Social Media Algorithms: Navigating Organic Reach
1. Introduction: The Shifting Landscape of Organic Visibility
Social media algorithms have fundamentally transformed how content reaches audiences. What began as chronological feeds has evolved into sophisticated ranking systems that determine which content appears in users' feeds based on complex sets of signals and predictive models. As platform economics have driven social networks toward paid models, organic reach—the unpaid visibility of content—has declined dramatically, with Facebook pages experiencing drops from 16% average reach in 2012 to less than 2% today.
For marketers, this algorithmic evolution represents both challenge and opportunity. While the days of guaranteed organic visibility have disappeared, brands that deeply understand platform algorithms can still achieve significant reach without relying exclusively on paid amplification. This algorithmic literacy has become a core competency for social media marketers operating in an increasingly competitive attention economy.
This article examines how today's major social platforms prioritize content, the strategic approaches for algorithmic optimization, implementation challenges, and emerging trends affecting organic reach in an increasingly AI-driven social media landscape.
2. Algorithm Fundamentals: Platform-Specific Mechanics
Each major platform employs distinct algorithmic approaches to content distribution:
a) Platform-Specific Ranking Factors
- Instagram: Prioritizes interest (predicted relevance based on past behavior), recency, relationship strength (interaction history), and time spent with similar content.
- TikTok: Emphasizes completion rate, rewatches, engagement velocity in early distribution, and time spent before scrolling, with content tested on progressively larger audience segments.
- LinkedIn: Weights professional relevance signals, credibility indicators, engagement ratio (interactions relative to impressions), and dwell time.
- Facebook: Focuses on meaningful interactions (particularly comments and shares), relationships (prioritizing friends/family over pages), and authenticated engagement.
- Twitter: Employs a combination of recency, user interaction history, engagement velocity, and network effects in determining visibility.
b) Common Algorithmic Principles
Despite platform differences, several universal principles apply:
- Engagement Velocity: Initial reaction speed often determines wider distribution potential
- Completion Metrics: Content that holds attention receives preferential treatment
- User Relationship Signals: Consistent interaction history influences visibility
- Topic/Interest Relevance: Content matching inferred user interests reaches more users
Research from social analytics platform Emplifi found that the time window for capturing initial engagement—one of the strongest algorithmic signals—has shortened across all platforms, with the first 30-90 minutes now critical for determining a post's distribution potential.
3. Key Strategies for Algorithmic Optimization
Effective organic reach requires strategic approaches tailored to current algorithmic realities:
a) Content Format and Structure Optimization
Aligning content with algorithm-favored characteristics:
- Platform-Native Formats: Embracing each platform's preferred content types (e.g., Reels for Instagram, Carousels for LinkedIn)
- Retention-Optimized Structure: Creating patterns that maintain viewer attention throughout content
- Front-Loaded Value: Delivering key points early to survive shortened attention spans
Example: Hubspot increased its Instagram reach by 172% by shifting 60% of content to Reels format, with retention-optimized structures that maintain 65%+ completion rates through pattern interrupts and visual hooks.
b) Audience Engagement Cultivation
Developing strategic engagement approaches:
- Response Management: Actively participating in comments to trigger additional algorithmic signals
- Community Activation: Encouraging meaningful interaction beyond basic reactions
- Strategic Timing: Publishing when target audiences are most active and engaged
Example: Duolingo's TikTok strategy centers around rapid response to comments, which the company found generates 43% more algorithmic distribution than identical content without creator engagement.
c) Cross-Platform Content Adaptation
Tailoring content to platform-specific signals:
- Format Transformation: Adapting content to each platform's algorithmic preferences
- Platform-Specific Metrics Focus: Prioritizing key performance indicators that influence each algorithm
- Native Feature Utilization: Leveraging new features that typically receive algorithmic preference
Example: Chipotle's cross-platform strategy includes platform-specific adaptations of core content themes, with TikTok content optimized for completion rate (averaging 7-15 seconds) while Instagram content emphasizes saved posts and shares (primary engagement signals in that algorithm).
d) Strategic Consistency and Momentum
Building algorithmic favor through predictable patterns:
- Consistent Cadence: Maintaining regular publishing schedules to build algorithm "trust"
- Theme Development: Creating content series that build engagement momentum
- Format Consistency: Establishing recognizable patterns that audiences engage with repeatedly
Example: Morning Brew built its social reach by maintaining precisely timed daily posts across platforms, which analytics showed increased average reach by 34% compared to identical content posted irregularly.
