P-Value
Table of Contents
- What is a P-Value?
- Applications of P-Value
- Importance of P-Value
- Implementing P-Value in Python
- Conclusion
What is a P-Value?
A p-value (probability value) is a statistical measure used to evaluate the strength of evidence against a null hypothesis in hypothesis testing. It quantifies the probability of obtaining a test statistic as extreme as the one derived from sample data, assuming that the null hypothesis holds true.
In hypothesis testing, researchers begin with a null hypothesis (H₀), which assumes no effect or no significant difference, and an alternative hypothesis (H₁), which suggests the presence of a meaningful effect or difference.
The interpretation of p-values follows a standard approach:
- A low p-value (typically below a predetermined threshold, such as 0.05) indicates that the observed data is unlikely under the null hypothesis, leading to its rejection in favor of the alternative hypothesis.
- A high p-value suggests that there is insufficient evidence to reject the null hypothesis.
It is essential to remember that a p-value does not directly indicate the truth of either hypothesis but instead serves as a tool for decision-making based on statistical evidence. Researchers should consider other factors, such as effect size and study design, when interpreting results.
How is the P-Value Calculated?
The calculation of a p-value depends on the type of statistical test being used, as different tests are designed for different data types and hypotheses. Some key points to consider:
- The choice of statistical test (e.g., t-test, chi-square test, ANOVA) depends on the structure of the data and the hypothesis being tested.
- The number of independent variables in a test affects the magnitude of the test statistic required to yield a given p-value.
Pre-calculated p-value tables are available for various statistical tests, allowing researchers to estimate p-values based on their test statistic and degrees of freedom (which is the number of observations minus the number of independent variables).
P-Value and Statistical Significance
- Determining Statistical Significance: P-values are used to determine whether an observed result is statistically significant, meaning it is unlikely to have occurred by random chance.
- Common Significance Levels: A widely used threshold for statistical significance is p < 0.05, meaning there is less than a 5% probability that the observed result is due to chance. Some fields use stricter thresholds, such as p < 0.01 or p < 0.001.
- Alpha Value: The chosen threshold for significance is known as the alpha level (α), which defines the probability of making a Type I error (incorrectly rejecting a true null hypothesis).
Applications of P-Value
P-values are widely used across various disciplines to validate hypotheses and inform decision-making. Some common applications include:
- Hypothesis Testing Across Disciplines:
- Medical Research: Used in clinical trials to determine whether a new drug or treatment is significantly more effective than a placebo or existing treatment.
- Social Sciences: Helps researchers test theories related to human behavior, psychology, and societal patterns.
- Quality Control in Manufacturing:
- Ensures that production processes meet required quality standards by analyzing variations in product characteristics (e.g., weight, dimensions).
- Biology and Genetics:
- Assesses the significance of associations between genetic markers and diseases.
- Determines whether observed changes in biodiversity are statistically meaningful.
- Economics and Business:
- Evaluates the impact of policy changes, economic trends, or marketing strategies on financial outcomes.
- Environmental Science:
- Determines whether environmental changes, such as pollution exposure, have a statistically significant impact on ecosystems.
While p-values are a valuable tool, they should not be the sole criterion for decision-making. Other statistical measures, such as effect size and confidence intervals, should also be considered.
Importance of P-Value in Research and Decision-Making
The p-value plays a critical role in statistical analysis by providing evidence to support or refute hypotheses. Here are key aspects of its significance:
- Guiding Hypothesis Testing:
- The p-value helps researchers determine whether there is enough evidence to reject the null hypothesis in favor of the alternative hypothesis.
- Establishing Decision Criteria:
- Researchers use a pre-defined alpha level (e.g., 0.05) to decide whether a result is statistically significant. If the p-value falls below alpha, the null hypothesis is rejected.
- Measuring Strength of Evidence:
- A smaller p-value suggests stronger evidence against the null hypothesis, indicating a more significant effect or relationship.
- Communicating Statistical Findings:
- P-values help researchers explain whether their findings are statistically meaningful and whether the results are likely due to chance.
- Impact on Policy and Research Decisions:
- Many scientific conclusions and policy decisions are influenced by p-values, making them a fundamental aspect of data-driven decision-making.
However, reliance on p-values alone can be misleading. Researchers must interpret them carefully, considering sample size, effect size, and study methodology to draw meaningful conclusions.
Implementation of P-value
import scipy.stats as stats
import numpy as np
# Sample data for two groups
group1 = [25, 30, 35, 40, 45]
group2 = [20, 22, 25, 28, 30]
# Perform the Independent Samples T-test
t_statistic, p_value = stats.ttest_ind(group1, group2)
df = len(group1) + len(group2) - 2
# Print results
print(f'T-statistic: {t_statistic:.2f}')
print(f'P-value: {p_value:.4f}')
# Significance level
alpha = 0.05
# Interpretation
if p_value < alpha:
print('The difference between the groups is statistically significant.')
else:
print('There is not enough evidence to reject the null hypothesis.')
# Explanation
print("\nExplanation:")
print(f"The p-value of {p_value:.4f} is compared to the significance level (alpha) of {alpha}.")
if p_value < alpha:
print(f"Since the p-value is less than {alpha}, we reject the null hypothesis.")
print("This indicates that there is a significant difference between the two groups.")
else:
print(f"Since the p-value is greater than or equal to {alpha}, we fail to reject the null hypothesis.")
print("This suggests that there is not enough evidence to say there is a significant difference between the two groups.")
T-statistic: 2.51
P-value: 0.0365
The difference between the groups is statistically significant.
Explanation:
The p-value of 0.0365 is compared to the significance level (alpha) of 0.05.
Since the p-value is less than 0.05, we reject the null hypothesis.
This indicates that there is a significant difference between the two groups.
Performing the T-test:
The ttest_ind function computes the t-statistic and p-value for the independent samples t-test.
Interpreting the Results:
The p-value helps determine whether the observed difference between group1 and group2 is statistically significant.
If the p-value is less than the chosen significance level (0.05 in this case), it suggests that the difference between the groups is statistically significant.
Output:
The code prints the t-statistic and p-value, along with an interpretation based on the comparison of the p-value with the significance level.
