Implementing Customer Lifetime Value (CLV) Models: A Strategic Approach to Long-Term Customer Relationships
1. Introduction: Reimagining Customer Value in the Digital Era
Customer Lifetime Value (CLV) has emerged as a critical metric transforming how businesses conceptualize, acquire, and retain customers. In an increasingly competitive marketplace, understanding the long-term economic value of each customer has become paramount. Traditional transactional approaches are giving way to sophisticated, data-driven models that view customers as strategic assets rather than short-term revenue sources.
Renowned marketing strategist Peter Drucker's insight that "the purpose of business is to create a customer" has never been more relevant. Modern organizations are shifting from acquisition-focused strategies to comprehensive value creation ecosystems that prioritize sustainable, long-term customer relationships.
2. Fundamentals of Customer Lifetime Value (CLV)
Defining CLV
Core components of comprehensive CLV models:
- Predictive economic valuation Explanation: Calculating the total revenue a business can reasonably expect from a single customer account throughout their relationship.
- Holistic customer relationship assessment Explanation: Moving beyond immediate transaction values to understand the complete potential of a customer relationship.
- Dynamic value projection Explanation: Continuously updating customer value estimates based on evolving interactions and behaviors.
Mathematical Foundations
Key calculation approaches:
- Predictive modeling techniques Explanation: Utilizing advanced statistical methods and machine learning algorithms to forecast future customer value.
- Probabilistic customer behavior analysis Explanation: Developing sophisticated models that account for variability in customer interactions and spending patterns.
- Multi-dimensional value assessment Explanation: Incorporating tangible and intangible factors that contribute to long-term customer value.
3. Technological Enablers of Advanced CLV Models
AI and Machine Learning Integration
Transformative technological capabilities:
- Predictive analytics Explanation: Leveraging artificial intelligence to generate more accurate and nuanced customer value predictions.
- Real-time value optimization Explanation: Dynamically adjusting customer strategies based on continuous data analysis.
- Advanced segmentation techniques Explanation: Creating highly granular customer groupings based on comprehensive value potential.
Case Study: Amazon's CLV Approach Strategic implementation highlights:
- Personalized recommendation engine Explanation: Utilizing sophisticated algorithms to maximize individual customer value through targeted suggestions.
- Predictive purchasing models Explanation: Anticipating customer needs before they become explicit requirements.
- Loyalty program optimization Explanation: Designing targeted incentives that enhance long-term customer retention and value.
4. Strategic Implementation Frameworks
Comprehensive CLV Development
Key strategic considerations:
- Data infrastructure development Explanation: Creating robust, integrated data collection and analysis systems.
- Cross-functional collaboration Explanation: Aligning marketing, sales, customer service, and technology teams around CLV objectives.
- Continuous learning mechanisms Explanation: Establishing adaptive systems that continuously refine customer value understanding.
Measurement and Optimization Strategies
Critical implementation approaches:
- Granular customer segmentation Explanation: Developing nuanced customer categories based on comprehensive value potential.
- Predictive intervention strategies Explanation: Proactively addressing potential customer churn or value reduction.
- Personalized engagement design Explanation: Crafting unique interaction strategies for different customer value segments.
5. Challenges in CLV Implementation
Complex Implementation Considerations
Key obstacles and solutions:
- Data quality and integration Explanation: Overcoming challenges in collecting and synthesizing comprehensive customer data.
- Technological complexity Explanation: Managing sophisticated analytical tools and infrastructure requirements.
- Organizational cultural adaptation Explanation: Shifting from transactional to relationship-centered business perspectives.
6. Emerging Trends in CLV Modeling
Future-Forward Approaches
Innovative development directions:
- Emotional value integration Explanation: Incorporating psychological and emotional factors into value calculations.
- Predictive ecosystem modeling Explanation: Understanding customer value across interconnected product and service landscapes.
- Ethical data utilization Explanation: Balancing advanced analytics with robust privacy and consent mechanisms.
7. Conclusion: The Strategic Imperative of CLV
The evolution of Customer Lifetime Value represents a fundamental transformation in business strategy:
- Holistic customer relationship management Explanation: Moving beyond transactional interactions to comprehensive value creation.
- Data-driven strategic decision-making Explanation: Utilizing advanced analytics to inform long-term business strategies.
- Continuous value optimization Explanation: Developing adaptive systems that dynamically enhance customer relationships.
