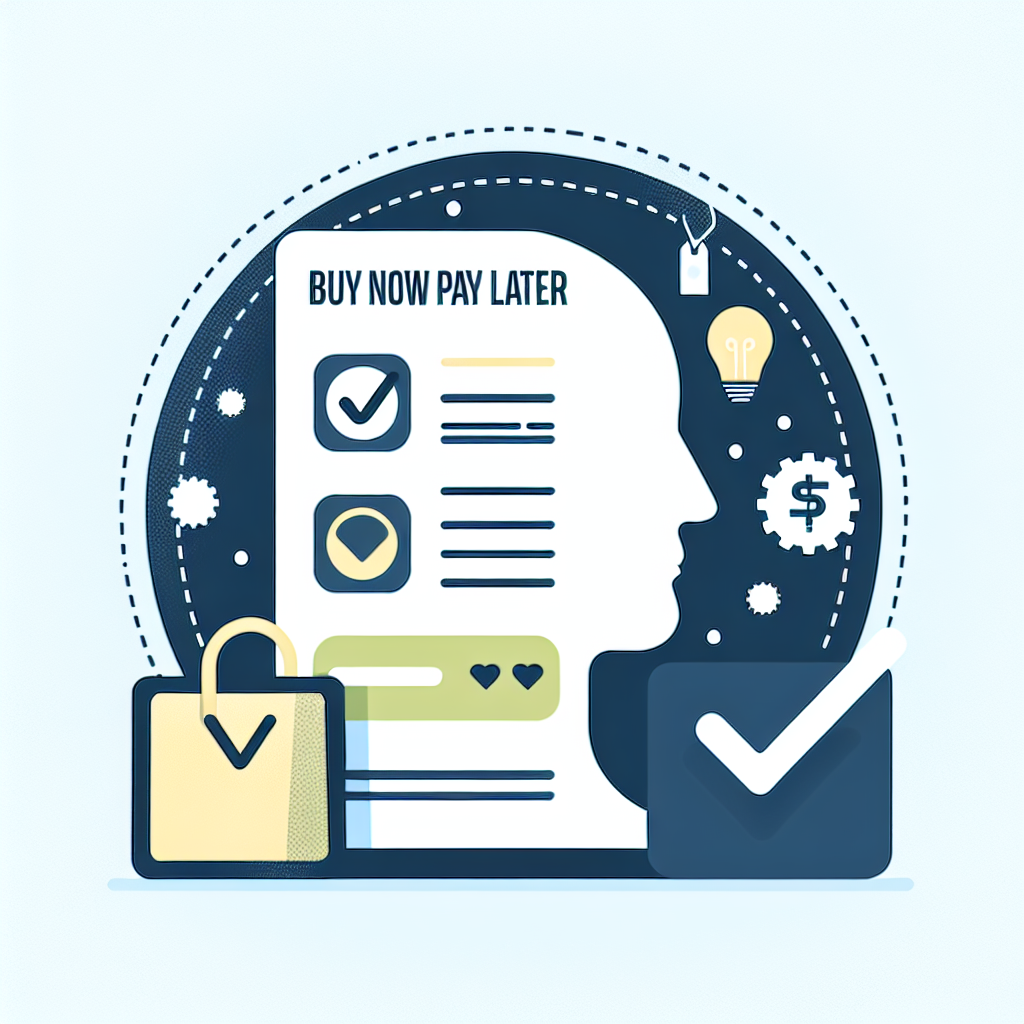
The Psychology Behind Buy Now Pay later
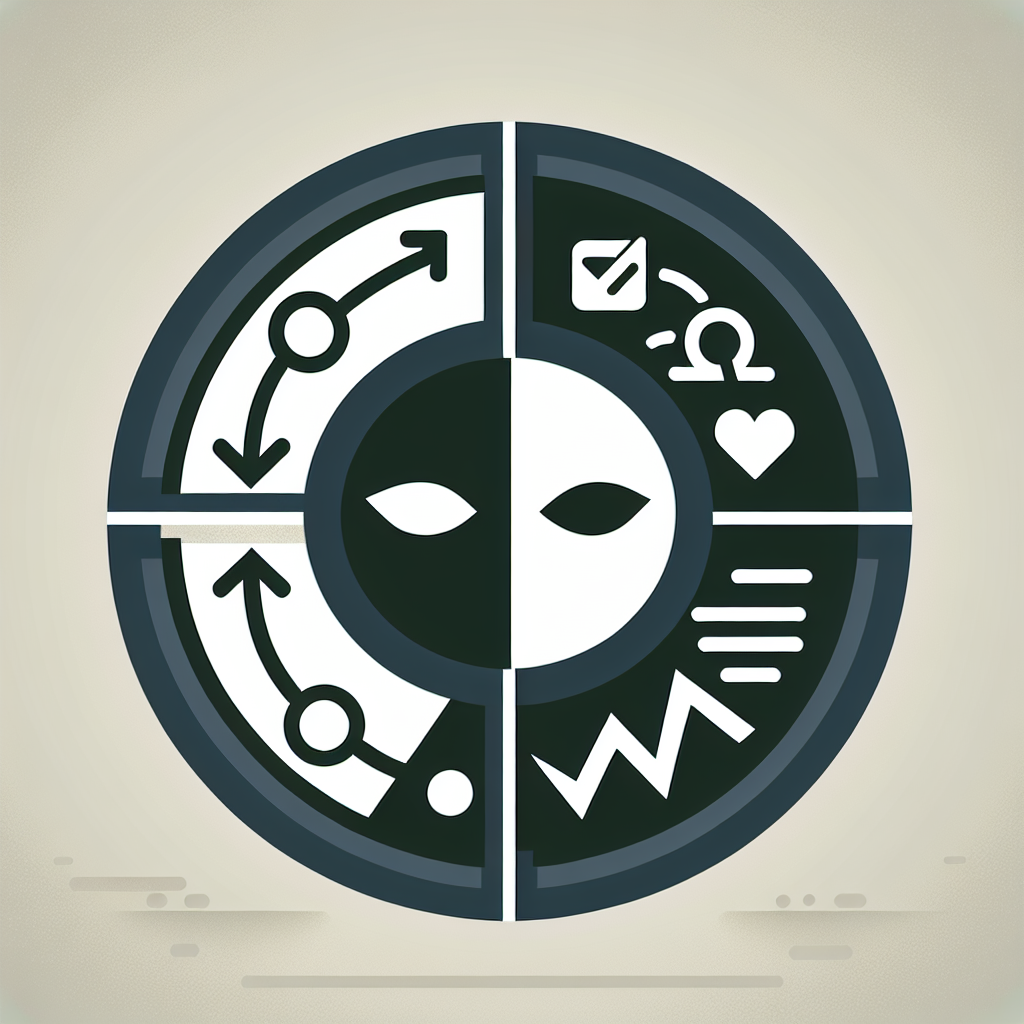
