Creating a Sampling Frame for Consumer Research
The realization dawned on Neeraj during a quarterly review with a luxury fashion client. The marketing director was presenting disappointing results from an expensive campaign targeting "affluent millennials." Something about the demographics in their presentation seemed off to Neeraj. "How did you define your target population?" he asked. Her response unveiled the issue—their sampling frame had been constructed entirely from their loyalty program database, effectively missing the very prospects they most needed to reach. Their entire strategy had been based on insights from existing customers, overlooking the unconverted prospects who represented their true growth opportunity. What initially appeared to be a marketing execution failure was, in fact, a fundamental error in the sampling frame.
Introduction: The Critical Blueprint of Research Validity
The sampling frame serves as the operational definition of a market—the practical boundary that determines whose voices will be heard and whose will remain silent in consumer research. While less discussed than analytical techniques or survey design, the sampling frame fundamentally determines research validity and the strategic decisions that follow.
In today's fragmented market landscape, constructing appropriate sampling frames has grown increasingly complex. Digital transformation has simultaneously created new opportunities for precision while introducing unprecedented challenges in population definition and access. According to research from the Marketing Science Institute, organizations that master sampling frame development show 34% higher success rates in new market entry and 27% more accurate sales forecasting.
As noted by Harvard Business School professor Gerald Zaltman, "The most sophisticated analysis cannot compensate for a fundamentally flawed sampling frame. Who we choose to study defines the boundaries of what we can learn."
1. Defining the Population
Population definition forms the conceptual foundation of sampling frame development. This critical step translates abstract market segments into concrete parameters that guide participant selection.
From business objectives to population parameters
The translation from business objectives to population definition requires both analytical rigor and strategic clarity. Research by Bain & Company found that companies with explicitly documented population definitions achieve 29% higher alignment between research insights and strategic action.
Successful organizations develop specific, measurable population criteria. Procter & Gamble's "Consumer Closeness" program defines populations through multi-dimensional frameworks combining demographic, behavioral, attitudinal, and category usage parameters. This comprehensive approach helped them identify unexpected growth opportunities in their beauty division, capturing market share from competitors who defined their populations too narrowly.
The digital transformation has enabled more sophisticated population definition approaches. Netflix employs dynamic population definitions that combine declared characteristics with observed behaviors, creating what they term "actual viewing cohorts" rather than traditional demographic segments. This approach resulted in content development decisions that increased viewer engagement by 23%.
Balancing inclusion and exclusion criteria
Population boundaries involve both inclusion and exclusion decisions. Setting these boundaries too broadly creates noise and increases costs; defining them too narrowly limits generalizability and creates blind spots.
Retail giant Walmart employs tiered population definitions with primary, secondary, and aspirational consumer groups. This concentric approach helps them prioritize research investments while maintaining awareness of emerging consumer segments. Their framework includes clearly documented inclusion and exclusion criteria for each tier, facilitating consistent application across research initiatives.
In the digital era, exclusion criteria have gained importance as protection against specialized respondent groups. Research by ESOMAR found that "professional respondents" who participate in multiple studies can significantly skew results in digital environments. Leading companies now implement specific exclusion protocols to identify and filter these participants.
2. Sourcing Sample Lists
Converting population definitions into accessible participant lists represents a critical operational challenge. The digital transformation has revolutionized sourcing approaches while introducing new quality concerns.
Internal data sources
Customer databases provide rich internal sampling frames but require careful evaluation for representativeness. American Express employs a "coverage matrix" methodology that systematically identifies gaps in their customer database when used as a sampling frame. This approach revealed that their internal data overrepresented certain usage patterns while underrepresenting important emerging segments.
The integration of cross-channel data has enhanced internal sampling frame development. Sephora's unified customer view combines in-store purchases, online behavior, mobile app usage, and loyalty program participation to create comprehensive sampling frames. This integrated approach improved research representativeness by 41% compared to single-channel sampling frames.
Machine learning algorithms increasingly enhance internal sampling frame development. Target's customer analytics team employs propensity modeling to identify attitudinal and behavioral proxies within their first-party data, allowing more precise alignment between database segments and research populations.
