Predictive Analytics in Forecasting Market Trends
1. Introduction: The Imperative of Accurate Market Forecasting
In today's dynamic business environment, accurate market forecasting is essential for strategic decision-making, risk management, and sustainable growth. Traditional methods of market analysis, such as historical data reviews and expert opinions, often fall short in capturing the complexities and rapid shifts of modern markets. Predictive analytics, powered by machine learning and AI, offers a more robust and data-driven approach to forecasting market trends with greater precision and foresight.
By analyzing vast datasets, identifying patterns, and predicting future outcomes, predictive analytics enables businesses to anticipate market changes, optimize resource allocation, and gain a competitive advantage. This article explores how predictive analytics is transforming market forecasting, its key applications, challenges, and the future of AI-driven market intelligence.
2. How Predictive Analytics Works: The Science Behind Market Forecasting
At its core, predictive analytics leverages statistical models and AI algorithms to analyze market data and predict future trends. The process follows a structured approach:
a) Data Collection: The Foundation of Market Prediction
Predictive analytics relies on diverse data sources, including:
- Market Data: Sales figures, market share, pricing data, and product performance metrics.
- Economic Indicators: GDP growth, inflation rates, unemployment rates, and interest rates.
- Consumer Data: Demographics, psychographics, purchasing behavior, and customer feedback.
- Social Media Data: Sentiment analysis, trending topics, and social engagement metrics.
- Web Analytics: Website traffic, bounce rates, conversion rates, and keyword rankings.
b) Machine Learning Algorithms: Transforming Data into Market Insights
Predictive models range from traditional statistical techniques to advanced AI-driven approaches:
- Time Series Analysis: Forecasts future values based on historical data patterns (e.g., ARIMA, Exponential Smoothing).
- Regression Models: Identifies relationships between variables and predicts outcomes (e.g., Linear Regression, Multiple Regression).
- Neural Networks and Deep Learning: Analyzes complex, non-linear relationships for more accurate predictions (e.g., Recurrent Neural Networks, Convolutional Neural Networks).
- Clustering Algorithms: Segments markets based on similar characteristics and behaviors (e.g., K-Means Clustering, Hierarchical Clustering).
- Bayesian Models: Updates predictions based on new data and prior knowledge (e.g., Bayesian Regression, Bayesian Networks).
c) Model Training and Optimization
Predictive algorithms are trained on historical data and continuously refined through feedback loops, ensuring they remain adaptive to changing market dynamics. Key steps include:
- Data Preprocessing: Cleaning, transforming, and normalizing data to improve model accuracy.
- Feature Engineering: Selecting and transforming relevant variables for model training.
- Model Validation: Testing model performance on unseen data to ensure generalization.
- Continuous Monitoring: Tracking model accuracy and retraining as needed to maintain performance.
3. Key Applications of Predictive Analytics in Market Forecasting
Predictive analytics is revolutionizing market forecasting across multiple domains:
a) Demand Forecasting and Inventory Optimization
- Description: Predicting future demand to optimize stock levels, reduce waste, and minimize lost sales.
- Example: Walmart uses predictive analytics to forecast demand for thousands of products, optimizing inventory levels and reducing stockouts.
b) Sales Forecasting and Revenue Projections
- Description: Predicting future sales performance to set targets, allocate resources, and manage revenue expectations.
- Example: Salesforce uses predictive analytics to forecast sales leads, identify high-potential customers, and optimize sales strategies.
c) Market Segmentation and Targeting
- Description: Identifying and segmenting market segments based on behavioral patterns, enabling targeted marketing campaigns.
- Example: Procter & Gamble uses predictive analytics to segment consumers based on purchasing behavior, tailoring marketing messages and product offerings to specific segments.
d) Trend Identification and Emerging Opportunities
- Description: Identifying emerging market trends and opportunities by analyzing data from various sources, including social media, web analytics, and market research.
- Example: Google Trends uses predictive analytics to identify trending topics and emerging opportunities in various industries.
e) Competitive Analysis and Benchmarking
- Description: Analyzing competitor activities and benchmarking performance to identify strengths, weaknesses, and opportunities for improvement.