The Role of Dark Patterns in Digital Marketing and Ethical Concerns
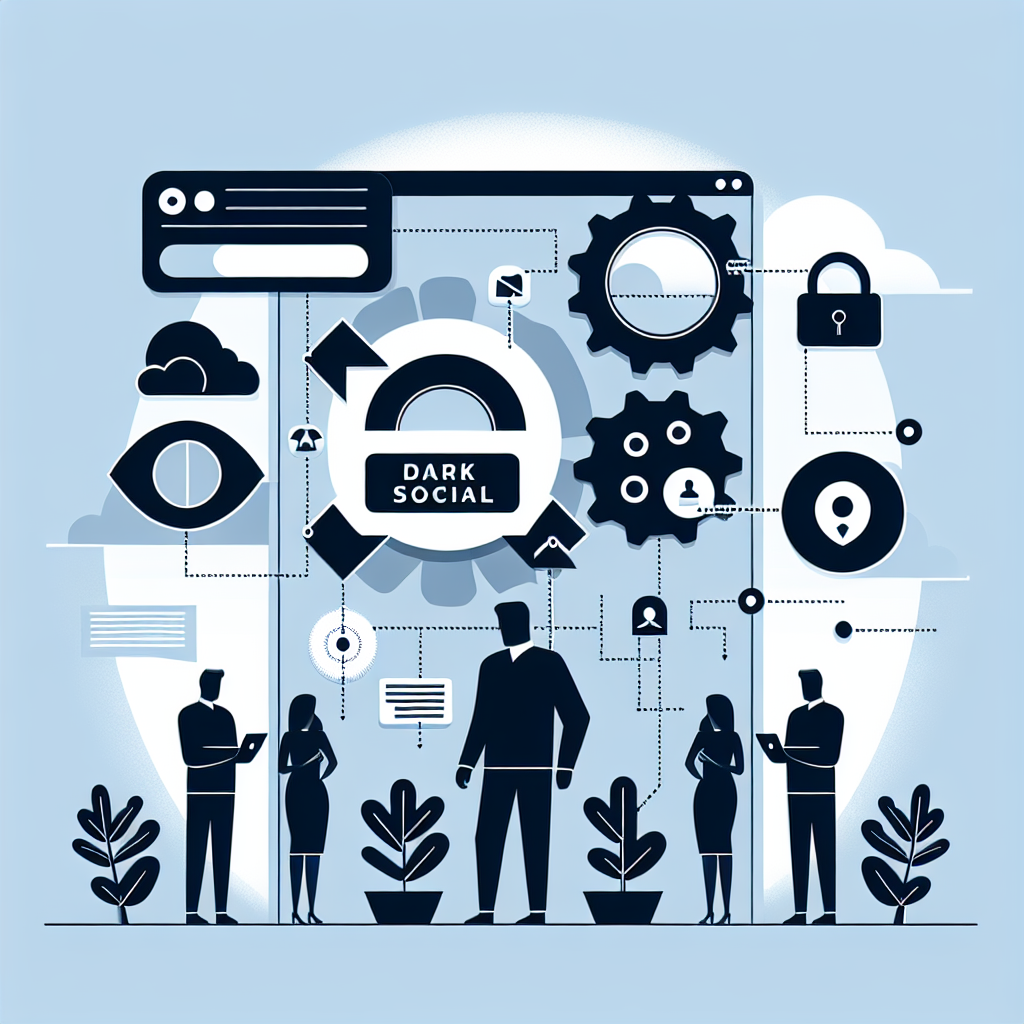
