Digital Twins in Marketing: Simulating Consumer Behavior
Introduction: The Emergence of Virtual Consumer Replication
Marketing has always sought to understand consumer behavior, progressing from basic demographic profiling to sophisticated psychographic segmentation. Yet, these approaches have traditionally relied on aggregate data and retrospective analysis. A revolutionary advancement is emerging through digital twin technology—the creation of dynamic virtual replicas that simulate individual consumer behavior with unprecedented accuracy. Originally developed for industrial applications, digital twins now represent one of marketing's most promising frontiers. Gartner predicts that by 2026, 60% of enterprise organizations will use digital twins to optimize customer experience design, up from less than 10% in 2022. This remarkable growth reflects the technology's capacity to transform marketing from reactive analysis to proactive simulation. As marketing strategist Seth Godin argues, "Don't find customers for your products; find products for your customers"—a principle that digital twins operationalize through virtual behavioral modeling. This article examines how digital twin technology is reshaping marketing strategy, its applications across industries, implementation challenges, and future trajectories in an increasingly simulation-driven marketplace where understanding consumer behavior becomes a matter of algorithmic prediction rather than historical inference.
1. The Evolution of Digital Twin Technology in Marketing
Digital twins have evolved rapidly through several generations of increasing sophistication:
a) From Industrial Origins to Consumer Applications
The conceptual journey of digital twins crossed industries:
- Originally developed for physical assets in manufacturing and aerospace
- Adapted for urban planning and supply chain optimization
- Now applied to individual consumer behavior modeling
- Evolving toward ecosystem-level market simulations
b) Technical Foundations and Architectures
Contemporary consumer digital twins leverage multiple technologies:
- Agent-based models incorporating behavioral economics principles
- Machine learning systems trained on individual interaction histories
- Reinforcement learning frameworks that adapt to changing preferences
- Integration with real-time data streams for continuous calibration
c) From Static Profiles to Dynamic Simulation
The representation of consumers has become increasingly sophisticated:
- First-generation systems relied on rules-based customer personas
- Modern approaches incorporate probabilistic decision-making models
- Advanced twins simulate emotional states and cognitive biases
- Next-generation systems will incorporate neurologically-informed decision models
2. Strategic Applications Across the Marketing Value Chain
Forward-thinking brands implement digital twins throughout the customer journey:
a) Product Development and Innovation
Professor Clayton Christensen's jobs-to-be-done framework finds new expression in digital twins:
- Example: P&G leverages consumer digital twins to simulate product usage scenarios before physical prototyping
- Automotive companies like BMW create digital twin customers to test feature preferences virtually
b) Personalization and Experience Design
Research from the Harvard Business Review indicates that personalization can lift revenues by 10-15%:
- Example: Sephora's digital twin approach simulates individual customer reactions to product combinations
- Financial services firm USAA uses behavioral twins to optimize digital journey designs
c) Marketing Mix and Campaign Optimization
Digital twins enable unprecedented testing possibilities:
- Example: Unilever deploys market-level digital twins to simulate campaign performance across channels
- Streaming platform Netflix tests content recommendations against viewer digital twins
3. The Science of Consumer Simulation
Effective digital twins require sophisticated behavioral modeling frameworks:
a) Behavioral Economics and Choice Architecture
Nobel laureate Richard Thaler's work on choice architecture informs simulation design:
- Incorporation of cognitive biases and heuristics into decision models
- Simulation of status quo bias and loss aversion in purchase decisions
- Modeling of temporal discounting in subscription and loyalty scenarios
b) Contextual and Environmental Factors
Behavioral context significantly influences simulation accuracy:
- Incorporation of social influence and network effects
- Situational variables including time pressure and decision complexity
- Emotional state modeling as decision modifiers
c) From Reactive to Predictive Behavioral Modeling
Advanced systems move beyond mimicry to anticipation:
- Simulation of preference evolution over time
- Prediction of category switching and brand exploration behavior
- Anticipation of life stage transitions and resulting behavior changes
4. Case Studies: Digital Twins in Marketing Practice
Several pioneering implementations demonstrate practical applications:
a) Walmart's Supply Chain Optimization
Walmart created digital twins of customer segments to simulate purchasing behavior under various scenarios, allowing them to optimize inventory placement and reduce stockouts by 30% while simultaneously decreasing overall inventory costs.