External sampling sources
Market research panels, social media platforms, and specialized population access partners offer external sampling frame options with their own advantages and limitations.
Johnson & Johnson employs a tiered sampling source strategy based on research objectives. Their framework utilizes research panels for quantitative validation, social listening for trend identification, and specialized recruitment partners for hard-to-reach populations. This diversified approach reduced sampling costs by 24% while improving population coverage.
The digital revolution has created new specialized sampling sources. Amazon Mechanical Turk, Prolific Academic, and industry-specific platforms offer access to niche populations. Research in the Journal of Marketing found that specialized platforms provide higher quality responses for certain populations but require rigorous validation protocols.
Integration across sampling sources represents an emerging best practice. Unilever's "Connected Consumer" framework combines multiple sampling sources through standardized screening approaches and demographic verification, creating a unified sampling framework that transcends individual source limitations.
3. Managing Dropout and Duplication
Even perfectly constructed sampling frames face operational challenges in implementation. Participant dropout and duplication create particular concerns that sophisticated research organizations systematically address.
Strategies to minimize dropout rates
Participant attrition introduces potential bias and reduces statistical power. The Market Research Society found average research dropout rates have increased from 12% to 21% over the past decade, driven largely by mobile survey abandonment.
Leading organizations employ comprehensive dropout mitigation strategies. Nike's research team implements "respondent-centric design" principles that optimize participation burden, engagement techniques, and incentive structures based on population characteristics. This approach reduced their abandonment rates by 34% in longitudinal studies.
The mobile revolution has particularly impacted dropout dynamics. Samsung addresses this through adaptive survey design that automatically adjusts question presentation based on device characteristics and participation patterns, resulting in 27% higher completion rates on mobile devices.
Technology increasingly enables dropout prediction and prevention. Nestlé employs AI algorithms that identify potential dropout signals (response patterns, timing changes, demographic indicators) and trigger real-time interventions, reducing abandonment by 19% in their consumer insight panels.
Deduplication techniques across sources
Participant duplication across sources threatens data quality and statistical validity. This challenge has intensified in the digital era, with ESOMAR research indicating 17% overlap across major research panels.
Microsoft addresses this challenge through sophisticated digital fingerprinting techniques that identify repeat participants across platforms. Their multi-factor authentication approach examines device characteristics, connection parameters, and response patterns to detect duplication that simple identity matching might miss.
Blockchain technology offers emerging solutions for participant verification. Pharmaceutical company Pfizer is testing blockchain-based research participant registries that create secure, privacy-compliant verification systems across research initiatives, reducing duplication by 41% in early implementations.
Conclusion: The Strategic Imperative of Sampling Frame Excellence
As consumer markets grow increasingly complex and fragmented, excellence in sampling frame development becomes a critical strategic capability rather than merely a technical consideration. The most successful organizations recognize that who they study fundamentally determines what they can learn.
Artificial intelligence and machine learning will continue transforming sampling frame development. AI systems increasingly help identify population coverage gaps, suggest optimal sampling sources, and detect potential biases before they impact research outcomes.
Integration across data streams represents the next frontier. Organizations like Disney now develop unified sampling frameworks that connect consumer research participants with analytics from operational data, creating comprehensive representations that transcend traditional sampling limitations.
Call to Action
For marketing leaders committed to building stronger sampling frame capabilities:
- Document explicit population definitions for key market segments
- Audit current sampling sources against target population parameters
- Develop systematic validation protocols for sampling frame quality
- Invest in technology that enables more sophisticated participant verification
- Establish clear documentation standards for sampling frame limitations
The future belongs not just to those with the most data, but to those who most clearly understand exactly who that data represents—and the strategic boundaries that implies.