The Rise of Dark Social and Its Impact on Marketing Measurement
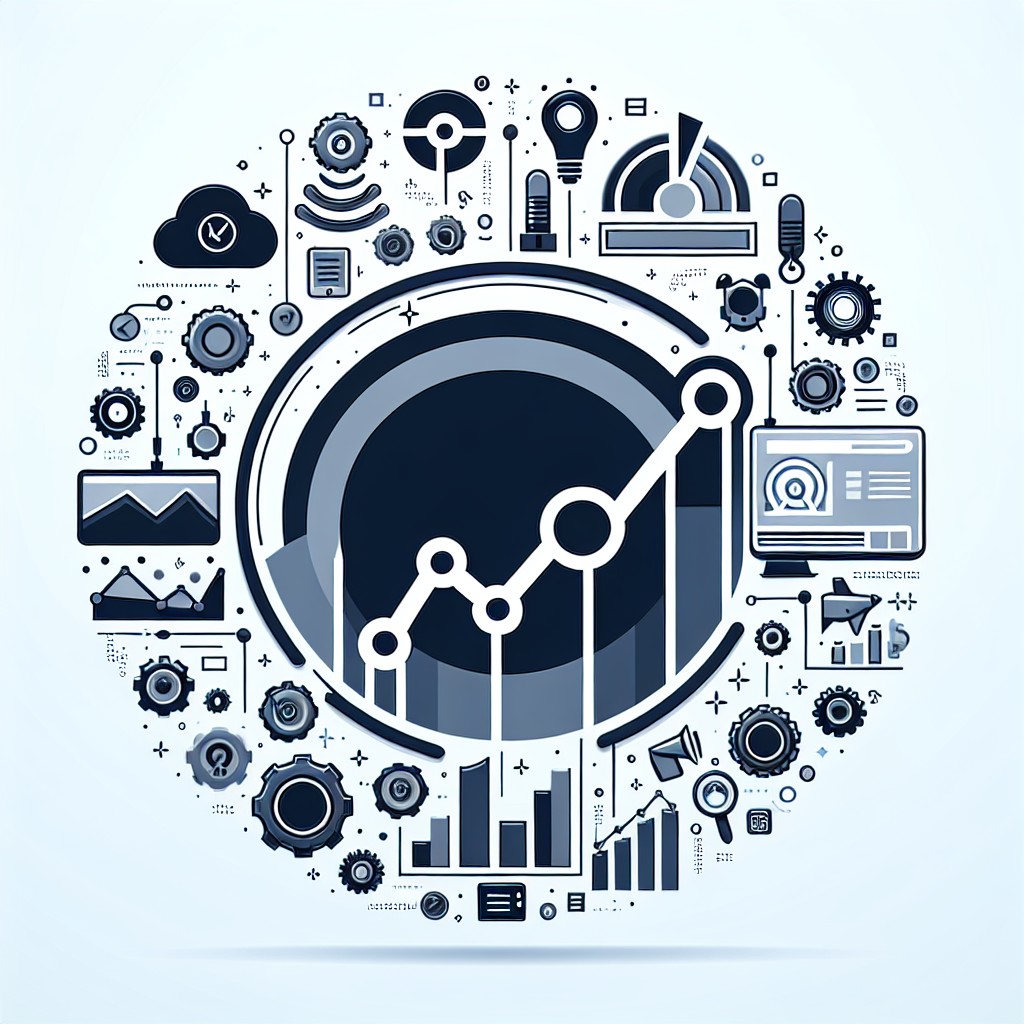
The Future of Retail