CLV is no longer a theoretical concept but a critical strategic imperative that demands sophisticated, technology-enabled approaches to customer understanding and engagement.
Call to Action
For leaders seeking to implement advanced CLV strategies:
- Develop comprehensive data infrastructure Explanation: Invest in robust, integrated data collection and analysis capabilities.
- Foster cross-functional collaboration Explanation: Create organizational structures that prioritize holistic customer value understanding.
- Implement advanced analytical capabilities Explanation: Leverage AI and machine learning to enhance predictive customer value modeling.
- Prioritize ethical data utilization Explanation: Establish transparent, consent-driven approaches to customer data management.
- Embrace continuous learning Explanation: Create adaptive systems that continuously refine customer value understanding.
The future of business lies in transforming customer relationships from transactional interactions to strategic, value-driven partnerships.
Featured Blogs
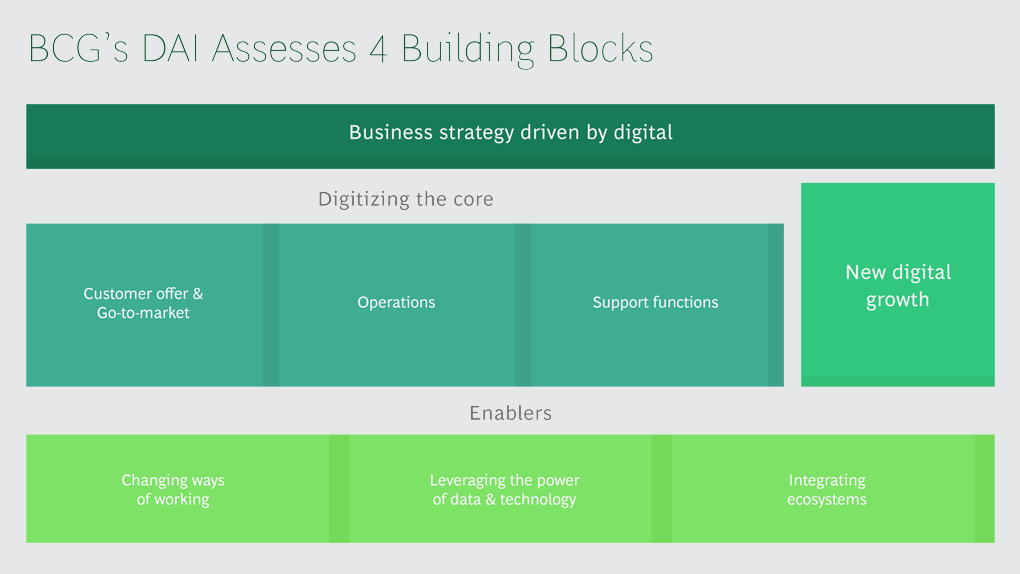
BCG Digital Acceleration Index