Featured Blogs
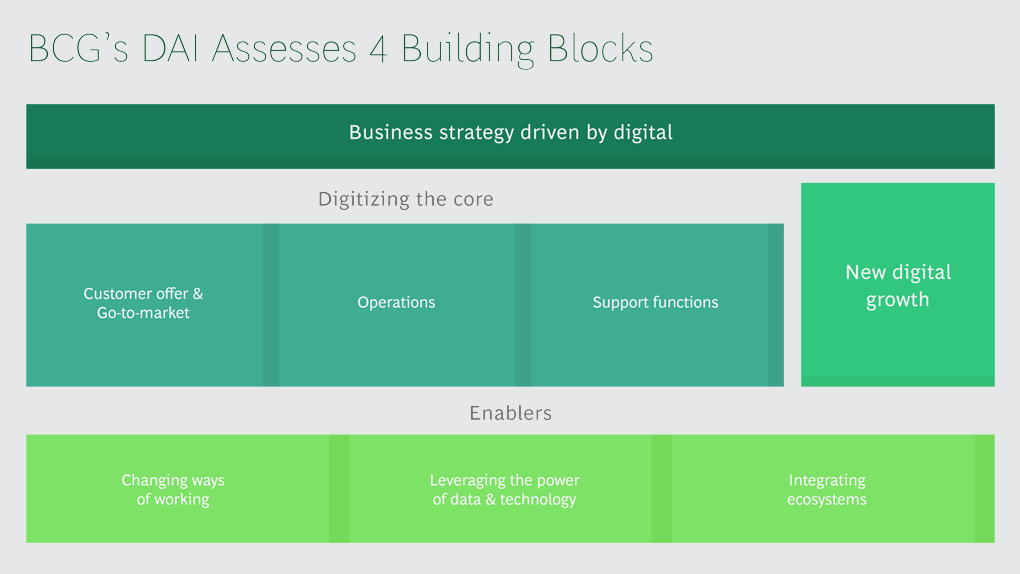
BCG Digital Acceleration Index
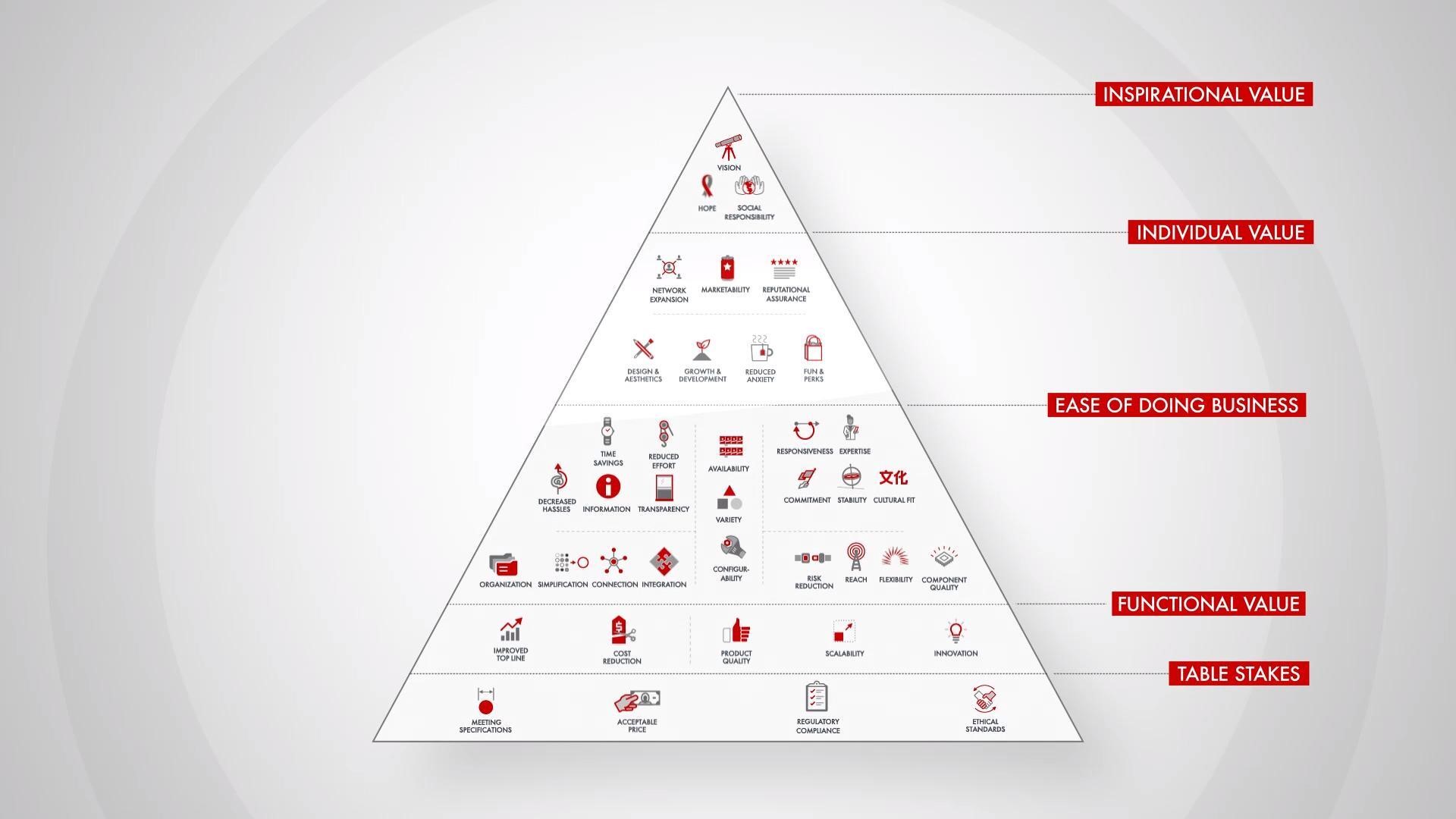