Media Networks and What Marketers Should Know
Recent Blogs
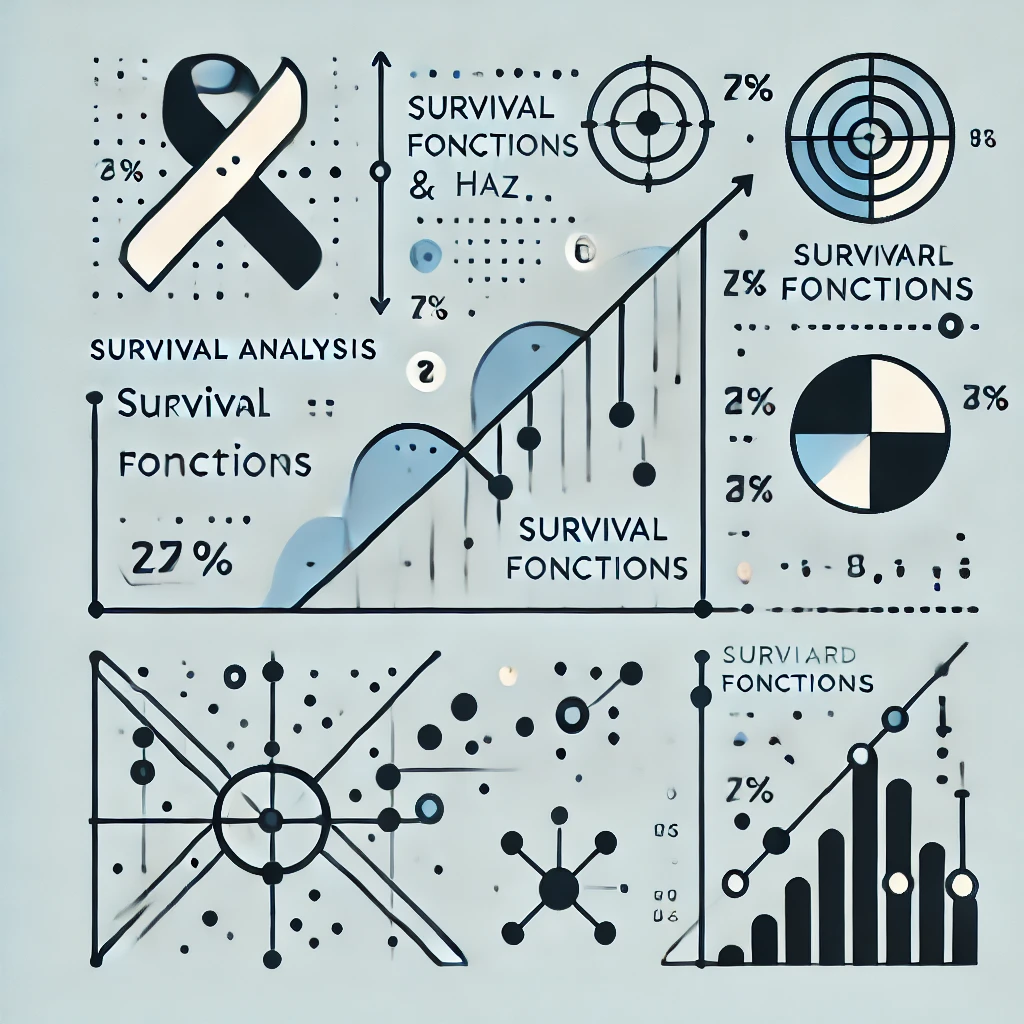