b) L'Oréal's Virtual Product Testing
L'Oréal developed digital twins of skincare customers to simulate reactions to ingredients and formulations, reducing physical testing requirements while accelerating product development cycles by 35% and increasing new product success rates.
c) American Express's Service Journey Simulation
American Express implemented behavioral digital twins to optimize customer service pathways, testing multiple interaction approaches virtually before implementation, resulting in a 25% reduction in resolution time and increased satisfaction scores.
5. Implementation Challenges and Ethical Considerations
Several obstacles affect digital twin implementation:
a) Data Integration and Quality Challenges
Realistic simulation demands comprehensive data foundations:
- Requirements for cross-channel behavioral data integration
- Privacy-preserving techniques for sensitive personal information
- Methods for addressing data gaps and biases in underrepresented segments
b) Ethical Frameworks and Transparency
Marketing ethicist Mary Gentile emphasizes responsible simulation practices:
- Explicit consumer consent for behavioral modeling
- Transparency in how digital twins influence marketing decisions
- Safeguards against manipulative applications of behavioral insights
c) Organizational Capabilities and Culture
Technical implementation requires organizational adaptation:
- Cross-functional collaboration between data science and marketing
- New metrics frameworks for simulation accuracy and business impact
- Cultural shifts toward experimentation and scenario planning
Conclusion: The Future of Simulated Consumer Understanding
Digital twins in marketing represent a fundamental shift in consumer understanding—moving from descriptive analytics to prescriptive simulation. As marketing scholar Philip Kotler observes, "The art of