4. The Business Impact: Measuring Algorithmic Success
Organizations implementing effective algorithmic strategies achieve measurable outcomes:
- 2-3x higher organic reach compared to industry averages
- 40-60% reduction in content promotion costs through improved organic distribution
- 15-25% higher engagement rates indicating improved content-audience alignment
- Increased algorithm "trust" reflected in consistent reach expansion over time
Case Study: A B2B Software Company's Algorithmic Approach
A software company restructured its social strategy around LinkedIn's algorithm signals, focusing on:
- Professional insight delivery in the first 3 seconds of video content
- Strategic commenting to drive conversation depth around posts
- Employee advocacy activation within the critical first hour of publishing
- Non-promotional, educational content formats that generate high dwell time
Results included:
- 217% increase in average organic reach per post
- 84% reduction in cost-per-engagement through reduced promotion needs
- 46% improvement in referral traffic to high-value landing pages
- Development of a proprietary algorithm response framework applied across all platforms
5. Implementation Challenges in Algorithm Navigation
Despite its potential, algorithmic optimization presents challenges:
a) Constant Platform Evolution
- Algorithm Updates: Frequent changes requiring continuous strategy adaptation
- Feature Prioritization Shifts: Platforms regularly altering which content types receive preference
- Signal Weighting Changes: Modifications to how engagement types influence distribution
b) Content Compromise Risks
- Format Over Substance: Prioritizing algorithmic performance over brand value
- Creative Limitation: Constraining creative expression to fit algorithmic preferences
- Platform Dependency: Becoming vulnerable to single-platform algorithm changes
c) Measurement Complexity
- Attribution Challenges: Difficulty connecting algorithmic performance to business outcomes
- Cross-Platform Comparison: Inconsistent metrics across social ecosystems
- Organic vs. Paid Delineation: Blurred boundaries between earned and paid reach
d) Resource Intensiveness
- Real-Time Monitoring: Need for constant performance assessment and adaptation
- Platform-Specific Expertise: Requiring specialized knowledge for each ecosystem
- Content Adaptation Demands: Creating multiple versions for algorithm alignment
6. The Future of Social Algorithms
Several emerging trends are reshaping algorithmic content distribution:
a) AI-Driven Personalization
- Hyper-Customized Feeds: Increasingly individualized content recommendations
- Nuanced Interest Mapping: More sophisticated understanding of content preferences
- Predictive Distribution: Algorithms anticipating content relevance before engagement
b) Creator-Focused Mechanisms
- Creator Rewards Programs: Platforms incentivizing specific creator behaviors
- Algorithmic Transparency Tools: More visibility into distribution mechanics
- Monetization Integration: Distribution increasingly tied to revenue-generating features
c) Authentic Engagement Emphasis
- Quality Over Quantity: Greater emphasis on meaningful versus surface-level engagement
- Conversation Merit: Prioritizing genuine discussion over reaction accumulation
- Value Exchange Focus: Rewarding content that delivers concrete audience benefits
d) Multi-Modal Content Preference
- Mixed Media Prioritization: Combining text, image, video and interactive elements
- Format Fluidity: Blending previously distinct content types
- Interactive Experience Emphasis: Favoring participatory over passive consumption
7. Conclusion: From Algorithm Victims to Algorithm Partners
The evolution of social media algorithms has shifted the organic reach paradigm from guaranteed visibility to earned distribution. Organizations that succeed in this environment recognize that algorithms aren't obstacles to overcome but partners to understand—complex systems designed to deliver relevant content to interested audiences.
The most effective approach treats algorithmic signals not as manipulation targets but as indicators of content value. By focusing on creating genuinely engaging content optimized for how each platform evaluates quality, brands can achieve sustainable organic reach while building genuine audience relationships that transcend algorithmic fluctuations.
8. Action Steps for Marketers
For marketing leaders navigating social algorithms:
- Develop platform-specific content strategies aligned with primary algorithmic signals
- Implement first-hour engagement plans to capitalize on velocity metrics
- Create cross-functional feedback loops between creative and analytics teams
- Build flexibility into content systems to adapt to algorithmic changes
By implementing these strategies, organizations can navigate the complex world of social algorithms not through manipulation but through creating the genuinely valuable content these systems are designed to prioritize.
