BCG AI@Scale Framework
Artificial Intelligence (AI) has become an indispensable force in transforming industries, revolutionizing operations, and enhancing customer experiences. However, many companies struggle to move beyond pilot projects and integrate AI into their core operations at scale. Recognizing this challenge, the Boston Consulting Group (BCG) developed the AI@Scale Framework—a comprehensive model designed to guide organizations in effectively integrating AI into their business strategies.
The BCG AI@Scale Framework emphasizes four key pillars:
- AI Strategy & Vision
- AI Talent & Culture
- Technology & Data Infrastructure
- Scaling AI Use Cases
Together, these components help organizations harness AI’s full potential, drive significant business value, and maintain a competitive edge in today’s digital world.
The BCG AI@Scale Framework is built on the premise that successfully leveraging AI goes beyond technology—it requires a holistic approach encompassing strategy, people, infrastructure, and application. While many organizations experiment with AI through isolated projects, achieving real value comes from scaling AI across the enterprise.
This framework helps businesses:
- Develop a clear AI vision aligned with business objectives
- Foster a culture and talent pool conducive to AI adoption
- Establish robust technology and data foundations
- Expand successful AI use cases across the organization
- By addressing these four pillars, companies can avoid common pitfalls, overcome barriers to scaling, and realize sustainable, long-term benefits from their AI initiatives.
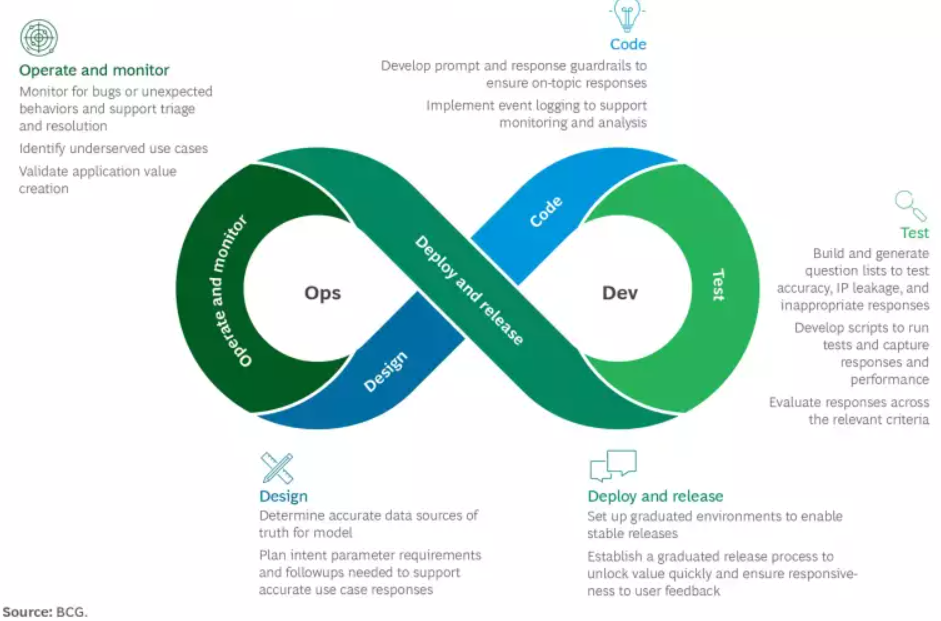
1. AI Strategy & Vision
Without a clear strategy and vision, AI initiatives often lack direction and fail to deliver meaningful impact. A well-defined AI strategy aligns AI investments with business goals, ensuring that AI solutions address high-priority challenges and opportunities.
Key Elements of an Effective AI Strategy :
- Alignment with Business Goals: AI initiatives should support overarching business objectives, whether it’s improving customer experience, enhancing operational efficiency, or driving revenue growth.
- Prioritization of Use Cases: Identifying and prioritizing AI use cases with the highest potential impact ensures optimal resource allocation.
- Clear Governance Structure: Establishing leadership roles, accountability, and decision-making frameworks is crucial for coordinated efforts.
- Risk Management and Ethics: Addressing ethical considerations, data privacy, and potential risks associated with AI deployment is vital for long-term success.
Developing a Vision for AI :
An inspiring and well-communicated AI vision encourages organizational buy-in and provides a road map for adoption. This vision should articulate how AI will transform the organization and benefit employees, customers, and stakeholders.