Bain’s Elements of Value Framework
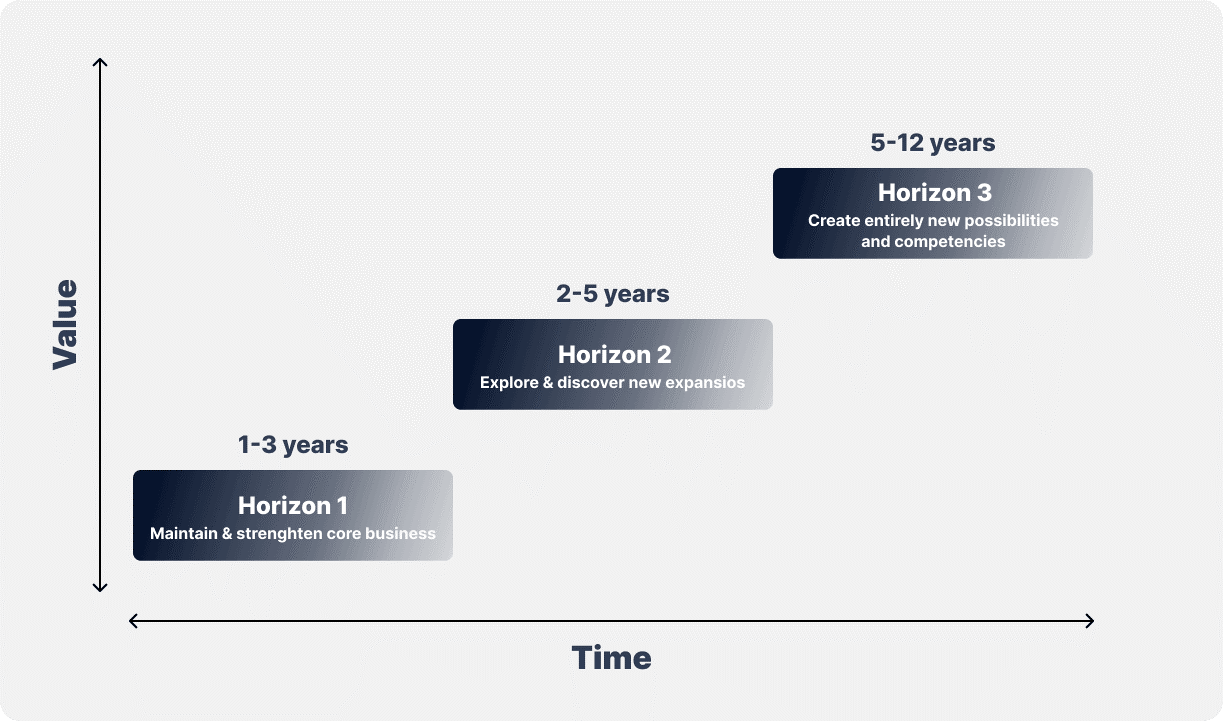
McKinsey Growth Pyramid