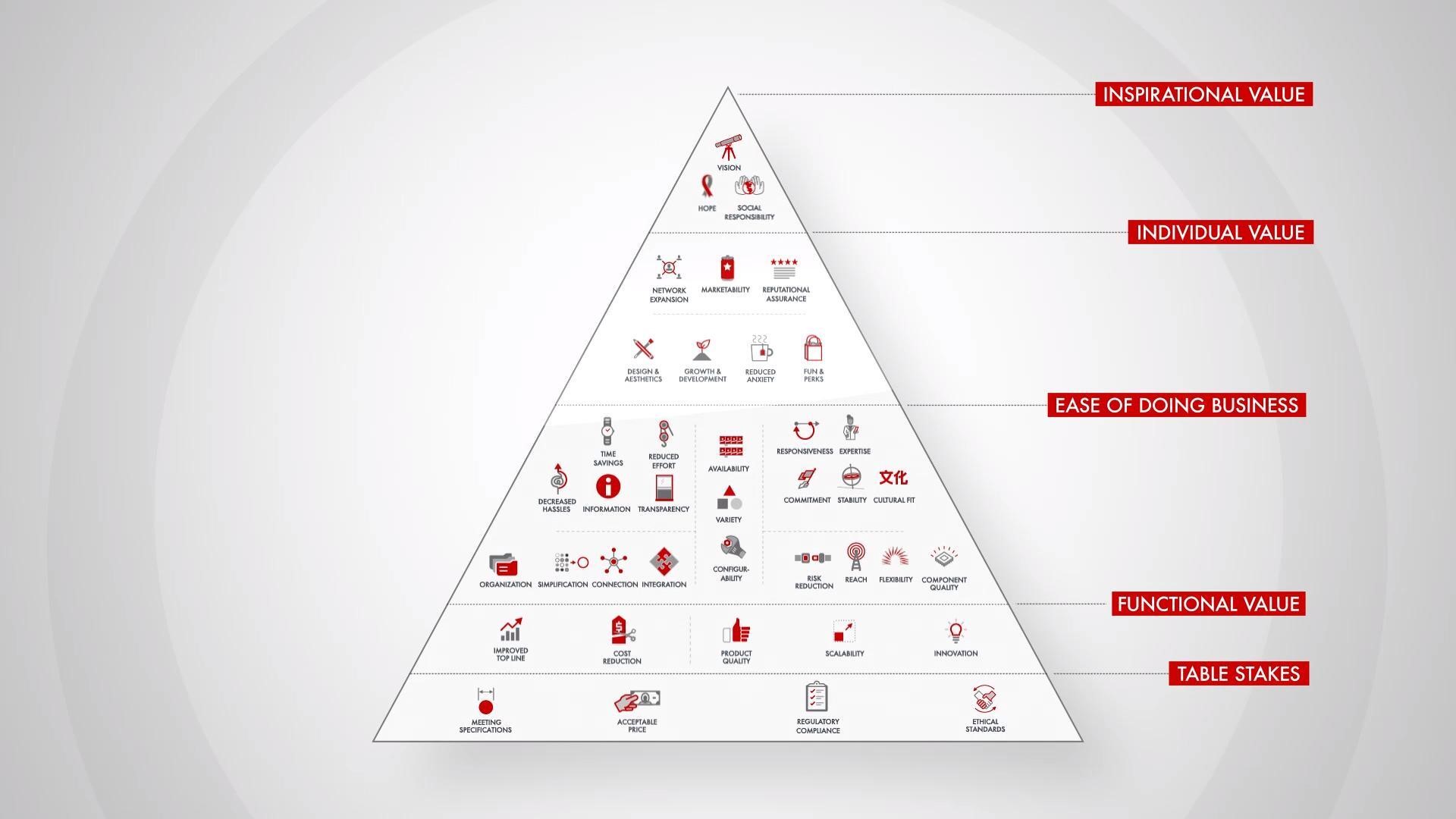
Bain’s Elements of Value Framework
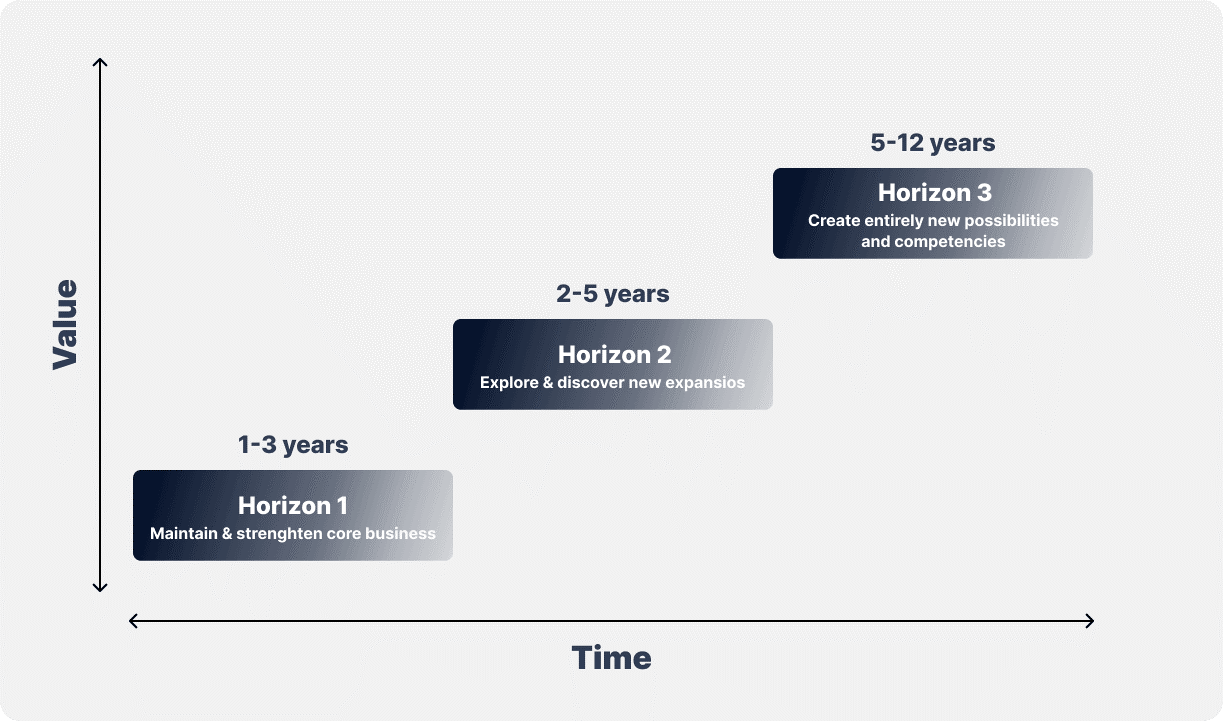
McKinsey Growth Pyramid
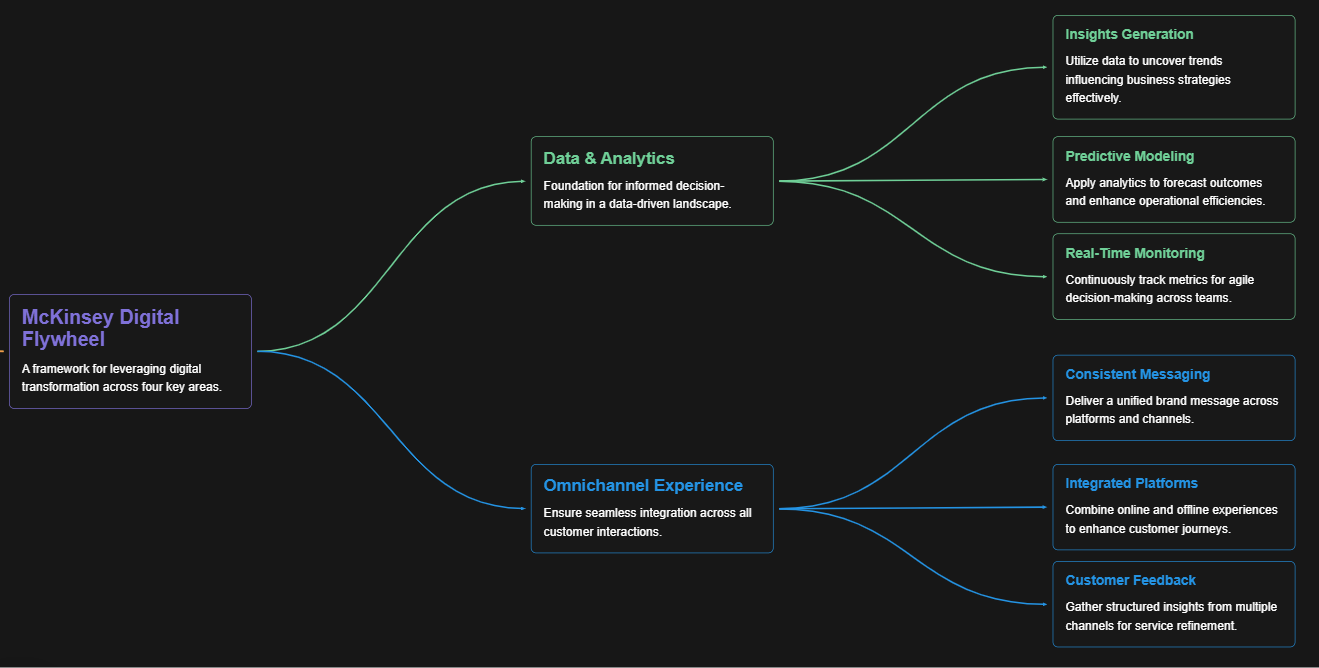
McKinsey Digital Flywheel
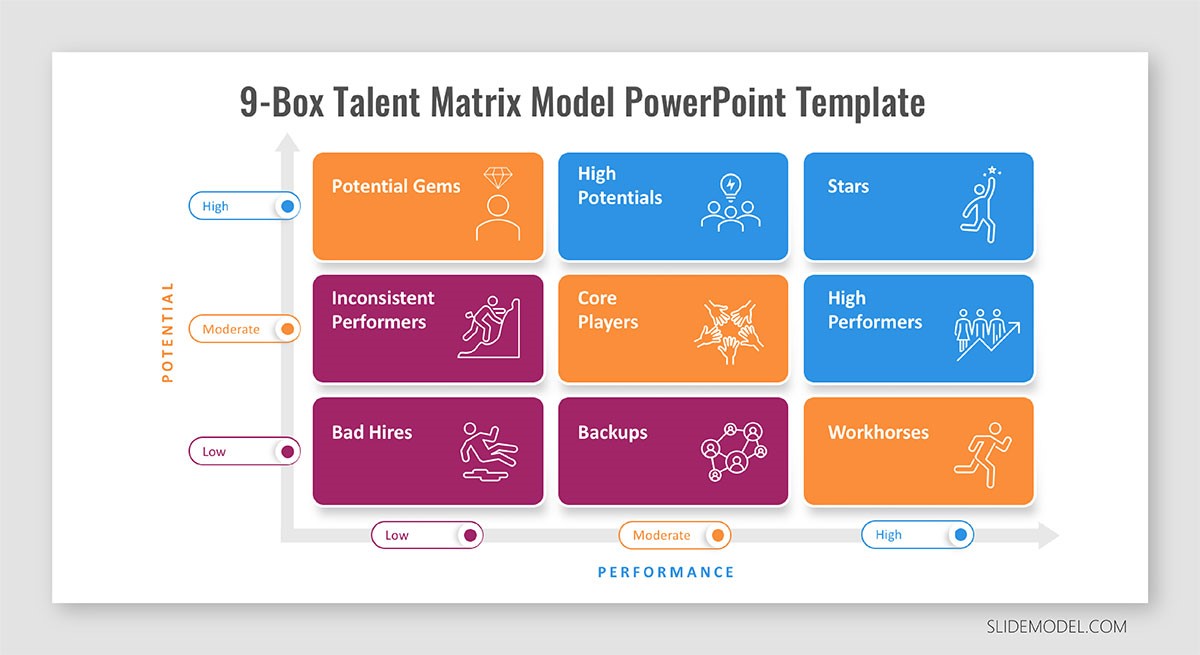
McKinsey 9-Box Talent Matrix