marketing is the art of brand building. If you are not a brand, you are a commodity." Digital twins enable this brand building through unprecedented customer understanding, allowing marketers to anticipate needs rather than merely respond to them. As the technology matures, we can expect increasing integration with other advanced technologies including augmented reality, the metaverse, and neuroinformatics. Organizations that master digital twin implementation—balancing technological sophistication with ethical responsibility and human insight—will gain significant advantages in predictive customer understanding, personalization at scale, and precision in marketing resource allocation. The marketing future belongs to those who can simulate it most accurately.
Call to Action
For marketing leaders exploring digital twin technology:
- Begin with a focused use case that addresses a specific high-value marketing challenge
- Develop clear ethical guidelines and consumer transparency policies before implementation
- Create cross-functional teams that combine behavioral science expertise with data engineering
- Implement progressive data integration strategies that respect privacy while building simulation capabilities
- Establish rigorous validation frameworks comparing simulation predictions with actual outcomes
Featured Blogs
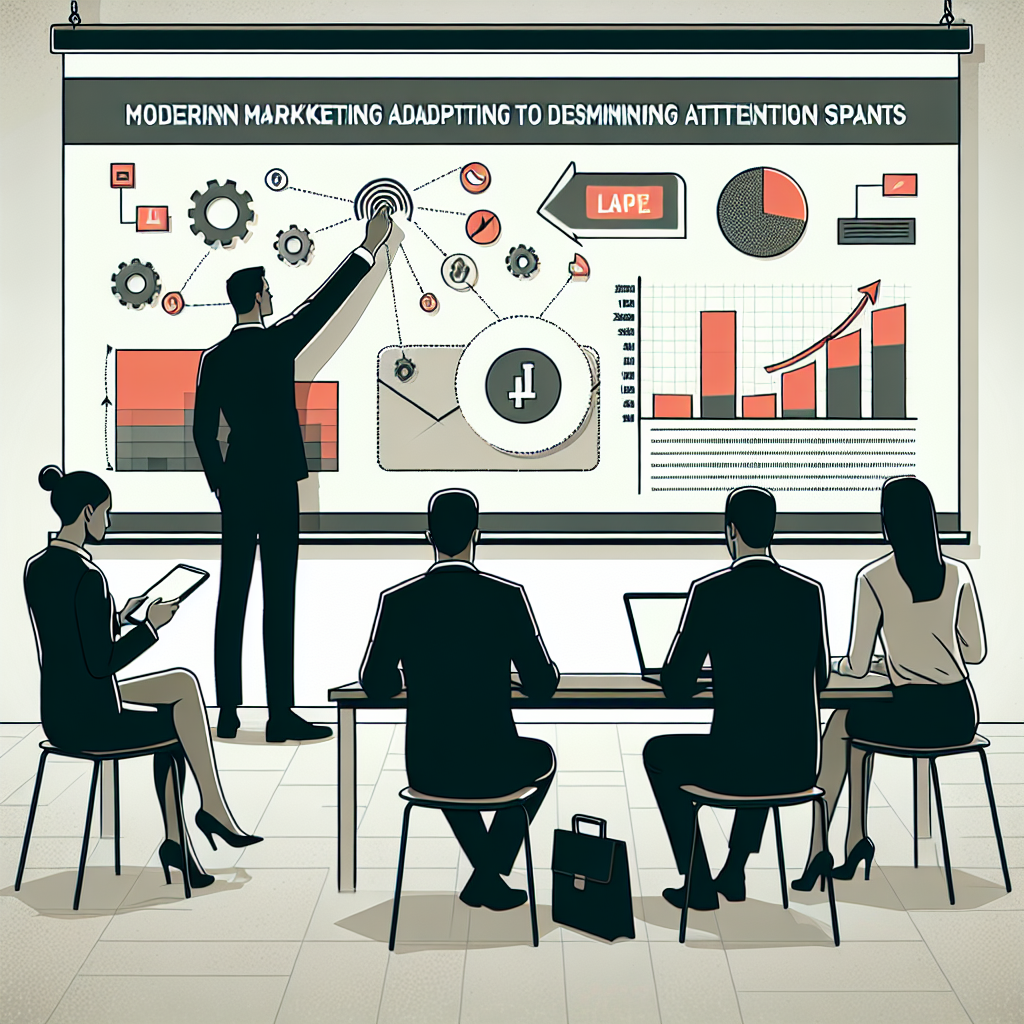
How the Attention Recession Is Changing Marketing
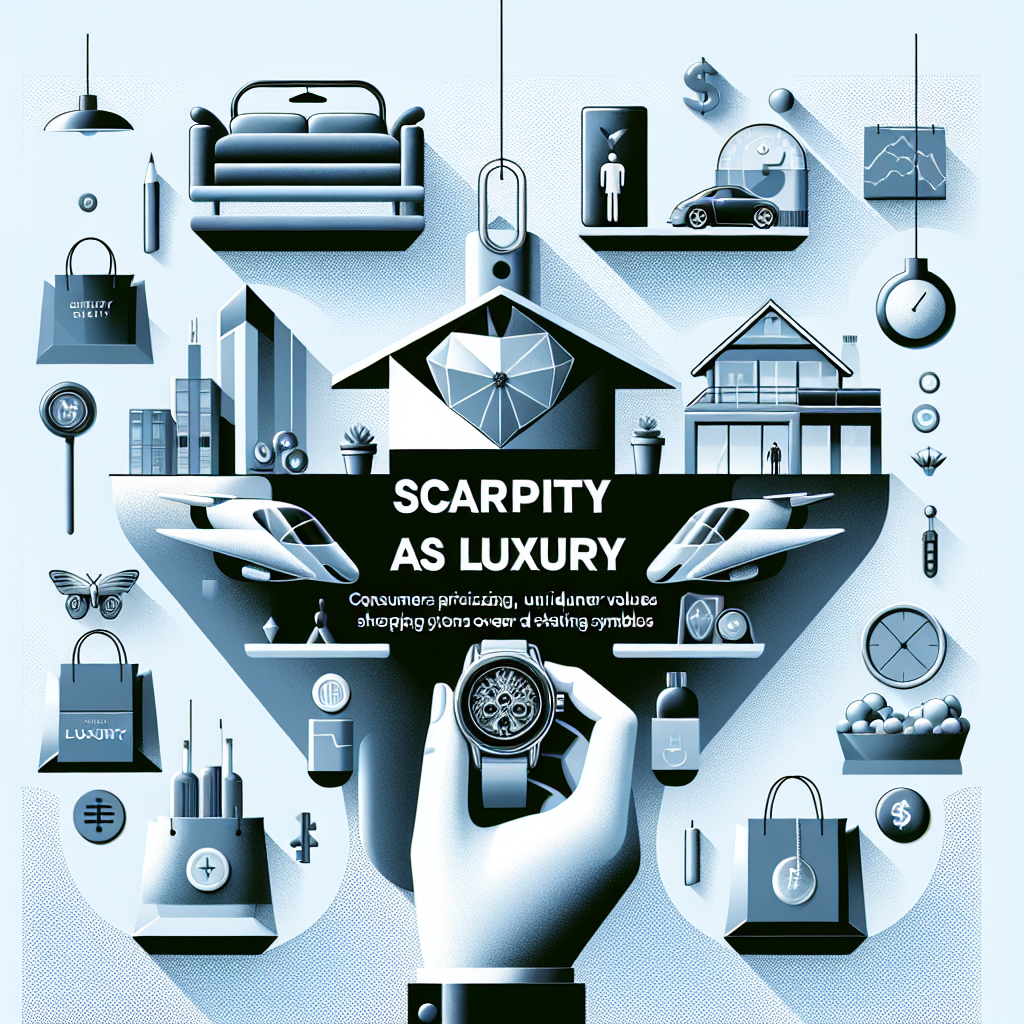
The New Luxury Why Consumers Now Value Scarcity Over Status
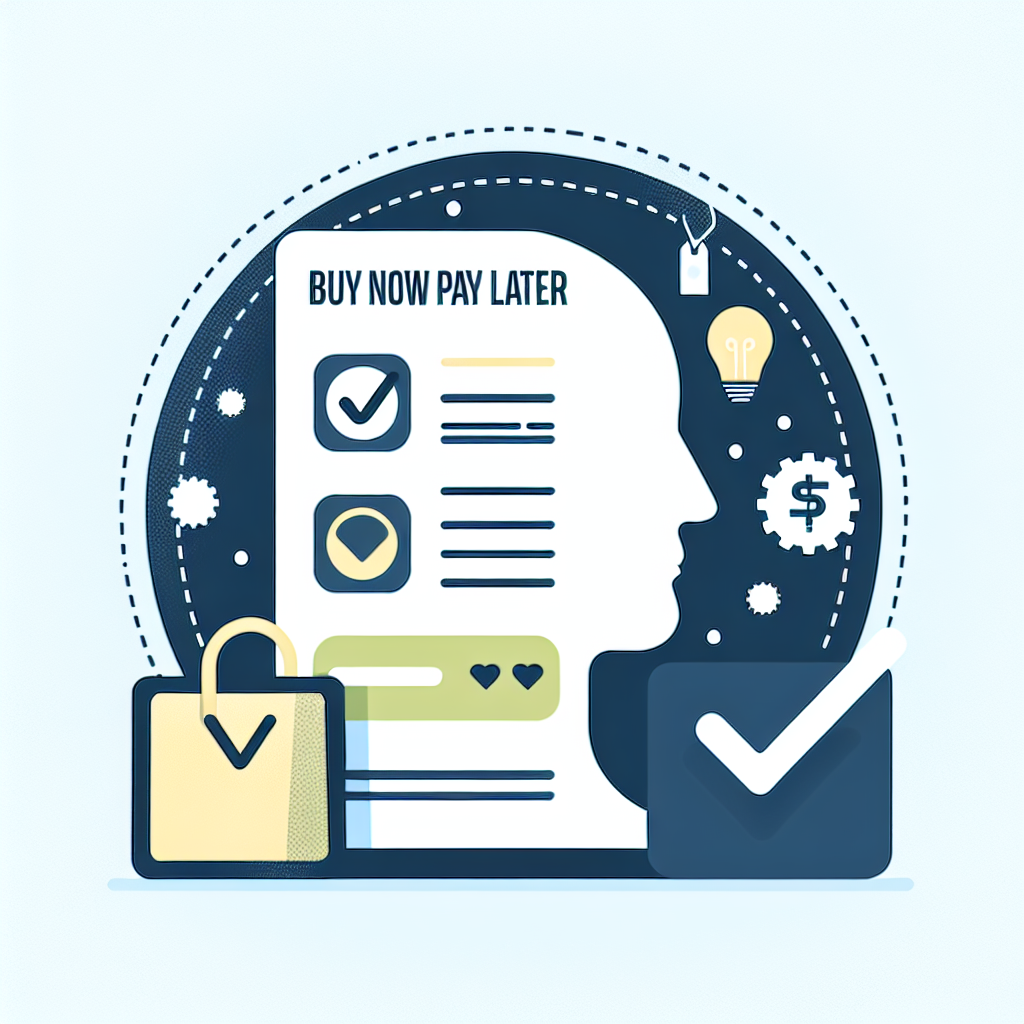
The Psychology Behind Buy Now Pay later
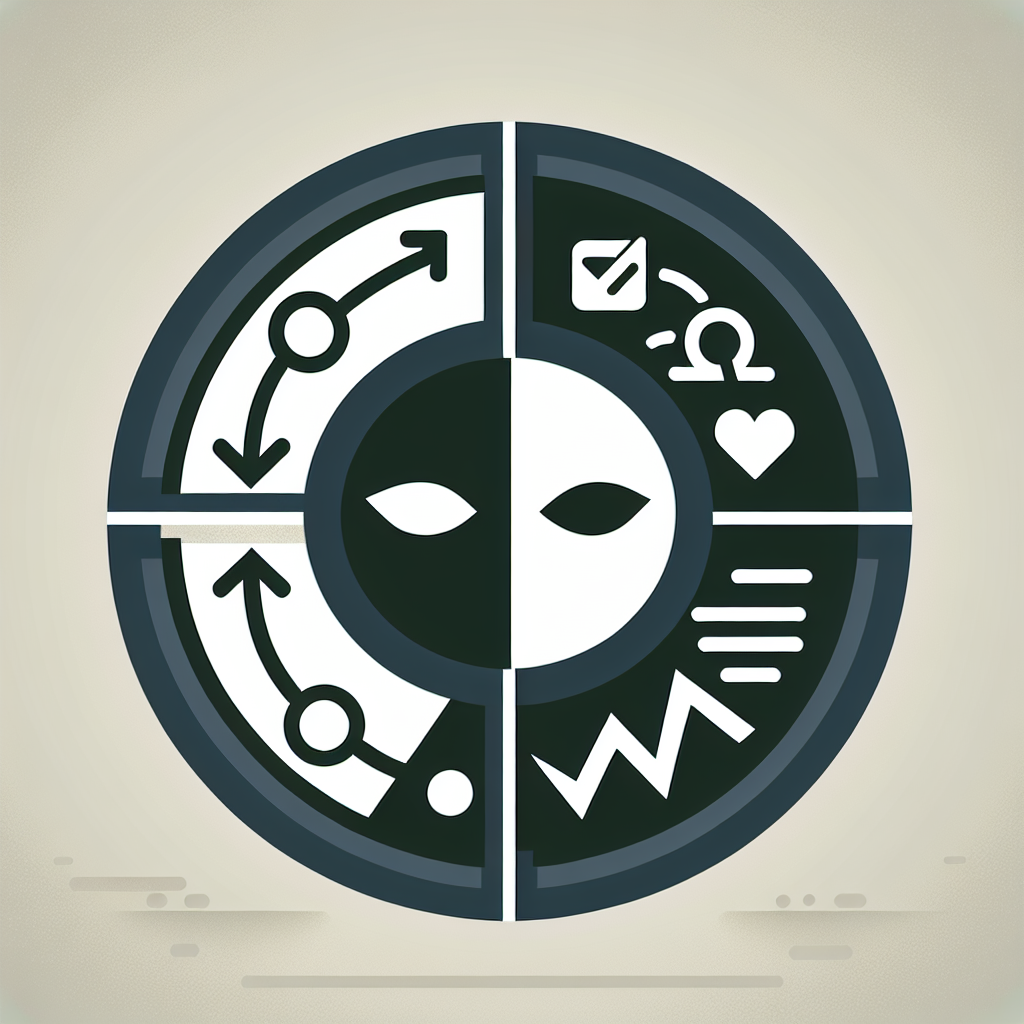
The Role of Dark Patterns in Digital Marketing and Ethical Concerns
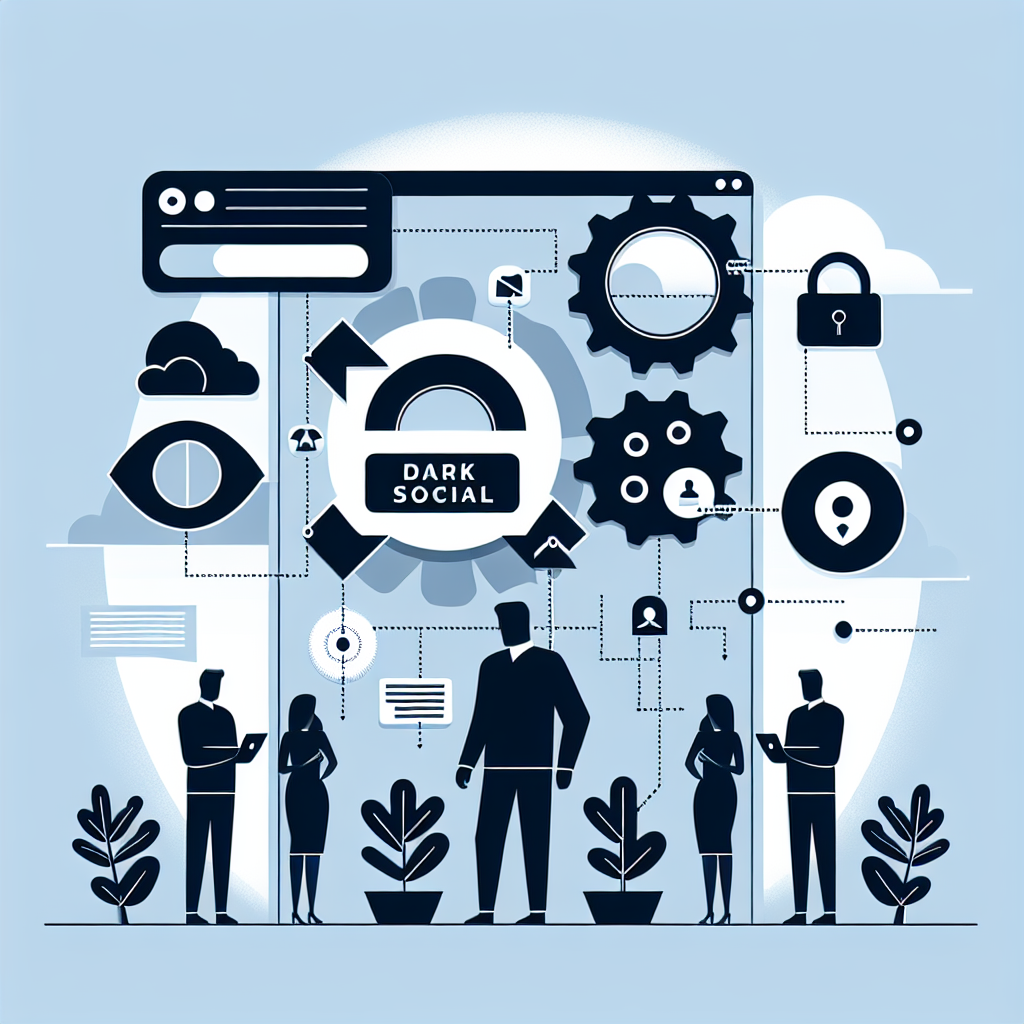
The Rise of Dark Social and Its Impact on Marketing Measurement
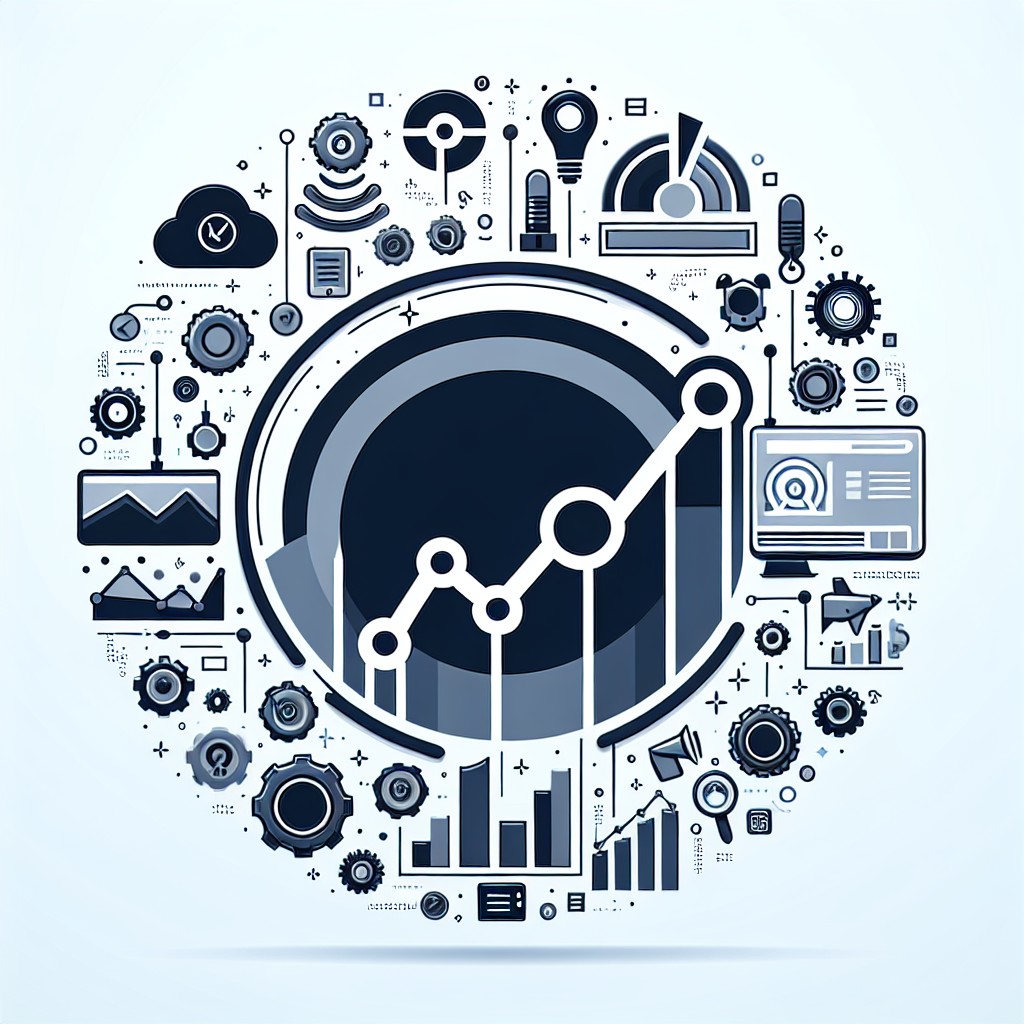