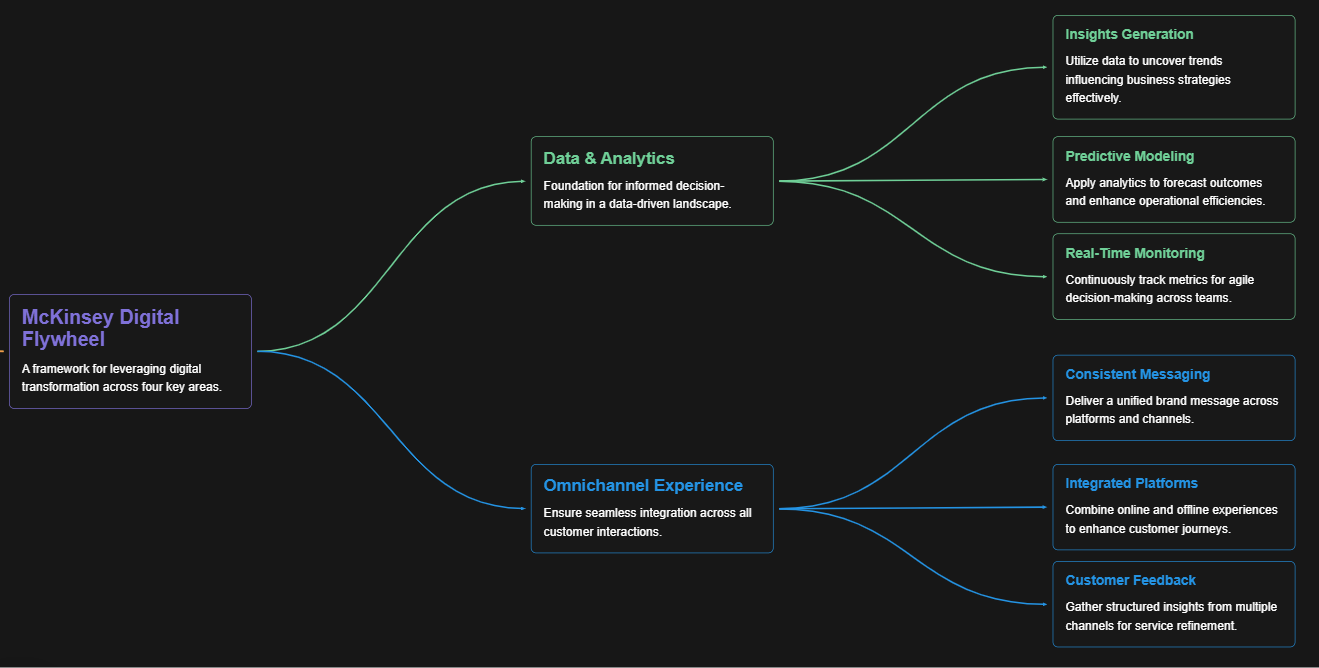
McKinsey Digital Flywheel
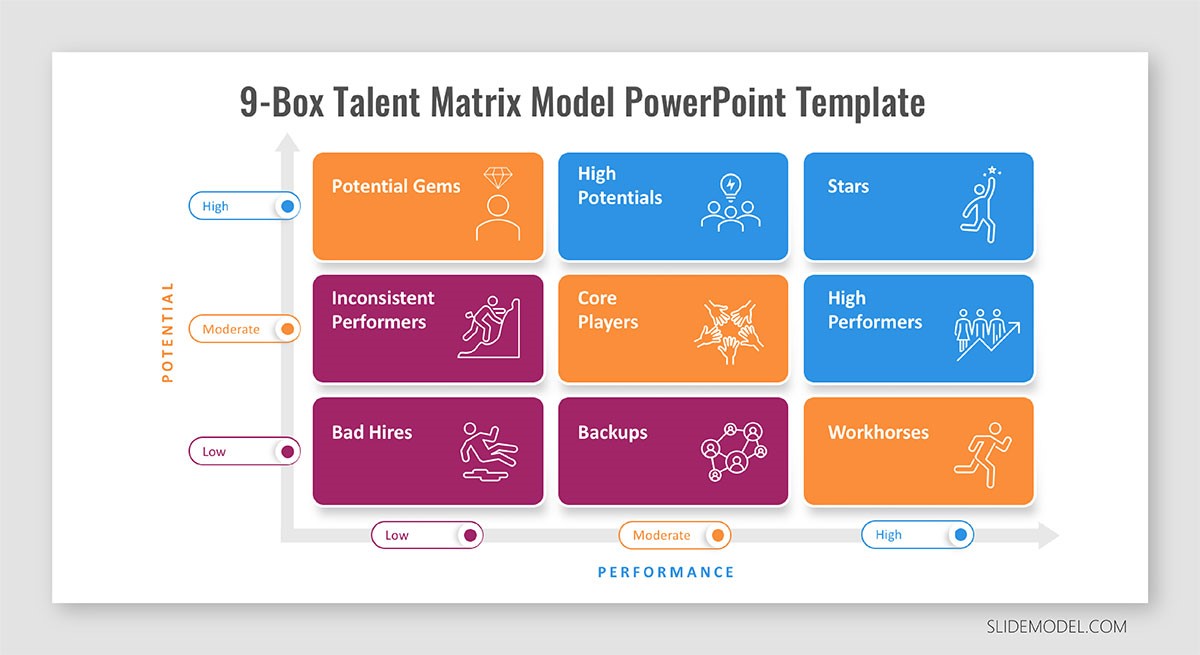