Featured Blogs
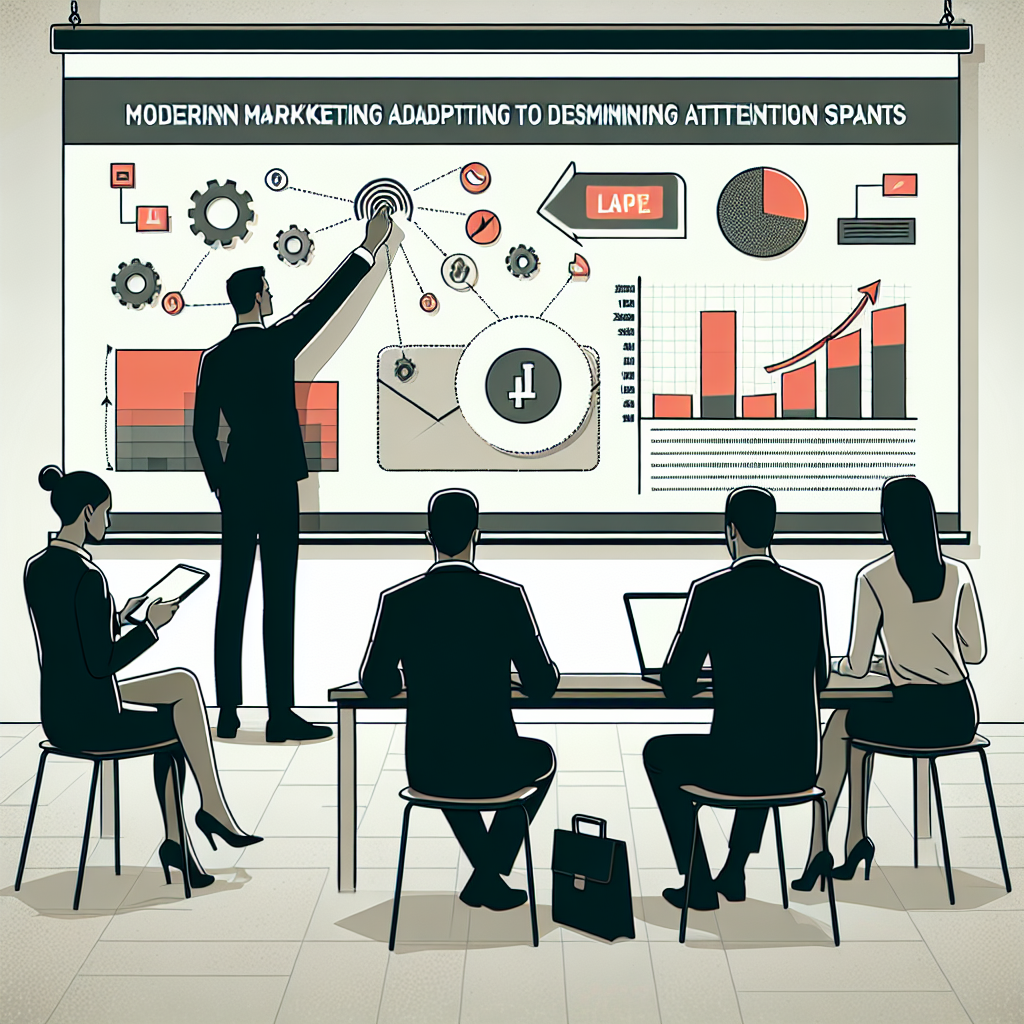
How the Attention Recession Is Changing Marketing