- Example: Nielsen uses predictive analytics to track competitor sales, market share, and pricing strategies, providing insights for competitive decision-making.
4. The Business Impact: Quantifying the Value of Predictive Market Forecasting
The adoption of predictive analytics yields measurable business outcomes:
- Improved Forecasting Accuracy: Reducing forecasting errors by 20-50%.
- Increased Revenue: Driving revenue growth through optimized inventory management and targeted marketing campaigns.
- Reduced Costs: Lowering costs through improved resource allocation and reduced waste.
- Enhanced Decision-Making: Improving strategic decision-making with data-driven insights.
5. Challenges in Implementing Predictive Analytics for Market Forecasting
Despite its potential, predictive analytics comes with challenges:
a) Data Quality and Integration
- Challenge: Incomplete, outdated, or biased data can lead to inaccurate predictions.
- Solution: Implement data governance policies and invest in data quality tools to ensure data accuracy and completeness.
b) Model Complexity and Interpretability
- Challenge: Complex AI-driven models can be difficult to interpret, making it challenging to understand the drivers of predictions.
- Solution: Use interpretable machine learning techniques and provide transparent model documentation to improve understanding and trust.
c) Skill Gap and Talent Acquisition
- Challenge: Lack of skilled data scientists and analysts can hinder the implementation and maintenance of predictive analytics models.
- Solution: Invest in training programs and partnerships with academic institutions to develop internal talent and attract external expertise.
d) Organizational Alignment and Collaboration
- Challenge: Predictive analytics requires collaboration across marketing, sales, and IT teams.
- Solution: Foster a data-driven culture and establish cross-functional teams to promote collaboration and knowledge sharing.
6. The Future of Predictive Analytics in Market Forecasting
Predictive analytics is evolving beyond static forecasting into real-time, AI-driven decision-making:
a) Real-Time Market Intelligence
- Trend: AI-driven platforms that provide real-time market insights and predictive alerts, enabling businesses to respond quickly to changing market conditions.
b) Predictive Scenario Planning
- Trend: AI models that simulate various market scenarios and predict the impact of different decisions, enabling businesses to make more informed strategic choices.
c) Automated Forecasting and Optimization
- Trend: AI-powered tools that automate the entire forecasting process, from data collection to model training to deployment, reducing the need for manual intervention.
7. Conclusion: Turning Prediction into Action
Predictive analytics is no longer a futuristic concept—it is a critical enabler of modern market forecasting. Companies that leverage AI-driven forecasting gain a strategic edge through:
- Enhanced market insights and trend identification.
- Optimized resource allocation and reduced costs.
- Proactive risk management and improved decision-making.
- Real-time, automated decision-making for marketing and sales execution.
However, successful implementation requires more than just technology—it demands a data-driven culture, cross-functional collaboration, and an agile approach to market intelligence. The future belongs to organizations that can not only predict market trends but also translate those insights into dynamic, automated, and highly personalized strategies.
Call to Action
For marketing and business leaders looking to scale predictive analytics, the focus should be on:
- Investing in AI-driven market intelligence platforms.
- Building strong data infrastructure and governance.
- Creating cross-functional teams that bridge marketing, sales, and data science.
- Fostering a culture of data-driven decision-making.
The organizations that execute on these priorities will not just forecast the future—they will shape it.