Conclusion
The p-value is a fundamental statistical measure widely used in hypothesis testing and decision-making across scientific research. Below are the key takeaways regarding its significance and proper interpretation:
- Role in Hypothesis Testing: P-values help determine whether the observed data provides sufficient evidence to reject the null hypothesis in favor of the alternative hypothesis.
- Threshold for Significance: Researchers define a significance level (commonly 0.05) as a benchmark. If the p-value falls below this threshold, it indicates that the observed results are unlikely to be due to random chance alone.
- Strength of Evidence: A lower p-value suggests stronger evidence against the null hypothesis, reinforcing the likelihood of a meaningful effect or relationship.
- Understanding Results: A statistically significant result (p-value < alpha) suggests that the observed effect is not merely due to chance. However, a higher p-value does not confirm the null hypothesis—it simply means there is insufficient evidence to reject it.
- Careful Interpretation: P-values should be evaluated alongside other factors such as effect size, study methodology, and the assumptions underlying statistical tests to avoid misleading conclusions.
In conclusion, while p-values serve as a valuable tool in statistical analysis, they should not be used in isolation. A holistic approach that incorporates study design, practical significance, and broader contextual insights is essential for drawing accurate and meaningful conclusions from data.
Featured Blogs
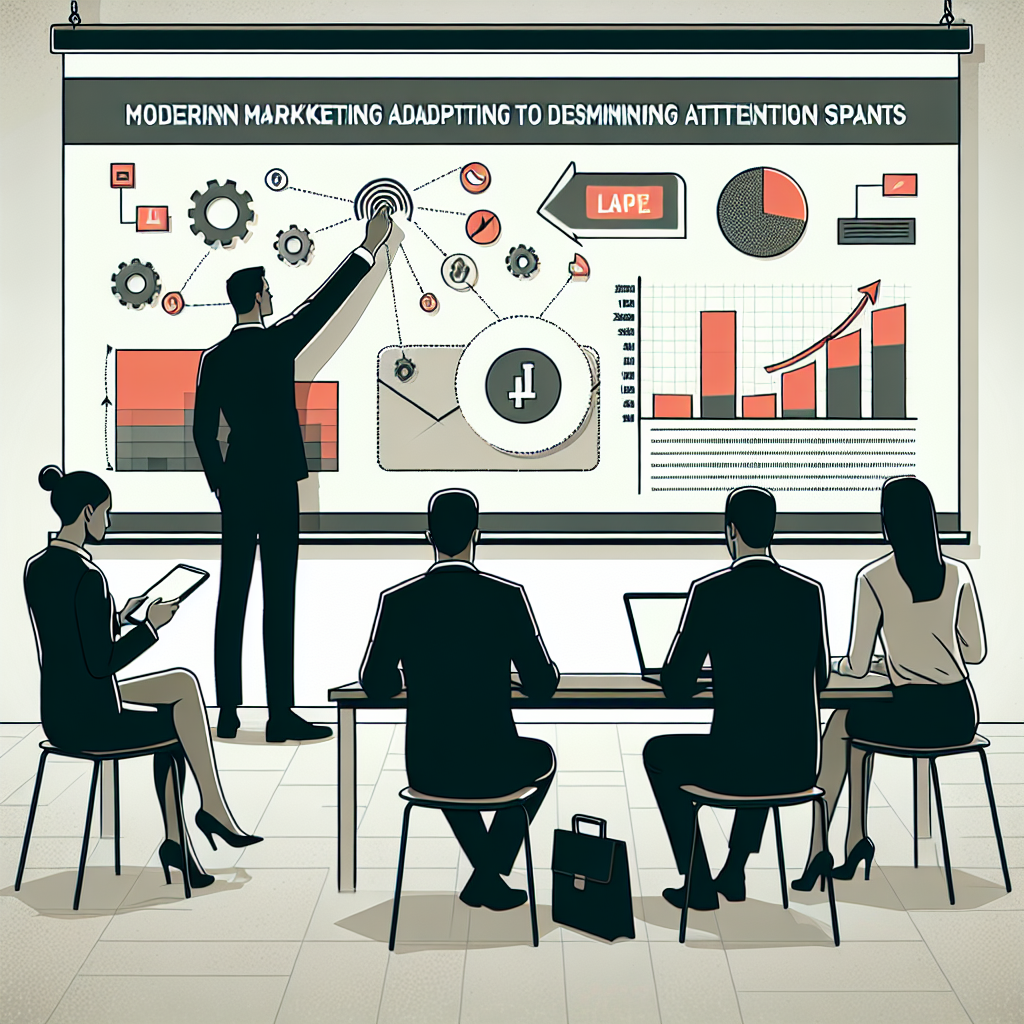
How the Attention Recession Is Changing Marketing
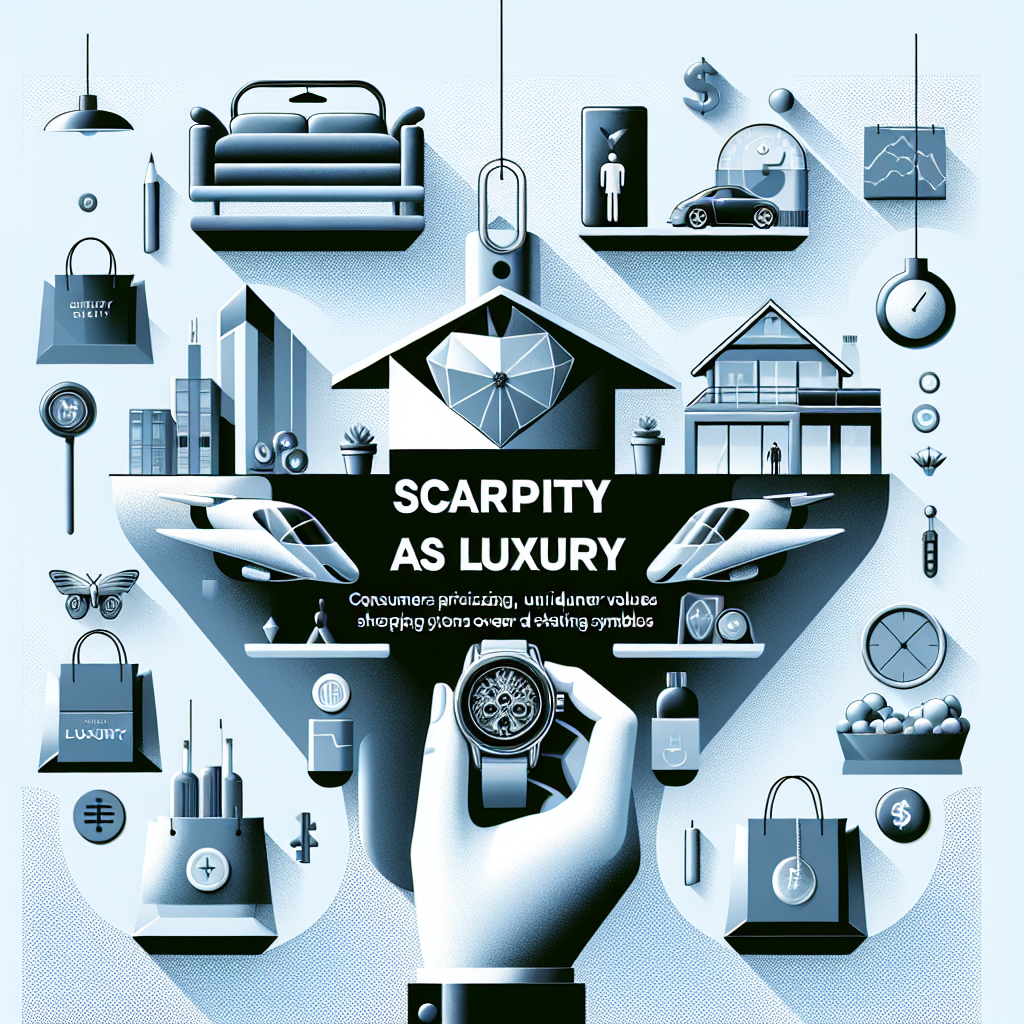
The New Luxury Why Consumers Now Value Scarcity Over Status
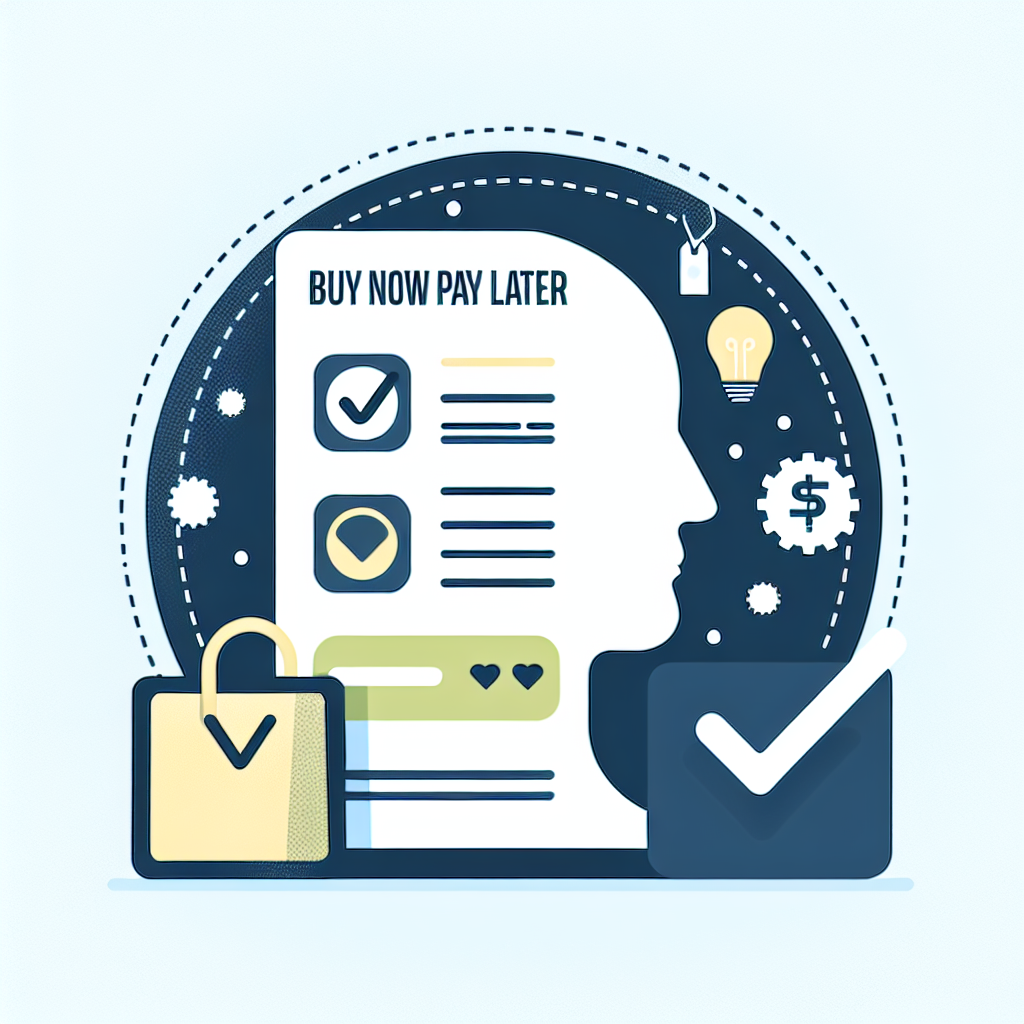
The Psychology Behind Buy Now Pay later
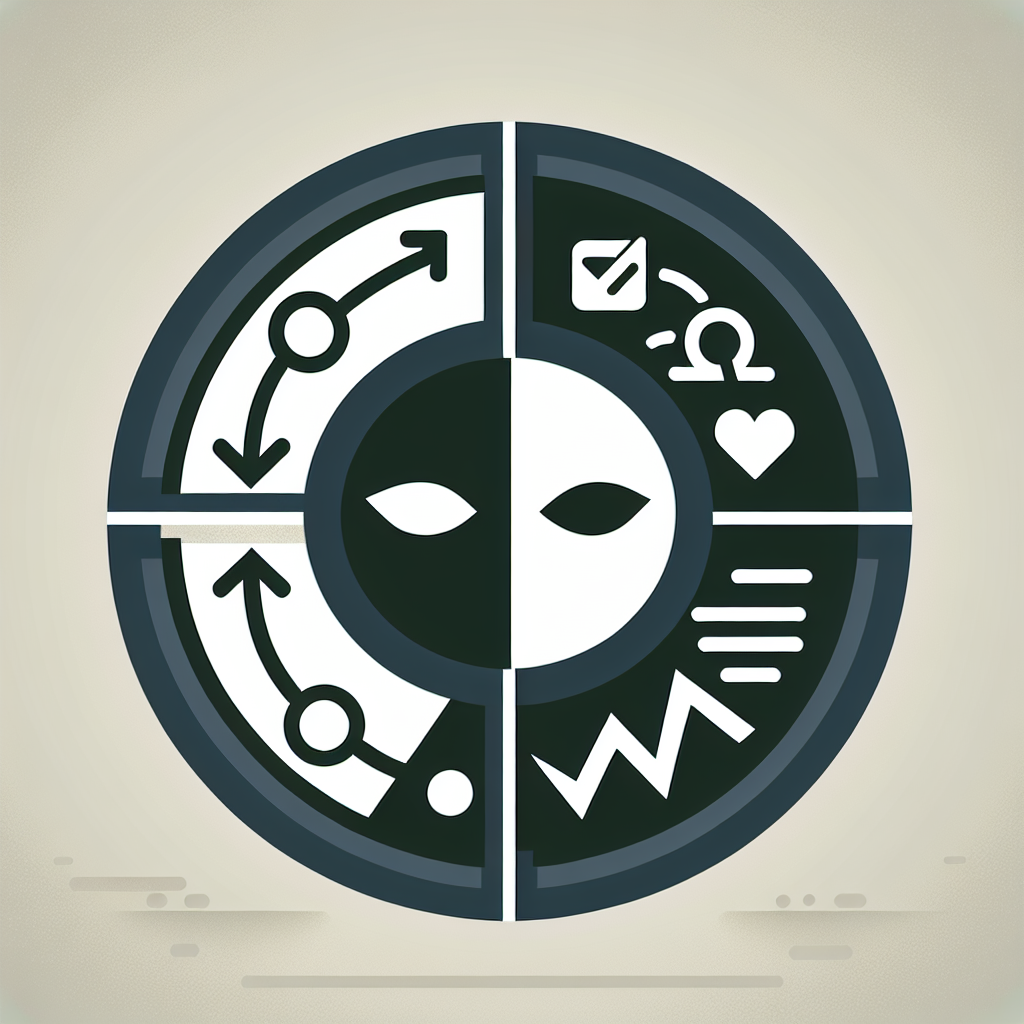
The Role of Dark Patterns in Digital Marketing and Ethical Concerns