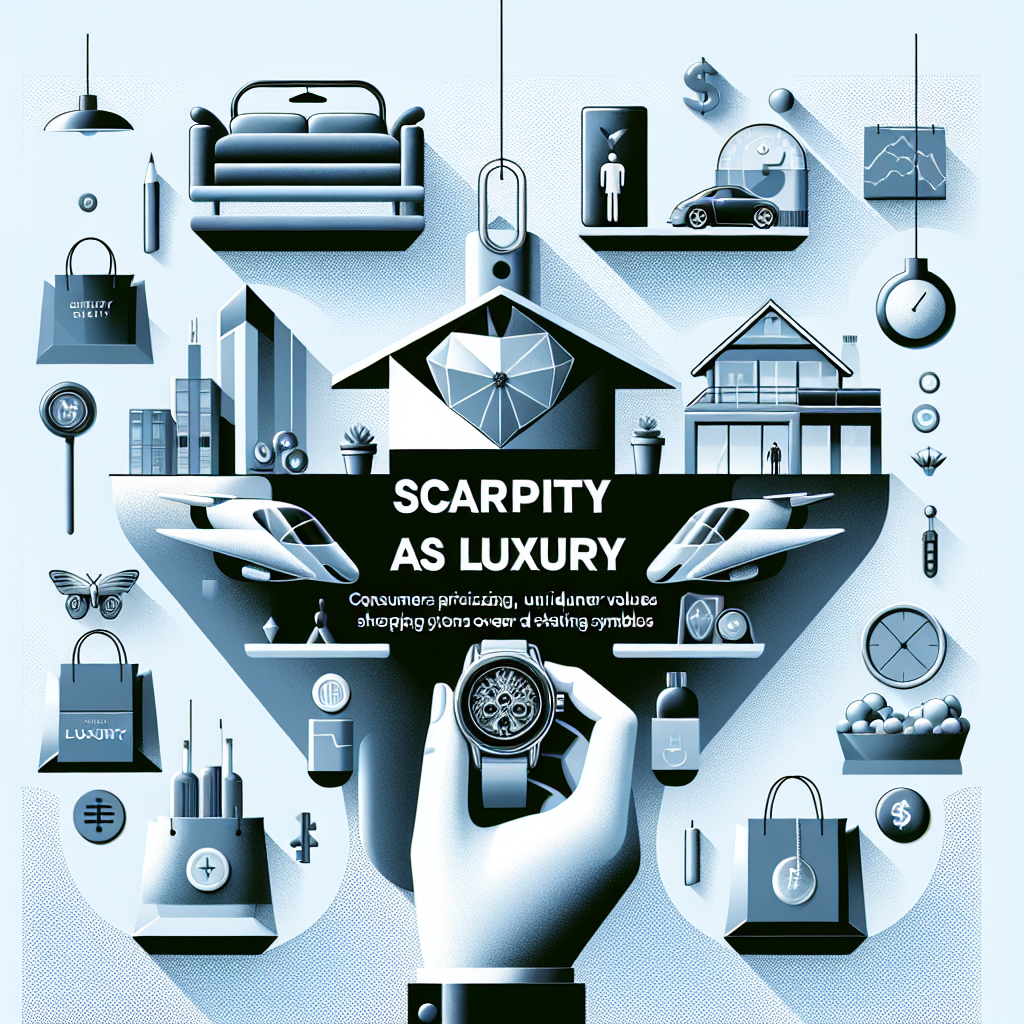
The New Luxury Why Consumers Now Value Scarcity Over Status