Featured Blogs
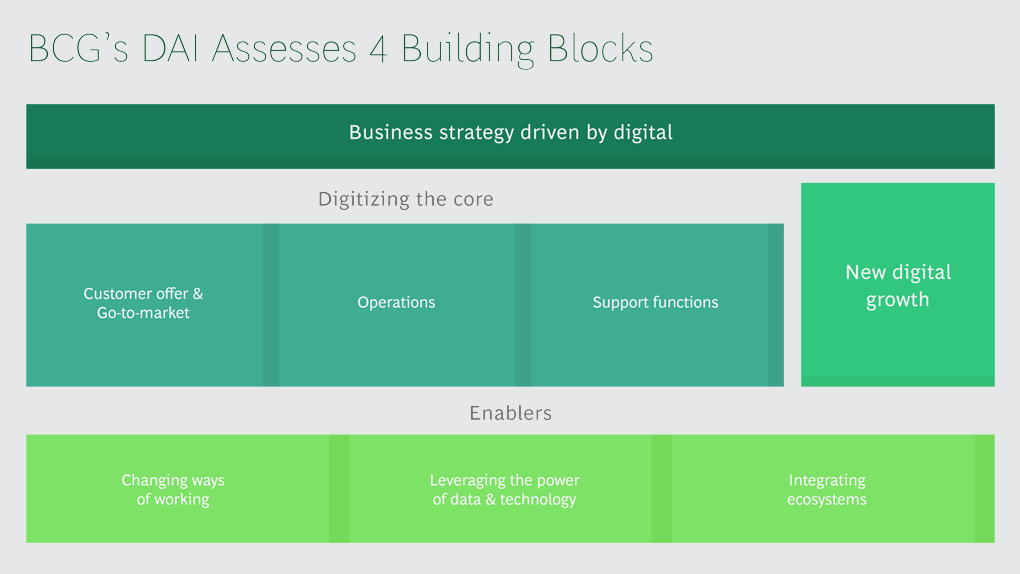
BCG Digital Acceleration Index
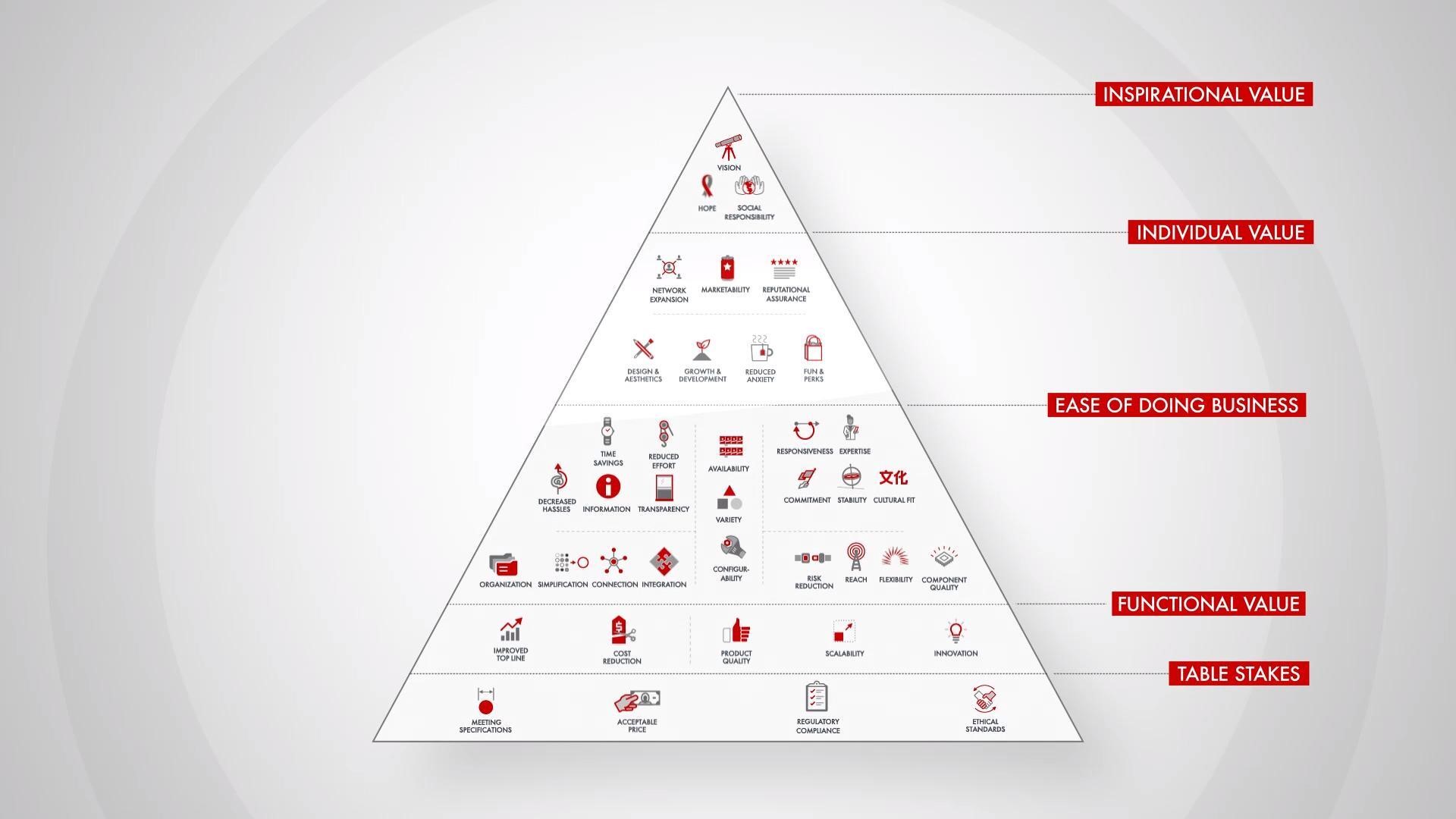