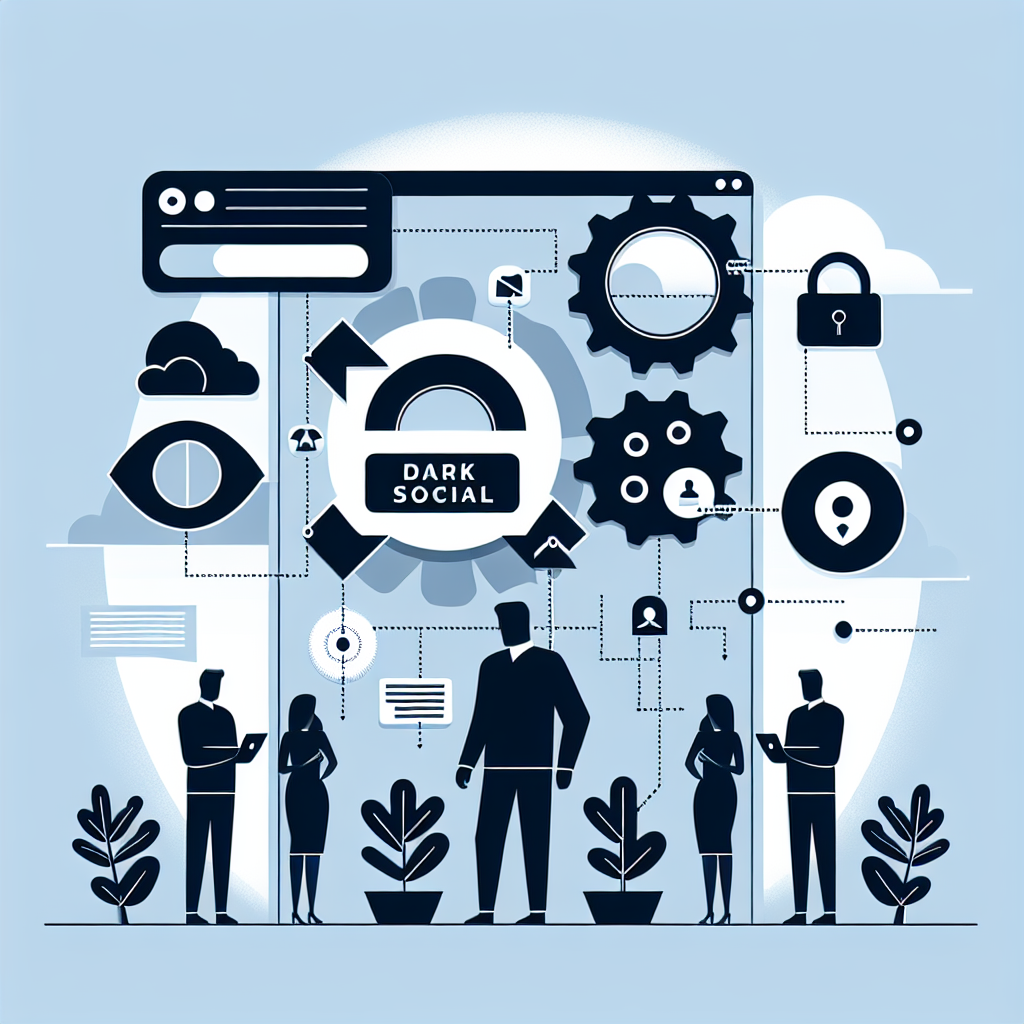
The Rise of Dark Social and Its Impact on Marketing Measurement
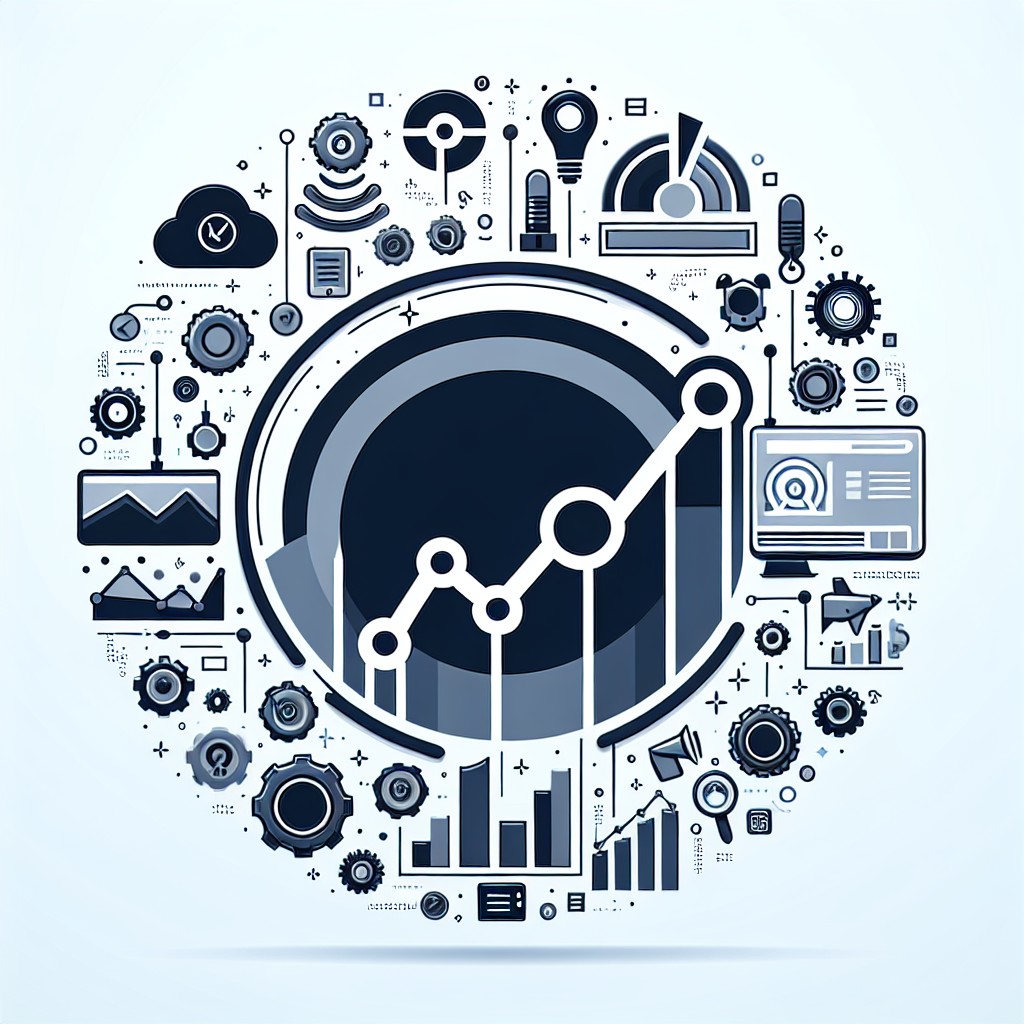
The Future of Retail Media Networks and What Marketers Should Know
Recent Blogs
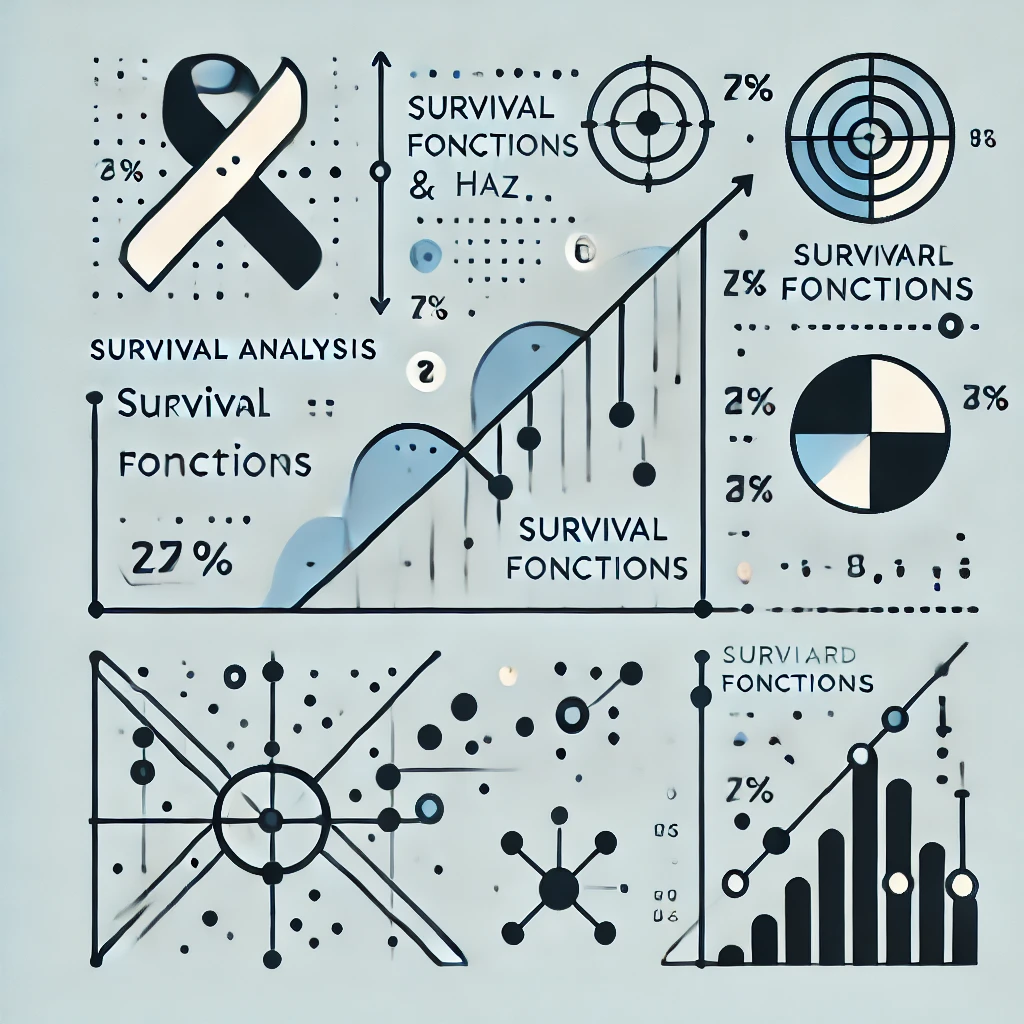
Survival Analysis & Hazard Functions: Concepts & Python Implementation
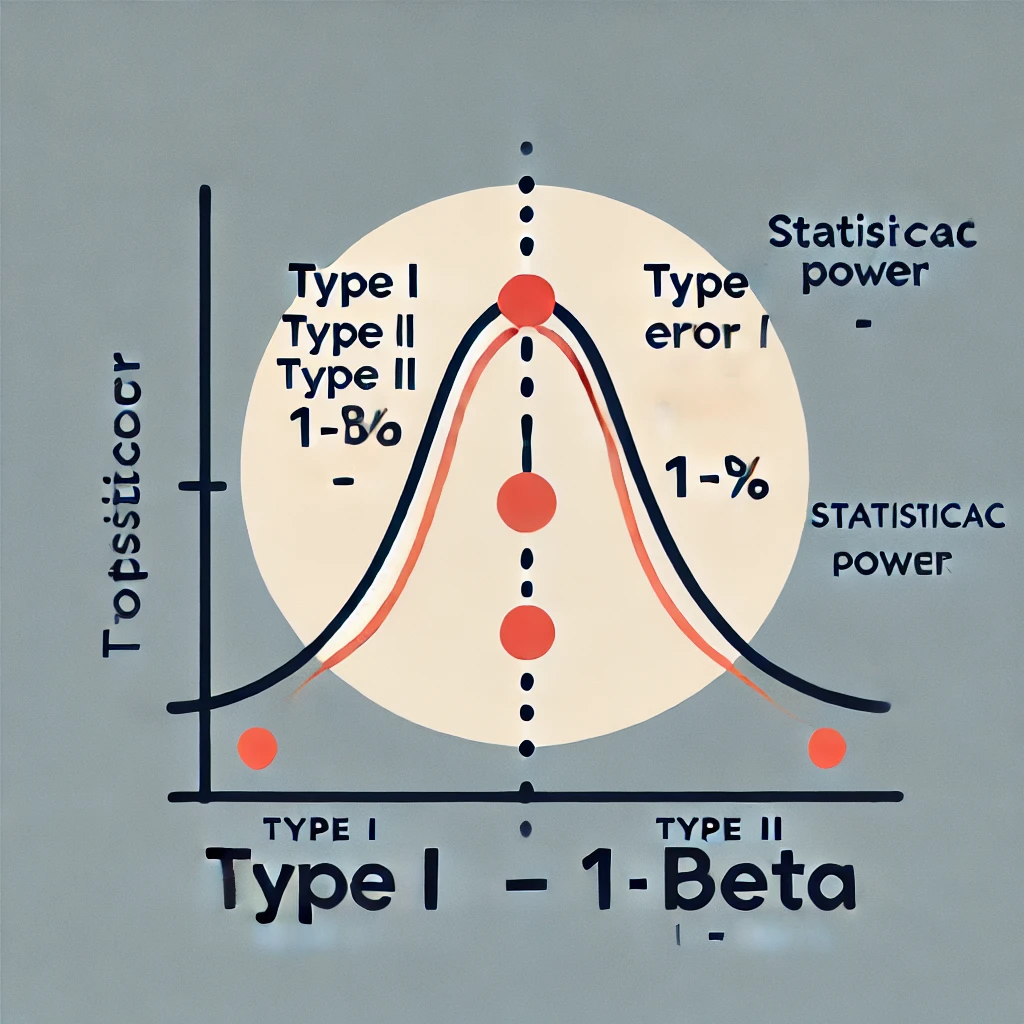