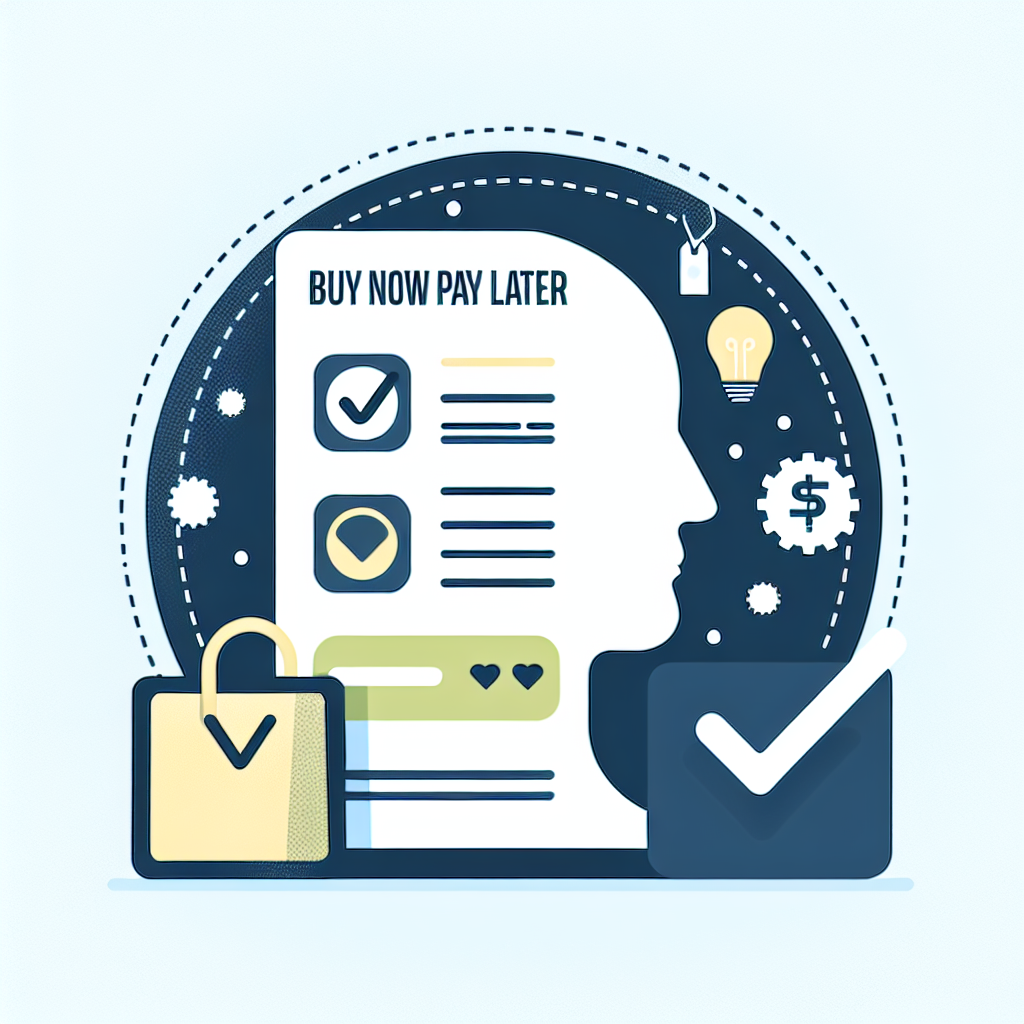
The Psychology Behind Buy Now Pay later
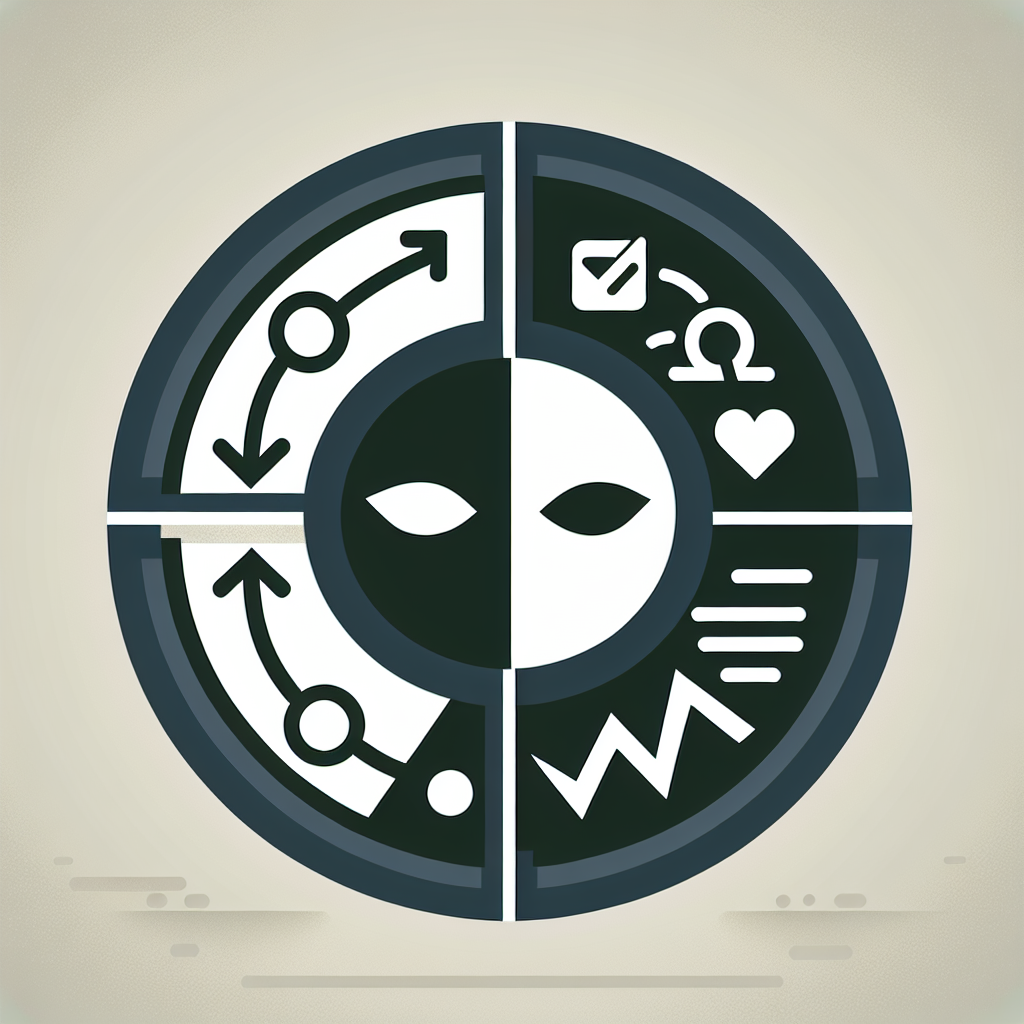