McKinsey 9-Box Talent Matrix
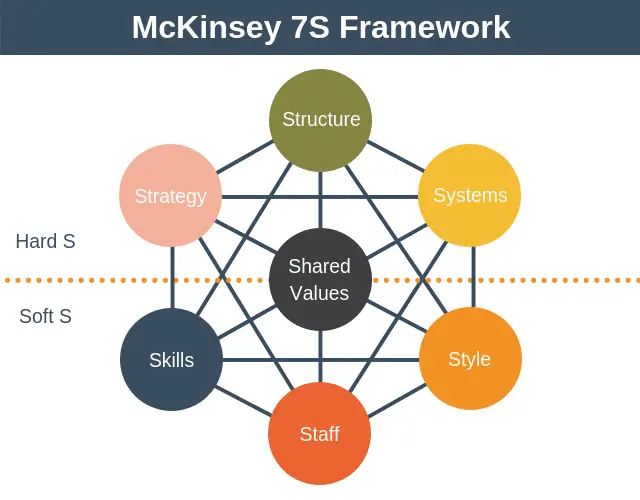
McKinsey 7S Framework
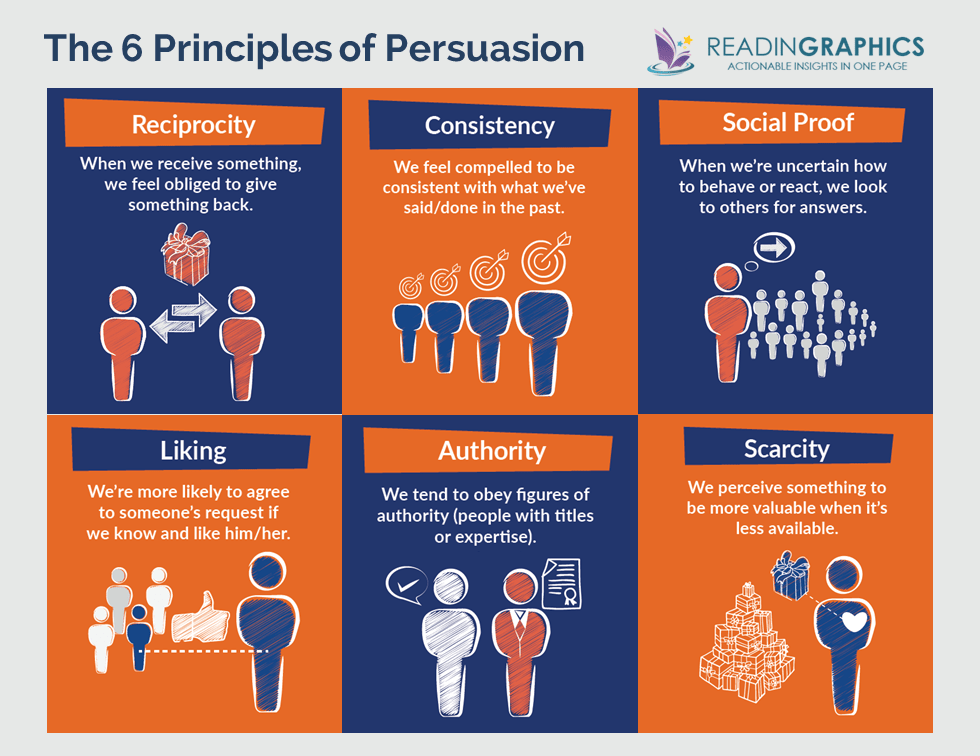
The Psychology of Persuasion in Marketing
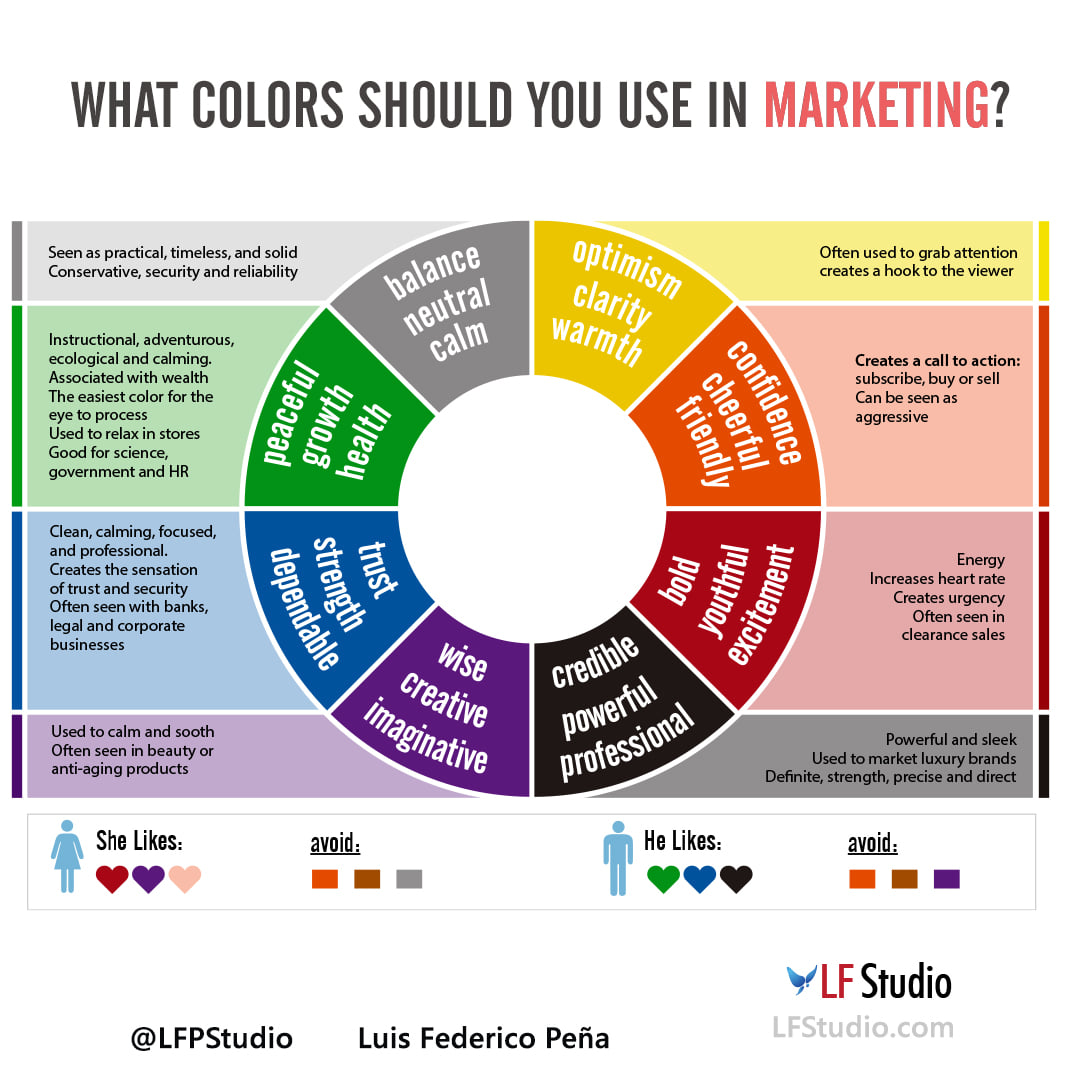