2. AI Talent & Culture
Technology alone cannot drive successful AI integration; people and culture play a pivotal role. Organizations need skilled professionals to develop, deploy, and maintain AI solutions, as well as a culture that embraces data-driven decision-making.
Building the Right AI Talent Pool requires -
- Data Scientists: Develop machine learning models and analyze complex datasets.
- AI Engineers: Focus on the technical implementation of AI solutions.
- Data Engineers: Ensure data quality, integration, and pipeline management.
- AI Product Managers: Bridge the gap between technical teams and business stakeholders.
Organizations can attract and retain talent through competitive compensation, continuous learning opportunities, and a supportive work environment.
Fostering an AI-Friendly Culture -
A successful AI culture encourages experimentation, collaboration, and openness to change. Key cultural attributes include:
- Data-Driven Decision-Making: Employees at all levels should use data insights to guide decisions.
- Cross-Functional Collaboration: Teams from IT, operations, marketing, and other departments should work together to implement AI solutions.
- Continuous Learning: Offering AI literacy programs and training helps non-technical staff understand and adopt AI.
- Leadership Support: Senior leaders must champion AI initiatives and set the tone for cultural transformation.
3. Technology & Data Infrastructure
No AI initiative can succeed without a strong technology and data infrastructure. High-quality data, robust computing power, and scalable platforms are essential for developing and deploying AI solutions.
Foundation Data is the fuel for AI and for that Organizations must:
- Ensure Data Quality: Clean, consistent, and accurate data is critical for effective AI models.
- Break Down Data Silos: Centralized data storage enables better access and utilization.
- Implement Data Governance: Clear policies ensure data security, privacy, and compliance.
Technology Infrastructure Components -
- Cloud Computing: Offers scalable storage and computing power for AI applications.
- AI Platforms and Tools: Frameworks like TensorFlow, PyTorch, and cloud-based AI services accelerate development.
- MLOps (Machine Learning Operations): Facilitates the deployment, monitoring, and maintenance of AI models.
- Ensuring Scalability and Security: Infrastructure should be designed to scale with growing AI demands while maintaining cybersecurity measures to protect sensitive data.
4. Scaling AI Use Cases
Many companies successfully run AI pilot projects but fail to scale them across the organization. Scaling AI use cases involves taking successful pilots and implementing them across relevant departments, regions, or product lines.
Steps to Scale AI Use Cases :
- Identify High-Impact Use Cases: Focus on AI applications with measurable business value.
- Create Repeatable Processes: Develop frameworks to replicate successful pilots in different areas.
- Ensure Cross-Department Collaboration: Scaling often requires input from various functions, including IT, operations, and marketing.
- Invest in Change Management: Prepare employees for new processes and technologies through communication and training.
- Monitor and Optimize: Continuously assess the performance of scaled solutions and refine them as needed.
Examples of Scalable AI Use Cases:
- Customer Service: AI chatbots and virtual assistants can be rolled out across multiple customer support channels.
- Supply Chain Optimization: AI models predicting demand can be applied to various regions and product lines.
- Marketing Personalization: AI-driven content recommendations can be expanded across platforms and customer segments.
- Predictive Maintenance: In manufacturing, AI can be used to anticipate equipment failures across multiple facilities.
Challenges and Solutions in Implementing the AI
Common Challenges:
- Data Quality and Accessibility: Poor data quality can undermine AI efforts.
- Talent Shortages: Finding skilled AI professionals is often challenging.
- Resistance to Change: Employees may resist adopting new technologies.
- Integration with Legacy Systems: Older systems may not support modern AI solutions.
- Ethical and Regulatory Concerns: Compliance with privacy laws and ethical standards is essential.
Solutions:
- Invest in Data Management: Develop strong data governance policies.
- Upskill Existing Workforce: Provide training to bridge the talent gap.
- Communicate Benefits Clearly: Help employees understand how AI improves their work.
- Use Middleware Solutions: Facilitate integration with existing systems.
- Prioritize Ethical AI: Develop guidelines to ensure responsible AI usage.
Final Thoughts
The BCG AI@Scale Framework offers a comprehensive road-map for organizations seeking to unlock the full potential of AI. By focusing on the four pillars—AI Strategy & Vision, AI Talent & Culture, Technology & Data Infrastructure, and Scaling AI Use Cases—businesses can move beyond isolated experiments and achieve enterprise-wide transformation.