Bain’s Elements of Value Framework
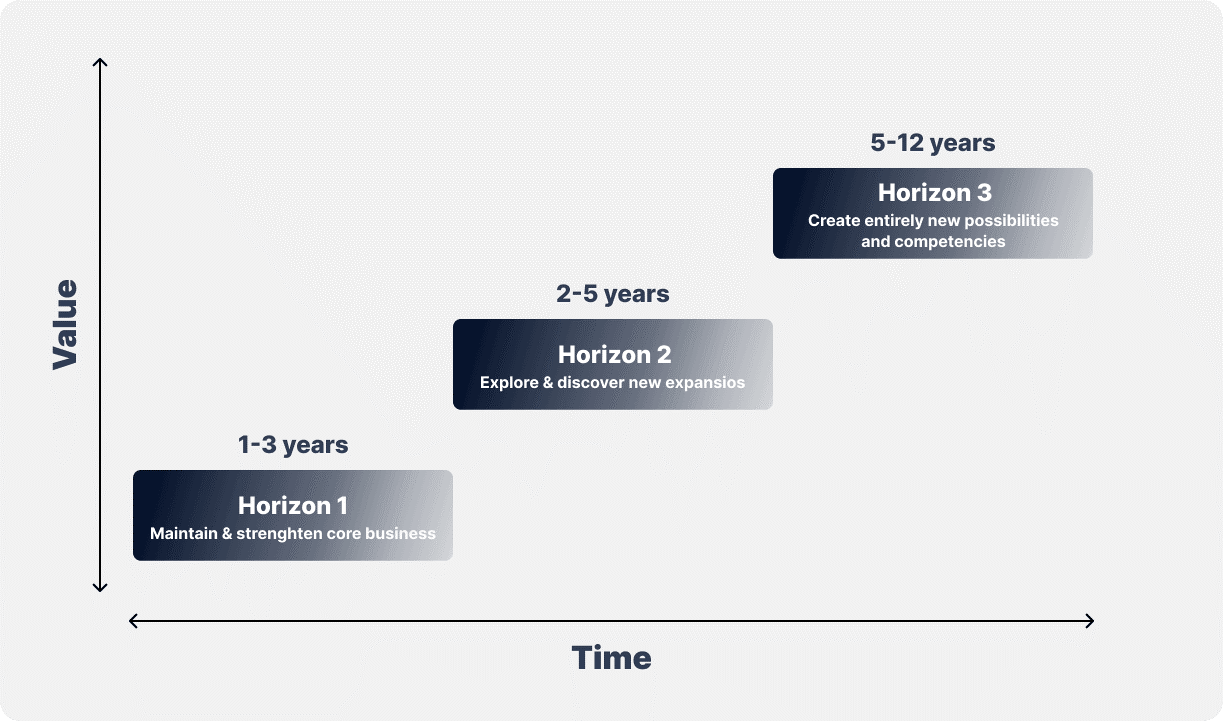
McKinsey Growth Pyramid