The Role of Dark Patterns in Digital Marketing and Ethical Concerns
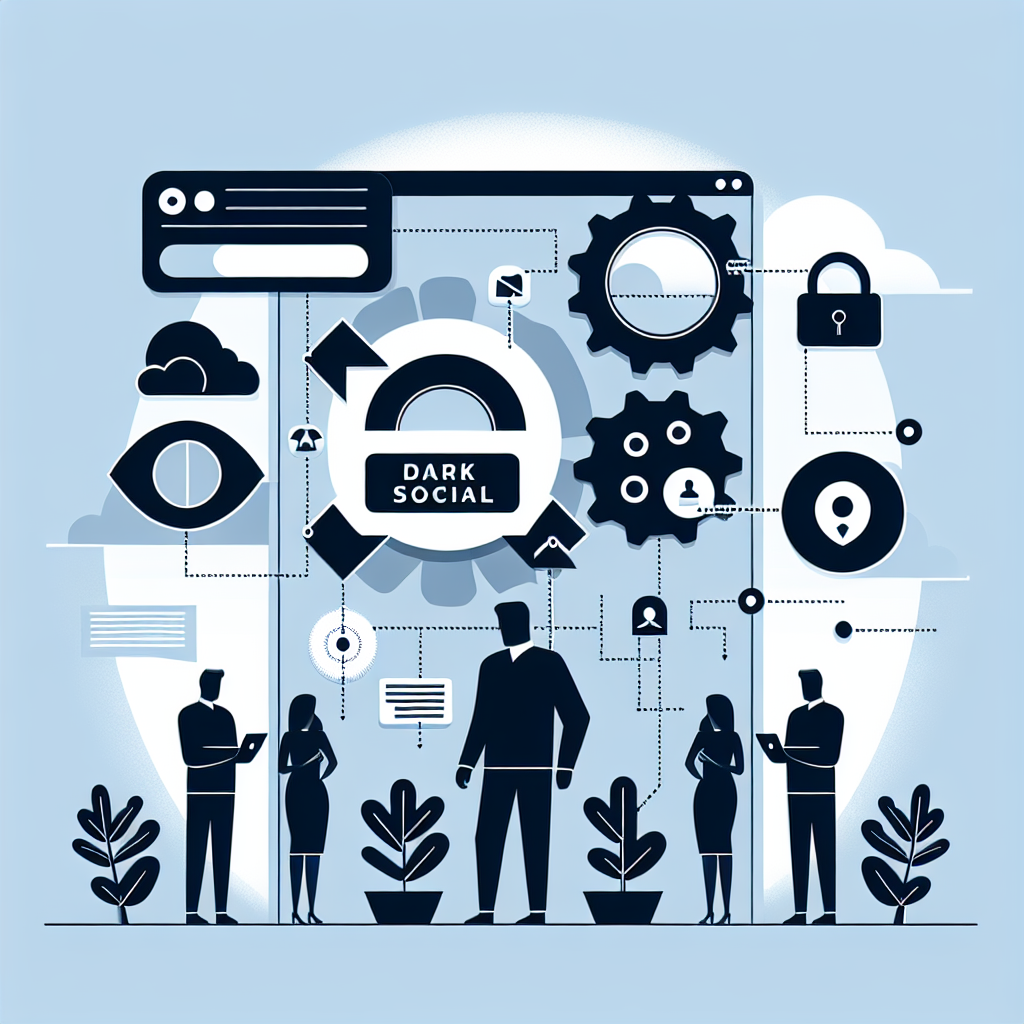