Implementing this framework requires commitment, collaboration, and continuous learning. While challenges exist, the rewards of successfully scaling AI—enhanced efficiency, improved customer experiences, and sustained competitive advantage—are well worth the effort.
In a world where technological advancements shape the future, companies that adopt the BCG AI@Scale Framework position themselves to thrive in the digital age.
Featured Blogs
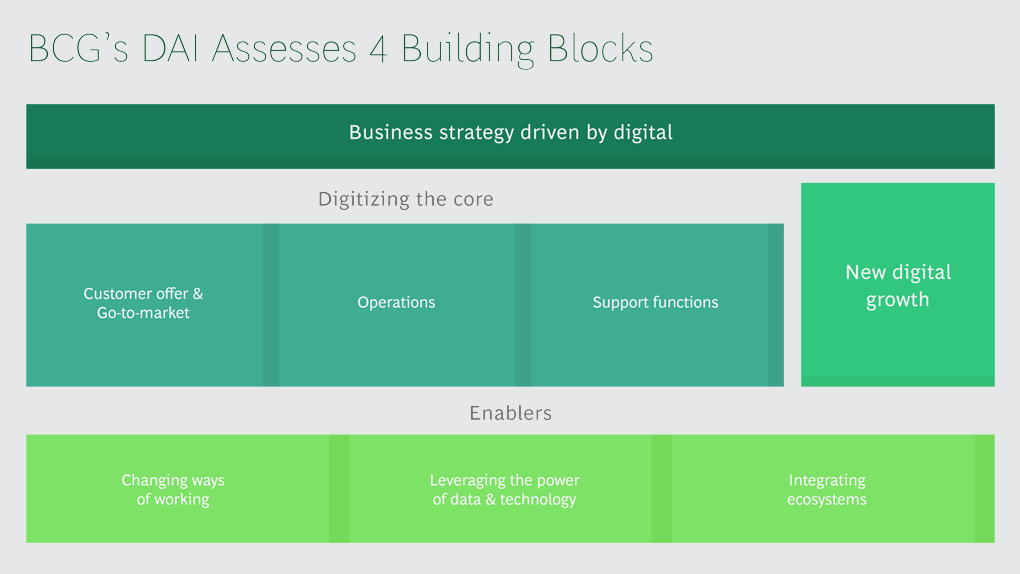
BCG Digital Acceleration Index
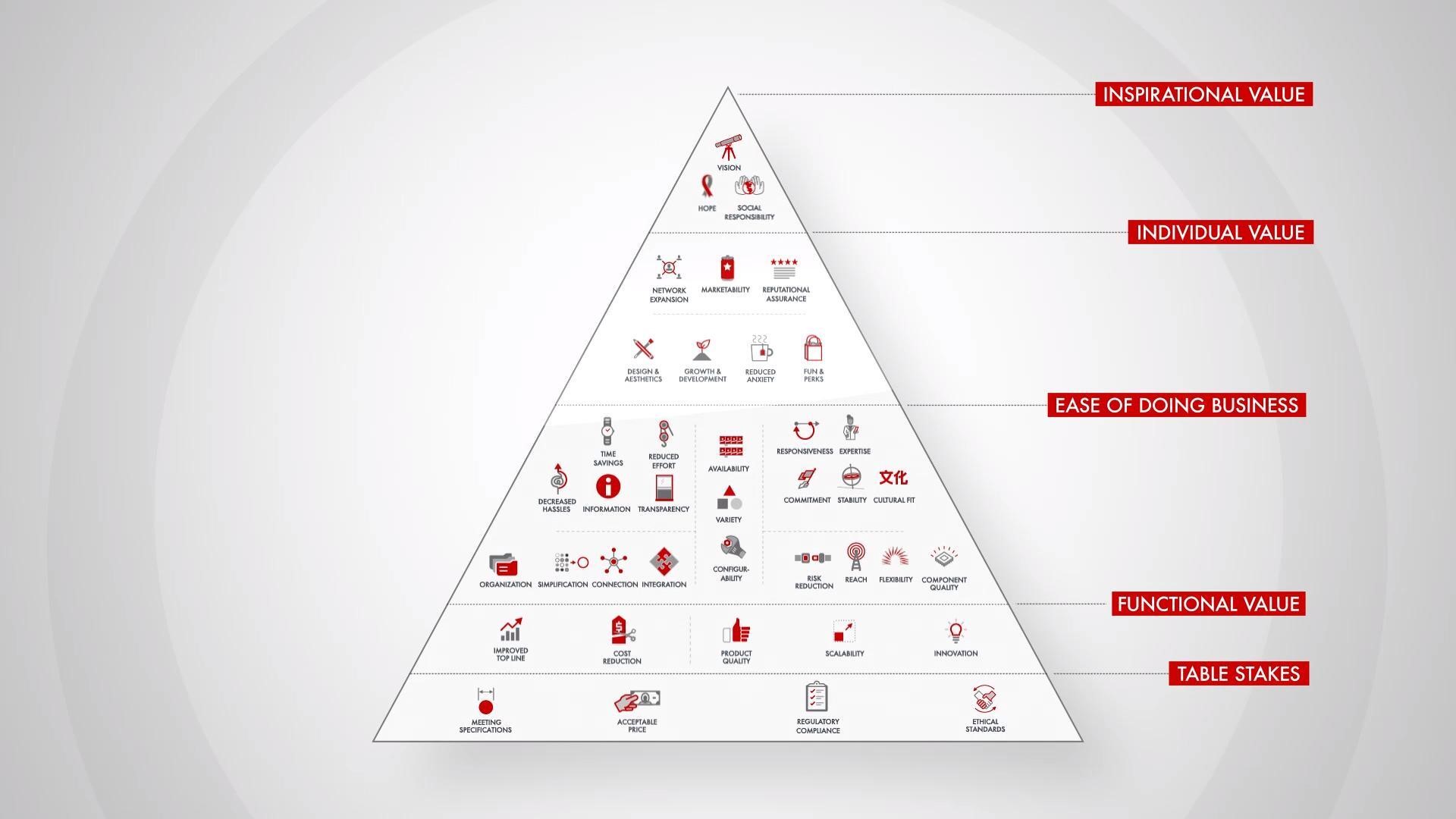