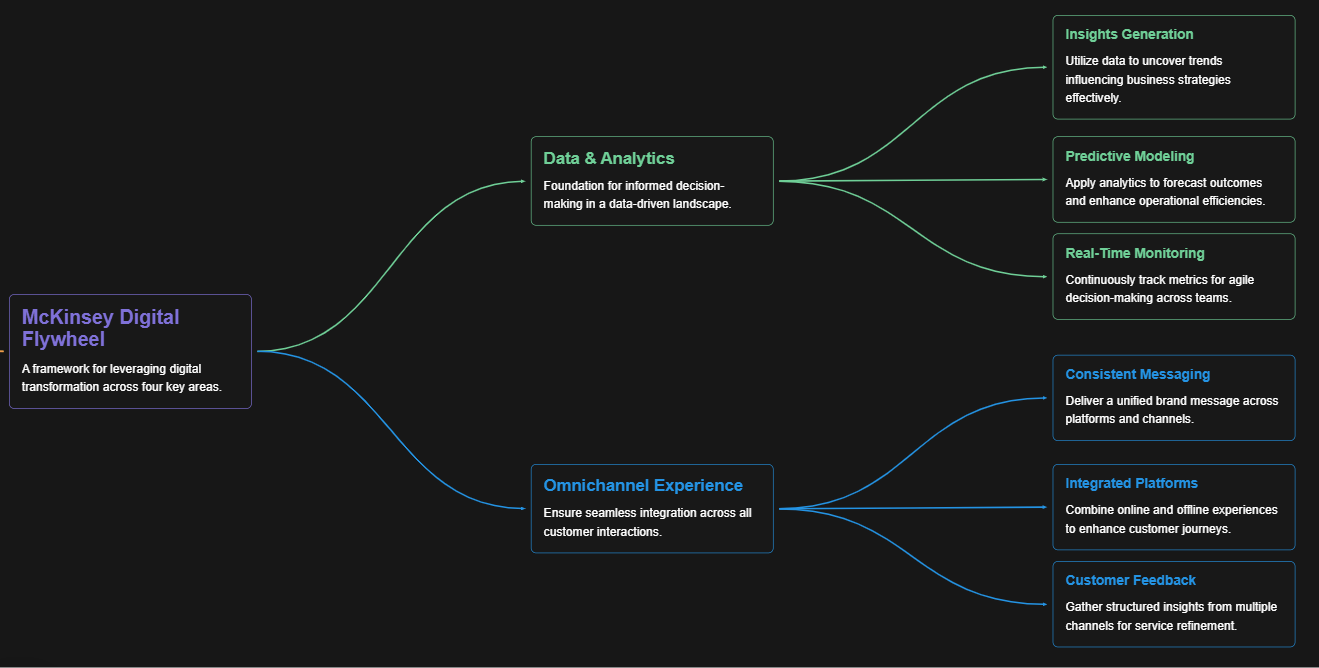
McKinsey Digital Flywheel
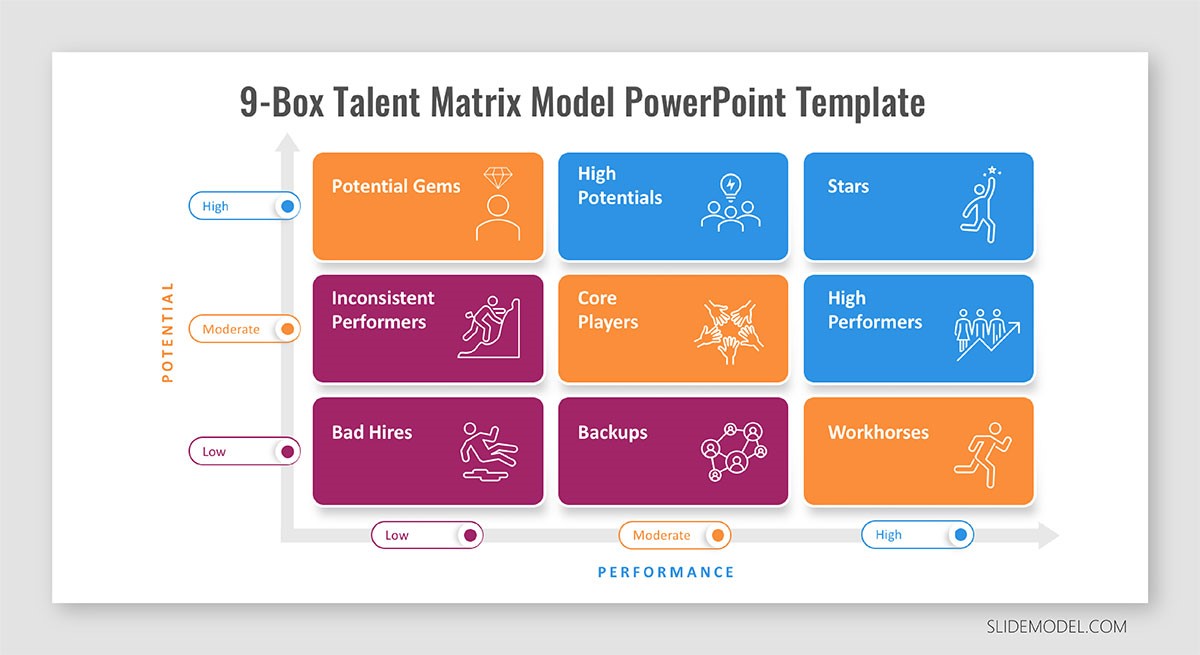