The Rise of Dark Social and Its Impact on Marketing Measurement
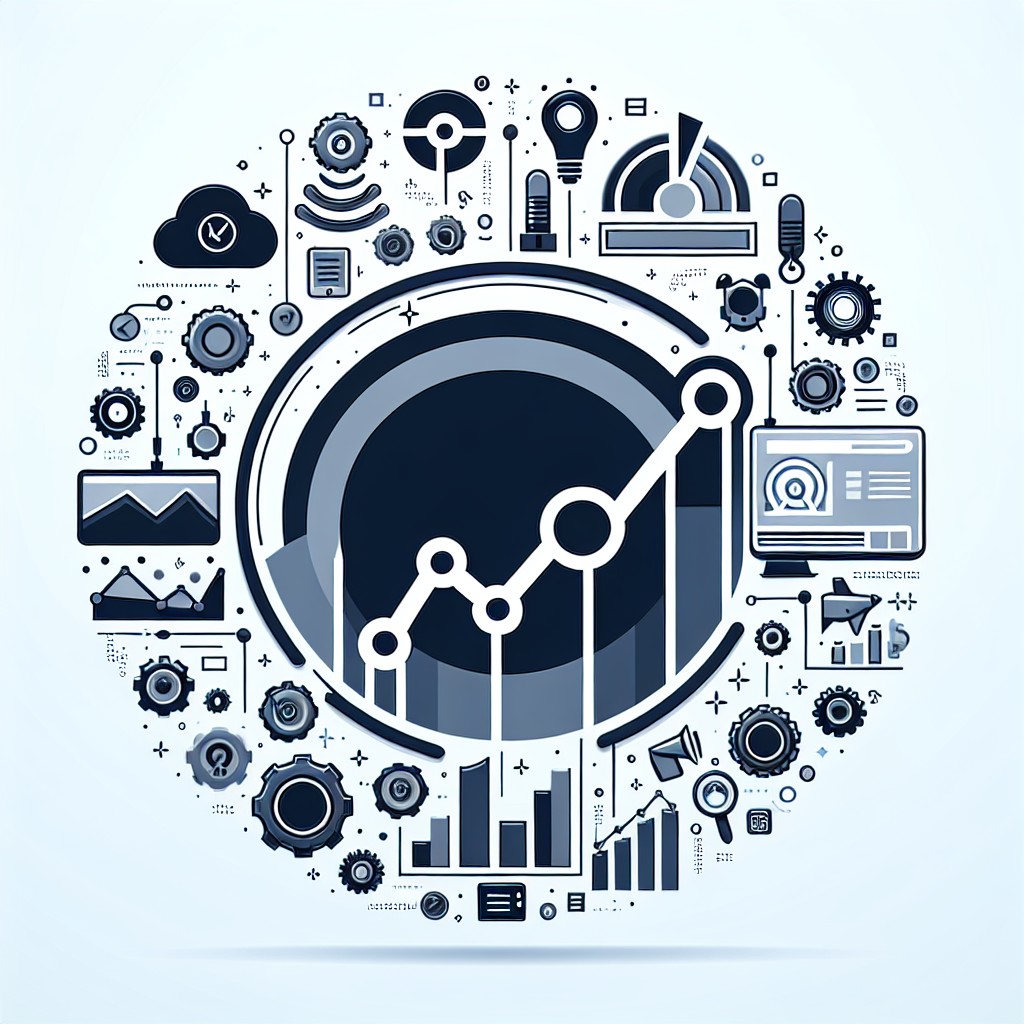