Bain’s Elements of Value Framework
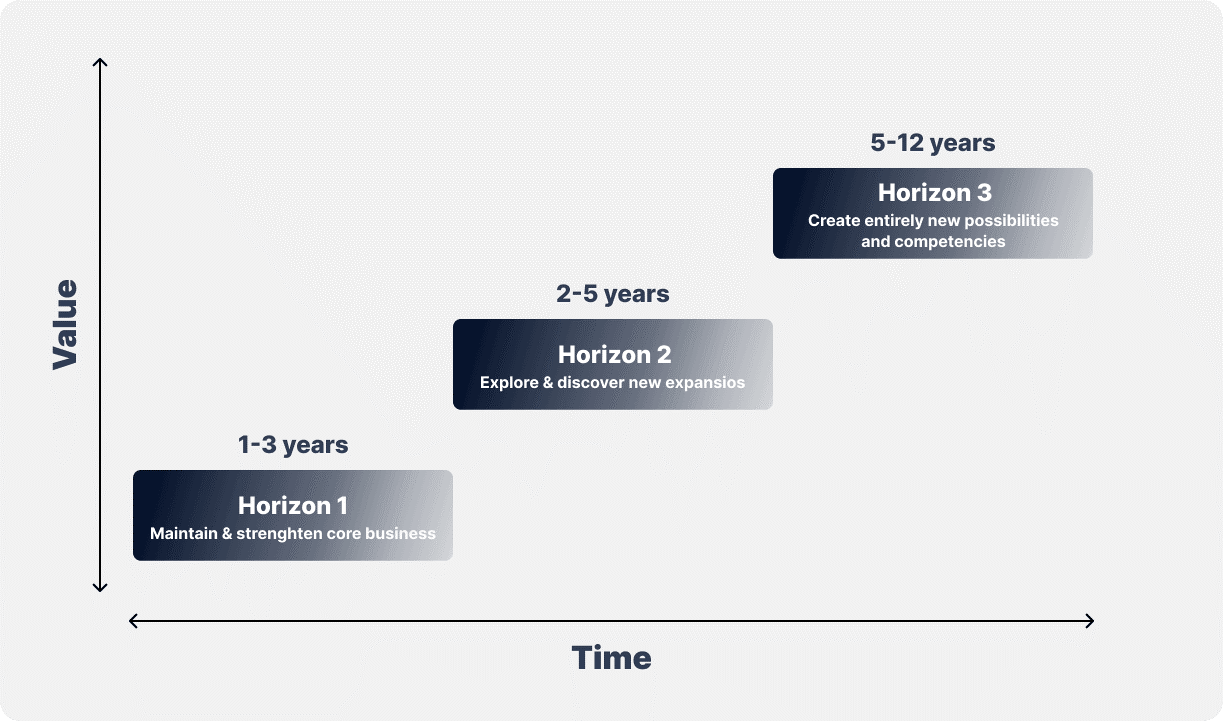
McKinsey Growth Pyramid
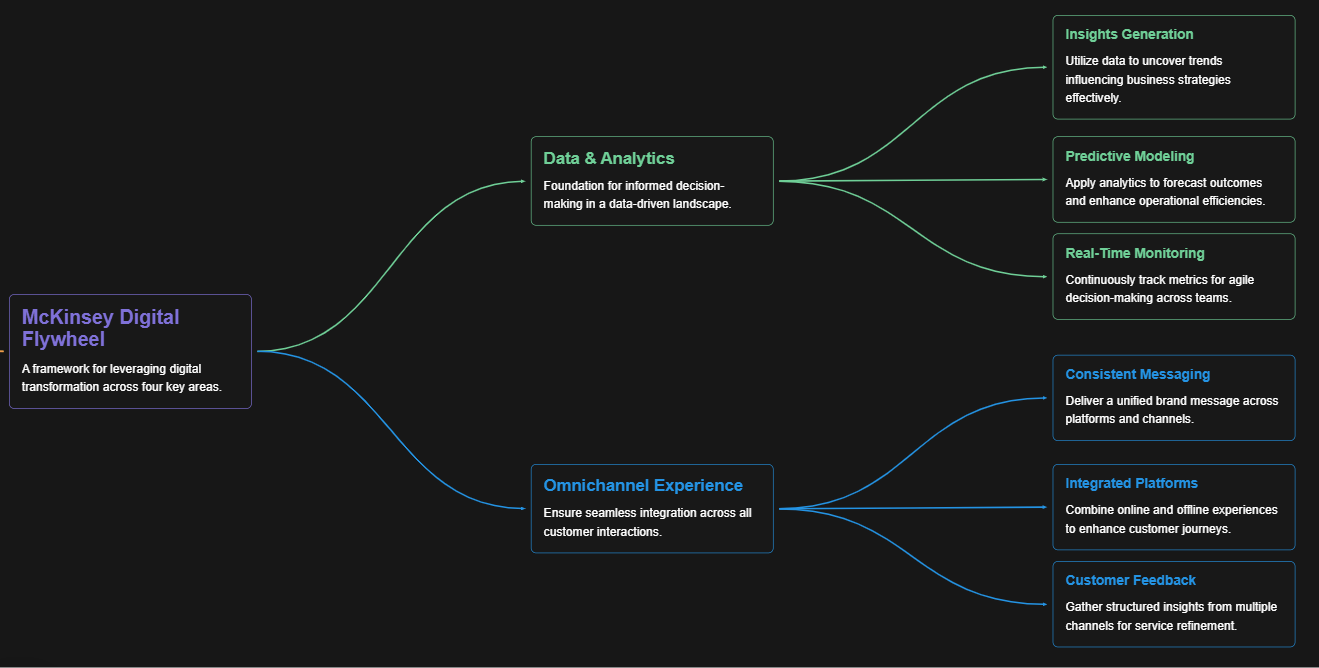
McKinsey Digital Flywheel