McKinsey 9-Box Talent Matrix
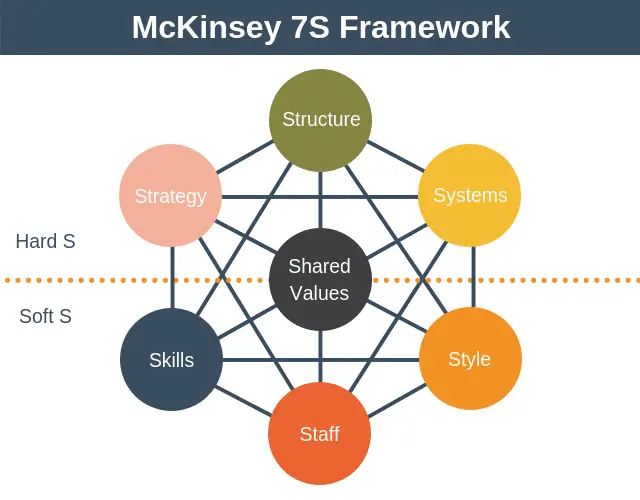
McKinsey 7S Framework
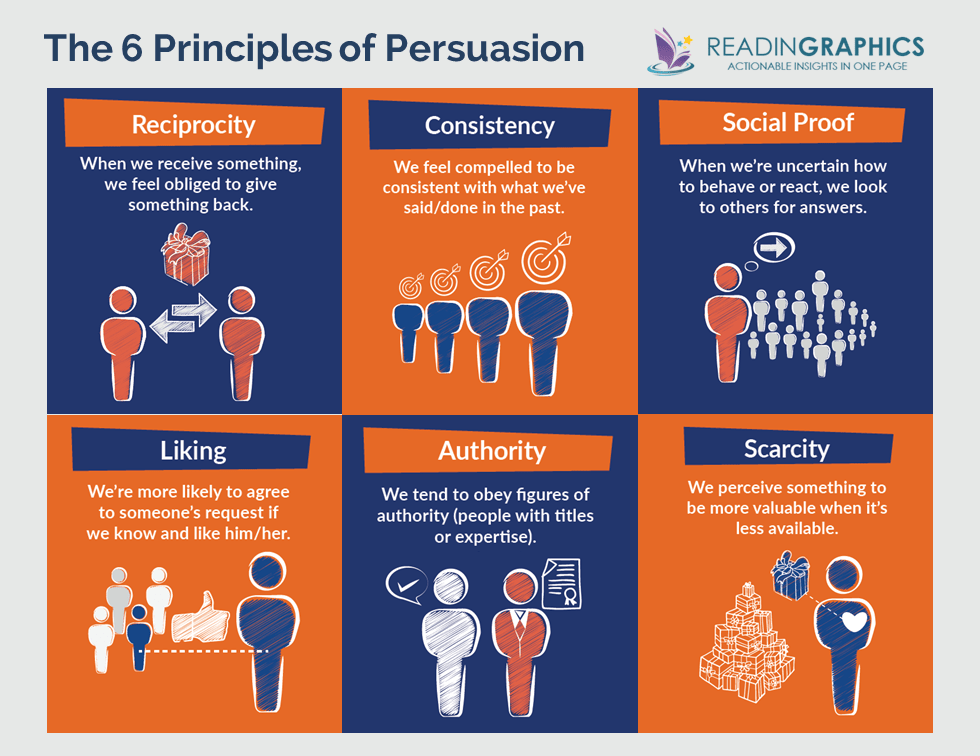
The Psychology of Persuasion in Marketing
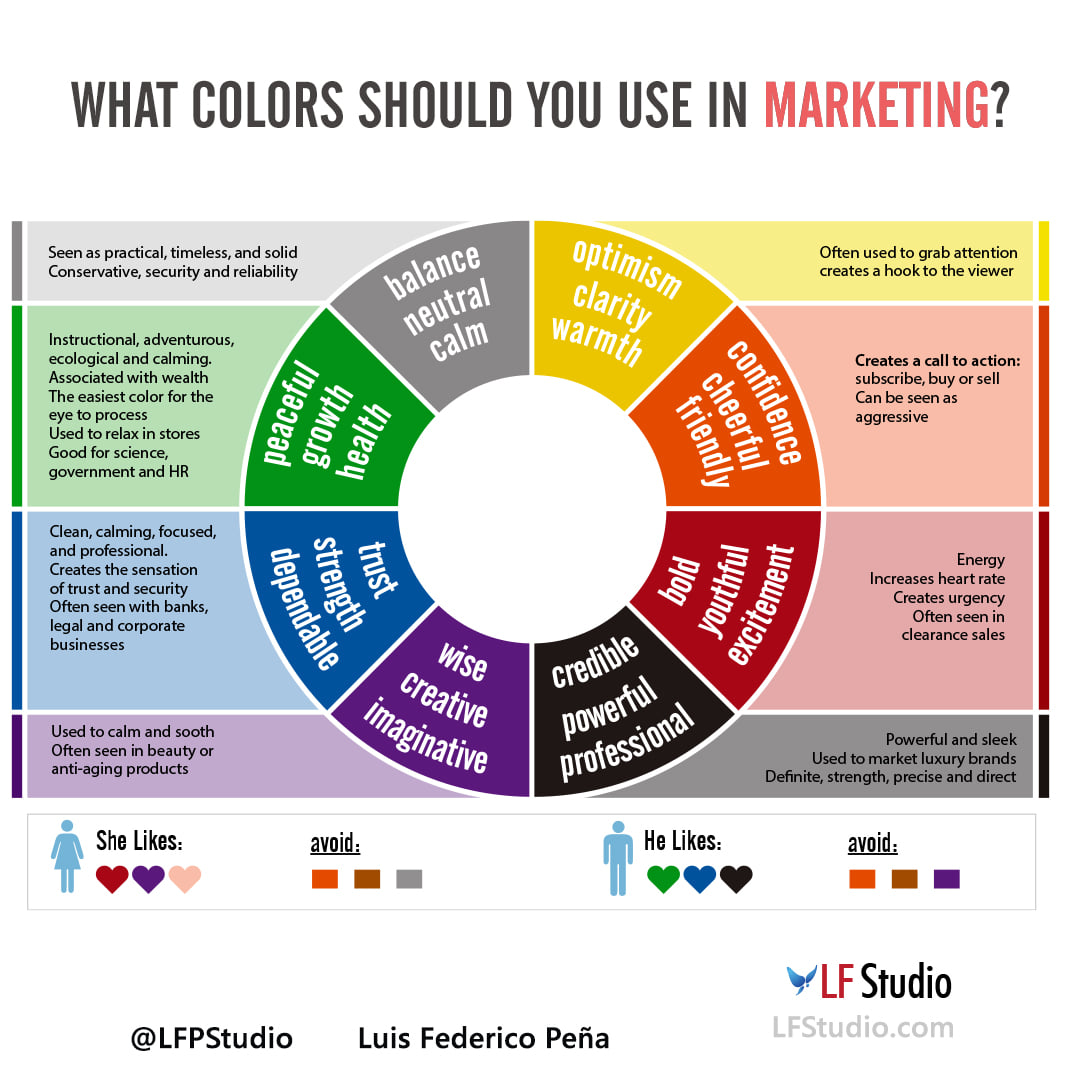