Survival Analysis & Hazard Functions: Concepts & Python Implementation
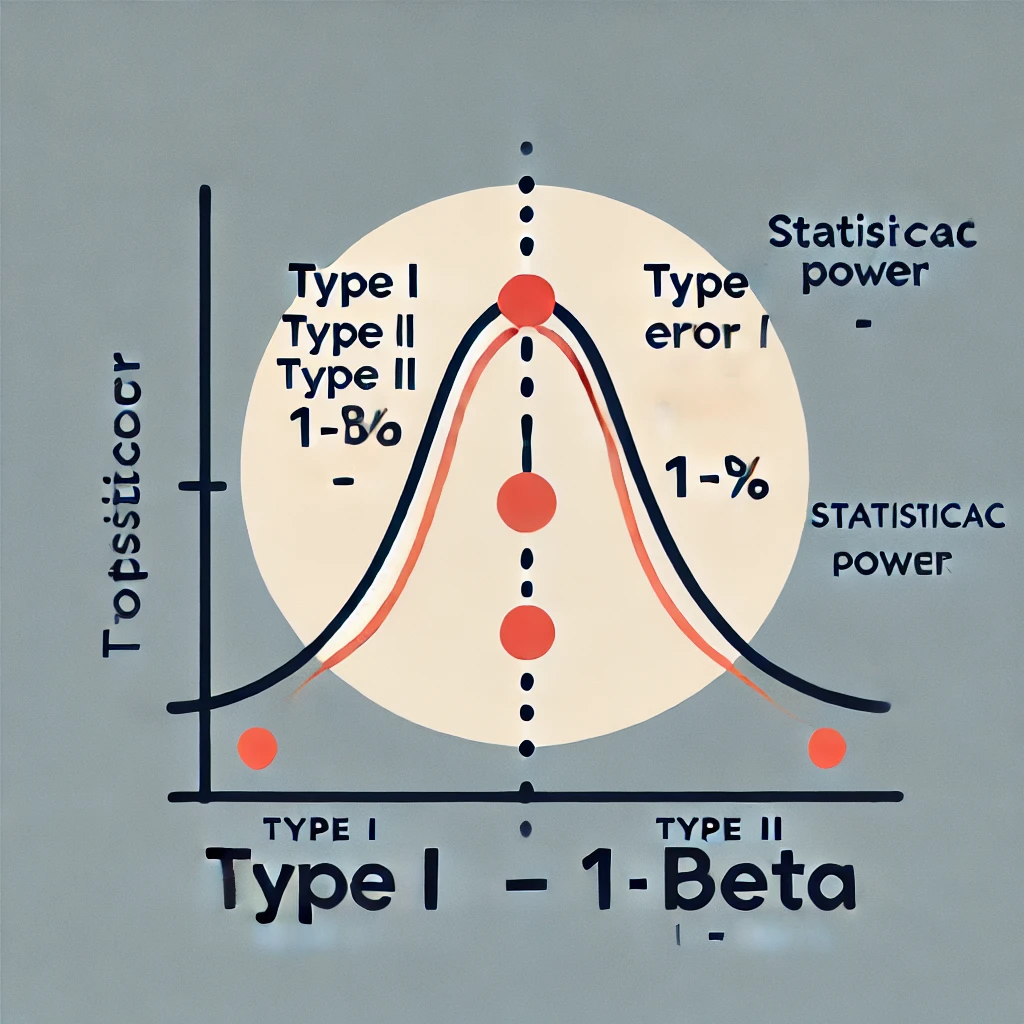
Power of a Statistical