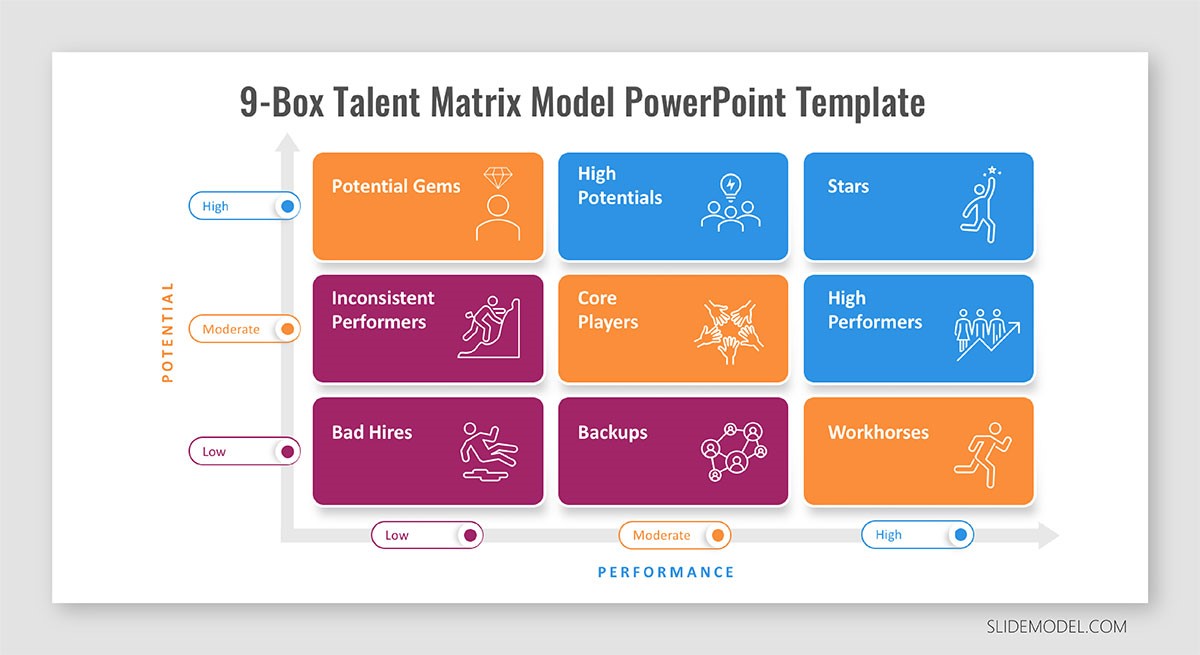
McKinsey 9-Box Talent Matrix
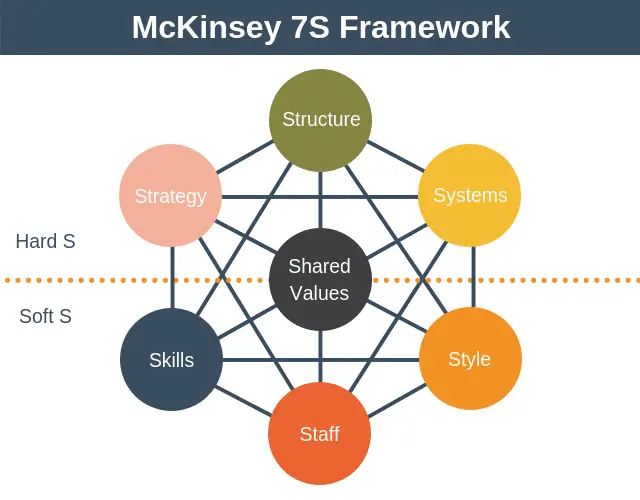
McKinsey 7S Framework
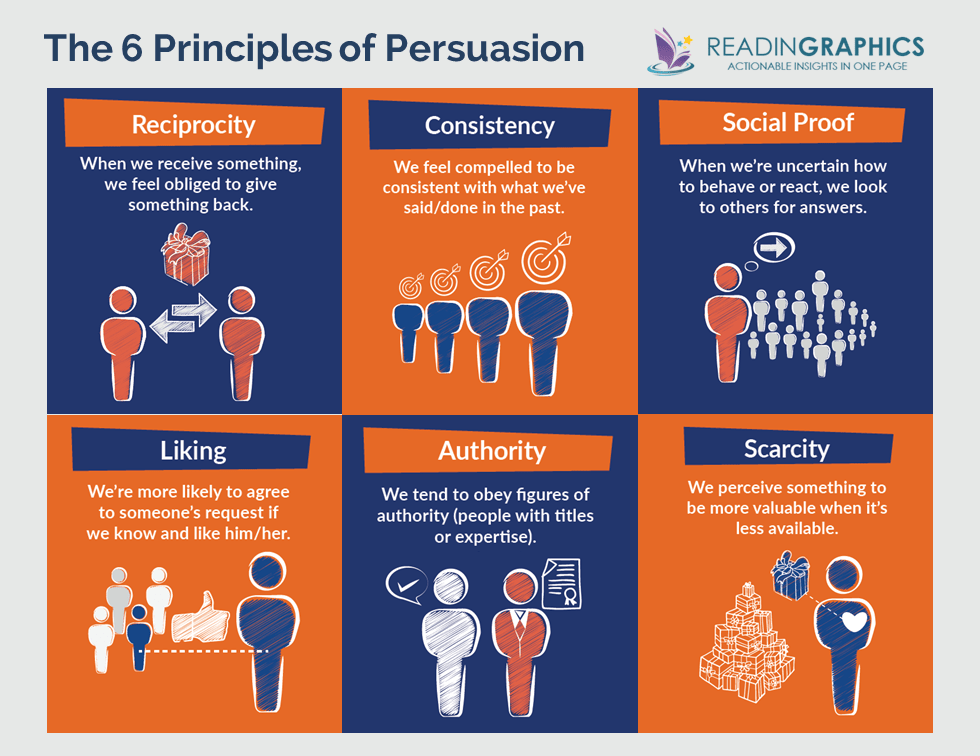