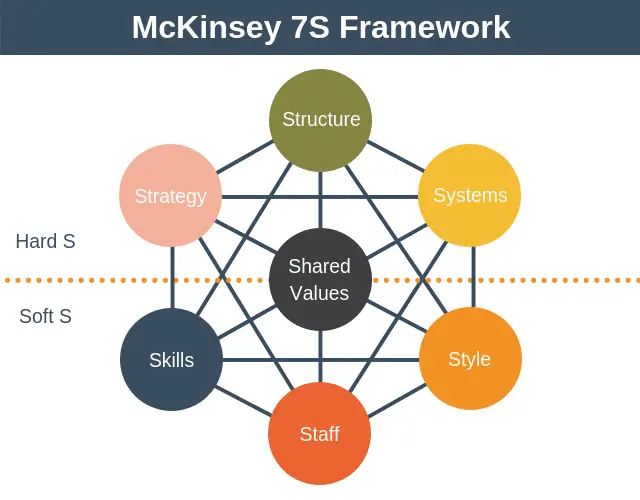
McKinsey 7S Framework
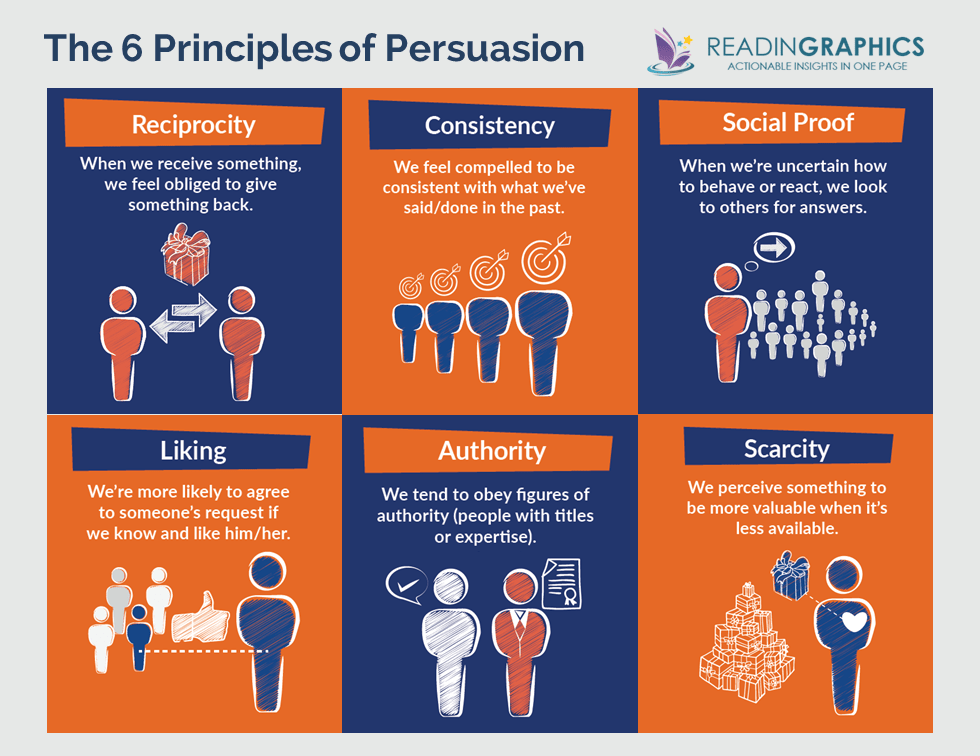
The Psychology of Persuasion in Marketing
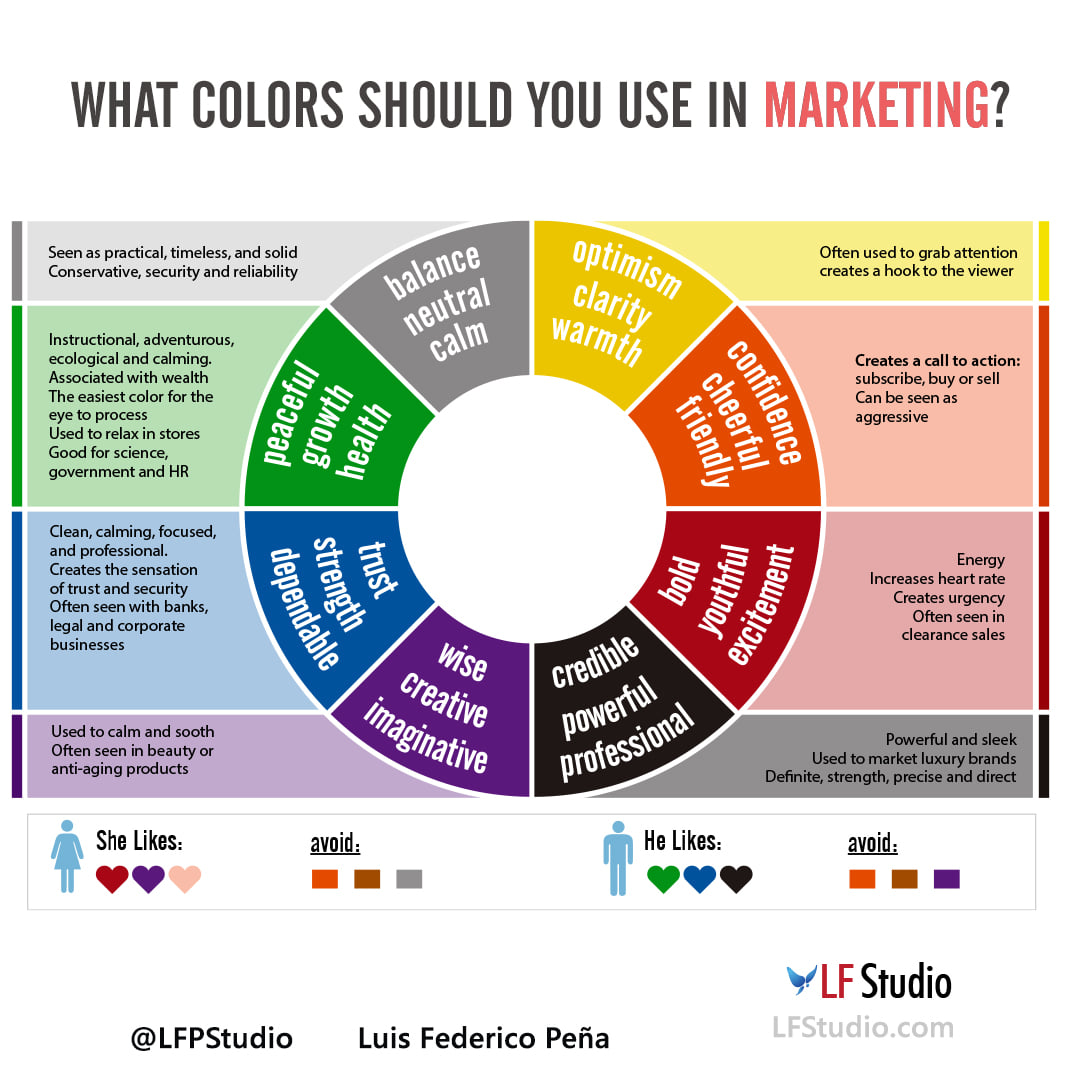
The Influence of Colors on Branding and Marketing Psychology
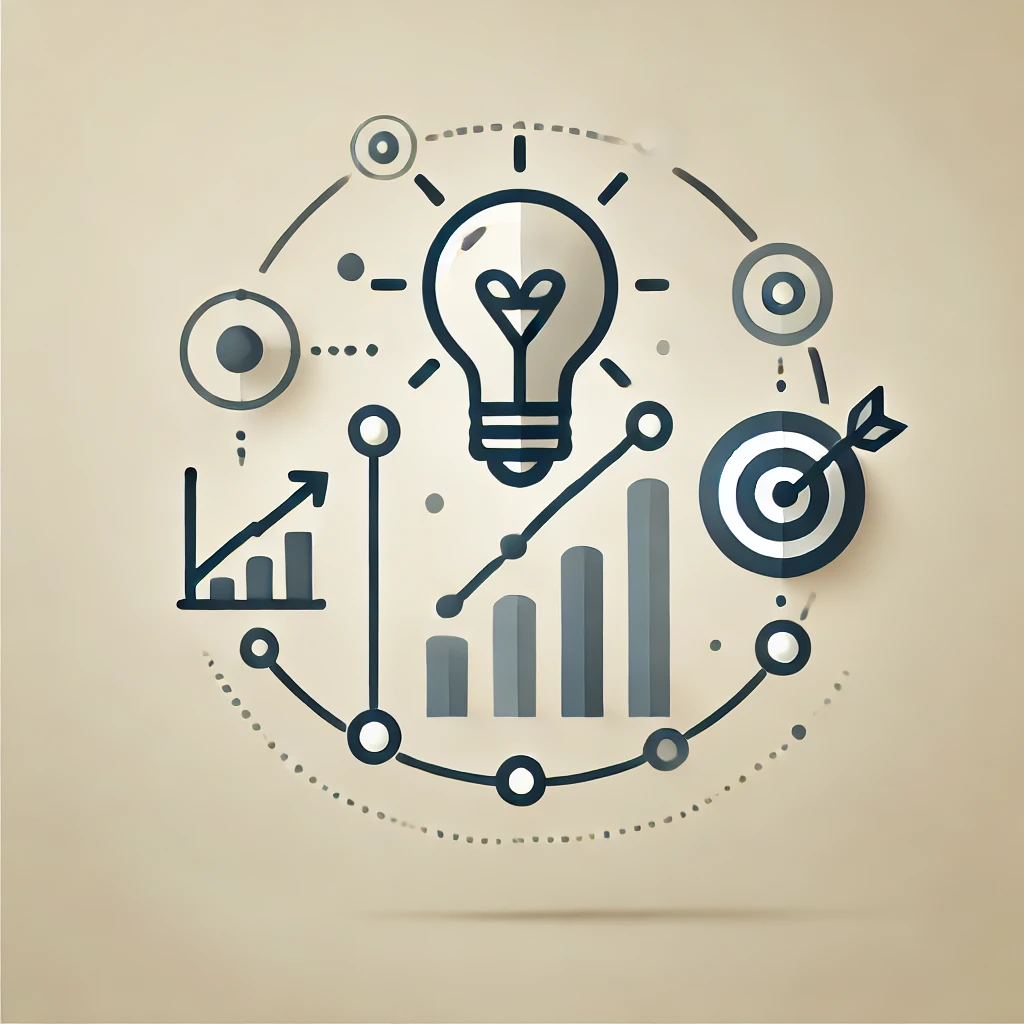