The Influence of Colors on Branding and Marketing Psychology
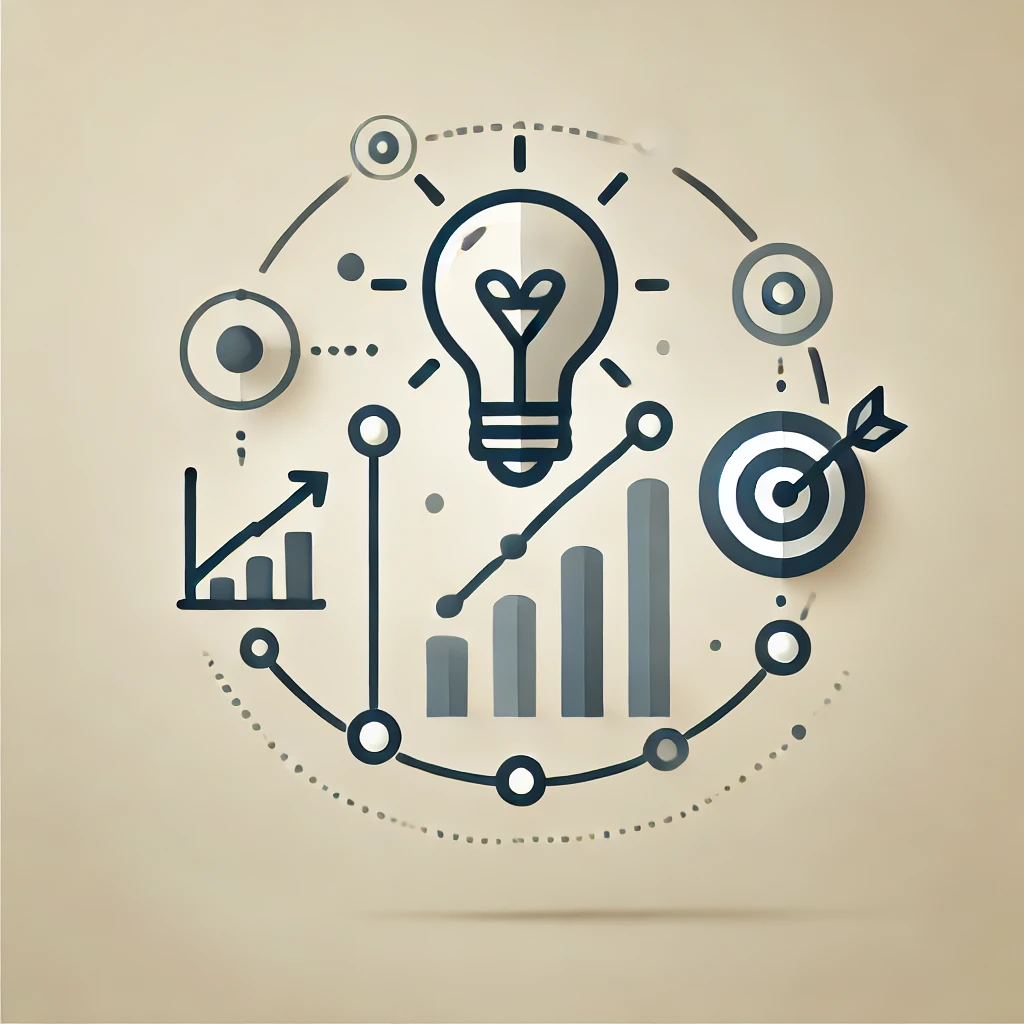