The Psychology of Persuasion in Marketing
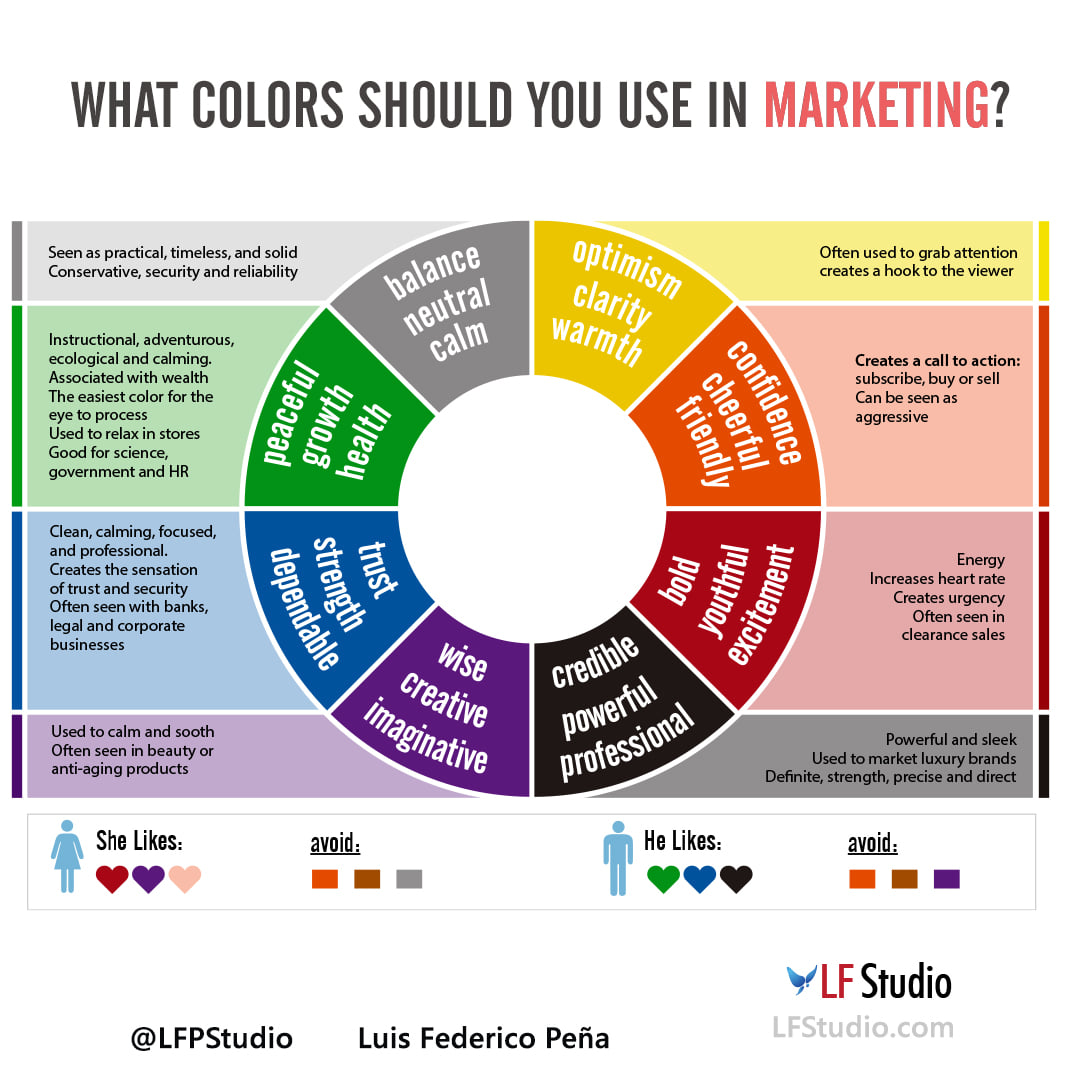
The Influence of Colors on Branding and Marketing Psychology
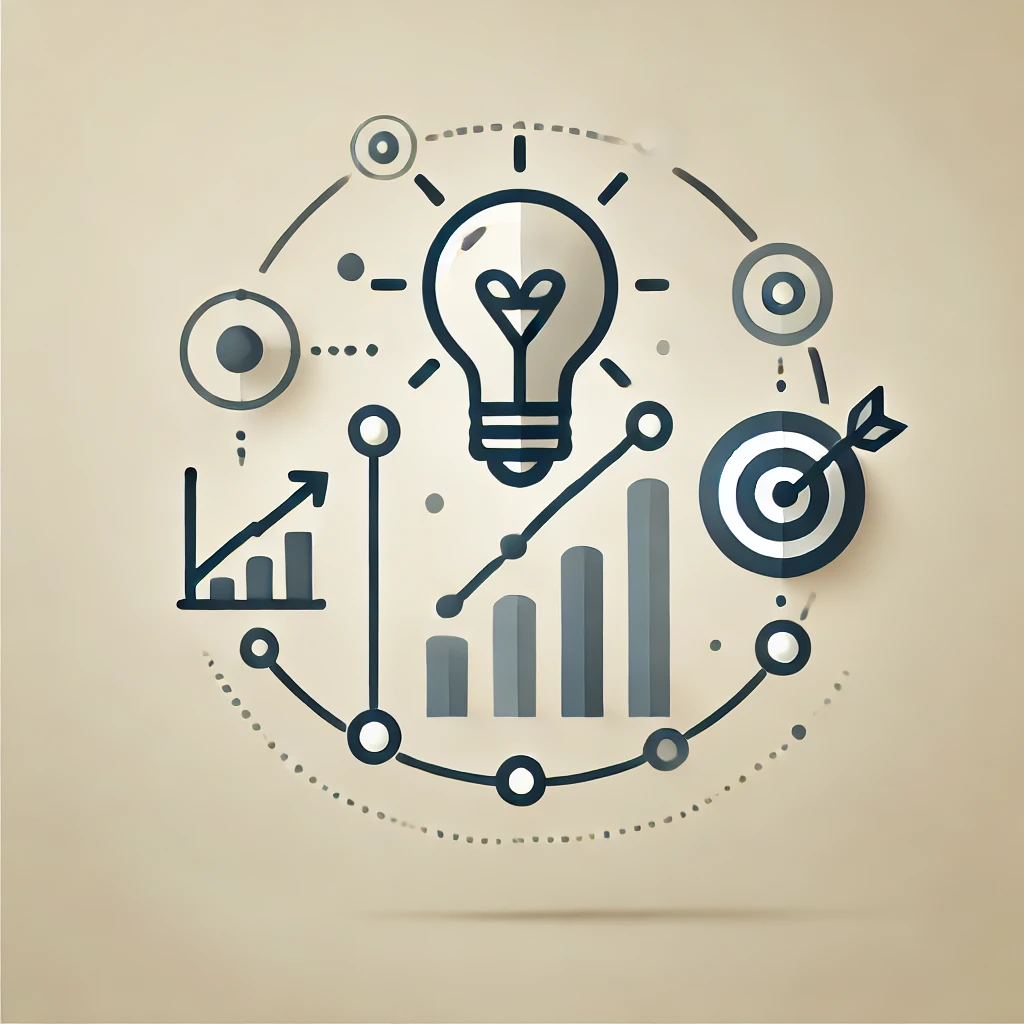