The Influence of Colors on Branding and Marketing Psychology
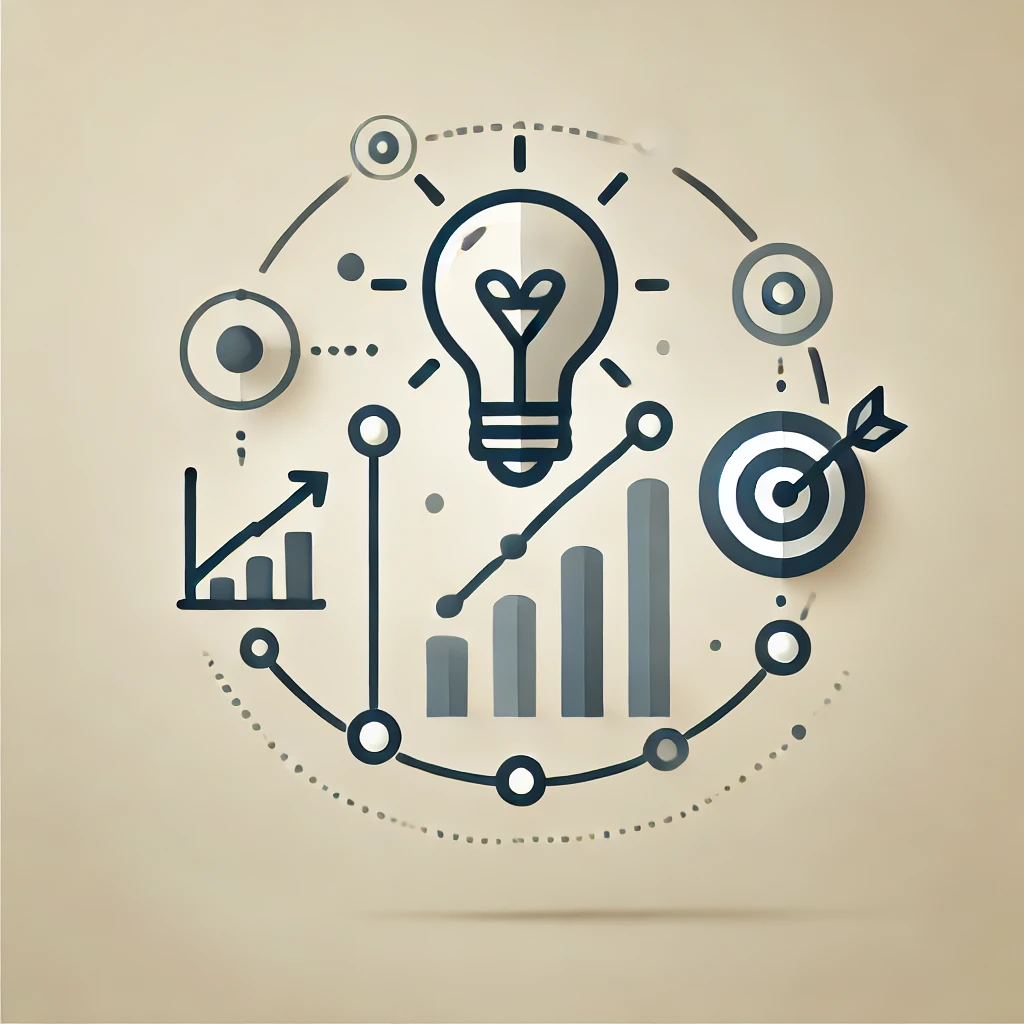