Power of a Statistical Test: Definition, Importance & Python Implementation
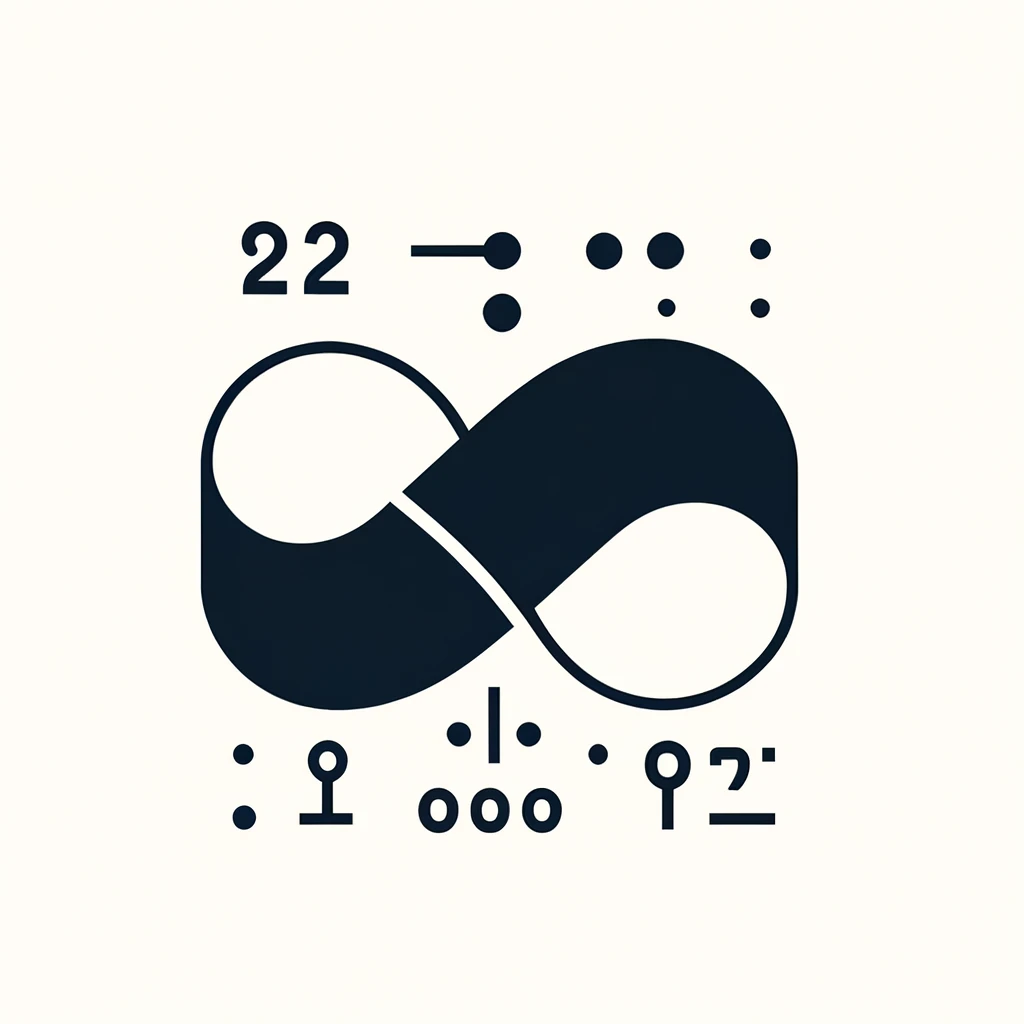
Logistic Regression & Odds Ratio: Concepts, Formula & Applications
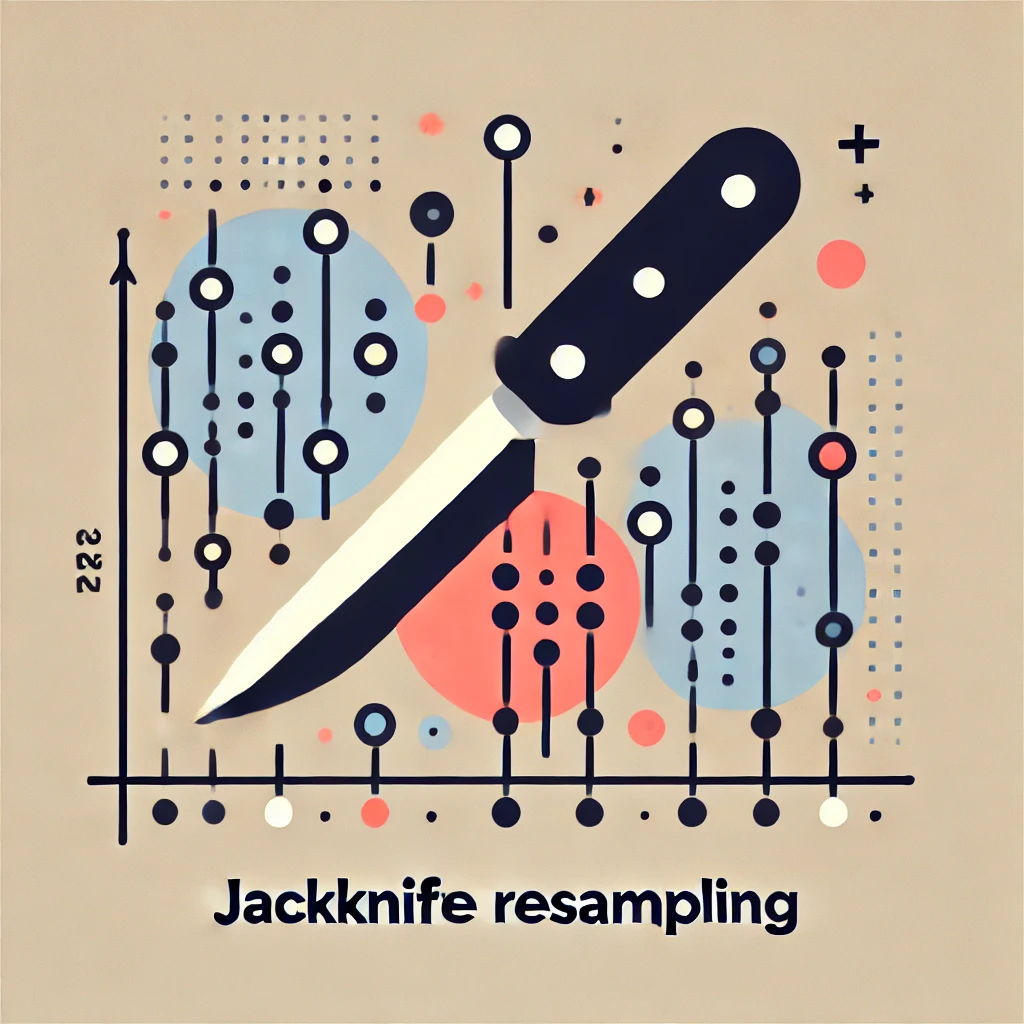
Jackknife Resampling: Concept, Steps & Applications
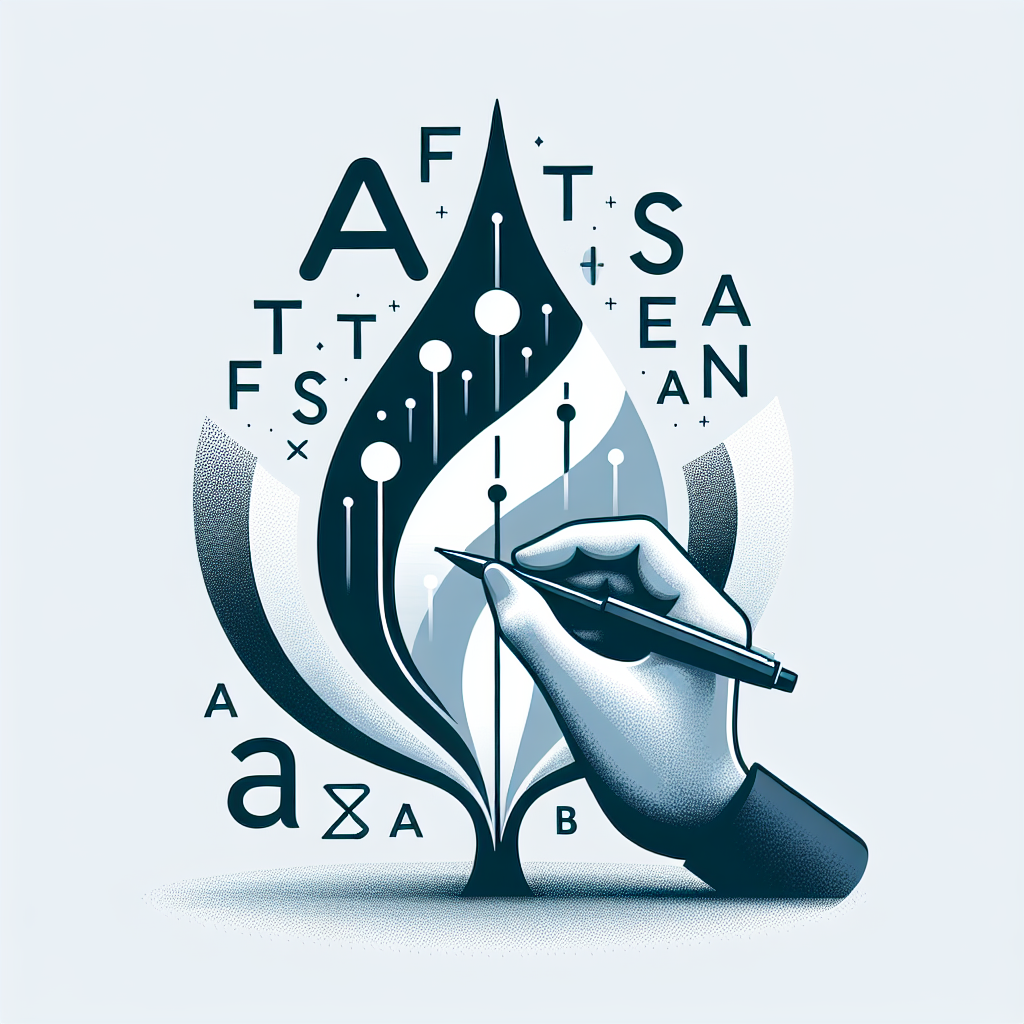
F test and Anova
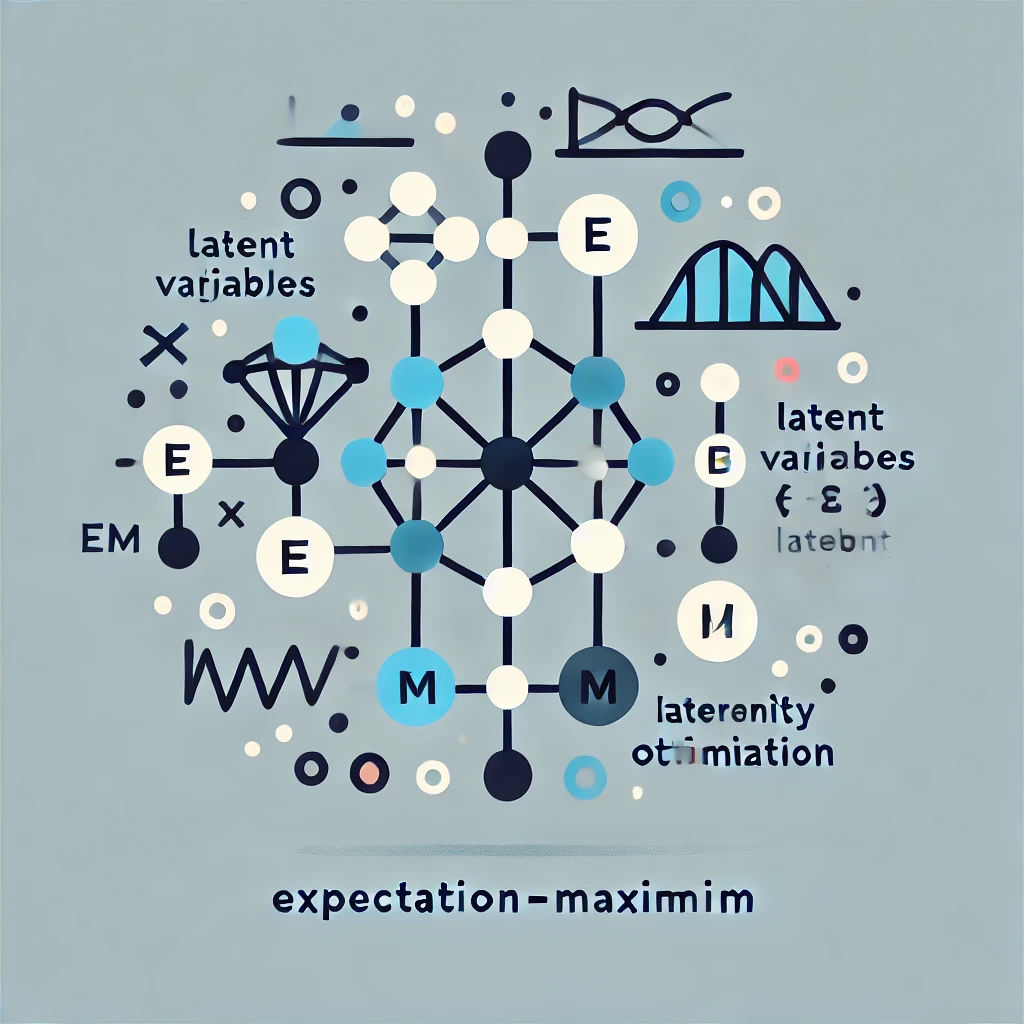