Test: Definition, Importance & Python Implementation
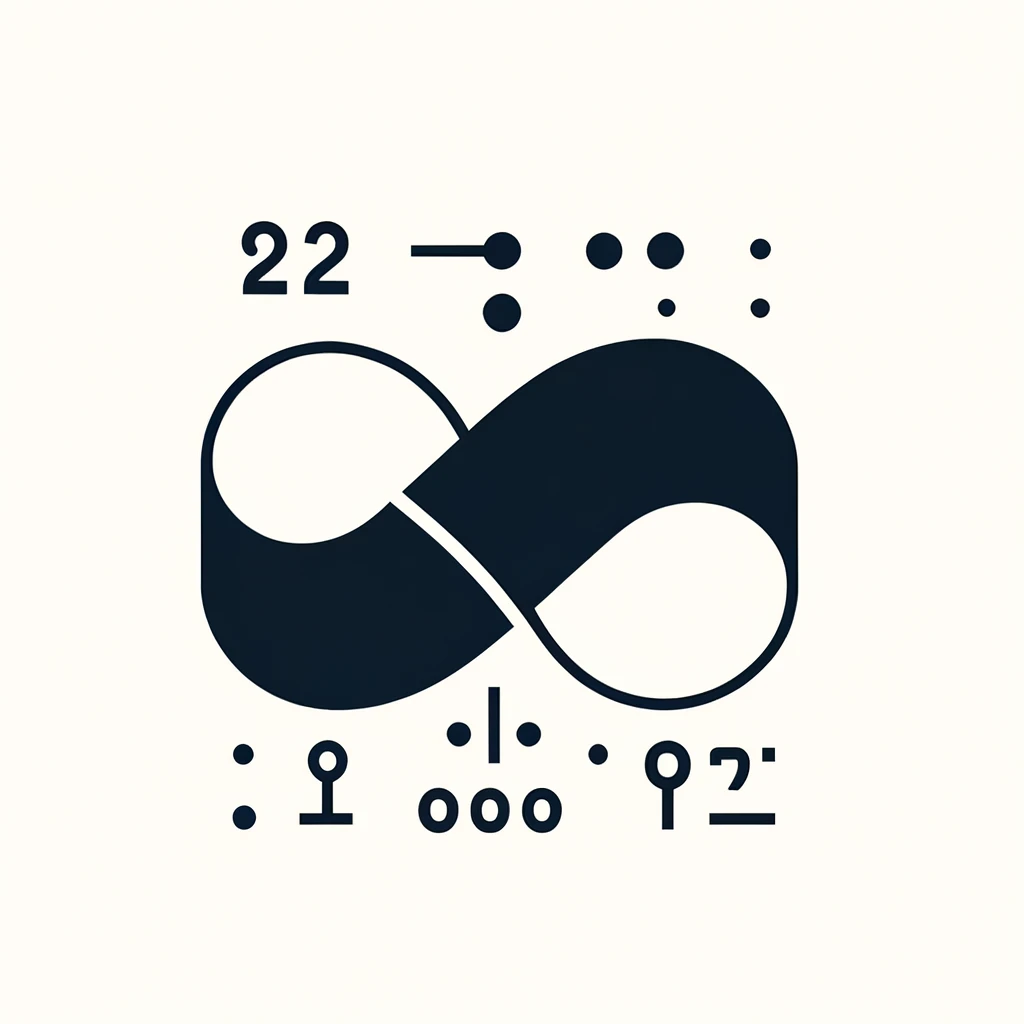
Logistic Regression & Odds Ratio: Concepts, Formula & Applications
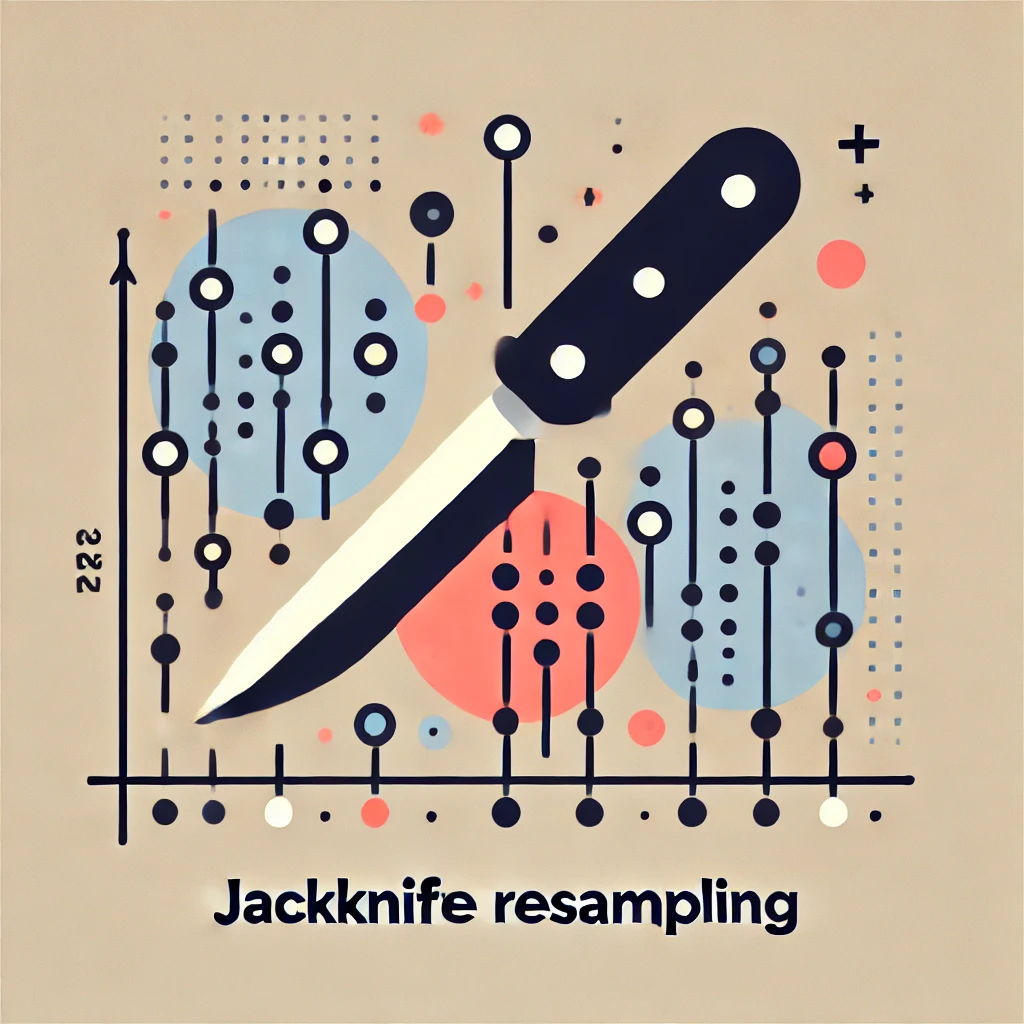
Jackknife Resampling: Concept, Steps & Applications
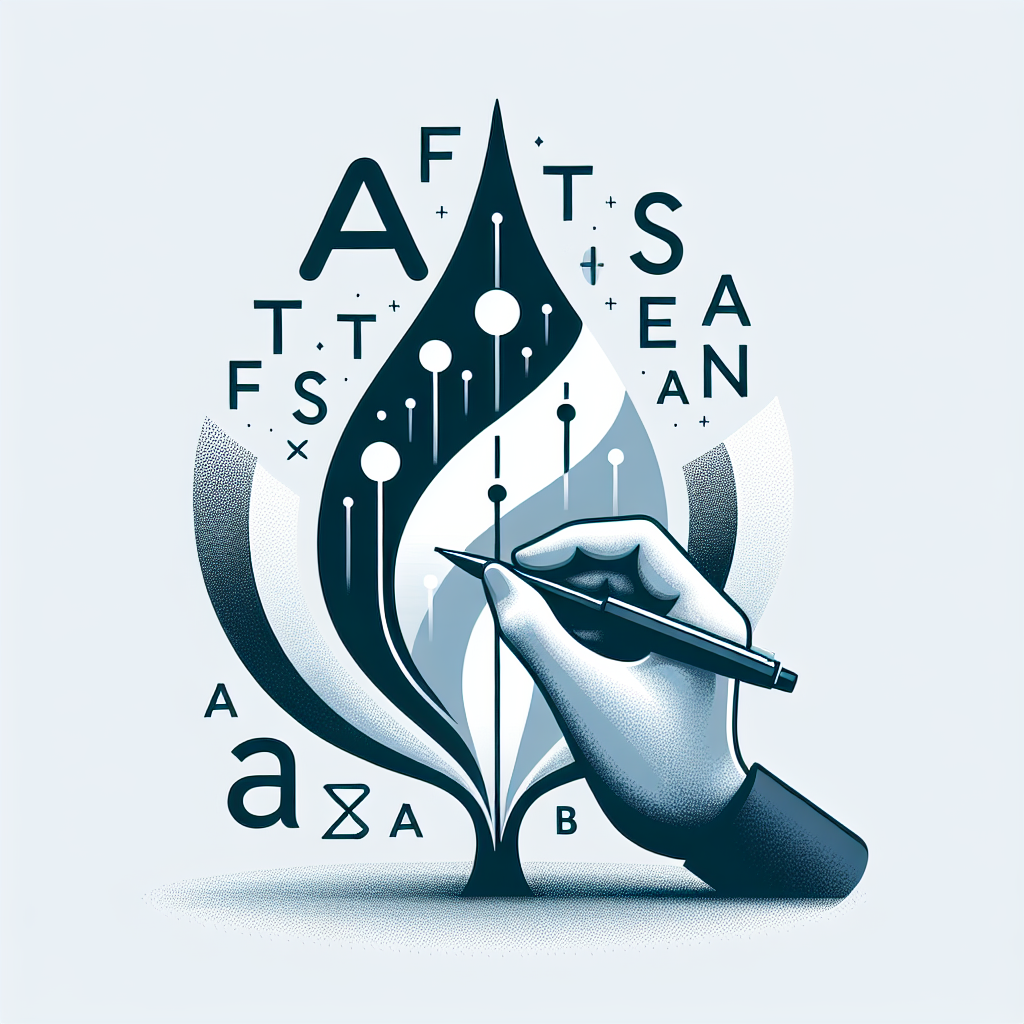
F test and Anova
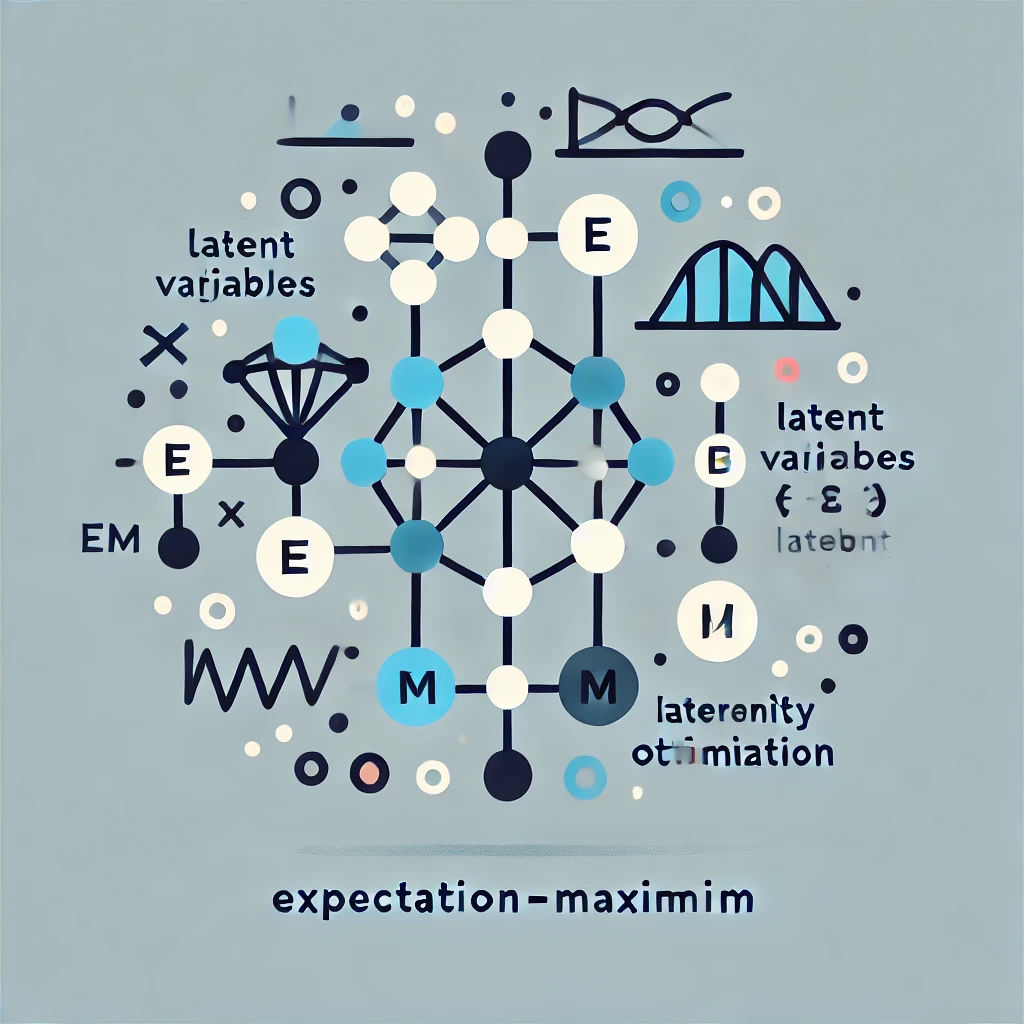